Data-driven demand forecasting is becoming an important tool for manufacturers. By understanding customer behavior, companies can optimize their operations and make more efficient use of resources.
Manufacturers need to forecast demand in order to plan production schedules, allocate resources effectively, and identify opportunities for improvement. The complexity of the data involved makes data-driven demand forecasting a difficult task.
There are several techniques that can be used to improve the accuracy of data-driven demand forecasting. using a data-driven approach can maximize profit by ensuring that products are produced in the most efficient way possible.
Let’s take a look at the table of content below:
- Meaning of Data-Driven Demand Forecasting in Manufacturing
- Importance of Maximizing Profitability in Manufacturing
- Understanding Data-Driven Demand Forecasting
- Leveraging Data for Demand Forecasting in Manufacturing
- Advanced Analytics Techniques for Demand Forecasting
- Enhancing Profitability with Data-Driven Demand Forecasting
- Overcoming Challenges in Data-Driven Demand Forecasting
- Case Studies: Successful Implementation of Data-Driven Demand Forecasting
- Future Trends in Data-Driven Demand Forecasting
- Wrapping Up
- How Deskera Can Assist You?
Let's get started!
Meaning of Data-Driven Demand Forecasting in Manufacturing
Data-driven demand forecasting in manufacturing refers to the process of using data and analytics to predict and estimate future demand for products or services in the manufacturing industry. It involves analyzing historical data, market trends, customer behavior, and other relevant information to generate accurate forecasts of product demand.
By leveraging data and advanced analytics techniques, manufacturers can make informed decisions about production planning, inventory management, supply chain optimization, and pricing strategies. This approach enables them to align their operations with anticipated demand, minimize stockouts and overstocks, improve customer satisfaction, and ultimately maximize profitability.
Data-driven demand forecasting involves collecting and integrating various types of data, such as sales data, market research data, customer data, and external factors like economic indicators or weather patterns.
Furthermore, this data is then processed, cleansed, and analyzed using statistical models, machine learning algorithms, or time series analysis methods to generate reliable demand forecasts.
The forecasts generated through data-driven demand forecasting provide manufacturers with valuable insights into future demand patterns, allowing them to allocate resources efficiently, make informed production decisions, optimize inventory levels, and adapt their operations to meet changing market conditions.
By leveraging data-driven demand forecasting, manufacturers can reduce costs, minimize waste, improve operational efficiency, and ultimately drive profitability in a highly competitive manufacturing landscape.
Importance of Maximizing Profitability in Manufacturing
Maximizing profitability is of paramount importance in the manufacturing industry for several reasons:
Financial Stability: Profitability is crucial for the financial stability of manufacturing companies. Profitable operations ensure that a company has sufficient resources to cover expenses, invest in research and development, upgrade equipment, and fund future growth. It provides a solid foundation for long-term sustainability.
Business Growth and Expansion: Profitability allows manufacturers to reinvest earnings back into the business. This capital can be used to expand production capacities, launch new product lines, enter new markets, or acquire technologies or companies that complement their operations. Profitability fuels business growth and helps manufacturers stay competitive in the industry.
Competitive Advantage: Profitability enables manufacturers to gain a competitive edge over their rivals. It provides the means to invest in innovative technologies, streamline processes, and enhance product quality. Furthermore, these investments can result in cost efficiencies, improved productivity, and superior products or services, which can attract customers, build brand reputation, and differentiate the company from competitors.
Risk Mitigation: Maximizing profitability helps manufacturers build a financial cushion to withstand unforeseen challenges and economic downturns. Moreover, it provides a buffer against market fluctuations, raw material price hikes, or unexpected disruptions in the supply chain. Profitable companies are better equipped to weather uncertainties and mitigate risks that can impact their operations.
Employee Well-being and Job Security: Profitability directly impacts employees within the manufacturing sector. A profitable company is more likely to provide stable employment opportunities, competitive compensation packages, and opportunities for career advancement. It fosters a positive work environment and employee satisfaction, leading to higher retention rates and attracting top talent.
Stakeholder Returns: Profitability is essential for satisfying the expectations of stakeholders, including shareholders, investors, and lenders. Generating consistent profits allows manufacturers to provide attractive returns on investments, dividends, or interest payments. This fosters investor confidence and facilitates access to capital for future expansion or investment initiatives.
Industry Contribution: Profitable manufacturing companies contribute to the overall economic growth of a region or country. They generate tax revenues, create jobs, and stimulate economic activity through their supply chain and associated services. Profitable manufacturers play a vital role in driving industrial development and supporting the overall well-being of communities.
In conclusion, maximizing profitability in manufacturing is critical for financial stability, growth, competitiveness, risk mitigation, employee well-being, stakeholder satisfaction, and overall economic contribution of manufacturing companies. It enables them to thrive in a dynamic market, invest in innovation, and achieve sustainable success.
Understanding Data-Driven Demand Forecasting
Following, we've thoroughly discussed understanding data-driven demand forecasting. Let's learn:
A. Definition and Benefits of Data-Driven Demand Forecasting:
Data-driven demand forecasting is a process that involves using historical data, market trends, and other relevant information to predict and estimate future demand for products or services in the manufacturing industry. It relies on data analysis and statistical models to generate accurate forecasts that inform decision-making and planning.
The benefits of data-driven demand forecasting include:
Improved Forecast Accuracy: Data-driven approaches leverage historical data and advanced analytics techniques to generate more accurate demand forecasts. By considering various factors and patterns, manufacturers can make more informed decisions about production, inventory, and supply chain management, reducing the risk of stockouts or overstock.
Enhanced Inventory Management: Accurate demand forecasts enable manufacturers to optimize inventory levels. By aligning production and procurement with anticipated demand, companies can minimize excess inventory and associated costs while ensuring sufficient stock availability to meet customer demands.
Efficient Resource Allocation: Data-driven demand forecasting helps manufacturers allocate resources more efficiently. By understanding demand patterns, companies can optimize production schedules, allocate labor and equipment effectively, and streamline operations to maximize productivity and minimize costs.
Improved Customer Satisfaction: Accurate demand forecasting enables manufacturers to meet customer demands more effectively. By ensuring product availability and timely deliveries, companies can enhance customer satisfaction, build loyalty, and maintain positive relationships with clients.
Cost Reduction: Data-driven demand forecasting allows manufacturers to optimize their supply chain and production processes, reducing costs associated with excess inventory, stockouts, inefficient resource allocation, and expedited shipping. It also helps in avoiding overproduction, wastage, and obsolescence, leading to cost savings.
Strategic Decision-Making: Reliable demand forecasts enable manufacturers to make strategic decisions related to capacity planning, market expansion, product development, and pricing strategies. By understanding market trends and customer preferences, companies can align their strategies with future demand, positioning themselves for growth and profitability.
B. Types of Data Used in Demand Forecasting:
Demand forecasting relies on various types of data to generate accurate forecasts. These include:
Historical Sales Data: Historical sales data provides insights into past demand patterns and trends, allowing manufacturers to identify seasonality, trends, and cyclical variations. It forms the foundation for forecasting future demand based on past performance.
Market Research Data: Market research data encompasses information about customer preferences, purchasing behavior, market trends, and competitor analysis. This data helps manufacturers understand market dynamics and anticipate shifts in consumer demand, enabling more accurate forecasting.
Customer Data: Customer data, including demographics, purchasing history, and feedback, provides valuable insights into individual customer preferences, buying habits, and potential future demand. It helps manufacturers segment their customer base and tailor forecasting models to different customer segments.
External Data: External data sources such as economic indicators, weather patterns, social media sentiment, and industry reports can provide additional context for demand forecasting. These data sources offer insights into factors that influence demand and help manufacturers refine their forecasting models.
C. Key Considerations for Effective Demand Forecasting:
Effective demand forecasting requires careful consideration of various factors. Some key considerations include:
Data Quality and Reliability: The accuracy and reliability of the data used in demand forecasting are crucial. Manufacturers need to ensure that the data is clean, consistent, and free from errors or biases. Data cleansing and validation techniques should be employed to eliminate outliers, correct inconsistencies, and ensure high-quality input for forecasting models.
Granularity and Time Horizon: Manufacturers should determine the appropriate level of granularity and time horizon for their demand forecasts. Granularity refers to the level of detail at which demand is analyzed, such as product SKU level or customer segment level. Moreover, the time horizon refers to the forecasted period, whether short-term, medium-term, or long-term. Selecting the appropriate granularity and time horizon depends on the specific industry, product characteristics, and business objectives.
Seasonality and Demand Patterns: Seasonality and demand patterns play a significant role in demand forecasting, especially in industries with distinct seasonal variations. Understanding seasonal fluctuations, trends, and cyclicality helps manufacturers adjust their forecasting models and allocate resources accordingly. Statistical techniques such as time series analysis can capture and incorporate these patterns into forecasting models.
Collaborative Approach: Effective demand forecasting often involves collaboration between different departments within the organization, such as sales, marketing, operations, and finance. Furthermore, collaboration facilitates the sharing of domain knowledge, market insights, and cross-functional data, leading to more accurate and comprehensive demand forecasts.
Continuous Monitoring and Evaluation: Demand forecasting is an iterative process that requires continuous monitoring and evaluation. Manufacturers should regularly compare actual demand with forecasted demand and analyze any discrepancies. This feedback loop helps refine forecasting models, identify improvement opportunities, and adapt to changing market conditions.
Flexibility and Adaptability: Demand forecasting should be flexible and adaptable to accommodate unforeseen changes in the market, customer preferences, or external factors. Manufacturers should be prepared to adjust their forecasts and strategies in response to emerging trends, disruptive events, or shifts in demand patterns.
By considering these key considerations, manufacturers can enhance the accuracy and effectiveness of their demand forecasting processes, leading to improved decision-making, cost savings, and increased profitability.
Leveraging Data for Demand Forecasting in Manufacturing
Following, we've discussed leveraging data for demand forecasting in manufacturing. Let's learn:
A. Data Collection and Integration:
Data collection involves gathering relevant data from various sources to build a comprehensive dataset for demand forecasting. In manufacturing, some key data sources for demand forecasting include historical sales data, market research data, customer data, and external data such as economic indicators.
Manufacturers can collect data through internal systems, such as enterprise resource planning (ERP) systems, customer relationship management (CRM) systems, and point-of-sale (POS) systems. External data can be obtained from market research firms, government databases, industry reports, and social media platforms.
Data integration is the process of combining and consolidating data from different sources into a unified dataset for analysis. It may involve data extraction, transformation, and loading (ETL) processes to ensure compatibility and consistency.
Furthermore, integration can be achieved through data integration tools, data warehouses, or data lakes. The goal is to have a centralized, clean dataset that incorporates relevant data for accurate demand forecasting.
B. Data Preprocessing and Cleansing Techniques:
Before applying forecasting models, it is essential to preprocess and cleanse the data to ensure its quality and reliability. Data preprocessing involves several steps:
Data Cleaning: This step involves identifying and resolving issues such as missing values, outliers, and inconsistencies in the data. Missing values can be imputed using techniques such as mean imputation or regression imputation. Outliers can be handled by removing them or transforming them based on their impact on the forecasting model.
Data Transformation: Data transformation techniques, such as normalization or logarithmic transformations, can be applied to ensure data is on a comparable scale and follows a normal distribution. This can help improve the accuracy of forecasting models.
Feature Engineering: Feature engineering involves creating new features or variables from the existing data that may have a significant impact on demand forecasting. For example, variables like seasonality indicators, lagged variables, or promotional events can be derived to capture specific demand patterns.
Data Aggregation: Aggregating data at different levels, such as by product, customer segment, or geographical region, can provide a more granular understanding of demand patterns and facilitate forecasting at different levels of detail.
C. Choosing Appropriate Demand Forecasting Models:
Selecting the right demand forecasting model is crucial for accurate predictions. Several forecasting models can be employed, depending on the nature of the data and the characteristics of the demand patterns. Some commonly used models in manufacturing include:
Time Series Models: Time series models, such as ARIMA (AutoRegressive Integrated Moving Average) or exponential smoothing methods (e.g., Holt-Winters), are suitable for data with temporal dependencies and recurring patterns. These models capture seasonality, trends, and other time-related patterns in demand data.
Regression Models: Regression models can be utilized when demand is influenced by various factors such as price, promotions, or marketing campaigns. Multiple linear regression, logistic regression, or polynomial regression models can be used to identify the relationship between demand and explanatory variables.
Machine Learning Models: Machine learning algorithms, such as random forests, support vector regression (SVR), or neural networks, can handle complex and non-linear relationships in-demand data. These models can capture intricate demand patterns and consider multiple variables simultaneously.
Forecast Combination: Forecast combination techniques, such as ensemble methods or weighted averaging, can be employed to combine multiple individual forecasts from different models. This approach can enhance the accuracy and robustness of the demand forecast by leveraging the strengths of various models.
The choice of the appropriate model depends on factors such as data characteristics, available historical data, the presence of external factors, and the level of accuracy required. It is often beneficial to compare and evaluate different models using metrics such as mean absolute error (MAE), mean squared error (MSE), or forecast accuracy before finalizing the forecasting model.
By effectively collecting and integrating data, preprocessing and cleansing it, and choosing suitable forecasting models, manufacturers can leverage data-driven demand forecasting to make accurate predictions and drive better decision-making in production planning, inventory management, and overall supply chain optimization.
Advanced Analytics Techniques for Demand Forecasting
Following, we've discussed advanced analytics techniques for demand forecasting. Let's learn:
A. Machine Learning Algorithms for Demand Forecasting:
Machine learning algorithms offer powerful tools for demand forecasting in manufacturing. These algorithms can learn patterns and relationships from historical data to make predictions. Some commonly used machine learning algorithms for demand forecasting include:
Linear Regression: Linear regression models can be used to identify linear relationships between demand and relevant variables such as price, promotions, or economic indicators. It can capture the impact of these factors on demand and provide forecasts based on their influence.
Decision Trees: Decision tree algorithms, such as Random Forests or Gradient Boosting, can handle complex relationships and interactions among variables. They can capture non-linear patterns and handle categorical variables effectively. Decision trees are robust and versatile for demand forecasting
Support Vector Machines (SVM): SVM algorithms are useful for both regression and classification tasks in demand forecasting. They can handle non-linear relationships and capture complex patterns in the data. SVM models have high generalization capabilities and can handle large datasets.
Neural Networks: Neural networks, such as feedforward or recurrent neural networks (RNN), are well-suited for capturing intricate and non-linear relationships in demand data. They can handle time dependencies, seasonality, and complex demand patterns. Deep learning architectures like Long Short-Term Memory (LSTM) can be employed for improved forecasting accuracy.
Ensemble Methods: Ensemble methods, such as bagging or boosting techniques, combine multiple individual models to improve forecasting accuracy. By aggregating predictions from multiple models, ensemble methods can reduce bias and variance, leading to more robust and accurate demand forecasts.
B. Time Series Analysis Methods:
Time series analysis focuses on analyzing and forecasting data that exhibits temporal dependencies and patterns. In demand forecasting, time series analysis methods are valuable for capturing seasonality, trends, and other time-related patterns. Some commonly used time series analysis methods include:
Moving Averages: Moving average models calculate the average of past demand values over a specific period to estimate future demand. Different types of moving averages, such as simple moving average (SMA) or exponential moving average (EMA), can be employed based on the characteristics of the data.
Autoregressive Integrated Moving Average (ARIMA): ARIMA models are widely used for time series forecasting. They consider autoregressive (AR), moving average (MA), and differencing (I) components to capture trends, seasonality, and stationarity in the data. ARIMA models are suitable for data with stationary properties
Seasonal Decomposition of Time Series (STL): STL decomposition separates a time series into seasonal, trend, and residual components. This decomposition allows for better understanding and modeling of seasonality and trend patterns, which can be used for forecasting.
Exponential Smoothing Methods: Exponential smoothing methods, such as Simple Exponential Smoothing (SES), Holt's Linear Exponential Smoothing, or Holt-Winters, provide flexible techniques to capture and forecast different types of trends and seasonality. These methods assign more weight to recent data while considering previous observations.
C. Predictive Modeling and Simulation Techniques:
Predictive modeling and simulation techniques offer additional capabilities for demand forecasting in manufacturing. These techniques enable manufacturers to simulate different scenarios, evaluate the impact of changes, and make informed decisions. Some commonly used techniques include:
Monte Carlo Simulation: Monte Carlo simulation involves generating multiple random scenarios based on probability distributions to forecast demand. It accounts for uncertainty and provides a range of possible outcomes for decision-making.
Agent-Based Modeling: Agent-based modeling simulates individual agents (e.g., customers, suppliers) and their interactions to simulate demand patterns. It captures complex dynamics, behavioral patterns, and market effects that influence demand.
Discrete Event Simulation: Discrete event simulation models the flow of events and activities in the manufacturing process and supply chain. It enables manufacturers to analyze different scenarios, optimize operations, and forecast demand based on simulated outcomes.
Optimization Models: Optimization models utilize mathematical programming techniques to find the best allocation of resources, production schedules, and inventory levels based on demand forecasts. Additinally, these models consider constraints, objectives, and optimization criteria to maximize profitability and efficiency.
These advanced analytics techniques complement traditional forecasting approaches, offering manufacturers more accurate and sophisticated methods to forecast demand, optimize operations, and make data-driven decisions. The choice of technique depends on the specific characteristics of the data, the complexity of the demand patterns, and the desired level of accuracy and granularity in the forecasts.
Enhancing Profitability with Data-Driven Demand Forecasting
Following, we've discussed enhancing profitability with data-driven demand forecasting. Let's learn:
A. Optimizing Inventory Management Based on Demand Forecasts:
Data-driven demand forecasting provides valuable insights into future demand patterns, enabling manufacturers to optimize their inventory management strategies. By leveraging accurate demand forecasts, manufacturers can:
Reduce Excess Inventory: Excess inventory ties up capital, incurs storage costs, and increases the risk of obsolescence. By aligning inventory levels with anticipated demand, manufacturers can minimize excess inventory, reduce carrying costs, and improve cash flow.
Avoid Stockouts: Stockouts can lead to lost sales, dissatisfied customers, and potential damage to the brand's reputation. By accurately forecasting demand, manufacturers can ensure sufficient stock availability, optimize reorder points, and reduce the risk of stockouts.
Improve Order Fulfillment: Accurate demand forecasts enable manufacturers to align production schedules and procurement activities with expected demand. Moreover, this ensures timely order fulfillment, reduces lead times, and enhances customer satisfaction.
Optimize Safety Stock Levels: Safety stock is held to mitigate uncertainties and variability in demand. By considering demand forecasts, manufacturers can optimize safety stock levels, balancing the cost of carrying excess inventory against the risk of stockouts.
Implement Demand-Driven Replenishment: By integrating demand forecasts with supply chain systems, manufacturers can adopt demand-driven replenishment strategies. This ensures that inventory is replenished based on actual customer demand, reducing waste and improving efficiency.
B. Streamlining Production and Supply Chain Operations:
Data-driven demand forecasting facilitates streamlined production and supply chain operations by providing insights into anticipated demand and demand patterns. Manufacturers can leverage demand forecasts to:
Optimize Production Schedules: Accurate demand forecasts help manufacturers optimize production schedules to match expected demand. By aligning production capacity with anticipated demand, manufacturers can avoid underutilization or overutilization of resources, minimize production bottlenecks, and improve overall operational efficiency.
Efficient Resource Allocation: Demand forecasts assist in allocating resources effectively, including labor, equipment, and raw materials. By understanding demand fluctuations and seasonal patterns, manufacturers can allocate resources efficiently, minimize idle capacity, and reduce costs.
Supplier Collaboration: Demand forecasts enable manufacturers to collaborate with suppliers to optimize procurement and logistics activities. By sharing forecasts with suppliers, manufacturers can enhance supply chain visibility, reduce lead times, and optimize inventory levels throughout the supply chain.
Demand-Sensitive Capacity Planning: Data-driven demand forecasts inform capacity planning decisions. By accurately predicting demand, manufacturers can optimize their capacity requirements, avoid overinvestment in capacity during low-demand periods, and adjust production capabilities to meet varying demand levels.
C. Pricing Strategies and Revenue Optimization:
Data-driven demand forecasting plays a critical role in developing effective pricing strategies and revenue optimization. By leveraging demand forecasts, manufacturers can:
Dynamic Pricing: Demand forecasts enable manufacturers to implement dynamic pricing strategies based on anticipated demand fluctuations, market conditions, and customer behavior. By adjusting prices in real-time, manufacturers can optimize revenue and profitability.
Promotions and Discounts: Accurate demand forecasts help manufacturers plan and execute targeted promotions and discounts. By aligning promotional activities with anticipated demand, manufacturers can maximize sales, stimulate demand, and optimize revenue generation
Product Mix Optimization: Demand forecasts inform decisions about product mix and assortment. By understanding customer preferences and anticipated demand for different products, manufacturers can optimize their product portfolio, focus on high-demand items, and reduce the risk of carrying slow-moving inventory.
New Product Introductions: Demand forecasts support the successful introduction of new products by estimating their potential demand and market acceptance. By leveraging accurate demand forecasts, manufacturers can make informed decisions regarding new product development, launch strategies, and resource allocation.
Data-driven demand forecasting empowers manufacturers to make strategic decisions and implement initiatives that enhance profitability. By optimizing inventory management, streamlining operations, and implementing effective pricing strategies, manufacturers can maximize revenue, reduce costs, improve customer satisfaction, and drive overall profitability.
Overcoming Challenges in Data-Driven Demand Forecasting
Following, we've discussed how you can overcome challenges in data-driven demand forecasting. Let's learn:
A. Data Quality and Reliability Issues:
Data quality and reliability are crucial for accurate demand forecasting. Manufacturers often face challenges related to data quality, such as missing values, outliers, and inconsistencies. To overcome these challenges:
Data Cleaning and Validation: Implement rigorous data cleaning techniques to identify and resolve data quality issues. This involves handling missing values, outliers, and inconsistencies through imputation, data transformation, or removal, ensuring the data is reliable and consistent.
Data Integration and Consolidation: Integrate data from multiple sources to create a unified and comprehensive dataset. Validate and reconcile data from different systems to ensure consistency and accuracy. Implement data governance practices to maintain data quality throughout its lifecycle.
Quality Assurance Processes: Establish quality assurance processes to monitor data quality continuously. Implement automated checks and validations to identify data discrepancies and anomalies. Regularly audit and validate the data to maintain its accuracy and reliability.
B. Dealing with Demand Volatility and Seasonality:
Demand volatility and seasonality pose challenges in accurately forecasting demand. To address these challenges:
Historical Data Analysis: Analyze historical data to identify patterns and trends related to demand volatility and seasonality. Utilize statistical techniques such as time series analysis, moving averages, or decomposition methods to capture and model these patterns.
Incorporate External Factors: Consider external factors that influence demand volatility and seasonality, such as holidays, weather conditions, or economic indicators. Incorporate these factors into the forecasting models to improve accuracy.
Demand Segmentation: Segmenting demand based on customer characteristics, regions, or product categories can help capture specific demand patterns and improve forecasting accuracy. Different forecasting models or parameters can be applied to each segment to account for variations in demand volatility and seasonality.
Collaborative Forecasting: Collaborate with stakeholders, such as customers, suppliers, or distributors, to gather insights and information on demand patterns. Incorporate their knowledge and expertise into the forecasting process to enhance accuracy in capturing demand volatility and seasonality.
C. Addressing Limitations and Biases in Forecasting Models:
Forecasting models may have limitations and biases that can impact the accuracy of demand forecasts. To address these challenges:
Model Evaluation and Selection: Evaluate and compare different forecasting models based on their performance metrics. Consider the strengths and weaknesses of each model in relation to the specific characteristics of the data and demand patterns. Select models that best align with the data and minimize biases.
Model Calibration and Refinement: Continuously monitor and refine forecasting models based on actual demand outcomes. Incorporate feedback loops to identify and rectify any biases or limitations in the models. Regularly update and recalibrate the models to improve accuracy over time.
Ensemble Forecasting: Implement ensemble forecasting techniques that combine multiple models to mitigate biases and increase accuracy. Ensemble methods, such as weighted averaging or model averaging, can leverage the strengths of different models and reduce the impact of individual biases.
Continuous Improvement and Learning: Foster a culture of continuous improvement in demand forecasting. Encourage feedback, learning, and experimentation to identify and address limitations and biases in forecasting models. Regularly update models based on new data, insights, and emerging trends.
By addressing data quality issues, effectively handling demand volatility and seasonality, and mitigating limitations and biases in forecasting models, manufacturers can enhance the accuracy and reliability of their data-driven demand forecasts. This, in turn, enables them to make informed decisions and drive better business outcomes.
Case Studies: Successful Implementation of Data-Driven Demand Forecasting
Following, we've discussed case studies associated with the successful implementation of data-driven demand forecasting. Let's learn:
A. Manufacturing Companies Achieving Profitability Gains through Data-Driven Forecasting
Company X: Company X, a consumer goods manufacturer, implemented data-driven demand forecasting to optimize their inventory management and production planning. By leveraging historical sales data, market trends, and promotional activities, they achieved a significant reduction in stockouts and excess inventory. This led to improved cash flow, reduced carrying costs, and increased profitability.
Company Y: Company Y, an automotive parts manufacturer, utilized machine learning algorithms for demand forecasting. By analyzing historical sales data, economic indicators, and customer behavior, they were able to accurately predict demand fluctuations and adjust their production schedules accordingly. This resulted in optimized resource allocation, reduced idle capacity, and improved operational efficiency, leading to increased profitability.
Company Z: Company Z, a pharmaceutical manufacturer, implemented advanced analytics techniques for demand forecasting. By combining time series analysis, predictive modeling, and simulation techniques, they accurately forecasted demand for different product categories. This enabled them to optimize their production planning, reduce lead times, and improve order fulfillment rates. As a result, they experienced higher customer satisfaction, improved sales performance, and enhanced profitability.
B. Lessons Learned and Best Practices from Case Studies:
Data Quality and Integration: Case studies highlight the importance of ensuring data quality and integrating data from multiple sources. Investing in data cleansing, validation, and integration processes is critical for accurate demand forecasting.
Advanced Analytics Techniques: Leveraging advanced analytics techniques, such as machine learning algorithms and time series analysis, enhances the accuracy and effectiveness of demand forecasting. Companies should explore different techniques and select the ones that best align with their data and business requirements.
Collaborative Approach: Collaboration with stakeholders, including customers, suppliers, and distributors, can provide valuable insights for demand forecasting. Engaging in collaborative forecasting processes improves accuracy by incorporating diverse perspectives and domain expertise.
Continuous Improvement: Successful implementation of data-driven demand forecasting requires a culture of continuous improvement. Regularly evaluating and refining forecasting models, incorporating feedback, and staying updated with market dynamics contribute to ongoing success.
Integration with Operations: Integrating demand forecasting with inventory management, production planning, and supply chain operations is crucial for maximizing profitability. Furthermore, aligning production schedules, optimizing inventory levels, and streamlining operations based on demand forecasts leads to cost reduction and efficiency gains.
Scalability and Adaptability: Implementing scalable and adaptable forecasting systems enables manufacturers to handle growing data volumes and changing business dynamics. Investing in flexible technologies and processes that can accommodate future growth and evolving market conditions is essential.
Training and Skill Development: Providing training and skill development opportunities to employees involved in demand forecasting is vital. Equipping them with the necessary analytical and data interpretation skills enhances the effectiveness of data-driven forecasting initiatives
These case studies demonstrate the positive impact of data-driven demand forecasting on manufacturing companies' profitability. Implementing best practices, such as ensuring data quality, leveraging advanced analytics techniques, fostering collaboration, pursuing continuous improvement, integrating forecasting with operations, and investing in training, can significantly enhance the success of data-driven demand forecasting initiatives.
Future Trends in Data-Driven Demand Forecasting
Following, we've discussed future trends in data-driven demand forecasting. Let's learn:
A. Advancements in Predictive Analytics and Artificial Intelligence:
Predictive analytics and artificial intelligence (AI) are expected to play a significant role in the future of data-driven demand forecasting in manufacturing. Advancements in these areas will lead to:
Enhanced Accuracy: Predictive analytics techniques, such as machine learning algorithms and deep learning models, will continue to improve demand forecasting accuracy. These techniques can handle large and complex datasets, identify patterns and correlations, and generate more accurate predictions.
Real-time Forecasting: AI-powered demand forecasting models can process real-time data and generate forecasts on the fly. This enables manufacturers to make dynamic and timely decisions, especially in fast-paced and volatile markets.
Automated Decision-making: AI algorithms can automate decision-making processes based on demand forecasts. This includes automated inventory management, production planning, and supply chain optimization, leading to increased efficiency and profitability.
Prescriptive Analytics: Future advancements in predictive analytics will go beyond forecasting and provide prescriptive insights. These insights will not only predict demand but also recommend optimal strategies and actions to meet the forecasted demand, such as pricing strategies, production adjustments, and inventory optimization.
B. Integration of the Internet of Things (IoT) and Sensor Data for Demand Forecasting:
The integration of IoT devices and sensor data into demand forecasting processes holds great potential for the future. IoT devices can collect real-time data on various parameters, such as customer behavior, product usage, and environmental conditions. This integration will result in
Improved Data Accuracy: IoT devices provide real-time, high-frequency data, enabling manufacturers to capture demand signals more accurately. This data can include information on product usage patterns, customer preferences, and supply chain dynamics.
Granular and Personalized Forecasting: IoT data allows for more granular and personalized demand forecasting. By capturing individual customer behavior and preferences, manufacturers can tailor their forecasting models to specific customer segments, leading to improved accuracy and targeted decision-making.
Demand Sensing: IoT devices can enable demand sensing, which involves monitoring and capturing real-time demand signals from various sources, such as point-of-sale systems, social media, and connected devices. This enables manufacturers to respond quickly to changes in demand and adjust their operations accordingly.
Predictive Maintenance: IoT sensors can provide insights into the condition and performance of manufacturing equipment. By integrating this data into demand forecasting, manufacturers can anticipate potential equipment failures, optimize maintenance schedules, and ensure uninterrupted production.
C. Emerging Technologies Shaping the Future of Demand Forecasting in Manufacturing:
Several emerging technologies have the potential to reshape demand forecasting in manufacturing:
Big Data Analytics: As data volumes continue to grow, big data analytics techniques will become increasingly important in demand forecasting. Advanced analytics tools and algorithms will be utilized to extract insights from large and diverse datasets, enabling manufacturers to make more accurate predictions.
Blockchain Technology: Blockchain technology can enhance transparency and traceability in supply chains. By recording and securely sharing demand data across the supply chain, manufacturers can gain a more comprehensive view of demand patterns, improve collaboration, and reduce information asymmetry.
Predictive Analytics Platforms: The development of integrated predictive analytics platforms specifically designed for demand forecasting will simplify the process for manufacturers. Furthermore, these platforms will provide user-friendly interfaces, pre-built models, and automated workflows, enabling manufacturers to leverage advanced analytics techniques without requiring extensive data science expertise.
Cloud Computing: Cloud computing offers scalable computing power and storage, making it easier for manufacturers to process and analyze large datasets for demand forecasting. Cloud-based solutions also facilitate collaboration, data sharing, and real-time access to demand forecasting models and insights.
Augmented Reality (AR) and Virtual Reality (VR): AR and VR technologies have the potential to enhance demand forecasting by providing immersive and interactive visualization of demand data. These technologies can facilitate scenario planning, decision-making, and collaboration among stakeholders, leading to more informed and effective forecasting strategies.
In conclusion, future trends in data-driven demand forecasting in manufacturing involve advancements in predictive analytics and AI, the integration of IoT and sensor data, and the adoption of emerging technologies such as big data analytics, blockchain, predictive analytics platforms, cloud computing, and AR/VR. These trends will empower manufacturers with more accurate, real-time, and actionable demand forecasts, enabling them to optimize their operations, enhance profitability, and stay competitive in a rapidly evolving marketplace.
Wrapping Up
In conclusion, data-driven demand forecasting is a powerful tool that can greatly enhance profitability in the manufacturing industry. By leveraging data and advanced analytics techniques, manufacturers can make more accurate predictions about customer demand, leading to optimized inventory management, streamlined operations, and effective pricing strategies. We have explored various aspects of data-driven demand forecasting, including its definition, benefits, data collection, preprocessing techniques, choice of forecasting models, and advanced analytics methods.
Furthermore, the article has highlighted the importance of maximizing profitability in manufacturing and how data-driven demand forecasting plays a crucial role in achieving this goal. It has also addressed the challenges that manufacturers may face in implementing data-driven demand forecasting and provided strategies for overcoming these challenges.
Additionally, the article discussed case studies that exemplify the successful implementation of data-driven demand forecasting, showcasing how companies have achieved profitability gains by leveraging accurate demand forecasts. It also emphasized the lessons learned and best practices derived from these case studies, offering valuable insights for manufacturers.
Finally, the article touched upon the future trends in data-driven demand forecasting, including advancements in predictive analytics and AI, integration of IoT and sensor data, and the emergence of technologies like big data analytics, blockchain, cloud computing, and AR/VR. These trends are expected to shape the future of demand forecasting in manufacturing and further enhance profitability by providing more accurate, real-time, and actionable insights.
In summary, embracing data-driven demand forecasting as a core strategy can enable manufacturing companies to optimize their operations, improve customer satisfaction, and maximize profitability. By leveraging data, advanced analytics techniques, and emerging technologies, manufacturers can gain a competitive edge in the market and navigate the ever-changing demands of customers and the industry.
How Deskera Can Assist You?
Deskera ERP and MRP systems help you to keep your business units organized. The system's key features of demand forecasting with Deskera include as follows:
- Establish Demand Forecasting Process
- Automate Data Collection
- Monitor Market Trends
- Analyze Historical Data
- Estimate Future Demand
- Adjust Production Levels
- Manage Supply Chain...and much more!
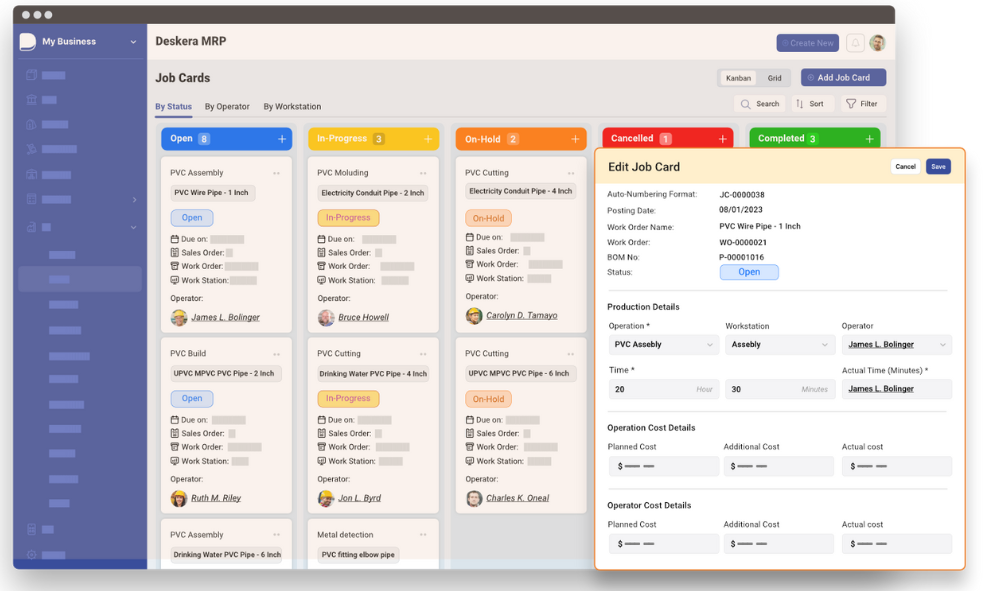
- Deskera's integrated financial planning tools enable investors to better plan and track their investments. It can assist investors in making faster and more accurate decisions.
- Deskera Books allows you to better manage your accounts and finances. Maintain good accounting practices by automating tasks like billing, invoicing, and payment processing.
- Deskera CRM is a powerful solution that manages your sales and helps you close deals quickly. It not only enables you to perform critical tasks like lead generation via email, but it also gives you a comprehensive view of your sales funnel.
- Deskera People is a straightforward tool for centralizing your human resource management functions.
Final Takeaways
We've arrived at the last section of this guide. Let's have a look at some of the most important points to remember:
- Data-driven demand forecasting in manufacturing refers to the process of using data and analytics to predict and estimate future demand for products or services in the manufacturing industry.
- Profitability is crucial for the financial stability of manufacturing companies. Profitable operations ensure that a company has sufficient resources to cover expenses, invest in research and development, upgrade equipment, and fund future growth. It provides a solid foundation for long-term sustainability.
- Accurate demand forecasts enable manufacturers to optimize inventory levels. By aligning production and procurement with anticipated demand, companies can minimize excess inventory and associated costs while ensuring sufficient stock availability to meet customer demands.
- Customer data, including demographics, purchasing history, and feedback, provides valuable insights into individual customer preferences, buying habits, and potential future demand. It helps manufacturers segment their customer base and tailor forecasting models to different customer segments.
- Understanding seasonal fluctuations, trends, and cyclicality helps manufacturers adjust their forecasting models and allocate resources accordingly. Statistical techniques such as time series analysis can capture and incorporate these patterns into forecasting models.
- Regression models can be utilized when demand is influenced by various factors such as price, promotions, or marketing campaigns. Multiple linear regression, logistic regression, or polynomial regression models can be used to identify the relationship between demand and explanatory variables.
- Accurate demand forecasts help manufacturers optimize production schedules to match expected demand. By aligning production capacity with anticipated demand, manufacturers can avoid underutilization or overutilization of resources, minimize production bottlenecks, and improve overall operational efficiency.
Related Articles
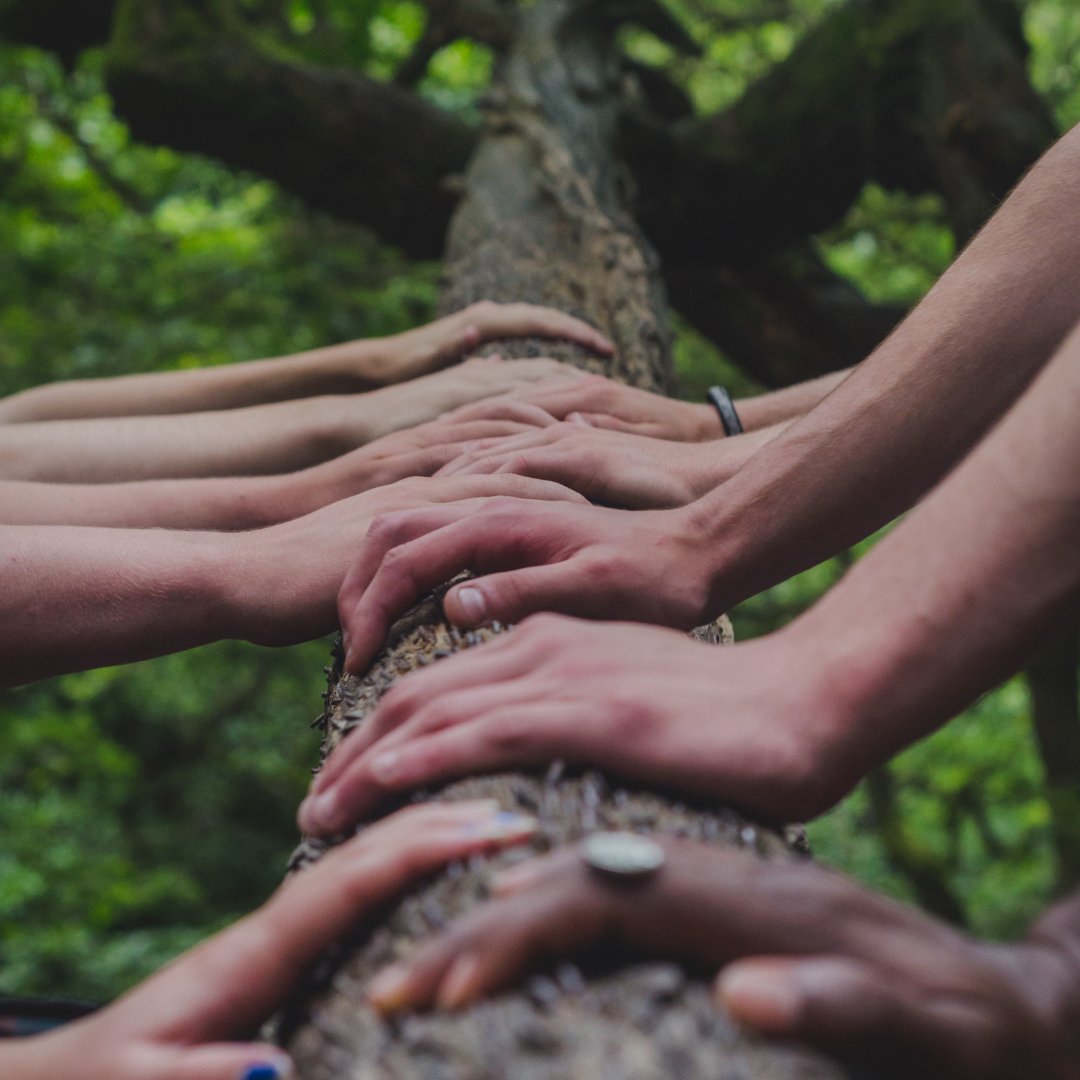
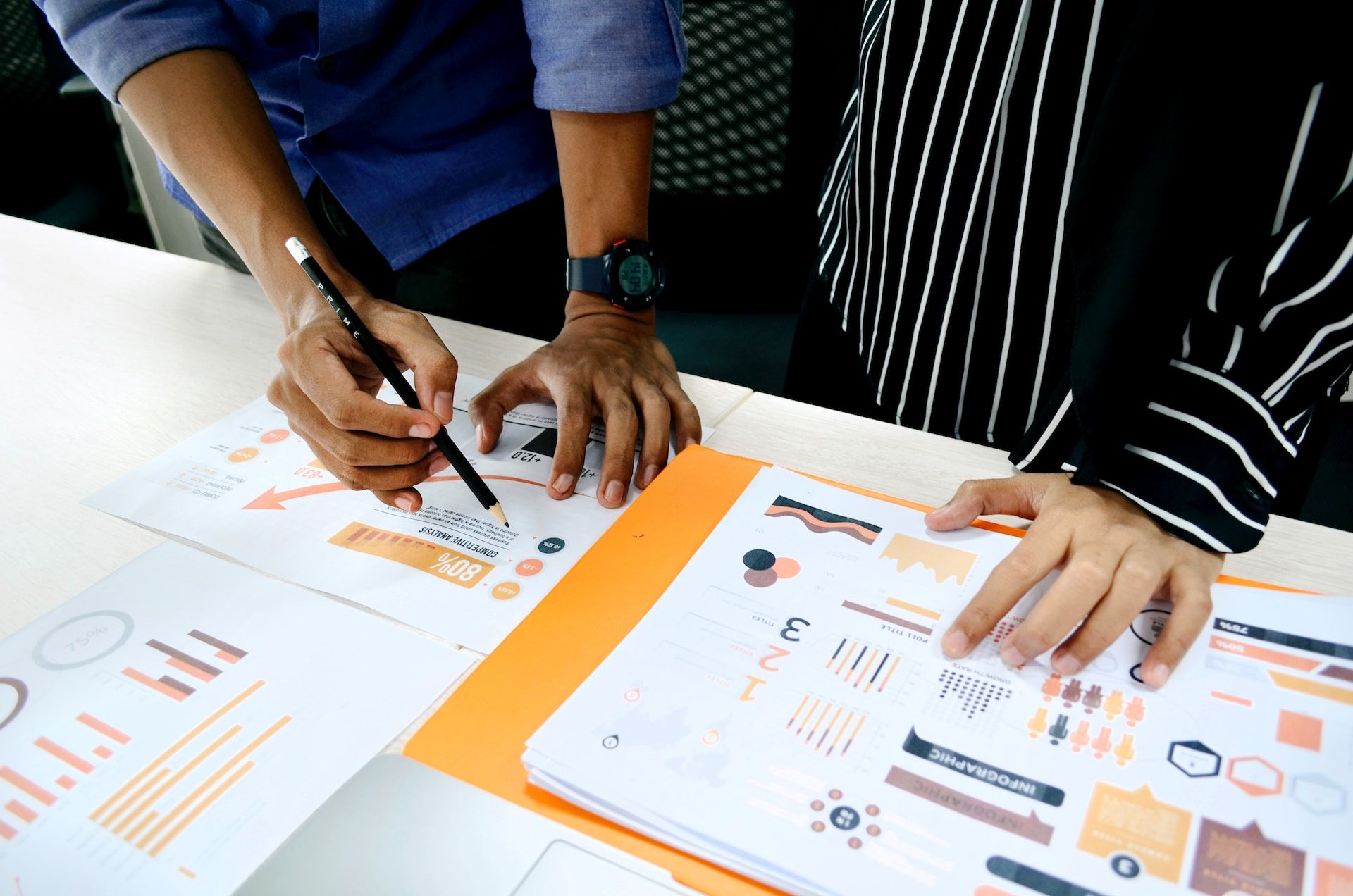
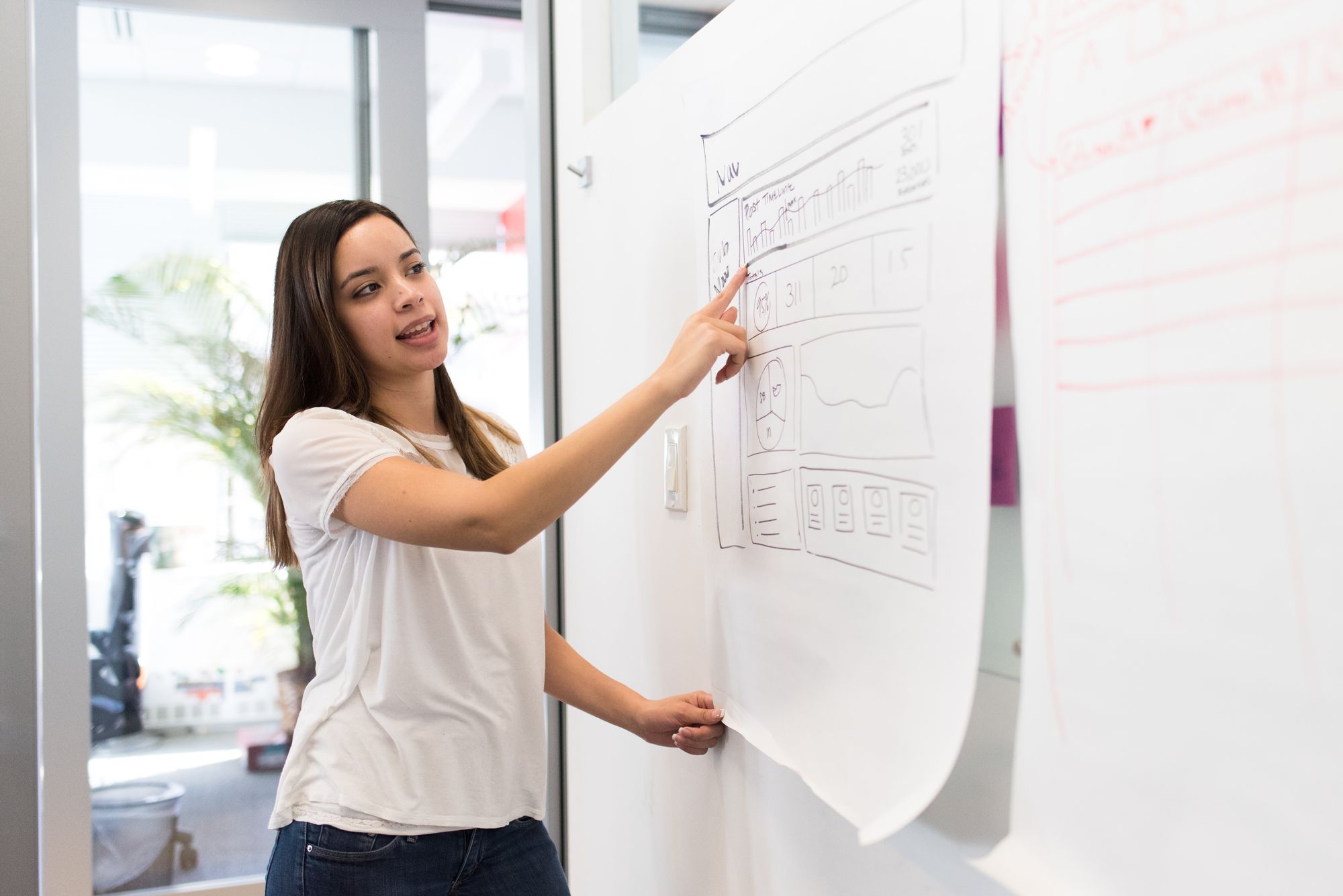
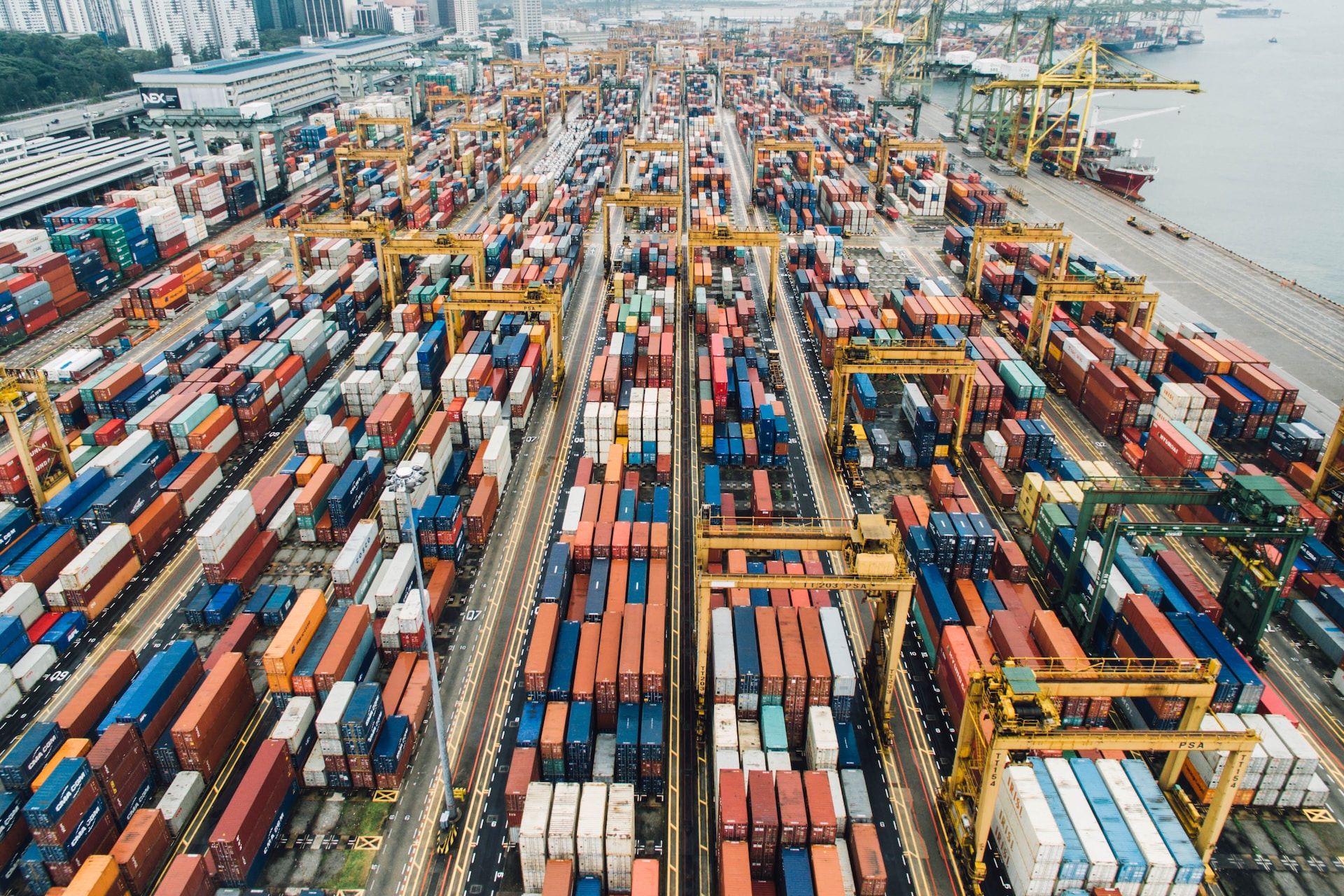
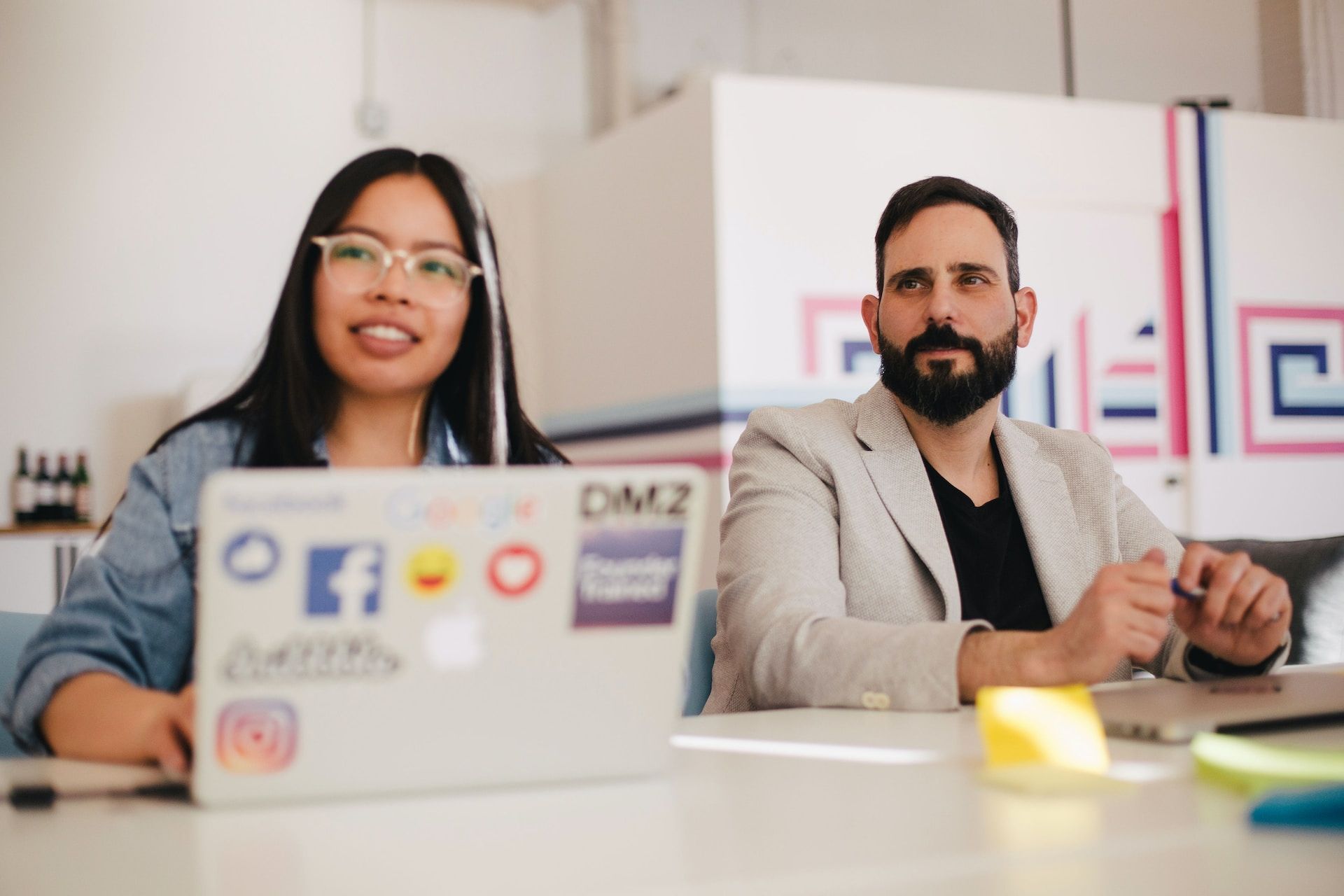