In the fast-paced and highly competitive world of manufacturing, effective inventory control is paramount to success. However, traditional methods of inventory management often fall short, leading to inefficiencies, stockouts, and missed opportunities.
Enter AI—the game-changer that is revolutionizing inventory control for manufacturing leaders. With its advanced algorithms and data-driven insights, AI has the potential to transform inventory management processes and drive significant improvements.
According to a recent study by McKinsey, companies that adopt AI in inventory control can achieve up to 20% reduction in inventory carrying costs and 50% decrease in stockouts. Furthermore, Gartner predicts that by 2023, 50% of global mid-size and larger organizations will have implemented AI-driven inventory management systems.
These statistics highlight the growing recognition of AI's transformative power in optimizing inventory control.
By leveraging AI's capabilities, manufacturing leaders can accurately forecast demand, proactively monitor inventory in real-time, and optimize supply chain operations. In this article, we will delve into the potential of AI in revolutionizing inventory control and explore the key benefits and applications for manufacturing leaders.
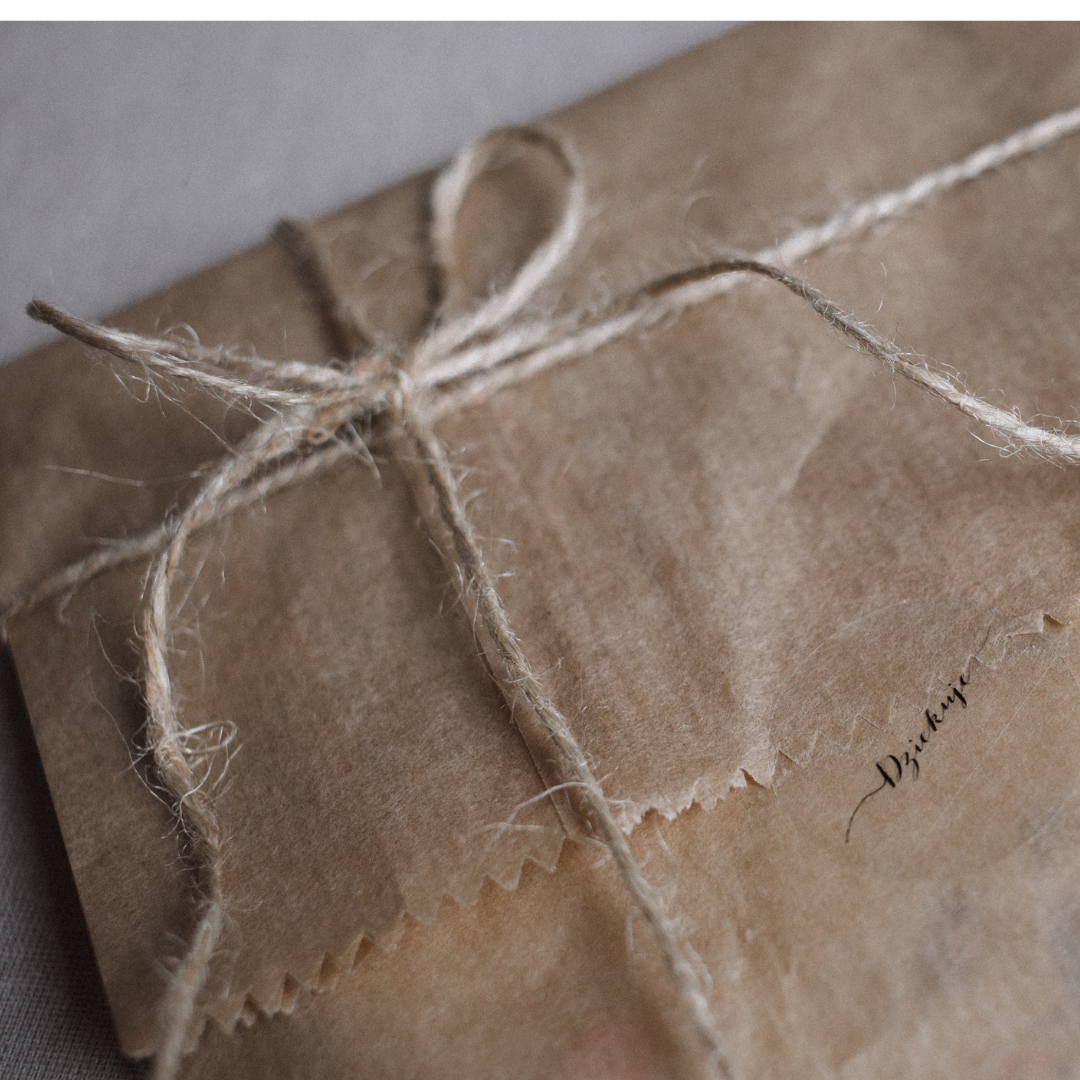
Get ready to unlock a new era of efficiency, profitability, and competitive advantage in the manufacturing industry.
Here is what we shall cover in this post:
- Importance of Efficient Inventory Control in the Manufacturing Industry
- The Traditional Methods of Inventory Management and their Limitations
- Potential of AI in Revolutionizing Inventory Control
- The Challenges of Inventory Control in Manufacturing
- The Role of AI in Inventory Control
- AI Applications in Inventory Control
- Case Studies of Successful AI Implementation in Inventory Control
- Overcoming Implementation Challenges
- Transformative Potential of AI In Revolutionizing Inventory Control for Manufacturing Leaders
- Embrace AI Technology and Reap its Benefits in Optimizing Inventory Management Processes
- Conclusion
- How can Deskera Help You?
- Key Takeaways
- Related Articles
Importance of Efficient Inventory Control in the Manufacturing Industry
Efficient inventory control is of paramount importance in the manufacturing industry due to its direct impact on a company's profitability, customer satisfaction, and overall operational efficiency. Inventory management involves the strategic planning, tracking, and optimization of raw materials, work-in-progress (WIP), and finished goods.
Firstly, efficient inventory control helps manufacturing companies minimize costs. Excess inventory ties up capital, increases storage expenses and leads to obsolescence and waste. On the other hand, insufficient inventory can result in stockouts, production delays, and missed sales opportunities. By maintaining optimal inventory levels, companies can reduce carrying costs while ensuring uninterrupted production and customer satisfaction.
Secondly, accurate inventory control enables improved production planning and resource allocation. By having real-time visibility into inventory levels, manufacturing leaders can effectively schedule production activities, allocate resources, and streamline the supply chain. This leads to better utilization of assets, reduced lead times, and improved operational efficiency.
Moreover, efficient inventory control enhances customer satisfaction. Having the right products available at the right time is crucial for meeting customer demands and delivering on-time orders. By leveraging accurate inventory data, manufacturers can avoid stockouts, backorders, and delays, thereby improving customer loyalty and retention.
Furthermore, inventory control is closely tied to forecasting and demand planning. By analyzing historical data, market trends, and customer preferences, manufacturing leaders can utilize inventory control systems powered by AI and machine learning to accurately forecast demand. This enables proactive decision-making, such as adjusting production volumes, optimizing procurement, and anticipating market fluctuations.
In summary, efficient inventory control in the manufacturing industry ensures cost reduction, improved production planning, enhanced customer satisfaction, and optimized resource allocation. Embracing advanced technologies, such as AI, can further revolutionize inventory control processes, enabling manufacturing leaders to stay competitive in a dynamic market environment.
The Traditional Methods of Inventory Management and Their Limitations
Traditional methods of inventory management have been widely used in the manufacturing industry, but they come with certain limitations. Some of the common traditional methods include:
- Manual Tracking: This involves manually recording inventory data, such as item counts, stock movement, and replenishment orders. While it may work for small-scale operations, manual tracking becomes cumbersome and prone to errors as the scale of inventory increases. Data entry mistakes, delays in updating records, and lack of real-time visibility can lead to inaccurate inventory information.
- Periodic Inventory Audits: Periodic physical inventory audits involve conducting manual counts of all items in stock at predetermined intervals, such as annually or quarterly. While audits can help identify discrepancies and reconcile inventory, they are time-consuming, disrupt operations, and provide only a snapshot of inventory levels at a specific point in time. This limits the ability to have real-time insights and react quickly to changes in demand or supply.
- Economic Order Quantity (EOQ) Model: The EOQ model calculates the optimal order quantity that minimizes total inventory costs, including holding costs and ordering costs. While it provides a theoretical framework for determining order quantities, it assumes stable demand patterns and fixed costs. In reality, demand fluctuates, and costs may vary, rendering the EOQ model less effective in dynamic manufacturing environments.
- Safety Stock: Safety stock is a buffer quantity of inventory maintained to mitigate uncertainties, such as unexpected demand spikes or supply chain disruptions. Traditional methods often rely on static safety stock levels, which may not accurately reflect changing market conditions or customer demands. This can result in either excess safety stock, tying up capital, or inadequate safety stock, leading to stockouts.
- Excel Spreadsheets: Many manufacturing companies still rely on manual data entry and Excel spreadsheets to manage inventory. While spreadsheets offer flexibility and familiarity, they are prone to errors, lack real-time data integration, and have limited capabilities for advanced analytics and forecasting.
These traditional methods of inventory management have limitations in terms of accuracy, real-time visibility, scalability, and adaptability to dynamic market conditions. They often result in suboptimal inventory levels, increased carrying costs, stockouts, and customer dissatisfaction.
To overcome these limitations, manufacturing leaders are turning to advanced technologies like AI and automation to revolutionize their inventory control processes.
Potential of AI in Revolutionizing Inventory Control
Artificial Intelligence (AI) has tremendous potential in revolutionizing inventory control. By leveraging AI technologies, manufacturing companies can overcome the limitations of traditional inventory management methods and achieve greater accuracy, efficiency, and cost savings.
Here are some key ways AI can transform inventory control:
- Data Analysis and Predictive Modeling: AI algorithms can analyze vast amounts of historical data, market trends, and customer behavior to identify patterns and forecast demand more accurately. This enables manufacturing leaders to optimize inventory levels, anticipate demand fluctuations, and make proactive decisions regarding procurement, production scheduling, and replenishment.
- Real-time Monitoring and Decision-making: AI-powered sensors, Internet of Things (IoT) devices, and connected systems can provide real-time visibility into inventory levels, locations, and conditions. This allows manufacturing leaders to monitor stock levels, track shipments, and identify potential bottlenecks or stockouts. With real-time data, they can make informed decisions quickly, such as reallocating inventory, adjusting production plans, or expediting orders.
- Automated Replenishment and Optimization: AI algorithms can automate the replenishment process by dynamically calculating optimal reorder points, safety stock levels, and reorder quantities based on demand patterns, lead times, and service level targets. This reduces the manual effort required for inventory management and ensures optimal stock levels to meet customer demands while minimizing costs.
- Supply Chain Optimization: AI can optimize the entire supply chain by analyzing data from multiple sources, including suppliers, distributors, and logistics partners. By identifying inefficiencies, optimizing routes, minimizing lead times, and synchronizing operations, AI helps streamline the supply chain, reduce stockouts, and improve overall inventory management.
- Exception Handling and Anomaly Detection: AI systems can automatically detect anomalies, such as unusual demand patterns, quality issues, or supply disruptions. By flagging these exceptions, manufacturing leaders can quickly investigate and take corrective actions, minimizing the impact on inventory control and ensuring smooth operations.
- Advanced Analytics and Insights: AI-powered analytics can generate valuable insights from inventory data, identifying trends, optimizing SKU rationalization, and identifying slow-moving or obsolete inventory. These insights enable manufacturing leaders to make data-driven decisions, improve profitability, and reduce waste.
Overall, AI revolutionizes inventory control for manufacturing leaders by enabling accurate demand forecasting, real-time monitoring, automated decision-making, supply chain optimization, exception handling, and advanced analytics. By embracing AI technologies, manufacturing companies can achieve more efficient and cost-effective inventory management, leading to improved operational performance and customer satisfaction.
The Challenges of Inventory Control in Manufacturing
Inventory control in the manufacturing industry comes with its fair share of challenges that can significantly impact production, customer satisfaction, and overall profitability. From maintaining optimal stock levels to addressing demand fluctuations and supply chain complexities, manufacturing leaders face a range of hurdles in effectively managing their inventory.
In this section, we will explore the challenges associated with inventory control in manufacturing and highlight the importance of overcoming these obstacles for achieving operational excellence.
Complex nature of inventory management in the manufacturing sector
Inventory management in the manufacturing sector is inherently complex due to several factors that manufacturing leaders must navigate. These complexities arise from the unique characteristics of the industry and the intricacies involved in handling various types of inventory.
Here are some key aspects that contribute to the complex nature of inventory management in manufacturing:
- Multi-level Inventory: Manufacturing companies typically deal with multiple levels of inventory, including raw materials, work-in-progress (WIP), and finished goods. Each level requires careful monitoring and control to ensure smooth production flow, minimize stockouts, and avoid excessive carrying costs. Coordinating inventory levels across these different stages adds complexity to the management process.
- Seasonality and Demand Fluctuations: Manufacturing often faces seasonality and demand fluctuations, making it challenging to accurately forecast and plan inventory levels. Products may have varying demand patterns influenced by factors such as holidays, consumer trends, or economic conditions. Managing inventory to meet fluctuating demand while avoiding overstocking or stockouts requires a proactive approach.
- Supply Chain Complexity: Manufacturing companies typically operate within complex supply chains involving multiple suppliers, distributors, and logistics partners. Coordinating inventory management across the supply chain is crucial to ensure timely deliveries, minimize lead times, and prevent disruptions. Effective collaboration and information sharing with supply chain partners are essential but can be challenging to achieve.
- SKU Proliferation: Manufacturing often involves a wide range of products or SKUs (Stock Keeping Units) with different attributes, variants, and configurations. Managing inventory for a diverse portfolio of SKUs adds complexity in terms of accurate demand forecasting, storage requirements, and SKU rationalization. Without proper control, SKU proliferation can lead to excess inventory, increased costs, and inefficient use of resources.
- Shelf-Life and Perishability: Some manufacturing industries deal with perishable or time-sensitive products with limited shelf-life, such as food, pharmaceuticals, or chemicals. Managing inventory with expiration dates or perishability constraints requires precise tracking, rotation, and effective first-in-first-out (FIFO) or first-expired-first-out (FEFO) methods. Failure to handle such inventory properly can result in waste, stock obsolescence, or quality issues.
- Balancing Cost and Service Level: Manufacturing leaders face the challenge of balancing inventory costs with service levels. On one hand, carrying excess inventory ties up capital increases storage costs, and raises the risk of obsolescence. On the other hand, insufficient inventory leads to stockouts, production delays, and dissatisfied customers. Striking the right balance between cost and service level is critical but requires robust inventory control strategies.
Navigating these complexities requires manufacturing leaders to adopt advanced inventory management techniques, leverage data-driven insights, and embrace technology solutions such as AI and automation.
Impact of poor inventory control on production, customer satisfaction, and profitability
Poor inventory control in the manufacturing industry can have a significant impact on production, customer satisfaction, and overall profitability. Here are the key areas where the consequences of inadequate inventory control become apparent:
- Production Disruptions: Insufficient inventory can lead to production disruptions and delays. Stockouts of critical raw materials or components can halt manufacturing processes, resulting in missed production targets and increased lead times. This not only affects the ability to fulfill customer orders on time but also disrupts the entire production schedule, leading to inefficiencies and increased costs.
- Increased Carrying Costs: Poor inventory control often results in excess inventory levels. Holding excess stock ties up capital and incurs additional costs associated with storage, handling, and insurance. The longer inventory sits idle, the higher the carrying costs become, negatively impacting overall profitability.
- Stockouts and Lost Sales: Inaccurate inventory control can result in stockouts, where products are not available when customers demand them. Stockouts lead to dissatisfied customers, lost sales opportunities, and potential damage to the company's reputation. Customers may turn to competitors for their needs, leading to customer attrition and revenue loss.
- Expedited Shipping and Additional Costs: In cases of inventory shortages or urgent customer orders, manufacturing companies may have to resort to expedited shipping or special arrangements to fulfill orders on time. These additional costs, including premium freight charges, overtime wages, or last-minute sourcing, erode profit margins and impact the overall cost structure.
- Inefficient Use of Resources: Poor inventory control can result in an inefficient use of resources. Excess inventory may require larger storage facilities, additional handling equipment, or increased manpower. On the other hand, inadequate inventory control can lead to underutilization of resources, resulting in higher per-unit production costs and reduced efficiency.
- Forecasting Inaccuracy: Inaccurate inventory control can compromise demand forecasting accuracy. Without reliable inventory data, forecasting models may produce unreliable projections, leading to suboptimal production planning, inventory imbalances, and inaccurate procurement decisions. This can further exacerbate the issues mentioned above.
Overall, poor inventory control negatively impacts production schedules, customer satisfaction, and profitability in the manufacturing industry. It disrupts operations, increases costs, leads to stockouts, and hampers the ability to meet customer demands.
Challenges faced by manufacturing leaders in maintaining optimal inventory levels
Manufacturing leaders face several challenges when it comes to maintaining optimal inventory levels. These challenges stem from various factors and can significantly impact the efficiency and profitability of their operations. Here are some key challenges faced by manufacturing leaders in inventory management:
Demand Forecasting Accuracy: Accurately predicting customer demand is essential for maintaining optimal inventory levels. However, forecasting demand in a dynamic market can be challenging. Fluctuating market conditions, changing customer preferences, and unpredictable external factors can make demand forecasting less reliable. Manufacturing leaders must invest in advanced forecasting techniques, leverage historical data and market insights, and continuously monitor and adjust their forecasts to overcome this challenge.
Supply Chain Variability: Manufacturing companies often rely on complex supply chains with multiple suppliers, transportation partners, and distributors. Variability within the supply chain, such as fluctuating lead times, delivery delays, or quality issues, can disrupt inventory management. These uncertainties make it difficult to maintain optimal inventory levels and necessitate effective collaboration, visibility, and communication with supply chain partners.
Inventory Visibility and Data Accuracy: Lack of real-time visibility into inventory levels and inaccurate data can hinder inventory control efforts. Manufacturing leaders may struggle to track inventory accurately across multiple locations, warehouses, or production stages. Incomplete or outdated information can lead to imbalances, stockouts, or overstocking. Implementing robust inventory tracking systems, integrating data sources, and ensuring data accuracy through regular audits and quality control processes are crucial in addressing this challenge.
Seasonality and Product Lifecycles: Manufacturing industries often experience seasonality or have products with limited lifecycles. This introduces additional complexities in inventory management, as demand fluctuates over time. Manufacturers must anticipate seasonality trends, adjust production schedules, and manage inventory levels accordingly to avoid stockouts during peak periods or excess inventory during low-demand periods.
SKU Proliferation and Complexity: Manufacturing companies often deal with a wide range of products with different variants, configurations, and attributes. Managing inventory for a large number of SKUs adds complexity. Each SKU requires accurate tracking, demand forecasting, and procurement planning. SKU proliferation can lead to excess inventory, increased carrying costs, and challenges in optimizing stock levels for each product variant.
Cost Constraints and Capital Allocation: Manufacturing leaders often face budgetary constraints and need to optimize their capital allocation. Striking the right balance between inventory investment and working capital is crucial. Limited financial resources may hinder their ability to maintain optimal inventory levels, leading to compromises in service levels or increased risks of stockouts.
Overcoming these challenges requires a combination of effective inventory control strategies, technology adoption, data-driven decision-making, and collaboration with supply chain partners. By addressing these challenges, manufacturing leaders can achieve efficient inventory management, reduce costs, enhance customer satisfaction, and improve overall profitability.
The Role of AI in Inventory Control
The advent of Artificial Intelligence (AI) has brought transformative possibilities to the field of inventory control. By harnessing the power of advanced algorithms and machine learning, AI offers manufacturing leaders unprecedented capabilities to optimize inventory levels, enhance forecasting accuracy, and streamline supply chain operations.
In this section, we will delve into the role of AI in inventory control and explore how it revolutionizes traditional approaches, empowering manufacturing leaders to make data-driven decisions and achieve greater efficiency, profitability, and customer satisfaction.
How AI technology can transform inventory control processes
AI technology has the potential to revolutionize inventory control processes in the manufacturing industry. By leveraging advanced algorithms and machine learning, AI can improve efficiency, accuracy, and decision-making.
Here's a brief explanation of how AI can transform inventory control:
AI enables accurate demand forecasting by analyzing historical data, market trends, and external factors, helping optimize inventory levels and minimize stockouts. Real-time inventory monitoring, facilitated by AI-powered sensors and IoT devices, provides visibility into inventory levels, locations, and conditions, enabling proactive actions to prevent stockouts or overstock situations.
AI algorithms optimize replenishment decisions by considering lead times, demand variability, and cost constraints, aligning inventory levels with changing demand patterns and minimizing holding costs.
Enhanced supply chain visibility and collaboration are achieved through AI, integrating data from various stakeholders to improve coordination, identify bottlenecks, optimize logistics, and enhance overall supply chain efficiency.
AI predicts equipment failures and maintenance needs, reducing production disruptions by enabling proactive maintenance scheduling based on real-time data and analysis.
Advanced analytics powered by AI provide deeper insights into inventory performance, trends, and optimization opportunities, enabling proactive decision-making, SKU rationalization, and better resource allocation.
By leveraging AI technology, manufacturing companies can improve demand forecasting accuracy, enhance real-time monitoring, optimize replenishment decisions, enable predictive maintenance, leverage advanced analytics, and ultimately reduce costs, improve customer satisfaction, increase operational efficiency, and gain a competitive edge.
Capabilities of AI, such as data analysis, predictive modeling, and real-time monitoring
AI offers several capabilities that revolutionize inventory control processes. Three key capabilities are data analysis, predictive modeling, and real-time monitoring. Let's discuss each of these capabilities:
Data Analysis: AI excels at analyzing vast amounts of data quickly and efficiently. In inventory control, AI can process historical sales data, market trends, customer behavior, and other relevant information to derive valuable insights. By identifying patterns, correlations, and anomalies within the data, AI algorithms can uncover hidden relationships and provide valuable information for decision-making.
Data analysis helps manufacturing leaders understand demand patterns, optimize inventory levels, identify cost-saving opportunities, and make informed business decisions.
Predictive Modeling: AI enables predictive modeling by utilizing historical data and machine learning techniques. By analyzing historical sales data, market trends, seasonality, and other relevant variables, AI algorithms can build predictive models that forecast future demand. These models take into account various factors, such as product lifecycle, customer behavior, economic conditions, and external influences, to generate accurate predictions.
Predictive modeling helps manufacturing leaders anticipate demand fluctuations, optimize inventory levels, and make proactive decisions to prevent stockouts or excess inventory.
Real-time Monitoring: AI facilitates real-time monitoring of inventory levels, locations, and conditions. Through the integration of sensors, IoT devices, and data analytics, AI systems can provide up-to-date visibility into inventory status. Real-time monitoring helps manufacturing leaders track inventory movements, identify potential stockouts or overstock situations, and take immediate action.
By receiving alerts and notifications, they can address issues promptly, adjust production schedules, or reorder supplies in a timely manner. Real-time monitoring enhances responsiveness, reduces inventory holding costs, and ensures efficient inventory management.
These capabilities of AI—data analysis, predictive modeling, and real-time monitoring—empower manufacturing leaders with valuable insights, accurate demand forecasting, and proactive decision-making.
By leveraging these capabilities, companies can optimize inventory levels, reduce costs, improve customer satisfaction, and enhance overall operational efficiency in the manufacturing industry.
Benefits of using AI for inventory control, including improved accuracy, efficiency, and cost savings
Using AI for inventory control in the manufacturing industry offers several benefits, including:
Improved Accuracy: AI algorithms can analyze large volumes of data and identify patterns and trends that humans might overlook. This leads to more accurate demand forecasting, inventory planning, and replenishment decisions.
By minimizing forecasting errors and reducing stockouts or overstock situations, businesses can improve their customer service levels and avoid unnecessary costs associated with inventory imbalances.
Enhanced Efficiency: AI automates repetitive tasks and streamlines inventory control processes, resulting in increased efficiency. AI systems can monitor inventory levels, track sales data, and adjust reorder points in real-time. This automation reduces manual effort and human errors, allowing employees to focus on more strategic activities.
Improved efficiency leads to better utilization of resources, optimized production schedules, and streamlined supply chain operations.
Cost Savings: AI-enabled inventory control helps reduce costs in several ways. Accurate demand forecasting minimizes inventory holding costs by ensuring optimal stock levels and preventing excess inventory.
Real-time monitoring and data analysis enable businesses to identify and resolve supply chain inefficiencies promptly, reducing wastage and minimizing production downtime. Additionally, AI can optimize logistics and transportation routes, leading to cost savings in the distribution process.
Enhanced Customer Satisfaction: AI-driven inventory control improves customer satisfaction by ensuring product availability and timely deliveries. Accurate demand forecasting helps meet customer demands efficiently, reducing instances of stockouts and backorders.
With AI, businesses can maintain optimal inventory levels, minimizing the risk of delayed shipments or unfulfilled orders. Improved customer satisfaction strengthens customer loyalty, increases repeat business, and enhances the overall brand reputation.
Data-Driven Decision Making: AI provides valuable insights and actionable recommendations based on data analysis. By leveraging AI's capabilities, manufacturing leaders can make informed decisions about pricing strategies, product assortment, and inventory optimization.
AI-driven analytics enable businesses to identify trends, anticipate market changes, and adapt their inventory management strategies accordingly. Data-driven decision making leads to improved profitability, better resource allocation, and a competitive advantage in the market.
In summary, utilizing AI for inventory control offers improved accuracy in demand forecasting, enhanced operational efficiency, cost savings, and increased customer satisfaction. By harnessing AI technology, manufacturing companies can optimize their inventory management processes, reduce costs, improve customer service levels, and gain a competitive edge in the industry.
AI Applications in Inventory Control
In the realm of inventory control, the potential of Artificial Intelligence (AI) is vast. AI offers a multitude of applications that can revolutionize how businesses manage their inventory. In this section, we will explore the diverse range of AI applications in inventory control, from demand forecasting and optimization to predictive maintenance and supply chain visibility.
By harnessing the power of AI, businesses can unlock new levels of efficiency, accuracy, and profitability in their inventory management processes.
AI applications that can revolutionize inventory control for manufacturing leaders
AI applications have the potential to revolutionize inventory control for manufacturing leaders by introducing advanced capabilities that enhance efficiency, accuracy, and decision-making. Here are some key AI applications that can drive this revolution:
- Demand Forecasting: AI algorithms can analyze historical sales data, market trends, and external factors to generate accurate demand forecasts. This helps manufacturing leaders optimize inventory levels, minimize stockouts, and reduce excess inventory.
- Replenishment Optimization: AI algorithms optimize replenishment decisions by considering factors such as lead times, demand variability, and cost constraints. This ensures that inventory levels align with changing demand patterns and minimize holding costs.
- Real-time Monitoring: AI-powered sensors and IoT devices provide real-time visibility into inventory levels, locations, and conditions. This enables proactive actions to address potential stockouts or overstock situations and ensures timely inventory management.
- Predictive Maintenance: AI can predict equipment failures and maintenance needs by monitoring equipment performance and analyzing sensor data in real-time. This reduces production disruptions caused by machine downtime and optimizes inventory control.
- Supply Chain Visibility and Collaboration: AI technology facilitates enhanced supply chain visibility and collaboration by integrating data from various stakeholders. This helps identify bottlenecks, optimize logistics, and improve overall supply chain efficiency.
- Analytics and Optimization: AI-powered analytics enable manufacturing leaders to gain deeper insights into inventory performance, identify optimization opportunities, and make data-driven decisions. This improves overall inventory control efficiency.
- Risk Management: AI can assess and mitigate inventory-related risks by identifying potential disruptions, such as supplier delays or demand fluctuations. By proactively addressing these risks, manufacturing leaders can ensure a more resilient and efficient inventory management process.
By leveraging these AI applications, manufacturing leaders can achieve greater efficiency, accuracy, and cost-effectiveness in their inventory control processes. AI empowers them to make data-driven decisions, optimize inventory levels, enhance supply chain visibility, and maintain a competitive edge in the manufacturing industry.
Inventory demand forecasting: How AI algorithms can analyze historical data and market trends to predict future demand
AI algorithms can analyze historical data and market trends to predict future demand accurately through a process known as inventory demand forecasting. Here's how AI algorithms leverage historical data and market trends to generate accurate demand forecasts:
- Data Collection: AI algorithms collect and aggregate historical data on product sales, customer behavior, market trends, and other relevant variables. This data includes information on sales volume, seasonal patterns, promotional activities, economic indicators, and external factors that may influence demand.
- Data Preprocessing: Before analyzing the data, AI algorithms preprocess it by cleaning, transforming, and normalizing it to ensure consistency and remove any inconsistencies or outliers that could skew the results. This step enhances the quality of the data used for analysis.
- Pattern Recognition: AI algorithms use various techniques, such as machine learning and statistical modeling, to identify patterns, correlations, and relationships within the historical data. By examining factors such as seasonality, trends, and demand fluctuations, the algorithms uncover meaningful insights that impact demand.
- External Factors Integration: AI algorithms also consider external factors that may influence demand, such as economic indicators, weather patterns, competitor activities, and market trends. By incorporating these factors into the analysis, the algorithms can capture the broader context and make more accurate demand predictions.
- Machine Learning and Predictive Modeling: AI algorithms employ machine learning techniques and predictive modeling to build forecasting models based on the historical data and identified patterns. These models can range from simple regression models to more complex algorithms like time series analysis, neural networks, or ensemble methods.
- Forecast Generation: Once the models are built, AI algorithms generate demand forecasts by applying the trained models to new data inputs. The algorithms take into account historical patterns, market trends, and external factors to generate accurate predictions of future demand levels.
- Iterative Refinement: AI algorithms continuously learn and improve over time. As new data becomes available, the algorithms can update their models, retrain them, and refine the forecasts to ensure ongoing accuracy.
By leveraging AI algorithms, businesses can obtain accurate demand forecasts that help optimize inventory levels, minimize stockouts and excess inventory, and make informed decisions about production, procurement, and supply chain management.
Real-time inventory monitoring: How AI-powered sensors and IoT devices can provide real-time data on inventory levels
AI-powered sensors and IoT devices play a crucial role in providing real-time inventory monitoring, enabling proactive decision-making in inventory control. Here's how these technologies work together:
Sensor Integration: AI-powered sensors are integrated into the inventory storage areas, shelves, or even individual products. These sensors are equipped with various capabilities such as barcode scanning, RFID (Radio Frequency Identification) tracking, or weight sensors to capture relevant inventory data.
Data Collection and Transmission: The sensors continuously collect data on inventory levels, movement, and conditions. This data is transmitted in real-time to a central database or cloud platform using IoT connectivity protocols such as Wi-Fi, Bluetooth, or cellular networks. The collected data includes information on the quantity, location, and status of each inventory item.
Data Processing and Analysis: AI algorithms receive the real-time data from the sensors and process it for analysis. The algorithms can interpret the data, identify patterns, and generate meaningful insights about inventory levels and trends. This analysis can include metrics such as stock levels, order fulfillment rates, or even demand patterns.
Alert Generation: Based on the analysis, AI algorithms can trigger alerts or notifications when certain inventory thresholds or conditions are met. For example, if inventory levels are falling below a predefined threshold, an alert can be sent to inventory managers to take proactive actions, such as initiating a reorder or adjusting production schedules.
Predictive Analytics: AI algorithms can also leverage historical and real-time data to perform predictive analytics. By considering factors like seasonality, historical demand patterns, and market trends, the algorithms can predict future inventory needs and potential stockouts or overstock situations. This enables proactive decision-making in inventory planning and management.
Proactive Decision-Making: With real-time inventory data and insights provided by AI-powered sensors and IoT devices, inventory managers can make proactive decisions. They can adjust inventory levels, reorder supplies in a timely manner, optimize production schedules, or allocate resources more effectively. By acting proactively based on real-time data, businesses can avoid stockouts, reduce excess inventory, and improve overall inventory control efficiency.
Integration with Inventory Management Systems: The real-time data from AI-powered sensors can be seamlessly integrated with inventory management systems or enterprise resource planning (ERP) systems. This integration ensures that the inventory information is readily available to inventory managers and other stakeholders, allowing for better coordination and decision-making across the organization.
This real-time data enables proactive decision-making, timely actions, and optimized inventory control, leading to improved operational efficiency, reduced costs, and enhanced customer satisfaction.
Supply chain optimization: How AI algorithms can optimize supply chain operations by identifying bottlenecks
AI algorithms can play a significant role in optimizing supply chain operations by identifying bottlenecks, optimizing routes, and minimizing lead times. Here's how AI can contribute to supply chain optimization:
- Data Integration: AI algorithms integrate data from various sources across the supply chain, including suppliers, manufacturers, distributors, and retailers. This data encompasses information such as order history, transportation data, inventory levels, production schedules, and customer demand.
- Demand Forecasting: AI algorithms leverage historical data, market trends, and external factors to accurately forecast demand. By predicting demand patterns, businesses can optimize production schedules, inventory levels, and resource allocation, minimizing stockouts and overstocks.
- Inventory Optimization: AI algorithms analyze data on inventory levels, sales patterns, and lead times to optimize inventory management. By dynamically adjusting reorder points, safety stock levels, and replenishment quantities, AI algorithms ensure optimal inventory levels while minimizing holding costs and stockouts.
- Route Optimization: AI algorithms optimize transportation routes by considering factors such as distance, capacity, traffic, and delivery constraints. By analyzing real-time data and historical patterns, AI algorithms can determine the most efficient routes for deliveries, reducing transportation costs and lead times.
- Supplier Management: AI algorithms analyze supplier performance data, quality metrics, and delivery times to identify the most reliable and efficient suppliers. By optimizing the selection and management of suppliers, businesses can ensure timely deliveries, quality assurance, and cost savings.
- Risk Management: AI algorithms analyze data on various risks, including supplier disruptions, natural disasters, or market volatility. By identifying potential risks, AI algorithms enable businesses to implement contingency plans, diversify suppliers, and minimize disruptions to the supply chain.
- Predictive Analytics: AI algorithms leverage predictive analytics to anticipate future demand, supply chain bottlenecks, and potential disruptions. By simulating scenarios and evaluating different strategies, AI algorithms help businesses make proactive decisions to optimize supply chain operations.
- Continuous Improvement: AI algorithms continuously learn from new data and adjust their models and recommendations accordingly. By analyzing performance metrics and feedback, AI algorithms can identify areas for improvement and suggest optimization strategies for the supply chain.
This leads to improved operational efficiency, reduced costs, enhanced customer satisfaction, and a more competitive position in the market. The ability of AI to process and analyze vast amounts of data enables businesses to make data-driven decisions and continuously improve their supply chain operations.
Case Studies of Successful AI Implementation in Inventory Control
In this section, we delve into real-world case studies that highlight the successful implementation of AI in inventory control. These case studies demonstrate how AI technologies have been leveraged by manufacturing leaders to overcome inventory management challenges, improve operational efficiency, and achieve remarkable results.
By examining these examples, we can gain valuable insights into the practical applications of AI in inventory control and understand the transformative impact it can have on businesses in the manufacturing industry.
Real-world examples of manufacturing companies that have successfully implemented ai for inventory control
Here are a few real-world examples of manufacturing companies that have successfully implemented AI for inventory control:
Amazon: Amazon is renowned for its extensive use of AI in inventory control and supply chain management. The company employs AI algorithms to analyze customer buying patterns, forecast demand, and optimize inventory levels in its fulfillment centers. AI helps Amazon ensure that products are available for customers while minimizing excess inventory and optimizing logistics operations.
Walmart: Walmart has implemented AI-powered inventory management systems that analyze historical sales data, market trends, and external factors to forecast demand accurately. By leveraging AI algorithms, Walmart optimizes replenishment decisions, reduces stockouts, and avoids overstocks. AI also enables the company to streamline its supply chain operations, resulting in improved efficiency and customer satisfaction.
Procter & Gamble (P&G): P&G, a global consumer goods company, has successfully implemented AI for inventory control and demand forecasting. By leveraging AI algorithms, P&G improved its demand forecasting accuracy, leading to optimized inventory levels, reduced stockouts, and enhanced production efficiency. The company also utilized AI to streamline its supply chain, resulting in cost savings and improved customer service.
Siemens: Siemens, a multinational conglomerate, has integrated AI-powered sensors and IoT devices into its manufacturing processes. These devices continuously monitor inventory levels, equipment performance, and supply chain data in real-time. This real-time data is then analyzed using AI algorithms to optimize inventory control, predict maintenance needs, and streamline the supply chain operations.
Zara: Zara, a global fashion retailer, utilizes AI for demand forecasting and inventory optimization. By analyzing sales data, market trends, and customer preferences, Zara's AI algorithms accurately forecast demand, enabling the company to optimize inventory levels and reduce stockouts. Zara's agile production and distribution model combined with AI-driven inventory control have contributed to their success in the fast-paced fashion industry.
These examples highlight how manufacturing companies across different sectors have successfully implemented AI for inventory control. By leveraging AI algorithms, these companies have achieved improved demand forecasting, optimized inventory levels, reduced stockouts, and enhanced operational efficiency.
These success stories demonstrate the transformative potential of AI in revolutionizing inventory control for manufacturing leaders.
Overcoming Implementation Challenges
In the journey of implementing AI for inventory control, businesses often face various challenges that can hinder the successful adoption and integration of AI technologies. In this section, we explore the common implementation challenges encountered by manufacturing leaders and delve into strategies and best practices for overcoming these hurdles.
Potential challenges and barriers to implementing ai for inventory control
Implementing AI for inventory control can present several challenges and barriers that organizations need to address. Here are some common challenges and strategies to overcome them:
- Data Availability and Quality: AI algorithms rely on large volumes of accurate and high-quality data to make accurate predictions. However, organizations may face challenges in collecting and preparing the necessary data. To address this, companies should invest in data collection infrastructure, ensure data accuracy, and implement data cleansing and preprocessing techniques.
- Data Integration and Compatibility: Organizations often have data stored in various systems and formats, making data integration a challenge. It is crucial to establish compatibility between different data sources and ensure seamless data flow. Adopting data integration technologies or utilizing APIs can help overcome these challenges.
- Skill and Knowledge Gap: Implementing AI requires specialized skills and knowledge. Organizations may face challenges in finding employees with AI expertise or training existing staff. Companies can address this by providing training programs, hiring AI experts, or collaborating with external partners who specialize in AI implementation.
- Change Management and Organizational Culture: Implementing AI may require changes in processes, roles, and responsibilities, which can encounter resistance from employees. Effective change management strategies, including communication, employee engagement, and training, can help overcome resistance and foster a culture of innovation and adoption.
- Cost and Investment: Implementing AI involves costs associated with technology infrastructure, software development, and staff training. Organizations should carefully evaluate the return on investment (ROI) and develop a clear business case to justify the expenses. Additionally, exploring cloud-based AI solutions can help reduce infrastructure costs.
- Ethical and Regulatory Considerations: AI implementation must adhere to ethical standards and comply with relevant regulations. Organizations should ensure transparency, fairness, and accountability in AI algorithms and address potential biases or discriminatory outcomes. Collaboration with legal and compliance teams is essential to navigate regulatory frameworks.
- Scalability and Flexibility: AI implementations need to be scalable and adaptable to accommodate changing business needs and growing data volumes. Designing AI systems with scalability in mind, utilizing cloud computing resources, and adopting flexible AI frameworks can help overcome scalability challenges.
- Evaluation and Continuous Improvement: AI implementations should be evaluated regularly to assess their effectiveness and identify areas for improvement. Ongoing monitoring, feedback collection, and iterative refinement are vital to ensure the AI system evolves and delivers value over time.
By proactively addressing these challenges and adopting the right strategies, organizations can overcome barriers to implementing AI for inventory control. Overcoming these challenges enables businesses to harness the full potential of AI technologies and unlock improved efficiency, accuracy, and profitability in their inventory management processes.
The need for data integration, infrastructure upgrades, and employee training
Data integration, infrastructure upgrades, and employee training are essential components when implementing AI for inventory control. Let's discuss the importance of each aspect:
Data Integration: Data integration involves bringing together data from various sources across the organization into a unified system. In the context of inventory control, this includes data on sales, customer demand, production, supply chain, and external factors.
Data integration is crucial because AI algorithms require comprehensive and accurate data to make accurate predictions and optimize inventory levels effectively. By integrating data sources, organizations can gain a holistic view of their inventory and make data-driven decisions.
Infrastructure Upgrades: Implementing AI for inventory control often requires upgrading the technology infrastructure to handle the increased computational requirements and data processing capabilities. AI algorithms consume significant computing resources and require robust storage and processing capabilities.
Upgrading infrastructure may involve investing in cloud computing resources, high-performance servers, and scalable storage systems. Adequate infrastructure ensures that AI algorithms can process and analyze large volumes of data efficiently and in a timely manner.
Employee Training: Introducing AI technologies for inventory control requires providing training and upskilling employees to work effectively with these new tools. Training programs should focus on developing AI literacy, understanding the capabilities and limitations of AI algorithms, and learning how to interpret and utilize the insights generated by AI systems.
By investing in employee training, organizations can empower their workforce to leverage AI tools effectively, make informed decisions, and adapt to the changing landscape of inventory control.
Data integration, infrastructure upgrades, and employee training are interconnected and vital for successful AI implementation in inventory control. Without proper data integration, AI algorithms may not have access to the comprehensive data needed for accurate predictions. Insufficient infrastructure can hinder the performance and scalability of AI systems, limiting their effectiveness.
Additionally, without proper employee training, organizations may struggle to fully leverage the capabilities of AI and may face resistance or inefficiencies in adopting AI-driven inventory control processes.
By addressing these needs, organizations can establish a strong foundation for AI implementation in inventory control. It enables businesses to harness the power of data, optimize their inventory management processes, and gain a competitive edge in the manufacturing industry.
Provide recommendations for manufacturing leaders to overcome these challenges effectively
Manufacturing leaders can take the following recommendations to effectively overcome the challenges of data integration, infrastructure upgrades, and employee training when implementing AI for inventory control:
Develop a Comprehensive Data Strategy: Define a clear data strategy that outlines the types of data needed, data sources, data quality standards, and data integration processes. Establish data governance practices to ensure data accuracy, consistency, and security. Collaborate with IT and data teams to implement data integration tools and technologies that facilitate seamless data flow across systems.
Prioritize Infrastructure Investments: Conduct a thorough assessment of your existing infrastructure to identify gaps and scalability limitations. Determine the computing power, storage, and network requirements for AI implementation. Consider cloud-based solutions that offer scalability, flexibility, and cost-effectiveness. Collaborate with IT teams and technology providers to design and implement a robust infrastructure that can support AI-driven inventory control processes.
Foster a Culture of Continuous Learning: Emphasize the importance of employee training and upskilling. Offer training programs that cover AI fundamentals, inventory control concepts, and hands-on experience with AI tools and technologies. Encourage employees to participate in workshops, webinars, and industry conferences to stay updated with the latest trends and best practices. Foster a culture that promotes curiosity, experimentation, and learning from AI-driven insights.
Create Cross-functional Teams: Form cross-functional teams comprising individuals from different departments, such as IT, operations, supply chain, and data analytics. These teams can collaborate to address data integration challenges, infrastructure upgrades, and employee training needs. Encourage knowledge sharing and collaboration among team members to leverage diverse expertise and ensure a holistic approach to AI implementation.
Collaborate with External Partners: Engage with external partners, consultants, or AI technology vendors who specialize in AI implementation for inventory control. Leverage their expertise and experience to guide you through the challenges and ensure a smooth implementation process. External partners can provide valuable insights, offer training programs, and assist in the selection and implementation of suitable AI technologies.
Start with Pilot Projects: Begin with small-scale pilot projects to test the effectiveness of AI technologies and processes. Identify a specific inventory control challenge or area for improvement and apply AI algorithms to address it. Evaluate the results, gather feedback, and iterate on the implementation before scaling up to larger operations. Pilot projects help validate the benefits of AI and build confidence among stakeholders.
Monitor Performance and Iterate: Continuously monitor the performance of AI systems and collect feedback from users. Measure key performance indicators (KPIs) such as inventory accuracy, stockout rates, and order fulfillment time. Use this data to identify areas for improvement and make iterative adjustments to the AI algorithms, data integration processes, infrastructure, and employee training programs.
By following these recommendations, manufacturing leaders can navigate the challenges of data integration, infrastructure upgrades, and employee training effectively. They can establish a strong foundation for successful AI implementation in inventory control, leading to optimized operations, improved decision-making, and enhanced business performance.
Transformative Potential of AI in Revolutionizing Inventory Control for Manufacturing Leaders
The transformative potential of AI in revolutionizing inventory control for manufacturing leaders is significant. AI technologies offer a range of capabilities that can enhance operational efficiency, improve decision-making, and drive business growth. By leveraging AI in inventory control, manufacturing leaders can unlock numerous benefits and stay ahead in today's competitive landscape.
First and foremost, AI enables accurate demand forecasting by analyzing historical data, market trends, and external factors. AI algorithms can identify patterns and relationships in vast amounts of data, allowing manufacturers to predict future demand with greater precision. This leads to optimized inventory levels, reduced stockouts, and improved customer satisfaction.
Real-time monitoring is another area where AI can revolutionize inventory control. By integrating AI-powered sensors and IoT devices, manufacturing leaders can gather real-time data on inventory levels, equipment performance, and supply chain activities. This real-time visibility enables proactive decision-making, early detection of issues, and swift response to changes in demand or supply. Consequently, manufacturers can optimize inventory replenishment, minimize lead times, and maintain seamless operations.
AI's data analysis capabilities empower manufacturing leaders to gain valuable insights from large and complex datasets. AI algorithms can process and analyze data from various sources, such as sales records, production data, and customer feedback, to identify trends, patterns, and anomalies. These insights provide a deeper understanding of inventory dynamics, supplier performance, and customer behavior, enabling proactive decision-making and strategic planning.
Moreover, AI-powered predictive modeling allows manufacturing leaders to simulate different scenarios and evaluate the impact of various inventory control strategies. By considering factors like seasonality, market trends, and production constraints, AI algorithms can recommend optimal reorder points, production schedules, and safety stock levels. This empowers manufacturers to make informed decisions, balance costs and service levels, and optimize their supply chain operations.
Another transformative aspect of AI in inventory control is its ability to automate routine tasks and streamline processes. By automating inventory monitoring, order processing, and data analysis, AI reduces manual effort and human error. This frees up valuable resources and allows employees to focus on more strategic tasks, such as analyzing insights, optimizing supply chain processes, and improving customer service.
Furthermore, AI can drive cost savings for manufacturing leaders. By optimizing inventory levels, reducing stockouts, and minimizing excess inventory, manufacturers can achieve cost efficiencies and improve overall profitability. AI algorithms can also identify opportunities for process optimization, such as identifying bottlenecks, optimizing routes, and streamlining procurement processes, leading to further cost savings.
In summary, the transformative potential of AI in revolutionizing inventory control for manufacturing leaders is substantial. By harnessing AI's capabilities, businesses can achieve accurate demand forecasting, real-time monitoring, data-driven insights, predictive modeling, process automation, and cost savings.
This enables manufacturers to operate with greater efficiency, respond quickly to market dynamics, and deliver superior customer experiences. Embracing AI in inventory control is becoming increasingly vital for manufacturing leaders to stay competitive and thrive in the fast-paced and evolving business landscape.
Embrace AI Technology and Reap its Benefits in Optimizing Inventory Management Processes
Manufacturing leaders, now is the time to embrace AI technology and unlock its tremendous benefits in optimizing your inventory management processes. By adopting AI, you can gain a competitive edge, drive operational efficiency, and achieve substantial improvements in your business outcomes.
Here's why you should embrace AI technology for inventory control:
- Enhanced Accuracy: AI algorithms can analyze vast amounts of data, including historical sales data, market trends, and external factors, to provide accurate demand forecasting. By leveraging AI's predictive capabilities, you can optimize inventory levels, reduce stockouts, and ensure you have the right products available at the right time.
- Improved Efficiency: AI-powered sensors and IoT devices enable real-time monitoring of inventory levels, equipment performance, and supply chain activities. With instant access to data, you can proactively address issues, optimize replenishment, and minimize lead times. AI automation also streamlines routine tasks, freeing up resources to focus on strategic initiatives.
- Data-Driven Insights: AI's data analysis capabilities allow you to extract valuable insights from complex datasets. By uncovering trends, patterns, and anomalies, you gain a deeper understanding of inventory dynamics, supplier performance, and customer behavior. These insights empower you to make data-driven decisions, identify optimization opportunities, and adapt quickly to changing market conditions.
- Predictive Modeling: AI algorithms enable you to simulate different scenarios and evaluate the impact of inventory control strategies. By considering factors like seasonality, market trends, and production constraints, you can optimize reorder points, production schedules, and safety stock levels. This predictive modeling empowers you to make informed decisions that balance cost-efficiency and service levels.
- Cost Savings: Optimized inventory levels, reduced stockouts, and minimized excess inventory lead to significant cost savings. AI's ability to identify process bottlenecks, optimize routes, and streamline procurement processes further contributes to cost efficiencies. By leveraging AI technology, you can improve profitability and allocate resources more effectively.
- Competitive Advantage: Embracing AI technology in inventory control gives you a competitive edge in the manufacturing industry. With accurate demand forecasting, real-time monitoring, and data-driven insights, you can respond swiftly to market changes, meet customer expectations, and deliver exceptional experiences. By staying ahead of the curve, you position your business for success in a rapidly evolving landscape.
To fully embrace AI technology in inventory control, consider these steps:
- Evaluate your current inventory management processes and identify areas for improvement.
- Develop a clear strategy for implementing AI, including data integration, infrastructure upgrades, and employee training.
- Collaborate with AI experts, technology providers, and external partners to ensure a successful implementation.
- Start with pilot projects to test AI solutions and validate their impact before scaling up.
- Continuously monitor and evaluate AI performance, gathering feedback to refine and optimize your inventory control processes.
Manufacturing leaders who embrace AI technology for inventory control are poised to reap its transformative benefits. The time to act is now. Embrace AI, optimize your inventory management processes, and lead your business towards greater efficiency, profitability, and success in the dynamic manufacturing landscape.
Conclusion
n conclusion, the potential of AI in revolutionizing inventory control for manufacturing leaders is immense. By harnessing the capabilities of AI, manufacturing companies can overcome the limitations of traditional inventory management methods and unlock numerous benefits. Efficient inventory control is crucial for maintaining optimal stock levels, meeting customer demands, and driving profitability.
AI offers transformative solutions to the challenges faced by manufacturing leaders in inventory control. Through data analysis, predictive modeling, and real-time monitoring, AI enables accurate demand forecasting, proactive decision-making, and process optimization. The integration of AI technology empowers manufacturing leaders to make data-driven decisions, streamline operations, and achieve cost savings.
Implementing AI in inventory control does come with challenges, including data integration, infrastructure upgrades, and employee training. However, by developing a comprehensive data strategy, investing in infrastructure upgrades, fostering a culture of continuous learning, and collaborating with external partners, manufacturing leaders can overcome these challenges effectively.
How can Deskera Help You?
Deskera ERP and MRP systems help you to keep your business units organized. The system's primary functions are as follows:
- Keep track of your raw materials and final items inventories
- Control production schedules and routings
- Keep a bill of materials
- Produce thorough reports
- Make your own dashboards
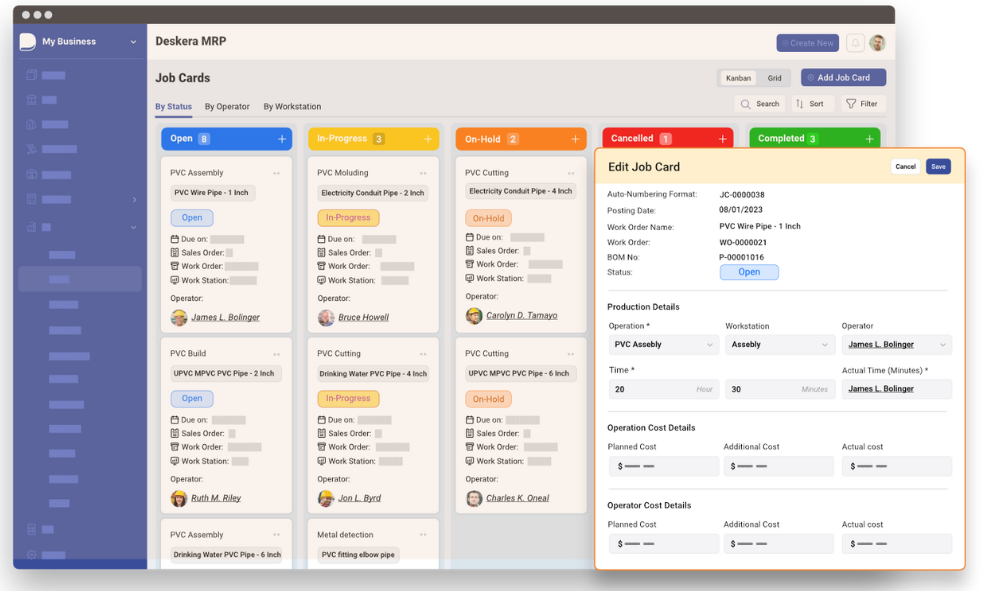
Deskera's integrated financial planning tools enable investors to better plan and track their investments. It can assist investors in making faster and more accurate decisions.
Deskera Books allows you to better manage your accounts and finances. Maintain good accounting practices by automating tasks like billing, invoicing, and payment processing.
Deskera CRM is a powerful solution that manages your sales and helps you close deals quickly. It not only enables you to perform critical tasks like lead generation via email, but it also gives you a comprehensive view of your sales funnel.
Deskera People is a straightforward tool for centralizing your human resource management functions.
Key Takeaways
- Efficient inventory control is crucial for manufacturing leaders as it helps maintain optimal stock levels, meet customer demands, and drive profitability.
- Traditional methods of inventory management have limitations, including manual processes, reliance on historical data, and reactive decision-making.
- AI has the potential to revolutionize inventory control by offering capabilities such as data analysis, predictive modeling, and real-time monitoring.
- AI algorithms can analyze historical data and market trends to accurately predict future demand, leading to optimized inventory levels and reduced stockouts.
- Real-time monitoring using AI-powered sensors and IoT devices enables proactive decision-making by providing instant visibility into inventory levels, equipment performance, and supply chain activities.
- AI algorithms can optimize supply chain operations by identifying bottlenecks, optimizing routes, and minimizing lead times, leading to improved efficiency and cost savings.
- Benefits of using AI for inventory control include improved accuracy in demand forecasting, increased efficiency through automation, and cost savings from optimized inventory levels.
- AI applications in inventory control include demand forecasting, real-time monitoring, supply chain optimization, and predictive modeling.
- Successful implementation of AI in inventory control requires overcoming challenges such as data integration, infrastructure upgrades, and employee training.
- Manufacturing leaders can embrace AI technology by developing a comprehensive data strategy, investing in infrastructure upgrades, fostering a culture of continuous learning, and collaborating with external partners.
Related Articles
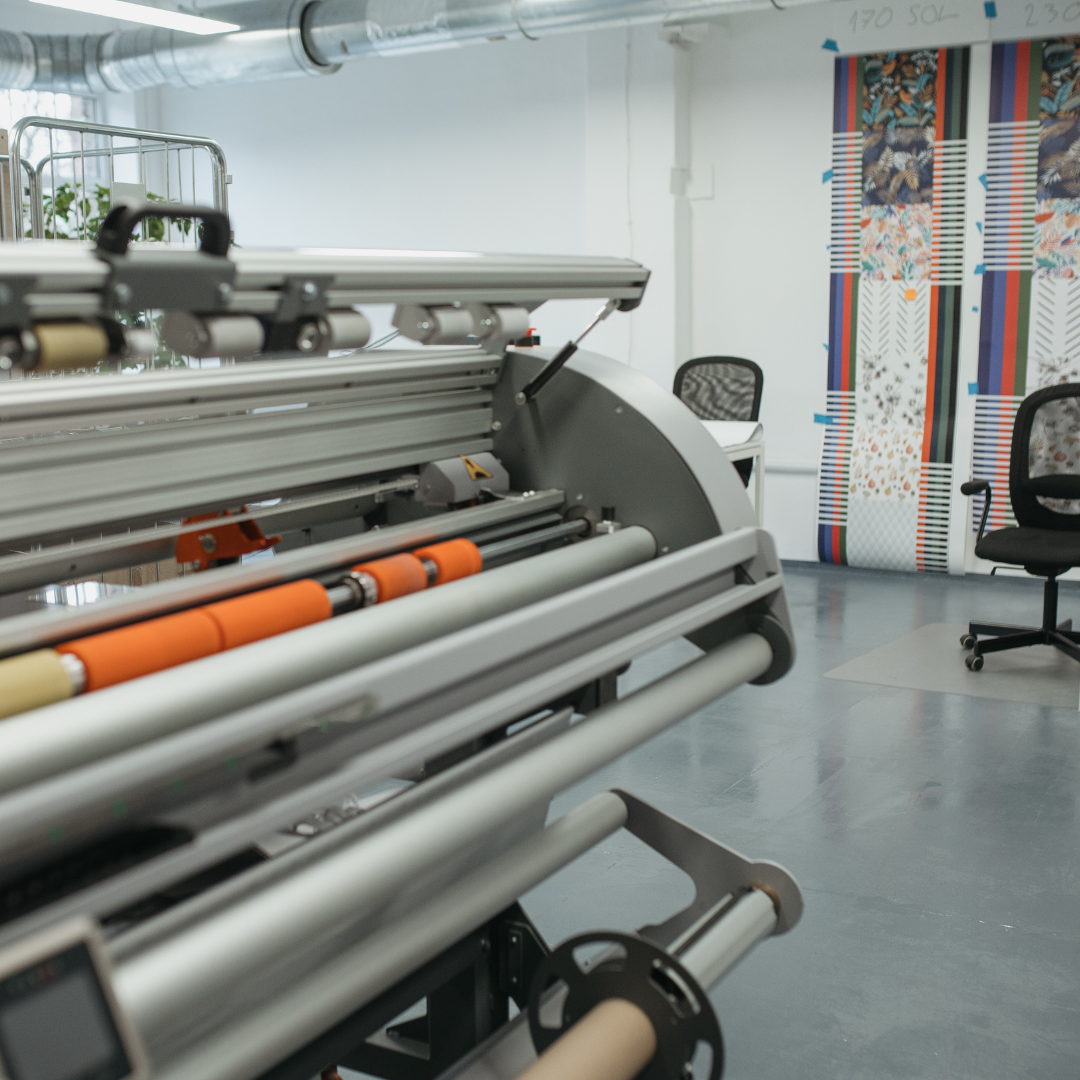
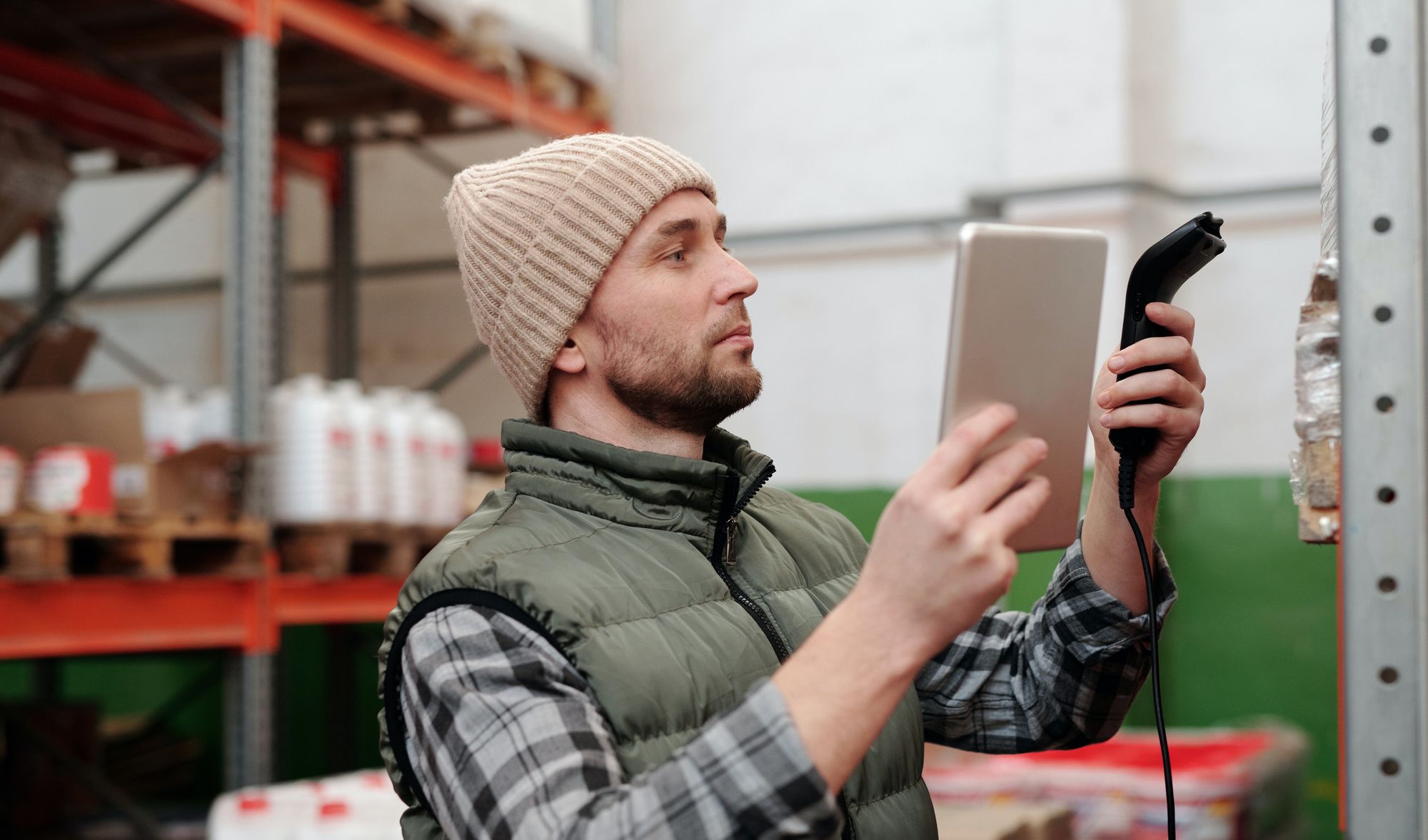