The National Association of Manufacturers has recently performed research, and they reveal that 76% of manufacturing executives believe data analytics to be essential to gaining a competitive advantage.
Furthermore, the adoption of predictive analytics in the manufacturing sector is projected to grow at a CAGR of 25.2% from 2021 to 2026, indicating a significant industry shift towards leveraging data-driven insights.
In today's data-driven world, manufacturers are increasingly turning to predictive and prescriptive analytics to optimize their processes, reduce costs, and improve overall efficiency.
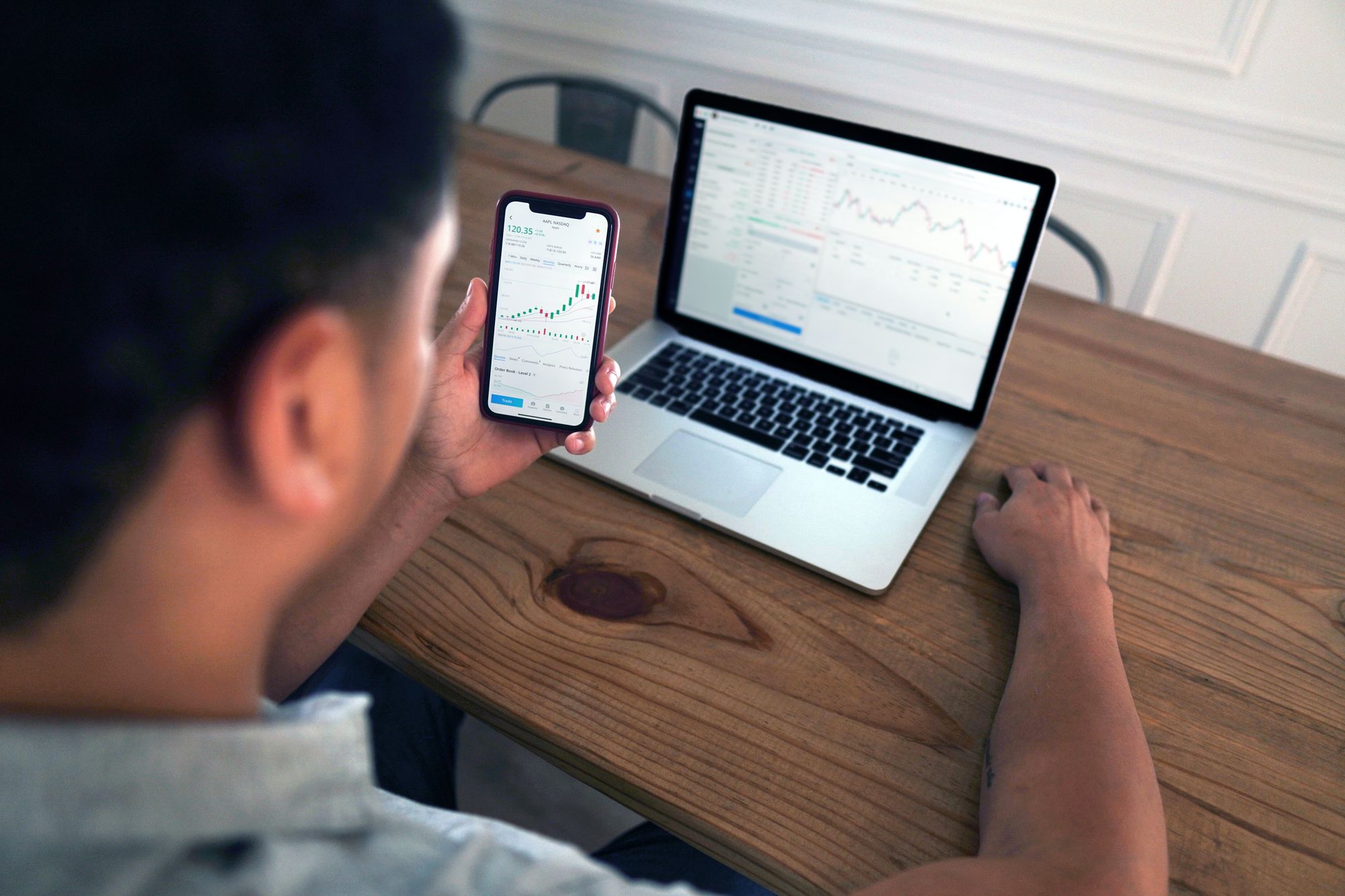
Predictive analytics involves using historical data, statistical algorithms, and machine learning techniques to forecast future outcomes and trends. By analyzing vast amounts of data, manufacturers can identify patterns, predict equipment failures, optimize maintenance schedules, and streamline production processes. This enables proactive decision-making, reduces downtime, and enhances overall operational efficiency.
On the other hand, prescriptive analytics takes predictive analytics a step further by providing actionable recommendations and insights. It considers various scenarios and constraints to optimize decision-making processes and guide manufacturers toward the most effective courses of action.
In this article, we will delve deeper into the applications, methodologies, and best practices of predictive and prescriptive analytics in manufacturing operations. So, let's embark on this journey of leveraging predictive and prescriptive analytics to revolutionize manufacturing operations and stay ahead of the competition.
Here is what we shall cover in this post:
- Introduction to Predictive and Prescriptive Analytics
- Applications of Predictive and Prescriptive Analytics
- Driving Operational Efficiency With Analytics
- Optimizing Production Planning With Predictive Models
- Real-Time Monitoring and Predictive Quality Control
- Maximizing Resource Allocation With Prescriptive Models
- Utilizing Machine Learning for Predictive Analytics
- Real-Time Alerts and Notifications for Proactive Decision-Making
- Future Trends: Advancements in Predictive and Prescriptive Analytics
- How Deskera Can Assist You?
- Conclusion
- Key Takeaways
Introduction to Predictive and Prescriptive Analytics
Predictive and prescriptive analytics are powerful techniques that enable organizations to extract valuable insights from their data, anticipate future outcomes, and make data-driven decisions.
What is Predictive Analytics?
Predictive analytics is the practice of using historical data, statistical algorithms, and machine learning techniques to analyze patterns and relationships within the data and make predictions about future outcomes.
It goes beyond traditional business intelligence by providing a proactive approach to decision-making based on data-driven insights. Predictive analytics leverages the power of advanced algorithms to identify patterns and trends that may not be apparent through simple observation.
Methodologies of Predictive Analytics: Predictive analytics involves several key methodologies that enable organizations to make accurate predictions:
- Data Collection: The first step in predictive analytics is to collect relevant data. This can include a wide range of information, such as customer data, transactional records, social media feeds, and sensor data. The data must be comprehensive, accurate, and representative of the problem at hand.
- Data Preprocessing: Once the data is collected, it needs to be cleaned, transformed, and prepared for analysis. This involves tasks such as removing outliers, handling missing values, and normalizing the data to ensure its quality and consistency.
- Exploratory Data Analysis: Exploratory data analysis is conducted to gain a deeper understanding of the data. This involves visualizing the data, identifying correlations, and uncovering patterns that may provide insights into the problem being addressed.
- Model Selection: After analyzing the data, the next step is to select an appropriate predictive model. There are various models available, such as regression analysis, decision trees, random forests, support vector machines, and neural networks. The choice of model depends on the nature of the problem, the type of data, and the desired outcome.
- Model Training: Once the model is selected, it needs to be trained using the historical data. During the training phase, the model learns the patterns and relationships within the data to make accurate predictions.
- Model Evaluation: After training, the model is evaluated using appropriate performance metrics. This allows organizations to assess the accuracy and effectiveness of the model. Common evaluation metrics include accuracy, precision, recall, and F1 score.
- Deployment and Monitoring: Once the predictive model is developed and validated, it can be deployed in real-world scenarios. Ongoing monitoring is crucial to ensure that the model continues to perform well and remains accurate as new data becomes available.
What is Prescriptive Analytics?
Prescriptive analytics is the practice of using advanced algorithms and optimization techniques to evaluate different decision scenarios and determine the best possible solution that maximizes desired objectives while considering various constraints and business rules.
It combines data analysis, mathematical modeling, and computational power to provide organizations with actionable recommendations for optimal decision-making.
Prescriptive analytics takes advantage of the insights derived from descriptive and predictive analytics to provide a proactive approach to decision-making. It not only answers the question of what is likely to happen in the future but also guides decision-makers on what actions should be taken to achieve the desired outcomes.
Methodologies of Prescriptive Analytics: Prescriptive analytics involves several key methodologies that enable organizations to make informed decisions:
- Problem Formulation: The first step in prescriptive analytics is to define the problem and identify the decision-making objectives and constraints. This includes specifying the desired outcomes, establishing key performance indicators (KPIs), and defining business rules.
- Data Gathering and Analysis: Relevant data is collected and analyzed to gain insights into the problem and its underlying factors. This may involve analyzing historical data, market trends, customer preferences, and operational constraints. The data serves as input for the prescriptive models.
- Model Development: Prescriptive models are developed using advanced mathematical optimization techniques, simulation, and other decision-making tools. These models consider various factors, such as resource constraints, cost considerations, and risk factors, to recommend the best course of action.
- Scenario Analysis: Prescriptive analytics allows decision-makers to simulate different scenarios and evaluate their potential outcomes. By testing various options and analyzing their impact, organizations can identify the optimal decision strategy and assess potential risks and rewards.
- Optimization and Decision Support: Prescriptive analytics leverages optimization algorithms to determine the best possible solution that aligns with the desired objectives and constraints. These algorithms evaluate different decision variables, such as pricing strategies, production schedules, inventory levels, and resource allocations, to find the optimal combination.
- Actionable Insights and Recommendations: The output of prescriptive analytics is actionable insights and recommendations that guide decision-makers on the best actions to take. These recommendations may include specific actions, resource allocation strategies, pricing optimizations, or supply chain improvements.
Applications of Predictive and Prescriptive Analytics
Applications of Predictive Analytics
Sales and Revenue Forecasting: Predictive analytics enables organizations to forecast future sales volumes and revenues based on historical data, market trends, customer behavior, and other relevant factors.
By understanding customer preferences, market dynamics, and external factors, organizations can optimize their sales strategies, allocate resources effectively, and make data-driven decisions to maximize revenue growth.
Customer Churn Prediction and Retention: Customer retention is a critical factor for the success of any business. Predictive analytics helps organizations identify customers who are at risk of churning. By analyzing historical customer data, transactional patterns, and behavioral attributes, predictive models can identify early warning signs of customer dissatisfaction or disengagement.
This allows organizations to take proactive measures to retain customers by offering personalized incentives, tailored retention campaigns, and improved customer experiences.
Fraud Detection and Prevention: Predictive analytics plays a crucial role in fraud detection and prevention across industries such as finance, insurance, and e-commerce. By analyzing transactional data, customer behavior, and historical patterns, predictive models can identify anomalies and patterns associated with fraudulent activities.
Organizations can then take immediate action to prevent fraud, minimize financial losses, and protect their customers.
Inventory Optimization: Predictive analytics helps organizations optimize their inventory management by forecasting demand patterns, identifying seasonality, and detecting trends.
By analyzing historical sales data, external factors (such as weather conditions and economic indicators), and market trends, predictive models can predict future demand accurately. This enables organizations to optimize their inventory levels, reduce stockouts, minimize holding costs, and ensure timely order fulfillment.
Supply Chain Management: Predictive analytics plays a vital role in optimizing supply chain operations. By analyzing historical data, market trends, and external factors, predictive models can provide accurate demand forecasts, optimize procurement strategies, improve logistics planning, and enhance inventory management.
This results in reduced lead times, improved order fulfillment rates, and enhanced supply chain visibility, leading to overall operational efficiency and customer satisfaction.
Quality Control and Maintenance: Predictive analytics helps organizations in quality control and maintenance by predicting equipment failures and identifying potential quality issues. By analyzing sensor data, historical maintenance records, and production data, predictive models can identify patterns and indicators of equipment failure or quality deviations.
This enables organizations to take proactive maintenance actions, reduce unplanned downtime, optimize maintenance schedules, and ensure consistent product quality.
Healthcare and Medical Diagnosis: Predictive analytics has transformative applications in healthcare and medical diagnosis. By analyzing patient data, medical history, symptoms, and diagnostic test results, predictive models can aid in early disease detection, personalized treatment plans, and clinical decision support.
Predictive analytics can assist healthcare professionals in predicting disease progression, identifying high-risk patients, optimizing resource allocation, and improving patient outcomes.
Financial Forecasting and Risk Management: Predictive analytics plays a crucial role in financial forecasting and risk management. By analyzing financial data, market trends, economic indicators, and customer behavior, predictive models can provide accurate forecasts for revenue, profitability, and market trends.
This helps organizations make informed financial decisions, identify potential risks, optimize investment strategies, and ensure regulatory compliance.
Applications of Prescriptive Analytics
Supply Chain Optimization: Prescriptive analytics plays a crucial role in optimizing supply chain operations. By considering factors such as demand patterns, production capacities, transportation costs, and inventory levels, prescriptive models can recommend the most efficient allocation of resources, optimal production schedules, and effective distribution strategies.
This helps organizations reduce costs, improve delivery times, minimize stockouts, and enhance overall supply chain efficiency.
Resource Allocation and Optimization: Prescriptive analytics aids organizations in optimal resource allocation. Whether it's allocating budgets, human resources, or production capacities, prescriptive models consider constraints, objectives, and business rules to recommend the best allocation strategies.
By leveraging optimization algorithms, organizations can allocate resources effectively, maximize efficiency, and achieve desired outcomes.
Pricing Optimization: Prescriptive analytics helps organizations optimize pricing strategies by considering market demand, competitive landscape, cost structures, and customer behavior.
By analyzing historical sales data, customer preferences, and market trends, prescriptive models can recommend optimal pricing strategies that maximize revenue, profit margins, or market share while remaining competitive in the market.
Demand and Inventory Management: Prescriptive analytics aids organizations in demand forecasting and inventory management. By analyzing historical sales data, market trends, and external factors, prescriptive models can accurately predict future demand patterns. This enables organizations to optimize their inventory levels, improve stock availability, minimize holding costs, and ensure timely order fulfillment.
Prescriptive analytics also helps in dynamically adjusting inventory levels based on demand fluctuations and optimizing replenishment schedules.
Maintenance and Asset Management: Prescriptive analytics is valuable in predicting equipment failures and optimizing maintenance schedules. By analyzing sensor data, historical maintenance records, and operational data, prescriptive models can identify patterns and indicators of potential equipment failures.
This allows organizations to implement preventive maintenance measures, reduce unplanned downtime, optimize maintenance schedules, and enhance asset management.
Energy Optimization: Prescriptive analytics plays a significant role in energy optimization across industries. By analyzing energy consumption patterns, operational data, and environmental factors, prescriptive models can recommend optimal energy usage strategies.
This helps organizations reduce energy costs, optimize energy distribution, and minimize environmental impact by making informed decisions on energy consumption, production, and allocation.
Transportation and Route Optimization: Prescriptive analytics aids organizations in optimizing transportation routes and logistics operations. By analyzing factors such as transportation costs, delivery deadlines, vehicle capacities, and traffic conditions, prescriptive models can recommend the most efficient routes, load allocation strategies, and delivery schedules.
Driving Operational Efficiency With Analytics
Data analytics can help businesses improve operational efficiency by providing insights into how to optimize processes, reduce costs, and improve customer service.
What is Operational Efficiency?
Operational efficiency is the ability to produce a desired output with a minimum of inputs. In other words, it is about doing things right the first time and avoiding waste. Operational efficiency can be improved by streamlining processes, reducing errors, and optimizing resources.
How Can Data Analytics Improve Operational Efficiency?
Data analytics can help businesses improve operational efficiency in several ways. For example, data analytics can be used to:
- Identify areas where costs can be reduced.
- Improve customer service by identifying and resolving problems quickly.
- Improve product quality by identifying and preventing defects.
- Increase productivity by optimizing processes.
- Make better decisions by providing insights into data.
Benefits of Using Data Analytics to Improve Operational Efficiency
There are many benefits to using data analytics to improve operational efficiency. These benefits include:
- Increased profits: By reducing costs, improving customer service, and increasing productivity, data analytics can help businesses increase profits.
- Improved customer satisfaction: By resolving problems quickly and providing a better overall customer experience, data analytics can help businesses improve customer satisfaction.
- Increased market share: By being more efficient than competitors, data analytics can help businesses gain market share.
- Reduced risk: By identifying and preventing problems, data analytics can help businesses reduce risk.
How to Get Started with Data Analytics
The first step in getting started with data analytics is to collect data. This data can come from a variety of sources, such as customer transactions, production data, and employee performance data. Once the data has been collected, it needs to be analyzed. This analysis can be done using a variety of tools, such as statistical software and data visualization tools.
The next step is to identify opportunities for improvement. Once opportunities for improvement have been identified, action plans can be developed to implement these improvements. The final step is to monitor the results of these improvements to ensure that they are effective.
Predictive Analytics for Demand Forecasting
Predictive analytics is a branch of analytics that uses historical data, statistical algorithms, and machine-learning techniques to make predictions about future events or outcomes. In the context of demand forecasting, predictive analytics leverages historical sales data, customer behavior, market trends, and other relevant factors to forecast future demand accurately.
By identifying patterns, trends, and correlations within the data, predictive analytics models can provide insights into future demand patterns, enabling organizations to make proactive and data-driven decisions.
Benefits of Predictive Analytics for Demand Forecasting: Implementing predictive analytics for demand forecasting offers several benefits for organizations:
- Improved Forecast Accuracy: Predictive analytics models have the potential to provide more accurate demand forecasts compared to traditional methods. By considering a wide range of data sources and utilizing sophisticated algorithms, predictive models can capture complex demand patterns, seasonality, trends, and factors that impact demand.
- Enhanced Planning and Resource Allocation: Accurate demand forecasts enable organizations to optimize their production planning, inventory management, and resource allocation. By aligning production levels with expected demand, organizations can avoid overstocking or stockouts, minimize holding costs, reduce lead times, and optimize operational efficiency.
- Increased Customer Satisfaction: Accurate demand forecasting ensures that organizations can meet customer demands effectively. By having the right products available at the right time, organizations can improve customer satisfaction, increase on-time deliveries, and enhance overall customer experience.
- Cost Reduction: Predictive analytics helps organizations optimize their inventory levels, minimize excess inventory, and reduce holding costs. By aligning inventory levels with demand patterns, organizations can minimize stockouts and overstocking, reducing costs associated with inventory management and storage.
- Better Decision-Making: Predictive analytics provides organizations with valuable insights that enable informed decision-making. By understanding demand patterns and trends, organizations can make strategic decisions regarding pricing, promotions, product launches, and market expansion.
Optimizing Production Planning With Predictive Models
Predictive modeling in production planning involves using historical data, mathematical algorithms, and statistical techniques to forecast future production requirements accurately.
It aims to identify patterns, trends, and relationships within the data to make informed predictions about future demand, resource allocation, and production schedules. By leveraging predictive models, organizations can optimize production planning processes, enhance efficiency, and ensure timely order fulfillment.
Applications of Predictive Modeling in Production Planning: Predictive modeling finds applications in various aspects of production planning across industries. Some notable applications include:
- Capacity Planning: Predictive models help organizations forecast future production capacity requirements based on historical data and anticipated demand. This allows them to make informed decisions regarding capacity expansion, workforce planning, and equipment investments.
- Inventory Management: By accurately forecasting demand, predictive modeling helps organizations optimize inventory levels, minimize holding costs, and ensure timely order fulfillment. It enables organizations to maintain optimal inventory levels, reduce stockouts, and avoid excess inventory.
- Supplier Management: Predictive models aid organizations in supplier management by forecasting demand fluctuations and identifying critical suppliers. This allows organizations to proactively manage supplier relationships, negotiate contracts, and ensure a steady supply of raw materials.
- Quality Control: Predictive models can be used to identify patterns and indicators of quality issues in the production process. By analyzing data from quality inspections, machine sensors, and historical records, organizations can implement proactive quality control measures to reduce defects and improve product quality.
- Lead Time Optimization: Predictive models help organizations optimize lead times by considering factors such as order size, production capacity, and transportation times. This enables organizations to provide accurate delivery estimates to customers and manage customer expectations effectively.
Preventive Maintenance Through Predictive Analytics
Equipment failures and unexpected downtime can have a significant impact on productivity, profitability, and customer satisfaction. To mitigate these risks, organizations are turning to preventive maintenance strategies.
Traditional preventive maintenance schedules are based on fixed time intervals or predefined maintenance tasks, which may result in unnecessary maintenance activities or missed opportunities to address potential issues. However, with the emergence of predictive analytics, organizations now can implement more effective preventive maintenance programs.
The Shift from Reactive to Proactive Maintenance: Reactive maintenance, also known as "run-to-failure," involves repairing or replacing equipment after a failure occurs. This approach can result in unplanned downtime, increased repair costs, and a negative impact on production and customer satisfaction.
On the other hand, preventive maintenance aims to address potential issues before they lead to equipment failure. However, traditional preventive maintenance strategies are often based on fixed time intervals or predefined tasks, regardless of the actual condition of the equipment.
Predictive analytics takes preventive maintenance a step further by leveraging advanced data analysis techniques to predict equipment failures or degradation based on real-time data and historical patterns. This enables organizations to shift from reactive to proactive maintenance, resulting in improved equipment reliability and reduced downtime.
Predictive Analytics Techniques for Preventive Maintenance: Predictive analytics combines various techniques and technologies to analyze historical data, real-time sensor readings, and other relevant information to identify patterns and indicators of equipment failure or performance degradation. Some of the key techniques used in predictive maintenance include:
- Machine Learning: Machine learning algorithms can be trained on historical data to detect patterns and correlations between equipment conditions, sensor readings, and failure events. These models can then be used to predict future equipment failures or identify conditions that require maintenance intervention.
- Data Mining: Data mining techniques can be used to explore large datasets and extract valuable insights and patterns related to equipment failures. By analyzing historical maintenance records, sensor data, and operational parameters, organizations can identify key indicators and risk factors associated with equipment performance.
- Sensor Data Analysis: Sensors play a crucial role in collecting real-time data about equipment performance, temperature, vibrations, and other relevant parameters. Analyzing this sensor data can provide early indications of potential issues or abnormal conditions, allowing organizations to take proactive maintenance actions.
- Condition Monitoring: Condition monitoring involves continuously monitoring equipment health and performance using sensor data. By comparing real-time sensor readings with predefined thresholds or performance benchmarks, organizations can identify deviations or anomalies that may indicate impending equipment failures.
Real-Time Monitoring and Predictive Quality Control
Quality control has evolved over time, moving from manual inspections and random sampling to more data-driven and proactive approaches. Traditional quality control methods involved inspecting a sample of products after production to identify defects or deviations from the desired specifications.
While this approach has its merits, it is often limited in its ability to catch all quality issues and may not provide real-time insights into the production process.
- Real-time monitoring and predictive quality control, on the other hand, leverage advanced technologies and data analytics to monitor the production process continuously, identify potential quality issues in real time, and take proactive measures to ensure product quality.
- Real-time monitoring and predictive quality control (RQC) are two important concepts in the manufacturing industry. Real-time monitoring refers to the collection and analysis of data from manufacturing processes in real-time. Predictive quality control refers to the use of data analytics to predict the quality of products before they are released to the market.
- RQC can be used to improve the quality of products, reduce costs, and improve efficiency. By collecting and analyzing data in real-time, manufacturers can identify potential problems early on and take corrective action before they cause problems. This can help to reduce the number of defective products, improve customer satisfaction, and reduce the cost of recalls.
- Predictive quality control can be used to predict the quality of products before they are released to the market. This can help manufacturers to identify products that are likely to be defective and take corrective action before they are shipped to customers. This can help to improve customer satisfaction and reduce the cost of recalls.
Benefits of Real-Time Monitoring and Predictive Quality Control: Implementing real-time monitoring and predictive quality control strategies offers several significant benefits for organizations:
- Early Detection of Quality Issues: Real-time monitoring allows organizations to detect quality issues as they occur or even before they manifest. By continuously collecting and analyzing data, organizations can identify deviations, anomalies, or trends that indicate potential quality problems. This enables them to take immediate corrective actions and prevent defective products from reaching customers or causing further production issues.
- Improved Process Control: Real-time monitoring provides organizations with detailed insights into their production processes. By analyzing real-time data, organizations can identify variations, bottlenecks, or inefficiencies in the production line that may impact product quality. This allows them to make adjustments, optimize process parameters, and ensure consistent quality throughout the production cycle.
- Enhanced Customer Satisfaction: Real-time monitoring and predictive quality control help organizations deliver products with higher quality and reliability. By identifying and addressing quality issues proactively, organizations can reduce the number of defective products, minimize customer complaints, and improve overall customer satisfaction. Satisfied customers are more likely to become repeat customers and advocates for the organization's products or services.
- Cost Reduction: Detecting quality issues early and taking proactive measures can lead to significant cost savings. Real-time monitoring helps organizations identify quality problems before they escalate, reducing the need for rework, scrap, or costly recalls. By optimizing production processes and minimizing quality defects, organizations can also reduce operational costs associated with waste, downtime, and customer compensation.
- Increased Efficiency and Productivity: Real-time monitoring allows organizations to continuously evaluate the performance of their production processes. By analyzing data in real time, organizations can identify inefficiencies, bottlenecks, or deviations from expected standards.
How RQC Works
RQC uses a variety of data sources, including sensor data, machine learning algorithms, and historical data. This data is used to create a model of the manufacturing process. The model is then used to identify patterns and trends that can be used to predict potential problems.
When a potential problem is identified, a notification is sent to the quality control team. The quality control team can then take corrective action to prevent the problem from occurring.
Implementation of RQC
There are a few key steps involved in implementing RQC:
- Identify the processes that need to be monitored.
- Collect data from the processes.
- Build a model of the processes performance.
- Identify potential problems.
- Take corrective action to prevent potential problems.
Challenges of RQC
There are a few challenges associated with RQC, including:
- Data collection: Data collection can be challenging, as it requires access to sensor data, machine learning algorithms, and historical data.
- Data quality: Data quality can be a challenge, as the data may be incomplete, inaccurate, or corrupted.
- Model development: Model development can be challenging, as it requires expertise in machine learning and statistics.
- Maintenance: RQC systems require regular maintenance to ensure that they are functioning properly.
Prescriptive Analytics for Decision Optimization
Decision optimization is a key aspect of prescriptive analytics, focusing on finding the best possible solution to a specific problem or decision. It involves defining the decision problem, identifying the relevant variables and constraints, and formulating an optimization model that represents the problem mathematically.
The optimization model can then be solved using mathematical algorithms and optimization techniques to determine the optimal solution that maximizes or minimizes a defined objective function while satisfying all constraints.
Benefits of Prescriptive Analytics for Decision Optimization: Implementing prescriptive analytics for decision optimization offers several significant benefits for organizations:
- Improved Decision-Making: Prescriptive analytics provides organizations with data-driven insights and recommendations that guide decision-making processes. By considering multiple variables, constraints, and objectives, organizations can make informed decisions that optimize outcomes and align with strategic goals.
- Enhanced Efficiency: Prescriptive analytics helps organizations streamline their decision-making processes. By automating the analysis and optimization tasks, organizations can save time, reduce human errors, and achieve faster and more efficient decision-making cycles. This allows decision-makers to focus on strategic thinking and evaluating alternative scenarios rather than spending time on manual calculations or analysis.
- Optimal Resource Allocation: Many decision problems involve allocating scarce resources efficiently. Prescriptive analytics can optimize resource allocation by considering various factors, such as costs, capacity constraints, demand patterns, and performance metrics. By determining the optimal allocation strategy, organizations can maximize resource utilization and minimize waste.
- Risk Mitigation: Prescriptive analytics can help organizations assess and mitigate risks associated with decision-making. By incorporating risk factors and uncertainties into the optimization model, organizations can evaluate the impact of different decisions on risk exposure. This enables decision-makers to make risk-informed decisions and take appropriate actions to minimize potential negative outcomes.
- Cost Reduction: Optimizing decisions through prescriptive analytics can lead to significant cost savings. By considering cost factors, such as production costs, inventory carrying costs, transportation costs, or labor costs, organizations can identify cost-effective solutions that minimize expenses while satisfying all operational and business constraints.
- Increased Competitiveness: Prescriptive analytics enables organizations to make decisions that give them a competitive advantage. By optimizing pricing strategies, supply chain operations, or marketing campaigns, organizations can improve customer satisfaction, increase market share, and differentiate themselves from competitors.
- Scalability and Adaptability: Prescriptive analytics models are scalable and adaptable, allowing organizations to address complex decision problems across different business functions.
Maximizing Resource Allocation With Prescriptive Models
Traditional resource allocation methods often rely on manual processes or simplistic rules of thumb, which may not consider the complexities and constraints involved. However, with the advent of prescriptive analytics and advanced modeling techniques, organizations now have the opportunity to leverage sophisticated prescriptive models to optimize resource allocation.
Understanding Resource Allocation: Resource allocation refers to the process of assigning resources, such as people, equipment, time, or budget, to different activities or projects within an organization. It involves making decisions on how to distribute limited resources efficiently to achieve the desired outcomes.
- Effective resource allocation is critical for organizations to optimize their operational efficiency, meet customer demands, and drive profitability. However, resource allocation can be complex due to various factors, including competing priorities, capacity constraints, uncertainty, and dynamic business environments.
- Prescriptive models leverage optimization techniques and mathematical algorithms to determine the best allocation of resources to achieve specific objectives. These models consider various factors, constraints, and trade-offs to identify the optimal resource allocation strategy. By incorporating data-driven insights, prescriptive models enable organizations to make informed decisions that maximize efficiency, minimize costs, and achieve desired outcomes.
Key Considerations in Resource Allocation: Effective resource allocation requires careful consideration of several key factors:
- Objective: Clearly defining the objective of resource allocation is essential. Whether it's maximizing profit, minimizing costs, optimizing production output, or achieving a specific service level, the objective should align with the organization's strategic goals.
- Constraints: Constraints play a crucial role in resource allocation decisions. These constraints can include budget limitations, capacity constraints, regulatory requirements, skill requirements, or time constraints. Understanding and incorporating these constraints into the optimization model ensures that the resource allocation strategy is feasible and practical.
- Trade-offs: Resource allocation often involves trade-offs between competing objectives or constraints. For example, allocating more resources to one project may result in less availability for another. Organizations must carefully consider these trade-offs and define the relative importance of different objectives to guide the optimization process.
- Uncertainty: Resource allocation decisions are often made in the presence of uncertainty. Factors such as demand fluctuations, market conditions, or supply chain disruptions can introduce uncertainty into the decision-making process. Prescriptive models can incorporate uncertainty through scenario analysis or probabilistic approaches, enabling organizations to make robust and risk-informed decisions.
Reducing Waste and Improving Sustainability Through Analytics
Waste is a major problem in today's world. It costs businesses billions of dollars each year, and it harms the environment. However, there are some ways to reduce waste and improve sustainability through analytics.
Understanding Waste and Sustainability: Waste refers to any material, energy, or resource that is discarded or unused during production, operations, or consumption. It can take various forms, including raw material waste, energy waste, process inefficiencies, and product waste. Sustainability, on the other hand, encompasses practices that minimize the negative impact on the environment, society, and the economy.
It involves adopting responsible and efficient resource management, reducing carbon emissions, promoting recycling and reuse, and embracing sustainable business models. By focusing on waste reduction and sustainability, organizations can improve their environmental footprint, enhance brand reputation, and achieve long-term profitability.
Analytics is the process of collecting, analyzing, and interpreting data. It can be used to improve decision-making, identify trends, and solve problems. Analytics can be used to reduce waste and improve sustainability in a variety of ways.
How Can Analytics Be Used to Reduce Waste?
Analytics can be used to identify areas where waste is occurring. This can be done by tracking the flow of materials through a process or by analyzing data on energy usage. Once areas of waste have been identified, analytics can be used to develop strategies for reducing or eliminating it.
For example, a manufacturing plant might use analytics to track the amount of waste generated by each machine. This information could then be used to identify machines that are generating more waste than others. The plant could then take steps to improve the efficiency of these machines or to replace them with more efficient models.
How Can Analytics Be Used to Improve Sustainability?
Analytics can be used to improve sustainability by identifying ways to reduce energy consumption, water usage, and emissions. It can also be used to identify opportunities to use renewable energy sources and to recycle or compost materials.
For example, a company might use analytics to track its energy usage over time. This information could then be used to identify areas where energy is being wasted. The company could then take steps to reduce energy consumption, such as by installing energy-efficient lighting or by improving insulation.
Benefits of Using Analytics to Reduce Waste and Improve Sustainability
There are several benefits to using analytics to reduce waste and improve sustainability. These benefits include:
- Cost savings: Reducing waste can save businesses money. For example, a study by the World Economic Forum found that companies that have implemented sustainability initiatives have saved an average of $1.26 million per year.
- Improved efficiency: Analytics can help businesses to improve their efficiency by identifying areas where waste is occurring and by developing strategies for reducing or eliminating it.
- Increased profits: Reducing waste and improving sustainability can lead to increased profits. For example, a study by the McKinsey Global Institute found that companies that have implemented sustainability initiatives have seen their profits increase by an average of 15%.
- Improved reputation: Businesses that are seen as being sustainable are often more attractive to customers and investors. For example, a study by the Boston Consulting Group found that 70% of consumers are willing to pay more for products that are made sustainably.
Enhancing Supply Chain Visibility With Predictive Insights
Supply chain visibility refers to the ability of organizations to track and monitor their inventory, orders, and shipments in real time across the entire supply chain network. It involves capturing and analyzing data from various sources, such as suppliers, manufacturers, logistics providers, and customers, to gain insights into the status and movement of goods throughout the supply chain.
Improved supply chain visibility enables organizations to make data-driven decisions, proactively identify bottlenecks or disruptions, and optimize their operations to meet customer demands effectively.
The Role of Predictive Insights in Supply Chain Visibility: Predictive insights play a vital role in enhancing supply chain visibility by leveraging advanced analytics techniques to forecast future events, anticipate risks, and optimize decision-making.
By analyzing historical and real-time data, organizations can identify patterns, trends, and correlations that enable them to predict potential disruptions, optimize inventory levels, and streamline their supply chain operations. Predictive insights provide organizations with actionable information to make proactive decisions, mitigate risks, and improve overall supply chain performance.
Here are some of the benefits of using predictive insights to enhance supply chain visibility:
- Improved customer service: By having visibility into their supply chains, businesses can better meet customer demand and avoid stockouts. This can lead to improved customer satisfaction and loyalty.
- Reduced costs: By identifying and mitigating risks, businesses can reduce the costs associated with disruptions to their supply chains. This includes the cost of lost sales, inventory carrying costs, and transportation costs.
- Increased profits: By improving efficiency and reducing costs, businesses can increase their profits.
- Improved sustainability: By reducing waste and emissions, businesses can improve their sustainability performance.
Here are some of the challenges of using predictive insights to enhance supply chain visibility:
- Data quality: The quality of the data used to generate predictive insights is critical. If the data is not accurate, the insights will be inaccurate.
- Model complexity: Predictive models can be complex and difficult to understand. This can make it difficult for businesses to use the insights generated by these models to make decisions.
- Technology investment: Predictive insights require investment in technology. This includes the cost of software, hardware, and data storage.
Despite the challenges, the benefits of using predictive insights to enhance supply chain visibility are significant. Businesses that can overcome these challenges can gain a competitive advantage by improving their supply chain performance.
Utilizing Machine Learning for Predictive Analytics
Predictive analytics is the process of using data to predict future events or outcomes. Machine learning is a type of artificial intelligence (AI) that allows computers to learn without being explicitly programmed.
When used together, machine learning and predictive analytics can be powerful tool for businesses to make better decisions. For example, predictive analytics can be used to:
- Predict customer churn
- Identify fraudulent transactions
- Forecast demand
- Optimize operations
- Improve customer satisfaction
Machine learning can be used to create predictive models that can learn from data and make predictions about future outcomes. There are many different types of machine learning algorithms, each with its strengths and weaknesses.
Machine Learning Techniques in Predictive Analytics
Machine learning techniques play a vital role in predictive analytics, enabling organizations to extract meaningful insights and make accurate predictions. Here are some key machine-learning techniques commonly used in predictive analytics:
Regression Analysis
Regression analysis is used to establish relationships between variables and make predictions based on observed data. It is useful for predicting continuous numerical outcomes, such as sales forecasts or customer lifetime value, by identifying patterns and correlations in the data.
There are several types of regression analysis, each suited for different scenarios and data types. The commonly used types of regression analysis include:
- Linear Regression: Linear regression is the most basic and widely used type of regression analysis. It assumes a linear relationship between the dependent variable and the independent variables. The goal of linear regression is to find the best-fitting straight line that minimizes the sum of the squared differences between the predicted and actual values. Linear regression can be further categorized into simple linear regression (with one independent variable) and multiple linear regression (with multiple independent variables).
- Polynomial Regression: Polynomial regression is an extension of linear regression that allows for non-linear relationships between the dependent variable and the independent variables. It involves fitting a polynomial function of a specified degree to the data. This type of regression can capture more complex relationships and curves.
- Logistic Regression: Logistic regression is used when the dependent variable is categorical or binary. It models the relationship between the independent variables and the probability of the occurrence of a specific category or outcome. Logistic regression estimates the parameters of a logistic function to predict the probability of belonging to a particular category.
- Ridge Regression: Ridge regression is a variant of linear regression that addresses the issue of multicollinearity (high correlation between independent variables). It adds a penalty term to the sum of squared differences to control the magnitude of the coefficients. Ridge regression helps reduce the impact of multicollinearity and provides more stable and reliable estimates.
- Lasso Regression: Lasso regression, similar to ridge regression, addresses multicollinearity but with a different approach. It uses the L1 regularization technique, which shrinks some coefficients to zero, effectively performing feature selection. Lasso regression is useful when there are a large number of independent variables, and it helps identify the most relevant variables for prediction.
- Elastic Net Regression: Elastic Net regression combines the techniques of ridge regression and lasso regression. It incorporates both L1 and L2 regularization terms to strike a balance between the two approaches. Elastic Net regression is helpful in situations where there are many correlated variables and a subset of them are relevant.
Methodologies of Regression Analysis:
Regression analysis involves several methodologies to estimate the regression model parameters and evaluate the model's performance. Some of the key methodologies include:
- Ordinary Least Squares (OLS): OLS is a widely used method for estimating the parameters of the regression model. It minimizes the sum of the squared differences between the observed values and the predicted values. OLS provides unbiased estimates when certain assumptions about the data are met.
- Maximum Likelihood Estimation (MLE): MLE is a statistical method used to estimate the parameters of the regression model. It maximizes the likelihood function, which measures the probability of observing the given data under the assumed regression model. MLE is particularly useful when dealing with logistic regression or other models with probabilistic assumptions.
- Residual Analysis: Residual analysis is used to evaluate the goodness of fit of the regression model. It involves examining the residuals, which are the differences between the observed values and the predicted values. Residual analysis helps identify patterns or outliers in the data and assesses the adequacy of the regression model.
- Model Validation: Model validation techniques such as cross-validation or train-test splits are used to assess the predictive performance of the regression model on unseen data. These techniques help evaluate the model's ability to generalize and make accurate predictions on new observations.
Classification Algorithms
Classification algorithms are used to classify data into distinct categories or classes. They are valuable for solving problems such as customer segmentation, fraud detection, or sentiment analysis. Popular classification algorithms include decision trees, logistic regression, and support vector machines.
There are numerous classification algorithms available, each with its strengths, weaknesses, and specific use cases. Some of the most commonly used classification algorithms include:
- Naive Bayes: Naive Bayes is a probabilistic classification algorithm based on Bayes' theorem. It assumes that the features are conditionally independent given the class. Naive Bayes is simple, fast, and performs well in text classification and spam filtering tasks.
- k-Nearest Neighbors (k-NN): k-NN classifies instances based on their similarity to the nearest neighbors in the training data. It assigns a new instance to the majority class among its k nearest neighbors. k-NN is non-parametric and suitable for non-linear decision boundaries.
- Gradient Boosting: Gradient boosting is an ensemble method that combines multiple weak classifiers to create a strong classifier. It builds the model iteratively by adding weak classifiers that correct the errors made by the previous classifiers. Gradient boosting algorithms, such as XGBoost and AdaBoost, often achieve high predictive accuracy.
Clustering Algorithms
Clustering algorithms group similar data points together based on their characteristics, enabling organizations to identify patterns and segments within their data. Clustering is useful for tasks such as market segmentation, customer profiling, or anomaly detection. Common clustering algorithms include k-means clustering and hierarchical clustering.
Types of Clustering Algorithms:
There are several types of clustering algorithms, each with its assumptions and working principles. The choice of clustering algorithm depends on factors such as the nature of the data, the desired cluster structure, scalability, and interpretability. The main types of clustering algorithms are:
- Partition-based Clustering: Partition-based algorithms aim to divide the data into a predefined number of clusters. One of the most popular partition-based algorithms is K-means clustering. K-means minimizes the sum of squared distances between data points and their assigned cluster centroids. It iteratively updates the centroids and reassigns data points to the nearest centroid until convergence.
- Hierarchical Clustering: Hierarchical clustering algorithms create a hierarchy of clusters by iteratively merging or splitting existing clusters based on their similarity. Agglomerative hierarchical clustering starts with each data point as a separate cluster and iteratively merges the closest pairs of clusters until a single cluster remains. Divisive hierarchical clustering starts with a single cluster and recursively splits it into smaller clusters until each data point is a separate cluster.
- Density-based Clustering: Density-based algorithms identify clusters as regions of high density separated by regions of low density. One popular density-based algorithm is DBSCAN (Density-Based Spatial Clustering of Applications with Noise). DBSCAN groups data points that are close to each other and have sufficient density, while outliers or noise points are left unassigned.
- Model-based Clustering: Model-based algorithms assume that the data points are generated from a probabilistic model. They aim to estimate the parameters of the underlying model and assign data points to clusters based on the estimated model. Gaussian Mixture Models (GMM) is a widely used model-based clustering algorithm that assumes data points are generated from a mixture of Gaussian distributions.
- Fuzzy Clustering: Fuzzy clustering assigns data points to multiple clusters with varying degrees of membership. Instead of hard assignments, fuzzy clustering provides a membership value that indicates the degree to which a data point belongs to each cluster. Fuzzy C-means (FCM) is a popular fuzzy clustering algorithm that iteratively updates cluster centroids and membership values based on minimizing an objective function.
- Subspace Clustering: Subspace clustering algorithms aim to discover clusters in subspaces of the feature space, considering different subsets of features for different clusters. This is particularly useful when data points may belong to different clusters in different subspaces. Subspace clustering algorithms include CLIQUE (CLustering In QUEst), PROCLUS, and P3C.
Neural Networks
Neural networks are a class of machine learning models inspired by the structure and functioning of the human brain. They consist of interconnected nodes, or artificial neurons, organized in layers. Neural networks excel at solving complex problems and can be used for tasks such as image recognition, natural language processing, or time series forecasting.
Neural Network Architecture: Neural networks are composed of multiple layers, each serving a specific purpose. The key idea behind neural networks is to learn from data by adjusting the weights of the connections between neurons. This process, known as training, enables the network to recognize patterns, make predictions, or solve specific tasks.
Neural networks have gained prominence in predictive analytics due to their ability to automatically learn and extract meaningful representations from data, leading to highly accurate predictions.
Neural Network Architecture in Predictive Analytics:
Neural networks used in predictive analytics consist of interconnected layers of artificial neurons or units. The architecture of a neural network plays a crucial role in its ability to extract meaningful features and make accurate predictions. The following components make up the neural network architecture:
- Input Layer: The input layer receives the initial data and passes it through the network. Each neuron in the input layer represents a feature or attribute of the input data.
- Hidden Layers: Hidden layers are intermediary layers between the input and output layers. The number of hidden layers and the number of neurons in each layer vary depending on the complexity of the problem. Deep neural networks can have multiple hidden layers, allowing them to learn intricate representations of the data.
- Output Layer: The output layer produces the final predictions or classifications. The number of neurons in the output layer depends on the specific predictive task. For example, in a binary classification problem, there would be a single neuron representing the probability of the positive class, while in a multi-class classification problem, each class would have a corresponding neuron.
Activation Functions:
Activation functions introduce non-linearity into the neural network, allowing it to model complex relationships between input and output. Activation functions are applied to the output of each neuron in the network. Some commonly used activation functions include:
- Sigmoid Function: The sigmoid function maps the input to a range between 0 and 1, which can be interpreted as probabilities. It is often used in the output layer for binary classification problems.
- ReLU (Rectified Linear Unit): The ReLU function outputs the input directly if it is positive, and 0 otherwise. ReLU is widely used in hidden layers as it helps the network to learn complex representations.
- Tanh (Hyperbolic Tangent): The tanh function maps the input to a range between -1 and 1, offering a symmetric activation function that is often used in hidden layers.
Time Series Analysis
Time series analysis focuses on analyzing and forecasting data that changes over time. It is useful for predicting trends, identifying seasonality, and making future projections based on historical data. Techniques such as autoregressive integrated moving averages (ARIMA) and exponential smoothing models are commonly used in time series analysis.
Key Components of Time Series Analysis:
Time series analysis involves understanding and modeling the key components of a time series, which include:
- Trend: Trend represents the long-term pattern or direction of the time series. It indicates whether the series is increasing, decreasing, or following a more complex pattern. Identifying and modeling the trend component is essential for understanding the overall behavior of the time series.
- Seasonality: Seasonality refers to the recurring patterns or cycles that occur within a time series at regular intervals. It can be daily, weekly, monthly, or even yearly. Detecting and incorporating the seasonality component is crucial for accurately predicting values at specific time points.
- Cyclical Patterns: Cyclical patterns are fluctuations that occur over a longer time frame, often influenced by economic, social, or political factors. These patterns are not as regular as seasonality and can span several years or even decades. Identifying cyclical patterns helps in understanding the broader context and potential long-term trends in the time series.
- Residuals: Residuals, also known as the error term, represent the random or unpredictable component of the time series that cannot be explained by the trend, seasonality, or cyclical patterns. Analyzing the residuals can provide insights into model adequacy and any remaining patterns that need to be captured.
Time Series Analysis Techniques:
Time series analysis involves a range of techniques and models to understand and predict future values based on historical patterns. Some commonly used techniques in time series analysis include:
- Descriptive Analysis: Descriptive analysis aims to understand the properties and characteristics of a time series. It involves visualizing the data using plots such as line plots, scatter plots, and histograms. Descriptive statistics such as mean, median, and standard deviation provide insights into the central tendency and variability of the data.
- Smoothing: Smoothing techniques help in removing noise and revealing the underlying trends and patterns in the data. Moving averages and exponential smoothing methods are commonly used to smooth out short-term fluctuations and highlight long-term trends.
- Decomposition: Decomposition separates a time series into its trend, seasonality, and residual components. It enables a more detailed analysis of each component and helps in understanding the individual contributions to the overall behavior of the time series.
- Autocorrelation: Autocorrelation measures the correlation between a time series and its lagged values. Autocorrelation plots, such as the autocorrelation function (ACF) and partial autocorrelation function (PACF), provide insights into the presence of correlation and help identify potential lagged relationships.
- Stationarity Analysis: Stationarity refers to the statistical properties of a time series remaining constant over time. Stationary time series exhibit a constant mean, variance, and autocorrelation structure. Stationarity analysis involves checking for stationarity using statistical tests and, if required, applying transformations to achieve stationarity.
- Forecasting: Forecasting is the primary objective of time series analysis. It involves predicting future values of a time series based on historical patterns. Various forecasting models, such as autoregressive integrated moving averages (ARIMA), exponential smoothing, and seasonal decomposition of time series (STL), are used for accurate predictions.
Ensemble Methods
Ensemble methods combine multiple machine learning models to make more accurate predictions. Techniques such as random forests and gradient boosting algorithms create a combination of weak models to create a strong ensemble model. Ensemble methods are often used when high prediction accuracy is crucial, such as in credit scoring or fraud detection.
Ensemble methods can be categorized into two main types:
- Bagging: Bagging (Bootstrap Aggregating) is a technique where multiple models are trained independently on different subsets of the training data. Each model produces its prediction, and the final prediction is obtained by combining the individual predictions, typically through voting or averaging. Bagging helps to reduce the variance and increase the stability of predictions, especially when dealing with high-variance models such as decision trees.
- Boosting: Boosting is a technique where multiple models, called weak learners, are trained sequentially. Each model focuses on the misclassified instances or places more weight on the difficult examples, allowing subsequent models to learn from the mistakes of the previous models. Boosting aims to improve overall performance by creating a strong learner from a collection of weak learners. Examples of boosting algorithms include AdaBoost, Gradient Boosting, and XGBoost.
Benefits of Ensemble Methods:
Ensemble methods offer several advantages over individual models:
- Increased Accuracy: Ensemble methods often achieve higher accuracy than individual models. By combining the predictions of multiple models, ensemble methods can capture different aspects of the data, reducing biases and errors present in individual models.
- Robustness: Ensemble methods are more robust to noise and outliers in the data. Since the predictions are based on a consensus of multiple models, outliers or erroneous predictions from individual models are less likely to impact the final prediction.
- Reduced Overfitting: Overfitting occurs when a model learns to perform well on the training data but fails to generalize to new, unseen data. Ensemble methods help mitigate overfitting by combining multiple models, each trained on different subsets of the data or with different parameters. This diversity helps to capture the underlying patterns of the data more effectively.
- Improved Stability: Ensemble methods increase the stability of predictions. Since the predictions are obtained by aggregating the predictions from multiple models, the ensemble is less sensitive to small changes in the training data or model parameters. This stability is especially beneficial in real-world scenarios where data can be noisy or subject to variations.
The most common types of machine learning algorithms for predictive analytics include:
Decision Trees
Decision trees are a simple but effective type of machine-learning algorithm. They work by dividing the data into smaller and smaller groups until each group can be classified.
Construction of Decision Trees:
- The construction of a decision tree involves selecting the best features to split the data and determining the optimal split points. There are various algorithms for building decision trees, with the most popular ones being ID3, C4.5, and CART.
- The ID3 (Iterative Dichotomiser 3) algorithm uses entropy and information gain to measure the purity of a split. Entropy is a measure of impurity or disorder within a set of examples, while information gain quantifies the reduction in entropy achieved by a particular split. The ID3 algorithm selects the feature that maximizes information gain at each step to build the tree.
- The C4.5 algorithm is an extension of ID3 and handles both categorical and continuous features. It uses a similar approach to ID3 but incorporates a mechanism to handle missing values and can handle continuous features by creating multiple binary splits.
- The CART (Classification and Regression Trees) algorithm is commonly used for both classification and regression tasks. It uses the Gini impurity as a measure of the quality of a split. The Gini impurity measures the probability of misclassifying a randomly chosen element from the set. CART recursively partitions the data based on minimizing the Gini impurity until a stopping criterion is met.
Evaluating Decision Trees:
Once a decision tree is constructed, it needs to be evaluated to ensure its effectiveness and generalizability. The two primary metrics used to evaluate decision trees are accuracy and interpretability.
- Accuracy measures how well the decision tree predicts the target variable on unseen data. This can be assessed using techniques such as cross-validation, where the data is divided into training and testing sets. The decision tree is trained on the training set and then evaluated on the testing set to measure its predictive performance.
- Interpretability refers to the ability to understand and interpret the decisions made by the tree. Decision trees are highly interpretable models since they can be visualized and understood by non-technical stakeholders. The tree structure provides insights into the decision-making process, making it easier to explain and justify the predictions or classifications made.
Random Forests
Random forests are a type of ensemble learning algorithm that combines multiple decision trees to improve accuracy.
Construction of Random Forests:
The construction of Random Forests involves two main sources of randomness: random sampling of the training data and random feature selection.
- Random Sampling: For each decision tree in the Random Forest, a bootstrap sample is created by randomly selecting data points from the original training dataset. The bootstrap sample is typically of the same size as the original dataset but may contain duplicate instances. This sampling process introduces variability in the training data and helps create diverse decision trees.
- Random Feature Selection: At each decision point of a tree, a random subset of features is considered for splitting. This subset is typically smaller than the total number of features. By randomly selecting a subset of features, Random Forests ensure that different decision trees rely on different subsets of features, reducing the likelihood of one feature dominating the predictions.
Evaluation of Random Forests:
- Random Forests are evaluated using various metrics to assess their predictive performance and generalizability. The most common evaluation metrics include accuracy, precision, recall, F1-score, and area under the receiver operating characteristic curve (AUC-ROC).
- Accuracy measures the overall correctness of the Random Forest's predictions. It is the ratio of the number of correct predictions to the total number of instances. Precision quantifies the proportion of correctly predicted positive instances out of all instances predicted as positive, while recall measures the proportion of correctly predicted positive instances out of all actual positive instances. F1-score combines precision and recall into a single metric, providing a balanced assessment of the model's performance.
- AUC-ROC measures the ability of the Random Forest to discriminate between positive and negative instances across different probability thresholds. It plots the true positive rate against the false positive rate, with a higher AUC-ROC indicating better predictive performance.
Support Vector Machines
Support vector machines are a type of machine learning algorithm that can be used for both classification and regression tasks.
Working Principles of Support Vector Machines:
- Maximum Margin Classification: The primary objective of an SVM is to find a decision boundary that maximizes the margin between the classes. The margin is the distance between the decision boundary and the closest data points from each class, known as support vectors. SVMs aim to find the hyperplane that achieves the largest margin while minimizing the classification error.
- Soft Margin Classification: In real-world scenarios, it is often challenging to find a linear decision boundary that perfectly separates the classes due to overlapping or noisy data. SVMs handle such situations by introducing a soft margin, allowing some data points to be misclassified. The balance between the margin size and the classification error is controlled by a hyperparameter known as C. A smaller value of C allows for a wider margin but may tolerate more misclassifications, while a larger value of C emphasizes accurate classification but may lead to a narrower margin.
- Kernel Trick: SVMs can handle non-linearly separable data by applying a kernel function that transforms the original feature space into a higher-dimensional space where the data becomes linearly separable. The kernel function calculates the dot product between pairs of transformed feature vectors, avoiding the explicit computation of the higher-dimensional space. Common kernel functions include the linear kernel, polynomial kernel, and radial basis function (RBF) kernel.
Construction and Training of Support Vector Machines:
The construction of an SVM involves two main steps: feature transformation (if using a non-linear kernel) and training the model.
- Feature Transformation: If the data is non-linearly separable, a kernel function is applied to transform the original feature space into a higher-dimensional space. The transformed data is then used to find the optimal hyperplane that separates the classes.
- Training the Model: The training of an SVM involves finding the optimal hyperplane by solving an optimization problem. The objective is to minimize the classification error and maximize the margin. This optimization problem is typically solved using quadratic programming techniques.
During training, the SVM learns the support vectors, which are the critical data points that determine the position and orientation of the decision boundary. The support vectors play a crucial role in the SVM's generalization capabilities as they represent the most influential points for classification.
- The choice of machine learning algorithm will depend on the specific problem that is being solved. For example, decision trees are often a good choice for classification problems, while neural networks are often a good choice for regression problems.
- Once a predictive model has been created, it can be used to make predictions about future outcomes. These predictions can then be used to make better decisions. For example, a company could use a predictive model to predict customer churn. This information could then be used to target customers with retention offers.
- Machine learning and predictive analytics are powerful tools that can be used to improve decision-making. However, it is important to note that these tools are not perfect. They can make mistakes, and they should not be used as the sole basis for decision-making.
Optimization of Inventory Management With Prescriptive Analytics
Inventory management is a critical part of any business. It involves keeping track of inventory levels, ordering new inventory, and managing costs. Prescriptive analytics can be used to optimize inventory management by providing insights into demand, supply, and costs.
In the context of inventory management, prescriptive analytics helps organizations determine the best course of action to achieve optimal inventory levels while considering various constraints and objectives. By utilizing mathematical optimization models, algorithms, and simulation techniques, prescriptive analytics identifies the most efficient allocation of inventory, optimal order quantities, reorder points, and replenishment schedules. This enables organizations to make informed decisions that balance inventory costs, customer service levels, and operational efficiency.
Key Components of Prescriptive Analytics in Inventory Management:
Mathematical Modeling
Mathematical modeling is at the core of prescriptive analytics. It involves formulating mathematical representations of inventory management problems, including constraints, objectives, and decision variables.
Various optimization techniques, such as linear programming, integer programming, or stochastic programming, are utilized to solve these models and find optimal solutions.
Mathematical modeling allows organizations to consider multiple factors, such as demand variability, lead times, storage capacities, and service levels, quantitatively and systematically.
Mathematical models in prescriptive analytics consist of the following elements:
- Objective Function: The objective function defines the goal or objective to be optimized. It quantifies the measure of success or effectiveness of the decisions being made. The objective can be to maximize or minimize a specific parameter, such as profit, cost, time, or resource utilization.
- Decision Variables: Decision variables represent the unknowns or parameters that can be controlled or manipulated in the system. These variables can take on different values to influence the outcomes or achieve the desired objectives. Decision variables can be discrete or continuous, depending on the nature of the problem.
- Constraints: Constraints capture the limitations, restrictions, or conditions that must be satisfied in the decision-making process. These constraints can be related to resource availability, capacity, budget, regulations, or any other relevant factors. They define the feasible region or space of acceptable solutions.
- Mathematical Relationships: Mathematical relationships describe the dependencies and interactions among the decision variables, constraints, and objective functions. These relationships are typically represented by mathematical equations, inequalities, or logical expressions. They ensure that the decisions made align with the desired goals and comply with the defined constraints.
Mathematical Optimization Techniques:
Mathematical optimization is a key component of prescriptive analytics, aiming to find the best possible solution to a given problem within the specified constraints. Various optimization techniques are used to solve mathematical models, including:
- Linear Programming (LP): Linear programming is a widely used optimization technique for solving linear mathematical models. LP aims to maximize or minimize a linear objective function subject to linear constraints. LP is often used in resource allocation, production planning, and scheduling problems.
- Integer Programming (IP): Integer programming extends linear programming by allowing decision variables to take only integer values. IP is useful when decisions need to be made in whole numbers, such as selecting the number of units to produce or the allocation of discrete resources.
- Nonlinear Programming (NLP): Nonlinear programming deals with optimization problems where the objective function or constraints involve nonlinear relationships. NLP techniques are used when the problem involves nonlinear equations or non-convex optimization.
- Mixed-Integer Programming (MIP): Mixed-integer programming combines both continuous and integer decision variables. It is used when a problem includes both discrete decisions (integer variables) and continuous decisions (continuous variables). MIP is widely applied in supply chain optimization, facility location, and project scheduling.
- Constraint Programming (CP): Constraint programming focuses on specifying the relationships and constraints between variables rather than optimizing an objective function. CP is particularly useful when the problem has complex logical constraints or a large number of feasible solutions.
- Stochastic Programming: Stochastic programming deals with optimization problems where some of the input parameters or constraints are uncertain or random. Stochastic programming techniques take into account the probabilistic nature of the problem and seek solutions that are robust to uncertainty.
Demand Forecasting
Accurate demand forecasting is crucial for effective inventory management. Prescriptive analytics leverages historical data, statistical techniques, and advanced forecasting algorithms to generate accurate demand forecasts.
By analyzing patterns, trends, seasonality, and external factors that influence demand, organizations can better anticipate future demand patterns and adjust their inventory levels accordingly. Demand forecasting plays a critical role in determining optimal reorder points, safety stock levels, and replenishment strategies.
Constraint Management
Inventory management involves dealing with numerous constraints that impact decision-making. These constraints can include factors such as budget limitations, storage capacities, production capacities, lead times, and service level requirements.
Prescriptive analytics takes into account these constraints while optimizing inventory decisions. By defining and managing constraints effectively, organizations ensure that the recommended inventory strategies align with operational capabilities and meet customer expectations.
Constraints can be classified into different types:
- Hard Constraints: Hard constraints are non-negotiable limitations that must be satisfied for a solution to be considered feasible. Violating a hard constraint renders a solution infeasible and unacceptable. Examples of hard constraints include physical limitations, legal regulations, safety requirements, and contractual obligations.
- Soft Constraints: Soft constraints, also known as a preference or optimization goals, represent desired conditions that can be compromised to some extent. While violating a soft constraint does not render a solution infeasible, it may result in suboptimal or less desirable outcomes. Soft constraints are used to guide the optimization process and prioritize conflicting objectives.
- Logical Constraints: Logical constraints capture the logical relationships between decision variables. They represent the interdependencies and restrictions that must be satisfied. For example, in an assignment problem, each task can only be assigned to one worker, and each worker can only be assigned to one task.
Constraint management is critical in prescriptive analytics for the following reasons:
- Real-World Alignment: Constraints reflect the real-world limitations, rules, and requirements that decision-makers must adhere to. Incorporating constraints into the decision-making process ensures that the generated solutions are feasible and align with the practical constraints of the problem domain.
- Solution Space Reduction: Constraints narrow down the solution space by eliminating infeasible or suboptimal solutions. By incorporating constraints, decision-makers can focus on exploring only the feasible region of the solution space, saving computational resources and time.
- Risk Mitigation: Constraints help in managing risks and avoiding potential issues or conflicts. By considering constraints, decision-makers can anticipate and address potential challenges and trade-offs associated with the decision-making process.
- Optimization with Trade-offs: Constraints often introduce trade-offs between conflicting objectives. Constraint management enables decision-makers to balance multiple objectives while respecting the limitations imposed by constraints. This allows for the identification of optimal solutions that meet both the desired objectives and the constraint requirements.
Scenario Analysis
Prescriptive analytics enables organizations to conduct scenario analysis to assess the impact of different scenarios on inventory management. By considering various "what-if" scenarios, organizations can evaluate the outcomes of alternative strategies and make informed decisions.
Scenario analysis helps organizations understand the sensitivity of inventory decisions to changes in demand patterns, lead times, pricing, or other factors. It allows decision-makers to assess potential risks, evaluate trade-offs, and select the best course of action.
The process of scenario analysis generally involves the following steps:
- Scenario Identification: The first step in scenario analysis is to identify relevant scenarios based on factors such as market trends, economic conditions, technological advancements, regulatory changes, and other external influences. Scenarios should cover a wide range of possibilities and reflect the uncertainties and risks inherent in the decision context.
- Scenario Development: Once the scenarios are identified, they need to be developed in more detail. This involves specifying the key variables, factors, and assumptions associated with each scenario. The scenarios should capture the variations in these factors and their potential impacts on the decision problem.
- Model Formulation: Mathematical models or simulation techniques are used to model the decision problem under each scenario. The models incorporate the relevant variables, constraints, objectives, and decision variables to represent the decision-making context accurately. The models are then solved or simulated to obtain decision recommendations for each scenario.
- Scenario Evaluation: The decision recommendations obtained from the models are evaluated for each scenario. Decision-makers assess the outcomes, risks, trade-offs, and robustness of their decisions under different scenarios. This evaluation provides insights into the strengths and weaknesses of the decisions and helps in selecting the most suitable course of action.
Simulation and Optimization
Simulation and optimization techniques are employed in prescriptive analytics to test and refine inventory management strategies. Simulation models replicate real-world inventory systems, considering factors such as demand variability, supply disruptions, lead times, and order quantities.
By simulating different inventory policies and strategies, organizations can assess their performance, identify bottlenecks, and evaluate the impact of changes before implementing them in real-time operations. Optimization techniques are then applied to find the best inventory management strategies based on the insights gained from simulations.
Decision Support Systems
Prescriptive analytics is often integrated into decision support systems (DSS) or inventory management software. These systems provide user-friendly interfaces, dashboards, and visualizations that facilitate decision-making. Decision support systems allow inventory managers to interact with the prescriptive analytics models, view key performance indicators, analyze scenarios, and receive recommendations for inventory optimization.
DSS can be classified into different types based on their functionality and application domain:
Model-Driven DSS: Model-Driven DSS utilizes mathematical, statistical, or simulation models to analyze data and generate insights. These models can be used to evaluate different scenarios, optimize decisions, or perform sensitivity analysis.
Model-Driven DSS provides decision-makers with analytical tools to explore and understand the implications of different decision alternatives.
Data-Driven DSS: Data-Driven DSS leverages large volumes of data to extract patterns, trends, and insights. These systems employ data mining, machine learning, and statistical techniques to analyze historical data and make predictions or recommendations.
Data-Driven DSS enables decision-makers to base their decisions on data-driven insights and improve the accuracy and effectiveness of their decision-making process.
Knowledge-Driven DSS: Knowledge-Driven DSS incorporates expert knowledge and rules into the decision-making process. These systems capture and formalize the expertise of domain experts and provide recommendations or suggestions based on predefined rules and decision criteria.
Knowledge-Driven DSS helps decision-makers leverage domain knowledge and best practices to make more informed and consistent decisions.
Communication-Driven DSS: Communication-Driven DSS facilitates communication, collaboration, and information sharing among decision-makers and stakeholders. These systems provide platforms for sharing data, exchanging ideas, and conducting discussions.
Communication-Driven DSS enhances the decision-making process by fostering a collaborative and participatory approach, ensuring that decisions are well-informed and supported by a diverse set of perspectives.
Real-Time Alerts and Notifications for Proactive Decision-Making
Real-time alerts and notifications play a crucial role in enabling proactive decision-making by providing timely insights and actionable information. By leveraging advanced technologies and data analytics, organizations can receive instant alerts and notifications based on predefined triggers or anomalies in their operational data.
These real-time alerts empower decision-makers to respond swiftly to critical events, identify emerging trends, and take proactive measures to drive business success.
Understanding Real-Time Alerts and Notifications: Real-time alerts and notifications are automated messages or notifications that are generated and sent to stakeholders in real-time or near real-time. These alerts are triggered by predefined rules, thresholds, or anomalies in data, signaling important events, changes, or deviations from expected patterns.
Real-time alerts can be delivered through various channels such as email, SMS, mobile applications, dashboards, or even automated phone calls, ensuring that decision-makers receive timely information wherever they are. By providing instant updates on critical information, organizations can proactively address issues, seize opportunities, and optimize their operations.
Many different types of real-time alerts and notifications can be used for proactive decision-making. Some of the most common types include:
- Performance alerts: These alerts notify users of changes in performance metrics, such as sales, revenue, or customer satisfaction.
- Security alerts: These alerts notify users of potential security threats, such as unauthorized access or data breaches.
- Operational alerts: These alerts notify users of operational problems, such as equipment failures or production delays.
The Role of Real-Time Alerts and Notifications in Proactive Decision-Making: Real-time alerts and notifications are instrumental in enabling proactive decision-making across various areas of an organization:
Operational Efficiency: Real-time alerts help organizations monitor key performance indicators (KPIs) and operational metrics in real time. By setting up alerts for specific thresholds or deviations, decision-makers can identify operational inefficiencies or bottlenecks promptly.
For example, an alert triggered by a sudden increase in production downtime can prompt immediate action to resolve the issue, minimizing the impact on productivity and customer satisfaction. Real-time alerts enable organizations to proactively address operational challenges and optimize resource allocation.
Risk Management: Real-time alerts play a vital role in risk management by detecting and notifying stakeholders about potential risks and anomalies. Organizations can set up alerts to monitor critical risk indicators, such as cybersecurity breaches, supply chain disruptions, or regulatory compliance violations.
When an alert is triggered, decision-makers can quickly investigate the issue, implement mitigation strategies, and prevent further damage or losses. Real-time alerts provide organizations with the ability to anticipate and mitigate risks before they escalate into major crises.
Customer Experience: Real-time alerts and notifications contribute to enhancing the customer experience by enabling proactive engagement and support. Organizations can set up alerts to monitor customer feedback, social media mentions, or website interactions.
When an alert is triggered, customer service representatives can respond promptly, addressing customer concerns and resolving issues in real time. Real-time alerts help organizations identify patterns and trends in customer behavior, enabling them to personalize offerings, improve service quality, and foster customer loyalty.
Supply Chain Management: Real-time alerts are invaluable in supply chain management, where timely information is critical for efficient operations. Organizations can set up alerts to monitor inventory levels, delivery delays, or disruptions in the supply chain. When an alert is triggered, supply chain managers can take immediate action to adjust production schedules, source alternative suppliers, or reroute shipments.
Real-time alerts help organizations optimize inventory levels, reduce lead times, and enhance overall supply chain visibility and responsiveness.
Financial Management: Real-time alerts support proactive financial management by monitoring financial metrics and market trends. Organizations can set up alerts to track changes in key financial indicators, such as cash flow, revenue, or expenses.
When an alert is triggered, decision-makers can analyze the underlying causes and take necessary steps to ensure financial stability.
Improving Product Quality and Reducing Defects Through Analytics
Customers have become increasingly demanding, expecting products that not only meet their requirements but also exceed their expectations. Additionally, with the rise of e-commerce and social media, the reputation of a brand can quickly be tarnished by negative reviews or reports of product defects. Therefore, it is imperative for companies to continuously improve product quality and reduce defects.
One powerful tool that has emerged in recent years to support these efforts is analytics. By leveraging data and advanced analytical techniques, companies can gain valuable insights into their manufacturing processes, identify areas for improvement, and make data-driven decisions to enhance product quality.
Understanding the Impact of Defects:
Before delving into the role of analytics in improving product quality, it is essential to understand the consequences of defects. Product defects can lead to a variety of negative outcomes for both customers and businesses. For customers, defects can result in dissatisfaction, reduced trust in the brand, and a reluctance to repurchase.
In some cases, defects can even pose safety hazards, leading to accidents or injuries. From a business perspective, defects can be costly. They result in increased warranty claims, higher customer support costs, and potential recalls or legal actions.
Defects also damage the reputation of the brand, leading to a loss of market share and revenue. Therefore, reducing defects is not just about meeting quality standards; it is a strategic imperative for organizations.
Here are some specific examples of how analytics can be used to improve product quality and reduce defects:
- Identifying potential problems early: Analytics can be used to track customer feedback, identify trends, and spot potential issues. For example, a company that sells software could use analytics to track customer reviews of its products. If a particular product is receiving a lot of negative reviews, analytics could be used to identify the specific problems that customers are having. This information could then be used to make changes to the product to improve its quality.
- Monitoring the production process: Analytics can be used to monitor the production process to ensure that products are being manufactured to the required standards. For example, a company that manufactures cars could use analytics to track the quality of the parts that are used to build its cars. If a particular part is found to be defective, analytics could be used to identify the specific problem with the part. This information could then be used to correct the problem or to find a new supplier for the part.
- Reducing costs: By identifying and preventing defects, businesses can save money on rework, recalls, and warranty claims. For example, a company that manufactures toys could use analytics to track the number of defective toys that are produced. If the number of defective toys is too high, analytics could be used to identify the specific problems that are causing the defects. This information could then be used to make changes to the manufacturing process to reduce the number of defects.
In addition to the benefits mentioned above, using analytics to improve product quality and reduce defects can also lead to several other benefits, such as:
- Increased customer satisfaction: Customers are more likely to be satisfied with products that are high quality and free of defects. This can lead to increased sales and revenue.
- Improved brand reputation: A reputation for high-quality products can help to attract new customers and retain existing customers.
- Increased employee morale: Employees are more likely to be motivated and engaged when they are working on products that are high quality and free of defects. This can lead to improved productivity and reduced turnover.
Root Cause Analysis and Corrective Actions:
Analytics can also be instrumental in identifying the root causes of defects and guiding corrective actions. Through advanced analytics techniques, such as machine learning algorithms and natural language processing, organizations can analyze vast amounts of data to identify the factors that contribute to defects. This analysis can help uncover hidden patterns and insights that may not be apparent through traditional methods.
Once the root causes are identified, organizations can take targeted corrective actions to address the underlying issues. For example, if the analysis reveals that a specific supplier consistently provides subpar raw materials, the company can either work closely with the supplier to improve the quality or find an alternative supplier.
Similarly, if a particular manufacturing process step is consistently associated with defects, the company can focus on optimizing that step or implementing additional quality control measures.
Future Trends: Advancements in Predictive and Prescriptive Analytics
Predictive and prescriptive analytics have emerged as powerful tools in the era of big data and advanced analytics. These techniques enable organizations to extract valuable insights from data, make accurate predictions, and optimize decision-making processes. As technology continues to advance, several future trends are shaping the field of predictive and prescriptive analytics.
Augmented Analytics
Augmented analytics combines the power of artificial intelligence (AI) and machine learning (ML) with human intuition to enhance the analytical capabilities of users. This approach leverages advanced algorithms to automate data preparation, model building, and insights generation.
Augmented analytics tools assist users in exploring data, identifying patterns, and generating actionable insights. Natural language processing (NLP) and natural language generation (NLG) techniques further enable users to interact with the analytics system through conversational interfaces, making analytics accessible to a broader audience.
Internet of Things (IoT) Analytics
The proliferation of connected devices and the Internet of Things (IoT) is generating vast amounts of data. IoT analytics focuses on extracting valuable insights from IoT data to drive predictive and prescriptive analytics.
By analyzing real-time sensor data, organizations can detect anomalies, predict equipment failures, optimize maintenance schedules, and improve operational efficiencies. IoT analytics also enables the integration of physical and digital data, providing a holistic view of business processes and enabling data-driven decision-making.
Streaming Analytics
Traditional analytics approaches often rely on batch processing, where data is collected, stored, and analyzed in batches. However, with the advent of real-time data streams, organizations are increasingly turning to streaming analytics. Streaming analytics enables the analysis of data as it is generated, providing immediate insights and enabling real-time decision-making.
This is particularly valuable in industries such as finance, healthcare, and transportation, where timely insights can make a significant impact. Advanced techniques such as complex event processing (CEP) and machine learning on streaming data are driving the development of sophisticated streaming analytics platforms.
Enhanced Natural Language Processing (NLP)
Natural Language Processing (NLP) is a field of artificial intelligence that focuses on the interaction between humans and computers through natural language. The advancement of NLP techniques, including sentiment analysis, named entity recognition, and language translation, is driving the adoption of text and voice data analytics in predictive and prescriptive analytics.
Enhanced NLP capabilities allow organizations to extract insights from unstructured data sources such as social media, customer reviews, and support tickets. By analyzing text and voice data, organizations can understand customer sentiment, detect emerging trends, and gain valuable insights into consumer behavior. NLP also plays a crucial role in automating data extraction from documents, enabling faster and more accurate analysis.
Automated Machine Learning (AutoML)
Building and deploying machine learning models can be a complex and time-consuming process. Automated Machine Learning (AutoML) aims to simplify and automate this process by leveraging AI and ML techniques. AutoML platforms enable non-experts to build and deploy ML models without extensive knowledge of algorithms and coding.
These platforms automate tasks such as data preprocessing, feature engineering, model selection, and hyperparameter tuning. AutoML empowers organizations to leverage predictive and prescriptive analytics capabilities without relying heavily on data scientists, thus democratizing the use of advanced analytics.
Explainable AI and Ethical Considerations
As AI and ML models become increasingly complex and influential, there is a growing need for transparency, interpretability, and ethical considerations. Explainable AI (XAI) techniques aim to provide insights into how AI models make decisions, enabling users to understand the underlying factors and biases.
XAI techniques such as feature importance analysis, rule extraction, and model-agnostic explanations are gaining prominence. Additionally, organizations are paying increased attention to ethical considerations in predictive and prescriptive analytics, ensuring that these technologies are deployed responsibly and without bias or discrimination.
Continuous Optimization
Traditionally, optimization models have been applied to static scenarios or solved periodically. However, with the availability of real-time data and advancements in optimization algorithms, continuous optimization is becoming a reality.
Continuous optimization involves solving optimization models dynamically as new data becomes available, leading to more adaptive and responsive decision-making. This is particularly relevant in domains such as supply chain management, logistics, and resource allocation, where decisions need to be made in real-time to adapt to changing conditions.
How Deskera Can Assist You?
Deskera ERP and MRP system can help you:
- Manage production plans
- Maintain Bill of Materials
- Generate detailed reports
- Create a custom dashboard
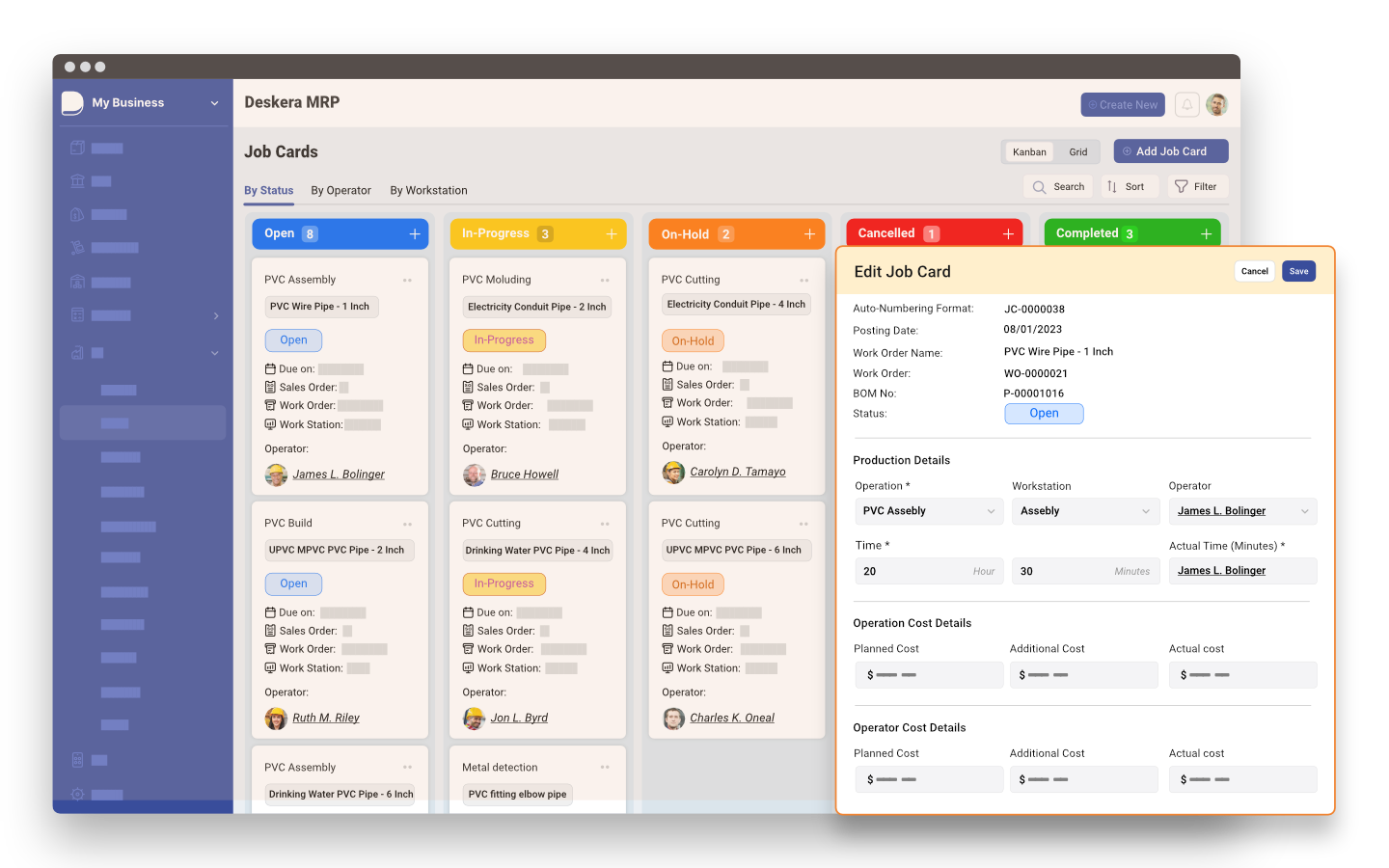
Deskera ERP is a comprehensive system that allows you to maintain inventory, manage suppliers, and track supply chain activity in real-time, as well as streamline a variety of other corporate operations.
Deskera MRP allows you to closely monitor the manufacturing process. From the bill of materials to the production planning features, the solution helps you stay on top of your game and keep your company's competitive edge.
Deskera Books enables you to manage your accounts and finances more effectively. Maintain sound accounting practices by automating accounting operations such as billing, invoicing, and payment processing.
Deskera CRM is a strong solution that manages your sales and assists you in closing agreements quickly. It not only allows you to do critical duties such as lead generation via email, but it also provides you with a comprehensive view of your sales funnel.
Deskera People is a simple tool for taking control of your human resource management functions. The technology not only speeds up payroll processing but also allows you to manage all other activities such as overtime, benefits, bonuses, training programs, and much more. This is your chance to grow your business, increase earnings, and improve the efficiency of the entire production process.
Conclusion
The use of predictive and prescriptive analytics in manufacturing operations has revolutionized the industry by enabling proactive decision-making, optimizing processes, and driving efficiency and profitability. The ability to leverage historical data, real-time data, and advanced analytics techniques empowers manufacturing leaders to make informed decisions based on insights and predictions about future outcomes.
By leveraging predictive analytics, manufacturers can identify potential issues before they occur, anticipate demand fluctuations, optimize maintenance schedules, and improve overall operational performance. Prescriptive analytics takes it a step further by providing actionable recommendations and strategies to address identified challenges and capitalize on opportunities.
Implementing predictive and prescriptive analytics in manufacturing operations does come with its challenges. Manufacturers need to ensure data quality and availability, establish robust data management systems, and overcome any existing silos within the organization.
The successful adoption of analytics requires skilled personnel who can effectively interpret and apply the insights generated by the analytics models. Additionally, the integration of analytics into existing processes and systems may require careful planning and coordination.
With the right tools, skills, and mindset, manufacturers can unlock the full potential of predictive and prescriptive analytics and pave the way for a more productive and profitable future.
Key Takeaways
- Predictive and prescriptive analytics play a crucial role in optimizing manufacturing operations by leveraging data-driven insights.
- Predictive analytics uses historical and real-time data to forecast future outcomes, enabling manufacturers to proactively address potential issues.
- Prescriptive analytics goes beyond predictions and provides actionable recommendations to optimize processes and improve operational efficiency.
- By leveraging predictive and prescriptive analytics, manufacturers can reduce downtime, improve asset performance, and increase production efficiency.
- Predictive maintenance allows manufacturers to identify equipment failures before they occur, minimizing unplanned downtime and reducing maintenance costs.
- Optimizing inventory management through predictive analytics helps manufacturers minimize stockouts and excess inventory, improving supply chain efficiency.
- Prescriptive analytics helps manufacturers make data-driven decisions on production scheduling, resource allocation, and process optimization.
- Predictive and prescriptive analytics enable manufacturers to anticipate demand fluctuations and adjust production levels accordingly, improving customer satisfaction.
- Real-time data integration and advanced analytics techniques allow manufacturers to respond quickly to market changes and optimize production plans.
- By optimizing maintenance schedules and reducing equipment downtime, predictive analytics improves overall equipment effectiveness (OEE) in manufacturing operations.
Related Articles
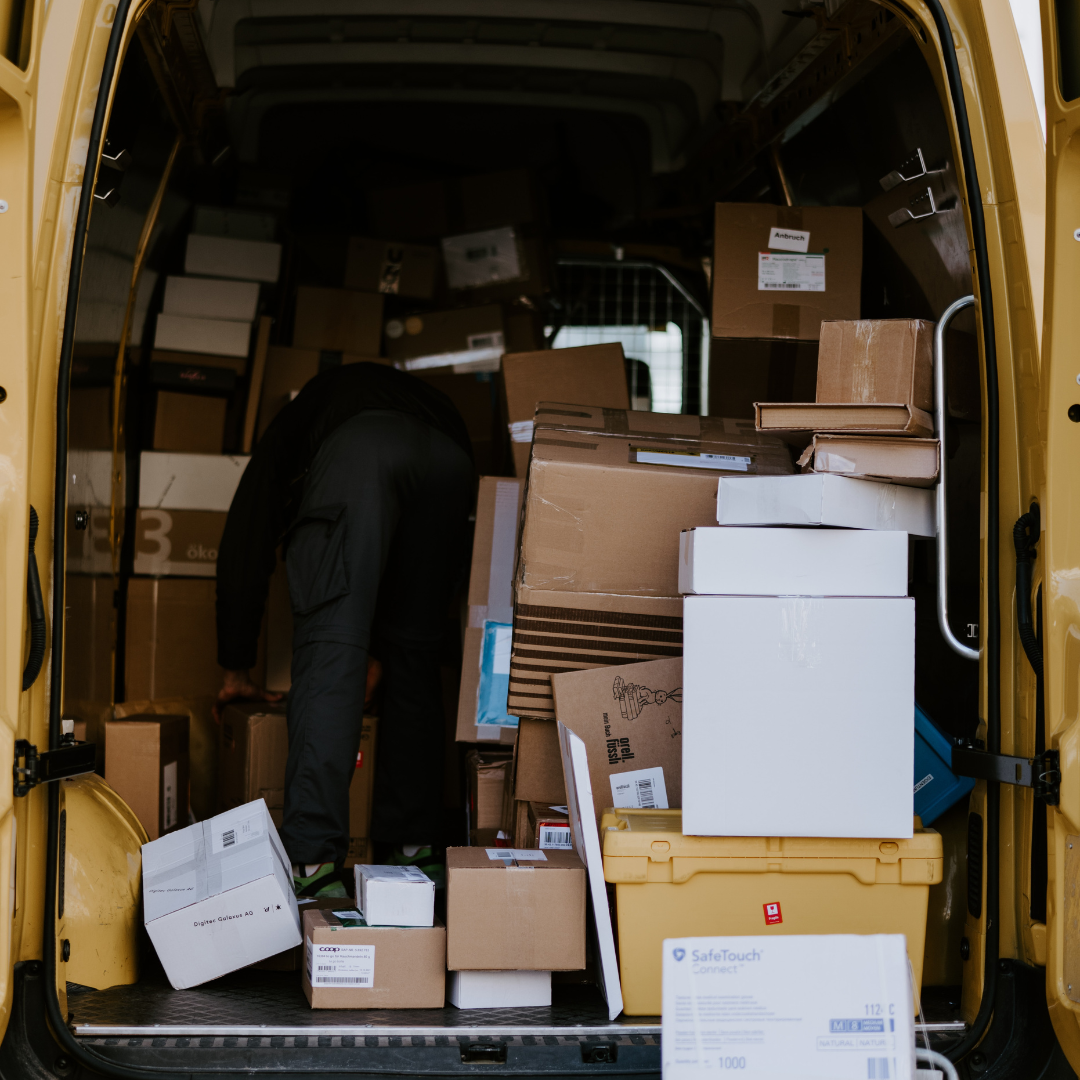
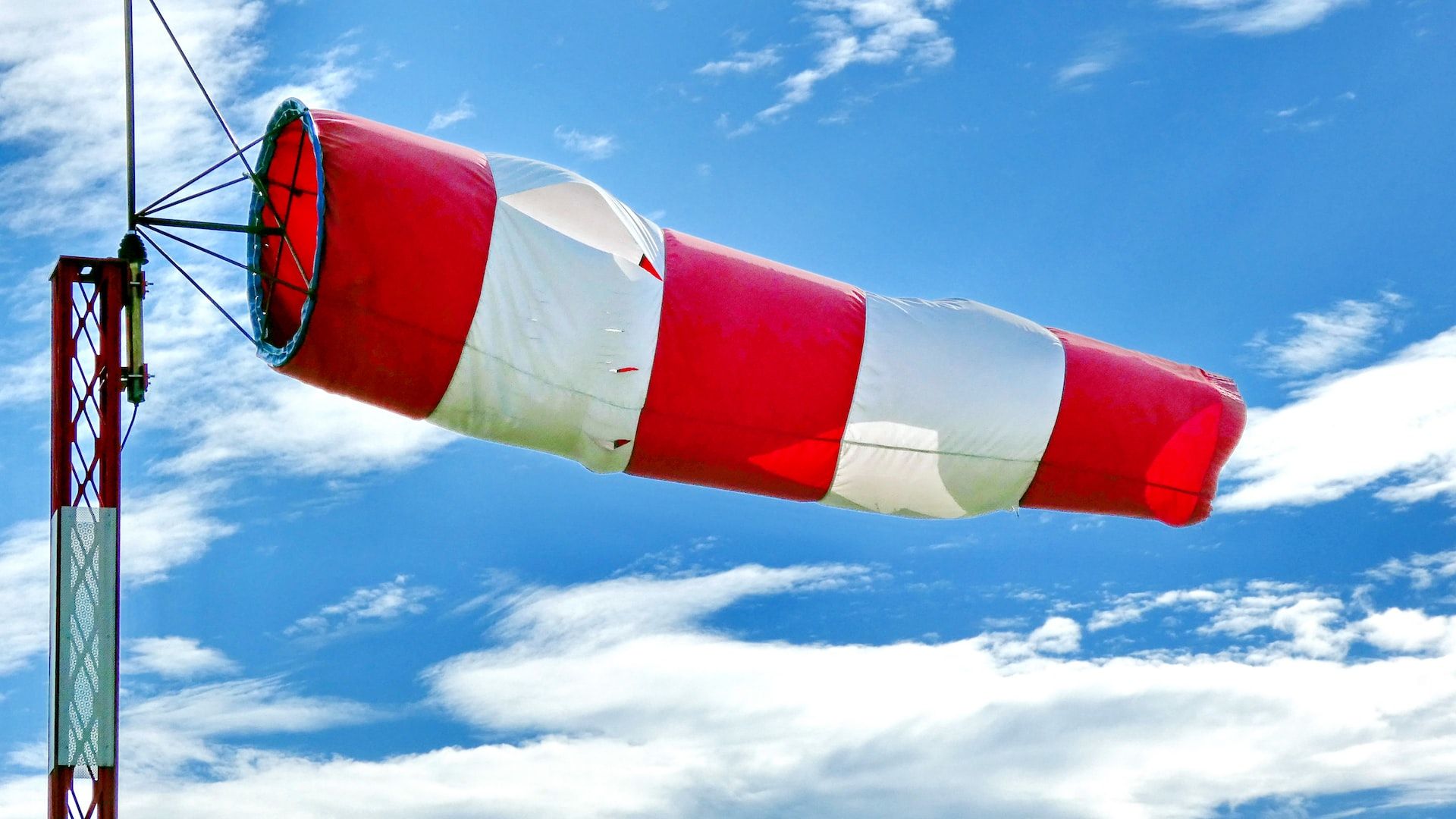
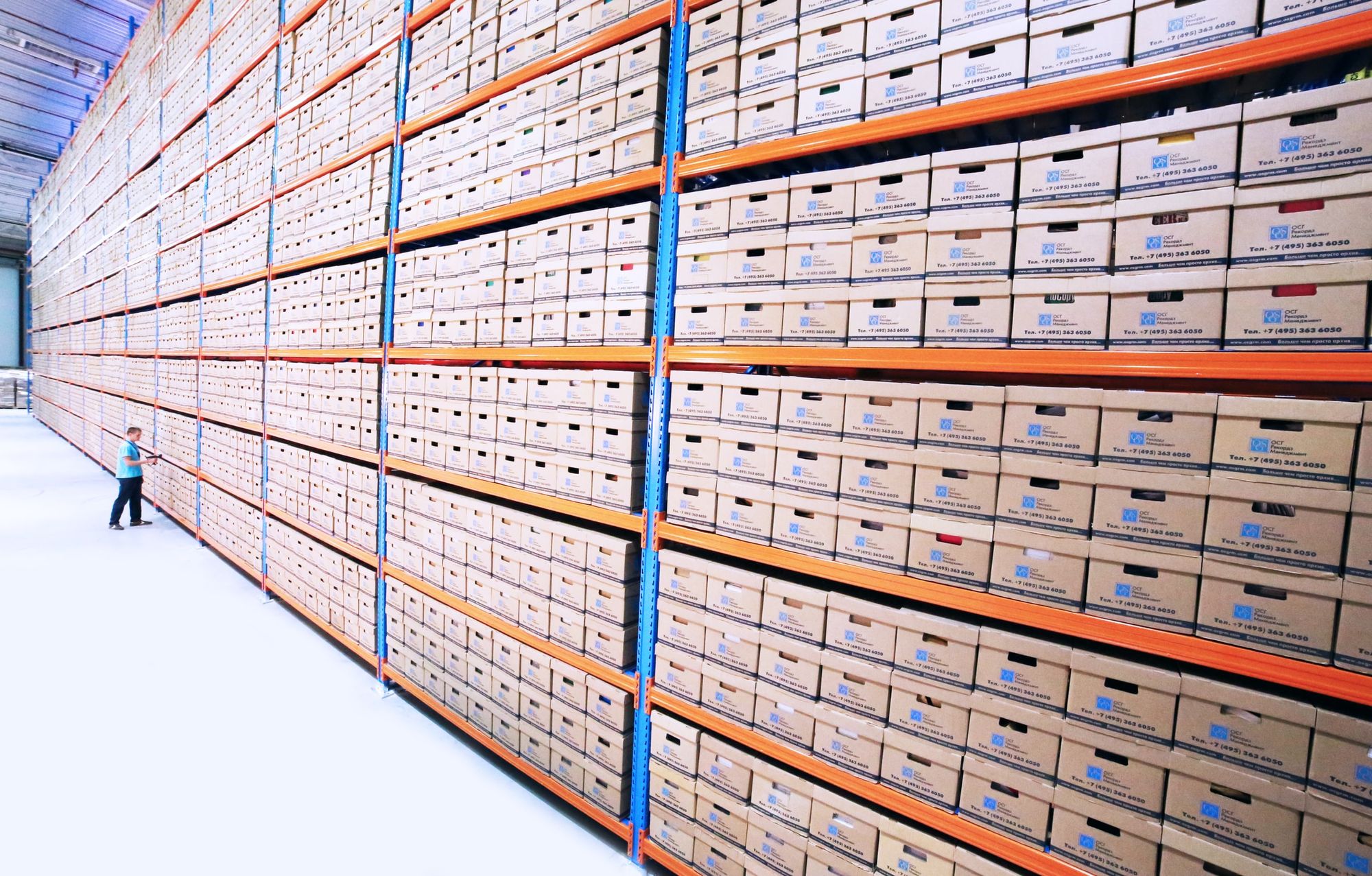