In today's fiercely competitive manufacturing landscape, the pursuit of operational efficiency has become a top priority for organizations. One key area that plays a pivotal role in achieving this goal is production planning. Efficient production planning ensures optimal resource allocation, reduces costs, and enhances customer loyalty and satisfaction.
However, traditional production planning methods often fall short in addressing the dynamic and complex nature of modern manufacturing processes.
According to a study conducted by McKinsey & Company, companies that implement data-driven production planning experience an average 15-20% increase in operational efficiency, leading to significant cost savings and improved productivity.
This is where data-driven production planning emerges as a game-changer. By leveraging real-time and historical data, manufacturers can gain valuable insights into their production operations, enabling them to make informed decisions, improve forecasting accuracy, and optimize resource allocation.
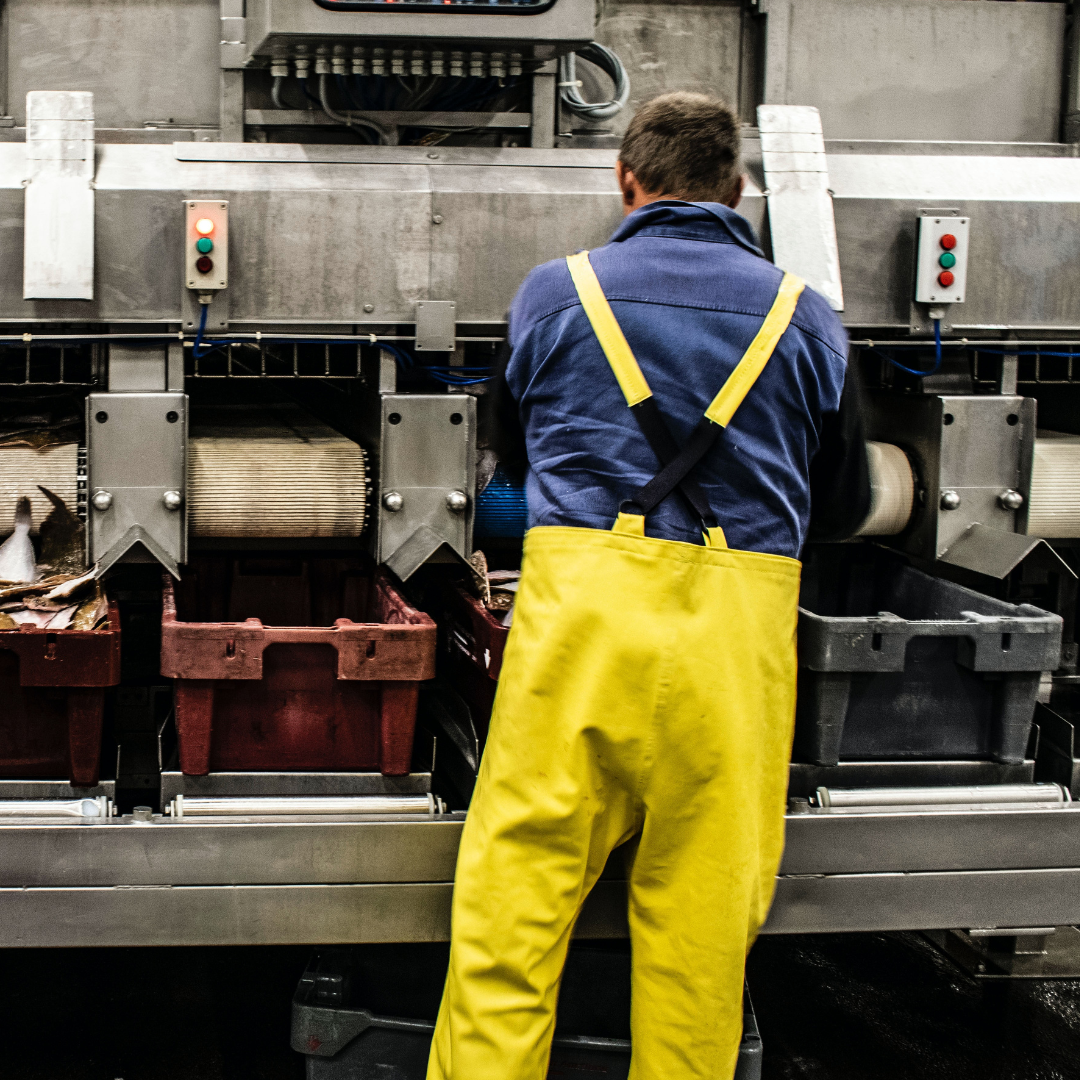
From the integration of Internet of Things (IoT) devices to the application of advanced analytics and machine learning algorithms, data-driven production planning has the potential to revolutionize manufacturing.
In this article, we will explore the benefits, challenges, and key components of data-driven production planning, shedding light on how manufacturers can maximize efficiency and stay ahead in the industry.
- Importance of Production Planning in Manufacturing
- Challenges Faced By Manufacturers In Optimizing Production Processes
- Concept Of Data-Driven Production Planning As A Solution
- Section 1: The Role of Production Planning in Manufacturing
- Section 2: Challenges in Traditional Production Planning
- Section 3: Introduction to Data-driven Production Planning
- Section 4: Key Components of Data-driven Production Planning
- Section 5: Implementation and Integration Challenges
- Section 6: Case Studies and Success Stories
- Summarizing the Advantages of Adopting a Data-driven Approach to Production Planning
- Embracing Data-Driven Production Planning for Competitive Advantage in the Evolving Manufacturing Landscape
- How can Deskera Help You?
- Key Takeaways
- Related Articles
Importance of Production Planning in Manufacturing
Production planning plays a crucial role in the manufacturing industry for several reasons. First and foremost, it ensures operational efficiency by effectively managing resources, minimizing waste, and optimizing production processes.
By aligning production activities with customer demand, production planning helps avoid stockouts or excessive inventory, resulting in cost savings and improved customer satisfaction.
Furthermore, production planning enables manufacturers to make informed decisions regarding capacity utilization, workforce allocation, and equipment utilization. It helps in identifying potential bottlenecks or inefficiencies in the production process, allowing for timely adjustments and improvements.
Moreover, effective production planning contributes to better forecasting accuracy, enabling manufacturers to anticipate market demand, plan production schedules, and optimize inventory levels. This helps in reducing lead times, avoiding production delays, and enhancing overall supply chain management.
Ultimately, production planning in manufacturing ensures smooth operations, maximizes resource utilization, minimizes costs, and enhances customer satisfaction. It is a vital component for achieving operational excellence and maintaining a competitive edge in the dynamic manufacturing landscape.
7 Challenges Faced by Manufacturers in Optimizing Production Processes
Manufacturers face various challenges when it comes to optimizing their production processes. These challenges can hinder efficiency, increase costs, and affect overall productivity. Some of the common challenges include:
- Demand Variability: Fluctuations in customer demand make it difficult for manufacturers to accurately forecast and plan production. Unpredictable shifts in demand can lead to underutilized capacity or production bottlenecks, resulting in inefficient resource allocation.
- Lack of Real-time Visibility: Limited visibility into real-time production data can hinder decision-making. Without access to accurate and timely information on machine performance, inventory levels, or production status, manufacturers may struggle to identify and address issues promptly.
- Manual Processes and Data Entry: Reliance on manual data entry and paper-based systems can introduce errors, delays, and inefficiencies into the production planning process. Manually updating spreadsheets or tracking production metrics can be time-consuming and prone to human errors.
- Siloed Data and Systems: Many manufacturers operate with fragmented systems and data sources that are not integrated. Siloed data across different departments or systems makes it challenging to have a holistic view of the production process, hindering effective decision-making.
- Complex Supply Chain Dynamics: Manufacturers often face complexities in managing their supply chain, including supplier reliability, transportation logistics, and inventory management. These challenges can impact production planning by introducing delays, disruptions, or inefficiencies.
- Limited Resource Optimization: Optimizing resource allocation, including labor, equipment, and raw materials, is crucial for efficient production planning. However, manufacturers may face constraints such as limited availability of skilled workers, equipment breakdowns, or supply chain disruptions that can impact resource optimization.
- Cost Pressures: Cost reduction is a constant concern for manufacturers. Balancing cost-saving measures with maintaining quality and meeting customer expectations can be challenging. Inefficient production processes can lead to increased costs, affecting the overall profitability of the business.
Addressing these challenges requires implementing strategies such as data-driven production planning, leveraging advanced technologies, streamlining processes, and fostering collaboration across departments. Overcoming these obstacles is essential for manufacturers to maximize efficiency, reduce costs, and maintain a competitive edge in the market.
Concept of Data-Driven Production Planning as a Solution
The concept of data-driven production planning revolves around utilizing real-time and historical data to optimize and improve the efficiency of production processes. It involves collecting, analyzing, and leveraging data to make informed decisions and drive improvements across the manufacturing operations. By harnessing the power of data, manufacturers can achieve greater accuracy, agility, and responsiveness in their production planning strategies.
Data-driven production planning offers several benefits:
- Enhanced Forecasting Accuracy: By analyzing historical data and real-time market trends, manufacturers can improve their demand forecasting capabilities. Accurate demand forecasts enable better production scheduling, inventory management, and resource allocation, minimizing stockouts and overstocking.
- Optimized Resource Allocation: Data-driven insights help manufacturers identify inefficiencies and optimize resource allocation. By monitoring machine performance, labor productivity, and equipment utilization, manufacturers can make data-backed decisions to maximize productivity and minimize downtime.
- Improved Decision-Making: Access to real-time production data enables faster and more informed decision-making. Manufacturers can identify production bottlenecks, address quality issues promptly, and adjust production plans dynamically based on changing market conditions or customer demand.
- Continuous Process Improvement: Data-driven production planning supports a culture of continuous improvement. By analyzing production data, manufacturers can identify areas for improvement, implement corrective actions, and measure the impact of process optimization initiatives over time.
- Enhanced Supply Chain Integration: Data-driven production planning facilitates integration with the broader supply chain ecosystem. Manufacturers can share data with suppliers, logistics partners, and customers, fostering collaboration, improving visibility, and optimizing supply chain coordination.
To implement data-driven production planning, manufacturers leverage technologies such as the Internet of Things (IoT), big data analytics, machine learning, and cloud computing. IoT devices and sensors collect real-time data from machines, equipment, and production lines.
Advanced analytics tools process this data to generate actionable insights, enabling predictive maintenance, quality control, and production optimization. Machine learning algorithms help in demand forecasting, anomaly detection, and predictive analytics, further enhancing production planning capabilities.
Overall, data-driven production planning empowers manufacturers to make data-backed decisions, optimize resource allocation, reduce costs, improve customer satisfaction, and stay competitive in today's rapidly evolving manufacturing landscape.
Section 1: The Role of Production Planning in Manufacturing
Production planning plays a crucial role in the manufacturing industry, serving as the backbone for efficient operations and optimized resource utilization. By strategically aligning production activities with customer demand, production planning ensures that manufacturing processes run smoothly, costs are minimized, and customer satisfaction is maximized.
It involves forecasting demand, determining production schedules, allocating resources effectively, and monitoring the progress of production activities.
A well-executed production planning process enables manufacturers to avoid stockouts, reduce lead times, optimize inventory levels, and make informed decisions regarding capacity utilization, labor allocation, and equipment utilization. Ultimately, production planning acts as a guiding force that enables manufacturers to meet customer demands, maintain competitive advantage, and achieve operational excellence.
In this section, we will delve into the significance of production planning in manufacturing, exploring its objectives, potential consequences of poor planning, and the crucial role it plays in optimizing overall efficiency.
What is production planning and its significance in achieving operational efficiency?
Production planning refers to the process of determining the most effective and efficient way to produce goods or services while meeting customer demand. It involves analyzing demand patterns, forecasting future demand, and creating a comprehensive plan that outlines the necessary resources, timelines, and activities required for successful production.
The significance of production planning in achieving operational efficiency cannot be overstated. Here are some key points:
- Resource Optimization: Production planning ensures that resources such as labor, raw materials, equipment, and facilities are allocated optimally. By carefully planning and coordinating these resources, manufacturers can minimize waste, reduce idle time, and maximize productivity.
- Meeting Customer Demand: Effective production planning aligns production activities with customer demand. By accurately forecasting demand and planning production accordingly, manufacturers can avoid stockouts or excessive inventory, thereby meeting customer requirements in a timely manner.
- Cost Reduction: Efficient production planning helps identify cost-saving opportunities. By optimizing production schedules, streamlining processes, and minimizing idle time, manufacturers can reduce costs associated with overproduction, inventory holding, and excessive setup or changeover times.
- Improved Quality Control: Production planning enables manufacturers to incorporate quality control measures into the production process. By setting standards and checkpoints, manufacturers can ensure that products meet quality requirements at every stage, reducing defects and rework.
- Timely Decision-Making: With a well-defined production plan, manufacturers can make informed decisions in a timely manner. Production planning provides visibility into upcoming demand, resource availability, and potential bottlenecks, allowing for proactive decision-making and effective problem-solving.
- Supply Chain Integration: Production planning plays a crucial role in integrating the supply chain. By sharing production plans and forecasts with suppliers, manufacturers can coordinate procurement activities, reduce lead times, and ensure the timely availability of raw materials or components.
- Continuous Improvement: Production planning facilitates a culture of continuous improvement. By monitoring and analyzing production data, manufacturers can identify areas for optimization, implement process enhancements, and measure the impact of improvement initiatives over time.
Overall, production planning is a vital component for achieving operational efficiency in manufacturing. It enables manufacturers to optimize resources, meet customer demand, reduce costs, maintain quality standards, and make data-driven decisions that drive continuous improvement throughout the production process.
Objectives of production planning, such as meeting customer demand, reducing costs, and improving resource allocation
The objectives of production planning in manufacturing encompass various aspects that contribute to overall operational efficiency and business success. Here are some key objectives:
- Meeting Customer Demand: One of the primary objectives of production planning is to meet customer demand effectively and efficiently. By accurately forecasting demand and planning production activities accordingly, manufacturers can ensure that products are available when and where customers need them. Meeting customer demand leads to higher customer satisfaction, improved brand reputation, and increased sales.
- Reducing Costs: Production planning aims to identify cost-saving opportunities and minimize expenses throughout the production process. By optimizing production schedules, streamlining processes, and implementing lean principles, manufacturers can reduce costs associated with overproduction, excessive inventory holding, and unnecessary downtime. Efficient production planning helps in maximizing resource utilization, minimizing waste, and achieving cost efficiency.
- Improving Resource Allocation: Effective production planning enables optimal resource allocation, including labor, equipment, and materials. By analyzing production requirements and availability of resources, manufacturers can allocate resources effectively, ensuring that they are utilized efficiently and minimizing idle time. This leads to increased productivity, reduced lead times, and improved operational efficiency.
- Enhancing Production Efficiency: Production planning aims to improve overall production efficiency by eliminating bottlenecks, reducing setup and changeover times, and optimizing production schedules. By identifying and addressing process inefficiencies, manufacturers can enhance throughput, minimize production delays, and increase overall productivity.
- Maintaining Quality Standards: Another objective of production planning is to maintain and improve product quality. By incorporating quality control measures and checkpoints into the production process, manufacturers can ensure that products meet the required standards. Effective production planning includes quality assurance activities that help in reducing defects, minimizing rework, and delivering high-quality products to customers.
- Managing Inventory: Efficient production planning helps in optimizing inventory levels, reducing excess inventory, and avoiding stockouts. By aligning production with demand and implementing inventory management strategies, manufacturers can minimize carrying costs, improve cash flow, and enhance supply chain performance.
- Enhancing Flexibility and Responsiveness: Production planning aims to enhance flexibility and responsiveness to changing market conditions and customer demands. By having a well-designed production plan in place, manufacturers can quickly adapt to changes, such as order variations, new product introductions, or changes in market demand. This agility enables manufacturers to seize opportunities, respond to customer needs promptly, and stay competitive.
By aligning production planning with these objectives, manufacturers can achieve operational excellence, improve customer satisfaction, reduce costs, and drive overall business success in the dynamic manufacturing landscape.
Potential consequences of poor production planning
Poor production planning in manufacturing can have significant consequences that negatively impact operations, profitability, and customer satisfaction. Here are some potential consequences of inadequate or ineffective production planning:
- Stockouts: Insufficient or inaccurate production planning can lead to stockouts, where products are not available to meet customer demand. Stockouts can result in lost sales, dissatisfied customers, and damage to the company's reputation. It may also lead to missed opportunities to capitalize on market demand and potential revenue loss.
- Production Bottlenecks: Inadequate production planning can result in bottlenecks, where certain stages or resources in the production process cannot keep up with the required output. Bottlenecks can cause delays, increased lead times, and reduced overall productivity. This can lead to inefficiencies, increased costs, and difficulties in meeting customer demand within the desired timeframe.
- Excessive Inventory: Poor production planning can lead to excessive inventory levels, where production exceeds customer demand. Excessive inventory ties up working capital, increases storage costs, and can lead to obsolescence or spoilage. It also indicates a mismatch between production and demand, resulting in potential financial losses for the company.
- Increased Costs: Inefficient production planning can result in increased costs throughout the production process. Overproduction, excessive inventory holding, or poor resource allocation can lead to higher carrying costs, increased waste, and additional expenses associated with storage, handling, and obsolescence. Poor planning may also result in rush orders, expedited shipping, or premium labor costs to compensate for inadequate production schedules.
- Inaccurate Demand Forecasting: Lack of accurate demand forecasting as part of production planning can lead to significant consequences. If demand is overestimated, manufacturers may produce more than necessary, leading to excessive inventory and associated costs. Conversely, underestimating demand may result in missed sales opportunities, stockouts, and dissatisfied customers.
- Poor Customer Satisfaction: Inefficient production planning can impact customer satisfaction adversely. Delays in product availability, stockouts, or inconsistent lead times can lead to unhappy customers and damage the company's reputation. Unsatisfied customers may turn to competitors, resulting in potential loss of market share and revenue.
- Operational Inefficiencies: Inadequate production planning can contribute to operational inefficiencies across the entire manufacturing process. This includes suboptimal resource allocation, inefficient scheduling, frequent changeovers, excessive downtime, and production disruptions. These inefficiencies lead to reduced productivity, increased costs, and compromised overall operational performance.
To mitigate these consequences, manufacturers should focus on improving production planning processes, enhancing demand forecasting accuracy, optimizing resource allocation, implementing lean principles, and leveraging technology and data-driven insights.
Section 2: Challenges in Traditional Production Planning
In the realm of manufacturing, traditional production planning methods often face a range of challenges that hinder operational efficiency and agility. These challenges stem from outdated processes, limitations in data accessibility, and the inability to respond effectively to evolving market demands.
In this section, we will delve into the various obstacles encountered in traditional production planning. By understanding these challenges, manufacturers can explore innovative solutions and strategies to overcome them, ultimately paving the way for improved production planning processes that align with the demands of today's dynamic manufacturing landscape.
Common limitations of traditional production planning methods
Traditional production planning methods often suffer from several limitations that can impede operational efficiency and hinder responsiveness to market dynamics. Here are some common limitations:
- Manual Data Entry: Traditional production planning often relies on manual data entry, which is time-consuming and prone to errors. This manual process increases the risk of inaccurate data, leading to flawed production plans and inefficient resource allocation.
- Reliance on Historical Data: Traditional methods heavily rely on historical data for forecasting and planning purposes. While historical data provides insights into past trends, it may not adequately capture the evolving market conditions or sudden changes in customer demand. Relying solely on historical data can result in inaccurate demand forecasts and suboptimal production plans.
- Limited Visibility into Real-time Production Processes: Traditional production planning methods often lack real-time visibility into production processes. This limitation hinders the ability to promptly identify production bottlenecks, equipment breakdowns, or quality issues. Without timely access to accurate production data, manufacturers may struggle to make informed decisions and address issues proactively.
- Lack of Integration and Collaboration: Traditional production planning methods may operate in silos, with limited integration and collaboration among departments. This lack of coordination between planning, production, and other functions can lead to communication gaps, inefficient workflows, and delays in decision-making.
- Inflexibility and Long Lead Times: Traditional production planning may be characterized by rigid schedules and long lead times. These methods may not easily accommodate changes in customer demand, new product introductions, or unexpected market shifts. The lack of flexibility can result in missed opportunities, increased costs, and delays in meeting customer requirements.
- Inadequate Resource Optimization: Traditional production planning methods may struggle to optimize resource allocation effectively. Limited visibility into resource availability and utilization can lead to underutilized or overburdened resources, leading to inefficiencies and increased costs.
- Inefficient Capacity Planning: Traditional approaches to capacity planning may rely on fixed capacity assumptions or historical averages, which may not accurately reflect actual capacity requirements. This can result in underutilization of available capacity or inability to meet increased demand during peak periods.
Overcoming these limitations requires the adoption of modern production planning techniques, such as data-driven approaches, advanced analytics, and technology integration.
By leveraging real-time data, predictive modeling, and collaborative platforms, manufacturers can enhance production planning processes, improve decision-making, and achieve greater operational efficiency in the face of dynamic market conditions.
Negative impact of these challenges on productivity, quality, and customer satisfaction
The challenges associated with traditional production planning methods can have a significant negative impact on productivity, quality, and customer satisfaction within the manufacturing process. Here are some key consequences:
- Reduced Productivity: Manual data entry and inefficient resource allocation processes can lead to reduced productivity. Errors and delays in data entry not only consume valuable time but can also result in inaccurate production plans and schedules. Inadequate resource allocation can lead to idle time, excessive setup or changeover times, and suboptimal utilization of labor and equipment. These factors collectively lower overall productivity, impacting throughput and operational efficiency.
- Compromised Quality: Reliance on historical data and limited visibility into real-time production processes can compromise product quality. Inaccurate demand forecasts may result in underproduction or overproduction, leading to stockouts or excess inventory. Limited visibility into production processes hampers the ability to promptly identify and address quality issues or deviations from desired specifications. As a result, product quality may suffer, leading to customer dissatisfaction, increased returns, and potential damage to the brand's reputation.
- Diminished Customer Satisfaction: Inefficient production planning can have a direct impact on customer satisfaction. Inaccurate demand forecasts can lead to delays in fulfilling customer orders, resulting in missed delivery deadlines. Stockouts or excessive lead times can frustrate customers, leading to dissatisfaction and potential loss of business. Furthermore, poor quality products resulting from inadequate planning can lead to customer complaints, returns, and a negative perception of the company's offerings.
- Increased Costs: Traditional production planning challenges can result in increased costs throughout the manufacturing process. Inefficient resource allocation and excess inventory levels contribute to higher carrying costs, increased waste, and additional expenses associated with storage, handling, and obsolescence. Delays, rework, and quality issues stemming from inadequate planning can also lead to increased costs due to production disruptions and the need for corrective actions.
- Missed Opportunities: Inflexibility and long lead times associated with traditional production planning methods can result in missed opportunities. Inability to respond quickly to changing market demands, introduce new products, or adapt to customer preferences can lead to lost sales and decreased market share. The lack of agility in planning and execution prevents manufacturers from capitalizing on emerging trends or capturing time-sensitive business opportunities.
- Operational Inefficiencies: Challenges in traditional production planning can contribute to overall operational inefficiencies. Siloed processes, communication gaps, and delayed decision-making hinder the coordination and synchronization of production activities. Inefficient workflows, inadequate capacity planning, and suboptimal resource utilization lead to reduced efficiency, increased downtime, and higher costs.
Addressing these challenges through modern production planning techniques, such as data-driven approaches, advanced analytics, and technology integration, can help mitigate the negative impact on productivity, quality, and customer satisfaction.
Need for a more advanced and data-driven approach to production planning
The rapidly evolving manufacturing landscape calls for a more advanced and data-driven approach to production planning. Traditional methods, relying on manual processes and historical data, are no longer sufficient to meet the complexities and demands of modern manufacturing. Here are several reasons highlighting the need for a more advanced and data-driven approach:
- Increasing Complexity: Manufacturing processes have become increasingly complex, with multiple variables and interdependencies to consider. A data-driven approach can analyze large volumes of data, identify patterns, and uncover insights that manual methods may overlook. This enables manufacturers to make more accurate forecasts, optimize production schedules, and manage resources effectively in a complex operational environment.
- Dynamic Market Demands: Customer preferences and market demands are constantly changing. A data-driven approach allows manufacturers to capture and analyze real-time market data, customer feedback, and industry trends. By leveraging this information, manufacturers can quickly respond to shifts in demand, adapt production plans accordingly, and introduce new products or variations to meet customer expectations.
- Improved Forecasting Accuracy: Data-driven production planning incorporates advanced forecasting models that consider multiple variables and use historical data along with real-time information. These models provide more accurate demand forecasts, enabling manufacturers to optimize inventory levels, reduce stockouts, and minimize excess inventory. Improved forecasting accuracy also helps align production capacities, labor requirements, and supply chain activities more effectively.
- Optimal Resource Allocation: Data-driven production planning facilitates optimal resource allocation by leveraging historical data, real-time production data, and advanced algorithms. This approach helps identify underutilized or overburdened resources, minimize setup or changeover times, and ensure efficient utilization of labor, equipment, and materials. By allocating resources optimally, manufacturers can enhance productivity, reduce costs, and achieve better overall operational efficiency.
- Enhanced Decision-Making: Data-driven production planning provides manufacturers with actionable insights for informed decision-making. By analyzing data on production processes, inventory levels, quality control metrics, and other relevant factors, manufacturers can identify bottlenecks, quality issues, and inefficiencies. These insights enable timely decision-making, proactive problem-solving, and continuous process improvements, resulting in better outcomes and higher customer satisfaction.
- Real-time Visibility and Collaboration: Advanced data-driven production planning systems provide real-time visibility into production processes, inventory levels, and resource utilization. This visibility enables better coordination, collaboration, and communication among different departments and stakeholders involved in the production process. Real-time data sharing and collaboration foster a more agile and responsive manufacturing environment, facilitating timely adjustments to production plans and quick resolution of issues.
- Continuous Improvement: Data-driven production planning facilitates a culture of continuous improvement by providing meaningful performance metrics and actionable insights. By analyzing production data, identifying trends, and benchmarking against key performance indicators (KPIs), manufacturers can continuously optimize processes, reduce waste, and enhance operational efficiency over time.
In summary, the need for a more advanced and data-driven approach to production planning arises from the increasing complexity of manufacturing, dynamic market demands, and the desire for improved accuracy, resource optimization, decision-making, visibility, collaboration, and continuous improvement.
Section 3: Introduction to Data-driven Production Planning
In the era of data-driven decision-making, the application of data-driven production planning has emerged as a transformative approach in the manufacturing industry. By harnessing the power of data and advanced analytics, manufacturers can optimize their production processes, enhance resource allocation, and respond swiftly to dynamic market demands.
In this section, we will delve into the concept of data-driven production planning, exploring its key principles, benefits, and implementation strategies.
By embracing data-driven methodologies, manufacturers can unlock new opportunities for operational efficiency, cost reduction, and customer satisfaction, setting the stage for a more agile and competitive manufacturing environment.
Concept of data-driven production planning and its reliance on real-time and historical data
Data-driven production planning is an approach that leverages real-time and historical data to optimize production processes, improve decision-making, and enhance overall operational efficiency in manufacturing. It involves collecting, analyzing, and utilizing data from various sources, such as production systems, sensors, supply chain data, customer feedback, and market trends.
Real-time data plays a crucial role in data-driven production planning as it provides up-to-the-minute information on production activities, resource utilization, and demand fluctuations. By monitoring real-time data, manufacturers gain visibility into the current status of production processes, allowing them to identify bottlenecks, track inventory levels, and respond promptly to changing circumstances. Real-time data helps in making agile and informed decisions, ensuring that production plans remain aligned with the latest information.
Historical data, on the other hand, provides valuable insights into past performance, trends, and patterns. It allows manufacturers to analyze historical demand patterns, production cycles, lead times, and quality metrics. By examining historical data, manufacturers can identify seasonality, forecast future demand, and establish benchmarks for productivity and efficiency. Historical data acts as a foundation for generating accurate demand forecasts, setting realistic production targets, and optimizing resource allocation.
The reliance on real-time and historical data in data-driven production planning enables manufacturers to adopt a proactive and predictive approach. Real-time data helps in identifying and addressing issues as they arise, while historical data provides a basis for predictive modeling and scenario analysis. By combining the two, manufacturers can optimize production schedules, align resources, mitigate risks, and improve overall operational performance.
To effectively utilize real-time and historical data, manufacturers often employ advanced analytics techniques, such as machine learning algorithms, predictive modeling, and data visualization tools. These technologies enable manufacturers to extract meaningful insights from data, identify patterns, forecast demand, and simulate various production scenarios. By leveraging these insights, manufacturers can make data-driven decisions, streamline production processes, and continuously improve performance.
Overall, the concept of data-driven production planning emphasizes the importance of leveraging both real-time and historical data to drive informed decision-making and achieve operational excellence. By utilizing the power of data, manufacturers can optimize production, improve resource allocation, reduce costs, and meet customer demands more efficiently and effectively.
Benefits of leveraging data in production planning
Leveraging data in production planning brings several benefits that contribute to improved operational efficiency and better decision-making.
Here are some key advantages:
- Improved Forecasting Accuracy: Data-driven production planning enables manufacturers to leverage historical and real-time data to enhance demand forecasting accuracy. By analyzing past trends, market dynamics, and customer behavior, manufacturers can generate more precise demand forecasts. This leads to optimized inventory management, reduced stockouts, minimized excess inventory, and improved customer service levels.
- Optimized Resource Allocation: Data-driven production planning allows manufacturers to optimize resource allocation by analyzing production data, capacity utilization, and performance metrics. By understanding resource availability, capabilities, and constraints, manufacturers can make informed decisions on labor allocation, equipment utilization, and materials sourcing. Optimized resource allocation leads to increased productivity, reduced costs, and improved overall operational efficiency.
- Enhanced Decision-Making: Data-driven production planning provides manufacturers with actionable insights for informed decision-making. By analyzing production data, quality metrics, and other relevant factors, manufacturers can identify bottlenecks, anticipate issues, and make proactive decisions. Real-time data and advanced analytics tools enable rapid and data-supported decision-making, leading to improved responsiveness, agility, and the ability to seize opportunities in dynamic market conditions.
- Efficient Production Planning and Scheduling: Leveraging data allows manufacturers to create more efficient production plans and schedules. By incorporating real-time production data, demand forecasts, and resource constraints, manufacturers can optimize production sequences, minimize changeover times, and reduce idle time. This results in increased throughput, reduced lead times, and improved overall production efficiency.
- Continuous Improvement: Data-driven production planning facilitates a culture of continuous improvement. By analyzing production data, manufacturers can identify inefficiencies, performance gaps, and areas for improvement. This enables targeted process optimization, quality enhancements, and the implementation of best practices. Continuous improvement initiatives based on data-driven insights drive ongoing efficiency gains and promote a culture of operational excellence.
- Proactive Issue Identification and Mitigation: Data-driven production planning enables the early identification of potential issues and risks. By monitoring real-time data, manufacturers can detect deviations from expected performance, quality issues, or supply chain disruptions. This allows for proactive problem-solving, preventing costly disruptions, reducing downtime, and ensuring smooth production operations.
- Better Supply Chain Collaboration: Leveraging data in production planning improves collaboration and communication with suppliers and other stakeholders in the supply chain. By sharing real-time production data, demand forecasts, and inventory levels, manufacturers can collaborate more effectively with suppliers, enabling timely adjustments, and enhancing overall supply chain efficiency.
Data-driven approaches empower manufacturers to be more responsive, agile, and efficient in meeting customer demands and navigating the complexities of the modern manufacturing landscape.
The role of technologies
Technologies such as the Internet of Things (IoT), big data analytics, and machine learning play a crucial role in enabling data-driven production planning. They provide the foundation for collecting, analyzing, and leveraging vast amounts of data to optimize production processes and enhance decision-making. Here's a closer look at their roles:
Internet of Things (IoT)
IoT devices are instrumental in capturing real-time data from various points within the production environment. Connected sensors and devices embedded in machinery, equipment, and production lines collect data on factors like temperature, pressure, speed, and energy consumption.
This data provides valuable insights into the performance, efficiency, and health of production assets. By integrating IoT devices into production systems, manufacturers gain real-time visibility into operations, enabling proactive monitoring, predictive maintenance, and timely intervention to prevent disruptions.
Big Data Analytics
Big data analytics refers to the process of extracting valuable insights from large and complex datasets. In the context of data-driven production planning, big data analytics enables manufacturers to analyze vast amounts of data collected from multiple sources, such as production systems, sensors, supply chain data, and customer feedback.
Advanced analytics techniques, including statistical analysis, data mining, and pattern recognition, are applied to uncover hidden patterns, correlations, and trends. This analysis helps manufacturers identify production bottlenecks, predict demand patterns, optimize resource allocation, and make data-driven decisions that improve operational efficiency.
Machine Learning
Machine learning is a subset of artificial intelligence that focuses on enabling systems to learn and improve from data without explicit programming. In data-driven production planning, machine learning algorithms can process large volumes of data, detect patterns, and make predictions or recommendations.
For example, machine learning algorithms can learn from historical production data to generate accurate demand forecasts, optimize production schedules, and identify optimal resource allocation strategies. Machine learning algorithms can also identify anomalies or deviations from expected performance, helping manufacturers identify quality issues or potential disruptions before they occur.
By harnessing the power of IoT, big data analytics, and machine learning, manufacturers can leverage real-time and historical data to drive data-driven production planning.
These technologies enable manufacturers to capture and analyze vast amounts of data, uncover valuable insights, and make informed decisions that optimize production processes, enhance resource allocation, and improve overall operational efficiency.
The integration of these technologies into production planning systems empowers manufacturers to operate with greater precision, agility, and responsiveness in a rapidly evolving manufacturing landscape.
Section 4: Key Components of Data-driven Production Planning
In the realm of data-driven production planning, several key components work together to enable manufacturers to optimize their operations and achieve higher levels of efficiency and productivity. These components encompass various elements, including data collection, analysis, forecasting, optimization, and visualization.
In this section, we will explore the key components of data-driven production planning, shedding light on their significance and how they contribute to the successful implementation of a data-driven approach.
By understanding these essential components, manufacturers can unlock the full potential of data-driven production planning and harness its benefits to drive operational excellence.
Key components of an effective data-driven production planning system
An effective data-driven production planning system consists of several key components that work in tandem to enable manufacturers to optimize their production processes and achieve operational excellence. Here are the key components:
Data Collection: The foundation of a data-driven production planning system is data collection. It involves gathering relevant data from various sources, such as production systems, sensors, supply chain data, customer feedback, and market trends. This data encompasses information on production volumes, resource utilization, quality metrics, demand patterns, and other crucial parameters.
Automated data collection methods, such as IoT devices and connected sensors, ensure real-time data capture, providing a comprehensive view of the production environment.
Data Analysis: Data analysis is the process of extracting meaningful insights from collected data. It involves applying statistical techniques, data mining, and exploratory analysis to uncover patterns, correlations, and trends within the data. Through data analysis, manufacturers can identify production bottlenecks, understand demand patterns, and assess performance metrics.
Advanced analytics tools and algorithms enable manufacturers to derive actionable insights from the data, guiding decision-making and optimizing production planning processes.
Modeling and Forecasting: Modeling and forecasting are critical components of data-driven production planning. Manufacturers use historical data, combined with statistical models and machine learning algorithms, to create accurate demand forecasts.
These models consider various factors such as seasonality, market trends, and historical sales data. By leveraging these forecasts, manufacturers can align production plans with anticipated demand, optimize inventory levels, and enhance resource allocation. Modeling and forecasting enable manufacturers to make informed decisions based on reliable predictions of future demand.
Optimization: Optimization is a key component that involves finding the best possible solution for production planning. Using mathematical optimization algorithms and techniques, manufacturers can determine optimal production schedules, resource allocation strategies, and inventory management approaches.
Optimization considers multiple variables and constraints, such as production capacity, labor availability, material availability, and customer demand. By optimizing production plans, manufacturers can maximize efficiency, minimize costs, and meet customer demands while considering resource limitations and operational constraints.
These key components work in a cyclical and iterative manner within a data-driven production planning system. Data is collected, analyzed, and used to build models and forecasts. These models and forecasts then inform the optimization process, which generates optimized production plans and resource allocation strategies. The system continuously learns from real-time data, updates models and forecasts, and refines optimization algorithms, allowing for continuous improvement and adaptability to changing market conditions.
By integrating these key components into their production planning processes, manufacturers can achieve enhanced operational efficiency, improved resource allocation, and better decision-making.
Data-driven production planning systems empower manufacturers to respond effectively to market dynamics, reduce costs, optimize production processes, and deliver products in a timely manner, ultimately leading to increased customer satisfaction and a competitive edge in the manufacturing industry.
How real-time data from sensors and connected devices can be used to monitor production processes and identify bottlenecks or inefficiencies
Real-time data from sensors and connected devices plays a crucial role in monitoring production processes and identifying bottlenecks or inefficiencies. Here's how it can be used:
- Real-time Monitoring: Sensors and connected devices embedded in machinery, equipment, and production lines continuously capture data on various parameters, such as temperature, pressure, speed, vibration, and energy consumption. This real-time data provides a live view of the production processes, allowing manufacturers to monitor the performance and health of their equipment in real-time. By analyzing this data, manufacturers can quickly identify deviations from normal operating conditions and detect potential issues or inefficiencies.
- Performance Metrics: Real-time data enables the tracking of key performance metrics related to production processes. By collecting and analyzing data on factors such as cycle time, throughput, quality metrics, and machine uptime, manufacturers can assess the efficiency and effectiveness of their production operations in real-time. Deviations from established performance benchmarks can indicate bottlenecks or inefficiencies that require attention.
- Predictive Analytics: Real-time data can be leveraged for predictive analytics, enabling manufacturers to anticipate and address potential bottlenecks or inefficiencies before they occur. By monitoring real-time data trends and comparing them to historical patterns, machine learning algorithms can identify early warning signs of production issues. This allows manufacturers to take proactive measures, such as adjusting production schedules, reallocating resources, or performing preventive maintenance, to mitigate bottlenecks and optimize production processes.
- Alerting and Notifications: Real-time data can trigger alerts and notifications to relevant stakeholders when specific thresholds or conditions are met. For example, if a machine's temperature exceeds a certain limit or if production output falls below a predetermined threshold, an alert can be sent to production managers or maintenance personnel. This immediate notification enables prompt action, helping to resolve bottlenecks or inefficiencies in a timely manner.
- Visualization and Dashboards: Real-time data can be visualized through interactive dashboards and visual displays. These visual representations provide a clear and intuitive overview of the production processes, highlighting performance metrics, equipment status, and production trends in real-time. Manufacturers can monitor these dashboards to identify bottlenecks or inefficiencies at a glance, enabling quick decision-making and intervention.
The ability to monitor performance metrics, detect deviations, and receive alerts in real-time empowers manufacturers to identify bottlenecks or inefficiencies promptly. This enables them to take proactive measures to optimize production processes, minimize downtime, and improve overall operational efficiency.
Real-time data-driven monitoring ensures that manufacturers can continuously monitor and optimize their production operations, leading to enhanced productivity, reduced costs, and improved customer satisfaction.
Importance of accurate data analysis and modeling in generating actionable insights for production planning
Accurate data analysis and modeling are crucial in generating actionable insights for production planning. Here's why they are important:
Informed Decision-Making: Accurate data analysis and modeling provide manufacturers with a solid foundation for making informed decisions. By analyzing production data, market trends, customer behavior, and other relevant factors, manufacturers can gain valuable insights into their production processes and the external factors that impact them.
These insights enable decision-makers to understand the current state of operations, identify areas for improvement, and make data-driven decisions to optimize production planning strategies.
Demand Forecasting and Planning: Accurate data analysis and modeling are essential for generating reliable demand forecasts. By analyzing historical sales data, market trends, customer preferences, and other relevant variables, manufacturers can develop accurate predictions of future demand.
This enables them to align production plans, inventory levels, and resource allocation strategies with anticipated customer requirements. Accurate demand forecasting helps manufacturers avoid stockouts, minimize excess inventory, and ensure timely delivery of products, ultimately improving customer satisfaction and optimizing production efficiency.
Resource Optimization: Accurate data analysis and modeling enable manufacturers to optimize resource allocation. By analyzing production data, performance metrics, and capacity utilization, manufacturers can identify opportunities to improve resource efficiency.
They can determine the optimal allocation of labor, equipment, and materials to maximize productivity, minimize costs, and meet production targets. Accurate data analysis helps manufacturers identify production bottlenecks, address capacity constraints, and optimize the utilization of resources, leading to improved operational efficiency.
Process Improvement: Accurate data analysis and modeling help identify areas for process improvement within production planning. By analyzing production data, manufacturers can identify inefficiencies, bottlenecks, and areas of underperformance. This allows them to implement targeted process improvement initiatives and optimize workflows.
Accurate data analysis helps manufacturers uncover root causes of production issues, implement corrective actions, and continuously improve production processes, resulting in enhanced productivity and quality.
Risk Mitigation: Accurate data analysis and modeling enable manufacturers to identify and mitigate risks associated with production planning. By analyzing historical data, performance metrics, and market trends, manufacturers can identify potential risks and anticipate their impact on production operations.
This allows them to develop contingency plans, proactively address potential disruptions, and minimize the negative impact on production schedules and customer deliveries. Accurate data analysis helps manufacturers improve their ability to manage risks and maintain a resilient production environment.
Accurate data analysis and modeling provide manufacturers with insights that drive actionable decision-making and optimization in production planning.
The ability to generate actionable insights from data analysis and modeling enables manufacturers to achieve higher levels of efficiency, productivity, and customer satisfaction in their production operations.
Section 5: Implementation and Integration Challenges
Implementing and integrating data-driven production planning systems in manufacturing environments can pose various challenges that need to be overcome for successful implementation. These challenges encompass technical, organizational, and cultural aspects that require careful consideration and planning.
In this section, we will explore the key challenges involved in implementing and integrating data-driven production planning systems and discuss strategies to address them effectively.
By understanding these challenges and their potential solutions, manufacturers can navigate the implementation process smoothly and unlock the full potential of data-driven production planning to drive operational excellence.
Addressing the potential challenges and considerations in implementing a data-driven production planning system
Implementing a data-driven production planning system in a manufacturing environment can be a complex undertaking. It involves addressing various challenges and considerations to ensure successful implementation. Here are some key challenges and strategies to overcome them:
Data Quality and Integration: One of the primary challenges is ensuring the quality and integration of data from different sources. Manufacturers need to establish data governance practices to ensure data accuracy, consistency, and completeness.
This involves defining data standards, validating data integrity, and implementing data integration processes to consolidate data from disparate systems. Employing data cleansing and transformation techniques can enhance data quality and facilitate seamless integration.
IT Infrastructure and Integration: Implementing a data-driven production planning system requires a robust and scalable IT infrastructure. This infrastructure should support the storage, processing, and analysis of large volumes of data.
Manufacturers need to assess their existing IT capabilities, identify any gaps, and make necessary upgrades or investments. Integration with existing systems, such as Enterprise Resource Planning (ERP) systems and Manufacturing Execution Systems (MES), should be carefully planned and executed to ensure seamless data flow and system interoperability.
Organizational Readiness and Change Management: Adopting a data-driven production planning system involves organizational changes and may encounter resistance from employees. It is crucial to create awareness and foster a culture of data-driven decision-making within the organization.
Employees need to understand the benefits of the new system and receive adequate training to effectively utilize the system. Change management strategies, such as communication, education, and stakeholder engagement, should be implemented to facilitate a smooth transition and gain buy-in from employees.
Skillset and Expertise: Implementing a data-driven production planning system requires a skilled workforce with expertise in data analytics, modeling, and optimization. Manufacturers may need to upskill existing employees or hire new talent to bridge any skill gaps.
Providing training and professional development opportunities can enhance the capabilities of the workforce and enable them to effectively utilize the data-driven tools and techniques.
Data Security and Privacy: As data becomes a critical asset in the production planning process, ensuring data security and privacy is paramount. Manufacturers need to implement robust data security measures, including access controls, encryption, and secure data transmission protocols. Compliance with relevant data protection regulations, such as GDPR or CCPA, is crucial to maintain customer trust and protect sensitive information.
Continuous Improvement and Adaptability: Implementing a data-driven production planning system is not a one-time event. It requires a mindset of continuous improvement and adaptability. Manufacturers should establish feedback loops and mechanisms to monitor system performance, collect user feedback, and incorporate improvements.
Regular evaluation and refinement of the system based on changing business needs, technological advancements, and industry trends are essential for long-term success.
By addressing these challenges and considerations, manufacturers can successfully implement a data-driven production planning system. It requires a holistic approach, involving technology, people, and processes.
Effective planning, stakeholder engagement, and a clear implementation roadmap are key to overcoming these challenges and leveraging the power of data-driven production planning to achieve operational efficiency, optimize resource utilization, and enhance overall competitiveness in the manufacturing industry.
Need for data integration from various sources and systems within the manufacturing environment
In a manufacturing environment, the need for data integration from various sources and systems is crucial for effective production planning and optimization. Here are some reasons highlighting the importance of data integration:
Holistic View of Operations: Manufacturing operations generate data from multiple sources such as production equipment, sensors, quality control systems, inventory management systems, and supply chain systems. Integrating data from these diverse sources provides a holistic view of the entire manufacturing process.
It allows manufacturers to analyze and understand the interdependencies between different aspects of production, identify bottlenecks, and make informed decisions to optimize the overall process.
Accurate and Real-time Information: Data integration ensures that relevant information from different systems is consolidated and updated in real-time. This enables manufacturers to access accurate and up-to-date information about production status, inventory levels, equipment performance, and other critical parameters.
Real-time access to integrated data allows for proactive decision-making, rapid response to changes, and timely adjustments to production plans, ensuring efficient operations and minimizing disruptions.
Improved Efficiency and Resource Utilization: Integrating data from various systems provides insights into the utilization of resources, such as equipment, labor, and materials. By analyzing this integrated data, manufacturers can identify opportunities to optimize resource allocation, streamline workflows, and eliminate inefficiencies.
For example, integrating data on equipment utilization and maintenance can help identify underutilized machines or maintenance needs, allowing for better scheduling and utilization of resources.
Enhanced Visibility and Transparency: Data integration enables a centralized and transparent view of production operations. It allows stakeholders at different levels to access relevant data and gain insights into the entire production process.
With improved visibility, managers can track performance metrics, monitor progress against production targets, and identify areas for improvement. This transparency promotes collaboration, facilitates data-driven decision-making, and aligns different departments towards shared production goals.
Seamless Supply Chain Integration: Data integration plays a critical role in integrating the manufacturing process with the broader supply chain. By integrating data from suppliers, logistics partners, and customers, manufacturers can gain end-to-end visibility into the supply chain, optimize inventory levels, and synchronize production plans with customer demand.
Seamless integration allows for effective demand forecasting, efficient order management, and just-in-time production, reducing lead times and enhancing customer satisfaction.
Advanced Analytics and Predictive Insights: Data integration provides a comprehensive dataset that can be leveraged for advanced analytics and predictive insights. By combining data from various sources, manufacturers can apply advanced analytical techniques and machine learning algorithms to uncover patterns, correlations, and trends.
This enables predictive modeling for demand forecasting, maintenance planning, quality control, and other critical aspects of production planning. These predictive insights empower manufacturers to make proactive decisions, anticipate challenges, and optimize production processes.
In summary, data integration from various sources and systems within the manufacturing environment is essential for obtaining a holistic view of operations, accessing accurate and real-time information.
All these factors help in improving efficiency and resource utilization, enhancing visibility and transparency, enabling seamless supply chain integration, and unlocking the power of advanced analytics.
Importance of data quality, data security, and data governance in ensuring the success of a data-driven approach
Data quality, data security, and data governance are vital aspects of ensuring the success of a data-driven approach in manufacturing. Here's why they are important:
Data Quality: Data quality refers to the accuracy, completeness, consistency, and relevance of data. High-quality data is essential for reliable analysis and decision-making. In a data-driven approach, accurate and trustworthy data is crucial for generating actionable insights and making informed decisions.
Poor data quality can lead to flawed analysis, erroneous conclusions, and ineffective production planning. By ensuring data quality through data validation, cleansing, and data quality management practices, manufacturers can rely on accurate data to drive their decision-making processes.
Data Security: Data security is crucial in protecting sensitive information and ensuring the confidentiality, integrity, and availability of data. In a data-driven approach, manufacturers deal with vast amounts of data, including production data, customer data, intellectual property, and trade secrets.
Data breaches or unauthorized access to this data can have severe consequences, including financial losses, reputational damage, and legal implications. Implementing robust data security measures, such as access controls, encryption, and data protection protocols, safeguards data from unauthorized access, ensuring the integrity and confidentiality of data assets.
Data Governance: Data governance involves establishing policies, processes, and controls to manage data effectively throughout its lifecycle. It ensures that data is managed consistently, complies with regulations, and aligns with the organization's goals and objectives. In a data-driven approach, data governance ensures that data is captured, stored, processed, and utilized in a standardized and controlled manner.
It establishes roles and responsibilities for data management, defines data ownership, and enforces data quality standards and data usage policies. Effective data governance ensures the reliability, consistency, and availability of data, fostering trust in the data-driven decision-making process.
Compliance with Regulations: Manufacturers must comply with various data protection and privacy regulations, such as the General Data Protection Regulation (GDPR) or industry-specific regulations. These regulations govern the collection, storage, processing, and sharing of personal and sensitive data.
Non-compliance can lead to severe penalties and reputational damage. By implementing data governance practices and ensuring data security, manufacturers can demonstrate compliance with regulations, protect customer privacy, and build trust with stakeholders.
Ethical Considerations: In a data-driven approach, ethical considerations surrounding data usage and privacy are essential. Manufacturers must handle data responsibly, ensuring transparency and obtaining consent when collecting and utilizing personal data. Ethical data practices contribute to maintaining a positive brand image, fostering customer loyalty, and building strong relationships with stakeholders.
Reliable and accurate data, protected by robust security measures and governed by effective data governance practices, forms the foundation for deriving actionable insights, making informed decisions, and optimizing production planning processes. It also helps manufacturers comply with regulations, maintain ethical standards, and build trust with customers, partners, and stakeholders.
Section 6: Case Studies and Success Stories
In this section, we delve into real-world case studies and success stories that highlight the practical application and positive outcomes of data-driven production planning in the manufacturing industry.
These case studies provide valuable insights into how organizations have leveraged data-driven approaches to optimize their production processes, enhance efficiency, and achieve remarkable results.
Real-world examples of manufacturers who have successfully implemented data-driven production planning strategies
- General Electric (GE): GE implemented data-driven production planning in its gas turbine manufacturing plants. By integrating data from sensors embedded in their turbines, they collected real-time performance data and combined it with historical data to create predictive models. This allowed them to optimize maintenance schedules, identify potential equipment failures in advance, and improve overall production efficiency.
- BMW: BMW implemented data-driven production planning to optimize its manufacturing processes. They used real-time data from various sources, including sensors on the production line and RFID tags on components, to monitor the production flow, identify bottlenecks, and optimize resource allocation. This approach helped BMW improve production efficiency, reduce downtime, and enhance product quality.
- Procter & Gamble (P&G): P&G implemented data-driven production planning across its global manufacturing operations. They integrated data from various sources, including sales forecasts, customer demand, and production capacity, to generate optimized production plans. This allowed P&G to align production with market demand, reduce inventory costs, and improve customer satisfaction.
- Airbus: Airbus leveraged data-driven production planning to optimize its aircraft manufacturing processes. By integrating data from sensors, they monitored equipment performance, identified maintenance needs in real-time, and optimized production schedules. This approach helped Airbus reduce downtime, enhance productivity, and ensure on-time delivery of aircraft.
- Toyota: Toyota implemented data-driven production planning as part of its lean manufacturing approach. They used real-time data from the production line to identify bottlenecks, optimize workflow, and reduce waste. By continuously monitoring and analyzing data, Toyota achieved significant improvements in production efficiency, quality, and overall operational excellence.
- Amazon: Amazon utilizes data-driven production planning strategies in its fulfillment centers. By analyzing real-time data from inventory systems, order volumes, and shipping times, they optimize product placement, inventory levels, and delivery schedules. This approach allows Amazon to streamline operations, reduce fulfillment time, and meet customer demands efficiently.
These real-world examples demonstrate the successful implementation of data-driven production planning strategies in diverse manufacturing contexts. Their success highlights the transformative potential of data-driven approaches in modern manufacturing operations.
Challenges they faced and how data-driven approaches helped them overcome those challenges
General Electric (GE): GE faced the challenge of optimizing maintenance schedules for their gas turbines. Traditional approaches relied on fixed time intervals for maintenance, which often resulted in unnecessary downtime or missed opportunities for preventive maintenance.
By implementing data-driven production planning, GE leveraged real-time performance data and predictive models to identify maintenance needs accurately. This allowed them to optimize maintenance schedules, perform proactive maintenance, and minimize unplanned downtime, ultimately improving production efficiency and reducing costs.
BMW: BMW faced challenges in identifying and resolving bottlenecks in their production processes. Without real-time visibility into the production flow, it was difficult to identify areas causing delays or inefficiencies. With data-driven production planning, BMW integrated data from sensors and RFID tags to monitor the production line in real-time.
This enabled them to identify bottlenecks promptly, analyze root causes, and make informed decisions to optimize resource allocation, eliminate inefficiencies, and improve overall production efficiency.
Procter & Gamble (P&G): P&G encountered challenges in aligning their production with customer demand and reducing inventory costs. Traditional production planning methods relied on historical data and static forecasts, leading to suboptimal production plans.
By implementing data-driven production planning, P&G integrated real-time sales forecasts, customer demand data, and production capacity information. This allowed them to generate optimized production plans that dynamically aligned with market demand, reduced inventory costs, and improved customer satisfaction.
Airbus: Airbus faced challenges in equipment maintenance and ensuring on-time delivery of aircraft. Traditional maintenance practices often relied on fixed schedules or reactive maintenance, leading to potential equipment failures and delays.
With data-driven production planning, Airbus utilized real-time data from sensors to monitor equipment performance and detect maintenance needs in advance. This proactive approach enabled them to optimize maintenance schedules, reduce downtime, and ensure on-time delivery of aircraft.
Toyota: Toyota encountered challenges in identifying and eliminating waste in their production processes. Traditional approaches relied on manual observation and periodic analysis, making it difficult to identify and address inefficiencies in real-time.
By implementing data-driven production planning, Toyota integrated real-time data from the production line, allowing them to monitor and analyze production processes continuously. This enabled them to identify bottlenecks, reduce waste, optimize workflow, and improve overall production efficiency.
Amazon: Amazon faced challenges in optimizing inventory levels and meeting customer demands efficiently. Traditional inventory management practices often relied on fixed reorder points or periodic reviews, leading to stockouts or excessive inventory. With data-driven production planning, Amazon utilized real-time data from inventory systems, order volumes, and shipping times.
This allowed them to dynamically adjust inventory levels, optimize product placement, and streamline delivery schedules. By leveraging data-driven insights, Amazon improved inventory management, reduced fulfillment time, and enhanced customer satisfaction.
In each of these cases, data-driven approaches helped manufacturers overcome traditional challenges by providing real-time visibility, accurate insights, and predictive capabilities.
Measurable benefits achieved through data-driven approach
The implementation of data-driven production planning strategies has yielded measurable benefits for manufacturers. Here are some of the notable outcomes achieved:
- Increased Production Efficiency: By leveraging data-driven approaches, manufacturers have experienced significant improvements in production efficiency. Real-time monitoring and optimization techniques have allowed them to identify and address bottlenecks, streamline workflows, and eliminate waste. As a result, manufacturers have achieved higher throughput rates, reduced cycle times, and increased overall production efficiency.
- Reduced Lead Times: Data-driven production planning has enabled manufacturers to optimize production schedules and resource allocation, leading to reduced lead times. By accurately predicting demand, dynamically adjusting production plans, and aligning production with customer requirements, manufacturers have been able to shorten lead times and respond more quickly to market demands.
- Improved Customer Satisfaction: Data-driven approaches have had a positive impact on customer satisfaction. By aligning production with customer demand and reducing lead times, manufacturers can fulfill orders more efficiently, deliver products on time, and improve overall service levels. Enhanced product quality, achieved through data-driven insights, has also contributed to improved customer satisfaction and loyalty.
- Enhanced Resource Allocation: Data-driven production planning facilitates optimized resource allocation. By analyzing data on production capacity, material availability, and workforce efficiency, manufacturers can allocate resources effectively, ensuring their optimal utilization. This leads to improved resource productivity, reduced idle time, and cost savings.
- Better Inventory Management: Data-driven approaches have improved inventory management practices. By analyzing real-time data on inventory levels, customer demand, and production forecasts, manufacturers can optimize inventory levels, reducing stockouts and excessive inventory. This results in cost savings, improved cash flow, and more efficient use of warehouse space.
- Accurate Demand Forecasting: Data-driven production planning enables accurate demand forecasting by analyzing historical data, market trends, and customer insights. This helps manufacturers anticipate fluctuations in demand, align production levels accordingly, and avoid overproduction or stockouts. Accurate demand forecasting leads to improved resource planning, minimized production disruptions, and enhanced overall operational efficiency.
These measurable benefits highlight the tangible outcomes achieved by implementing data-driven production planning strategies.
Summarizing the Advantages of Adopting a Data-Driven Approach to Production Planning
Adopting a data-driven approach to production planning in manufacturing offers several key advantages. These advantages can be summarized as follows:
- Enhanced Efficiency: By leveraging real-time and historical data, manufacturers can optimize production processes, identify bottlenecks, streamline workflows, and eliminate waste. This leads to increased production efficiency, improved throughput rates, and reduced cycle times.
- Improved Decision-Making: Data-driven production planning provides manufacturers with accurate insights and predictive capabilities. This enables informed decision-making, such as optimizing resource allocation, adjusting production schedules, and aligning production with customer demand. Data-driven insights facilitate proactive decision-making to enhance operational performance.
- Cost Reduction: Data-driven approaches help identify opportunities for cost reduction. By optimizing resource utilization, inventory levels, and production schedules, manufacturers can minimize production disruptions, reduce inventory costs, and eliminate unnecessary expenses. This contributes to overall cost savings and improved profitability.
- Accurate Demand Forecasting: Leveraging data for demand forecasting enables manufacturers to anticipate market fluctuations and customer demands. Accurate forecasting leads to optimized production planning, preventing stockouts or overproduction. Manufacturers can align their production levels with customer demand, reducing inventory costs and improving customer satisfaction.
- Improved Quality and Customer Satisfaction: Data-driven production planning allows manufacturers to monitor and analyze production processes in real-time. This helps identify quality issues promptly, leading to proactive quality control measures. Improved product quality enhances customer satisfaction, strengthens brand reputation, and fosters long-term customer loyalty.
- Optimal Resource Allocation: Data-driven approaches enable manufacturers to optimize resource allocation. By analyzing data on production capacity, material availability, and workforce efficiency, manufacturers can ensure the optimal utilization of resources. This results in improved resource productivity, reduced downtime, and cost savings.
- Agility and Adaptability: With real-time data and insights, manufacturers can quickly respond to changing market conditions and customer demands. Data-driven production planning enables agility in adjusting production plans, inventory levels, and supply chain operations, ensuring responsiveness and adaptability in a dynamic manufacturing landscape.
Embracing Data-Driven Production Planning for Competitive Advantage in the Evolving Manufacturing Landscape
In the rapidly evolving manufacturing landscape, embracing data-driven production planning is critical for manufacturers seeking to gain a competitive edge. Leveraging real-time and historical data, manufacturers can enhance agility, achieve cost efficiency, improve quality and customer satisfaction, differentiate themselves from competitors, and make informed decisions that optimize their production processes.
By embracing data-driven approaches, manufacturers can adapt to the changing market dynamics, deliver superior products, and position themselves for success in the highly competitive manufacturing industry.
How can Deskera Help You?
Deskera ERP and MRP systems help you to keep your business units organized. The system's primary functions are as follows:
- Keep track of your raw materials and final items inventories
- Control production schedules and routings
- Keep a bill of materials
- Produce thorough reports
- Make your own dashboards
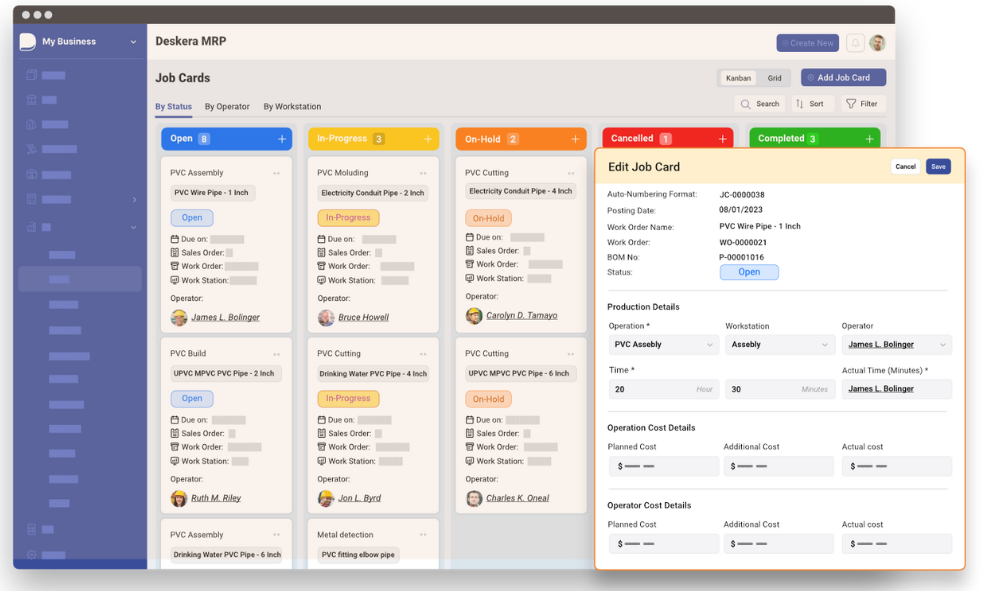
Deskera's integrated financial planning tools enable investors to better plan and track their investments. It can assist investors in making faster and more accurate decisions.
Deskera Books allows you to better manage your accounts and finances. Maintain good accounting practices by automating tasks like billing, invoicing, and payment processing.
Deskera CRM is a powerful solution that manages your sales and helps you close deals quickly. It not only enables you to perform critical tasks like lead generation via email, but it also gives you a comprehensive view of your sales funnel.
Deskera People is a straightforward tool for centralizing your human resource management functions.
Key Takeaways
- Data-driven production planning leverages real-time and historical data to optimize production processes, improve efficiency, and drive operational excellence.
- The objectives of data-driven production planning include meeting customer demand, reducing costs, improving resource allocation, and enhancing decision-making.
- Poor production planning can lead to stockouts, production bottlenecks, excessive inventory, and decreased productivity, quality, and customer satisfaction.
- Traditional production planning methods often face limitations such as manual data entry, reliance on historical data, and limited visibility into real-time production processes.
- Data-driven production planning overcomes these challenges by using advanced technologies like IoT, big data analytics, and machine learning to collect, analyze, model, and optimize production data.
- The benefits of leveraging data in production planning include improved forecasting accuracy, optimized resource allocation, enhanced decision-making, and better inventory management.
- Technologies like IoT enable real-time data collection from sensors and connected devices, allowing manufacturers to monitor production processes, identify bottlenecks, and improve efficiency.
- Accurate data analysis and modeling generate actionable insights for production planning, enabling manufacturers to make informed decisions and optimize their operations.
- Data integration from various sources and systems within the manufacturing environment is crucial for effective data-driven production planning.
- Ensuring data quality, data security, and data governance is essential for the success of a data-driven approach. By establishing robust data quality controls, manufacturers can ensure that the data used for production planning is accurate, reliable, and consistent.
Related Articles
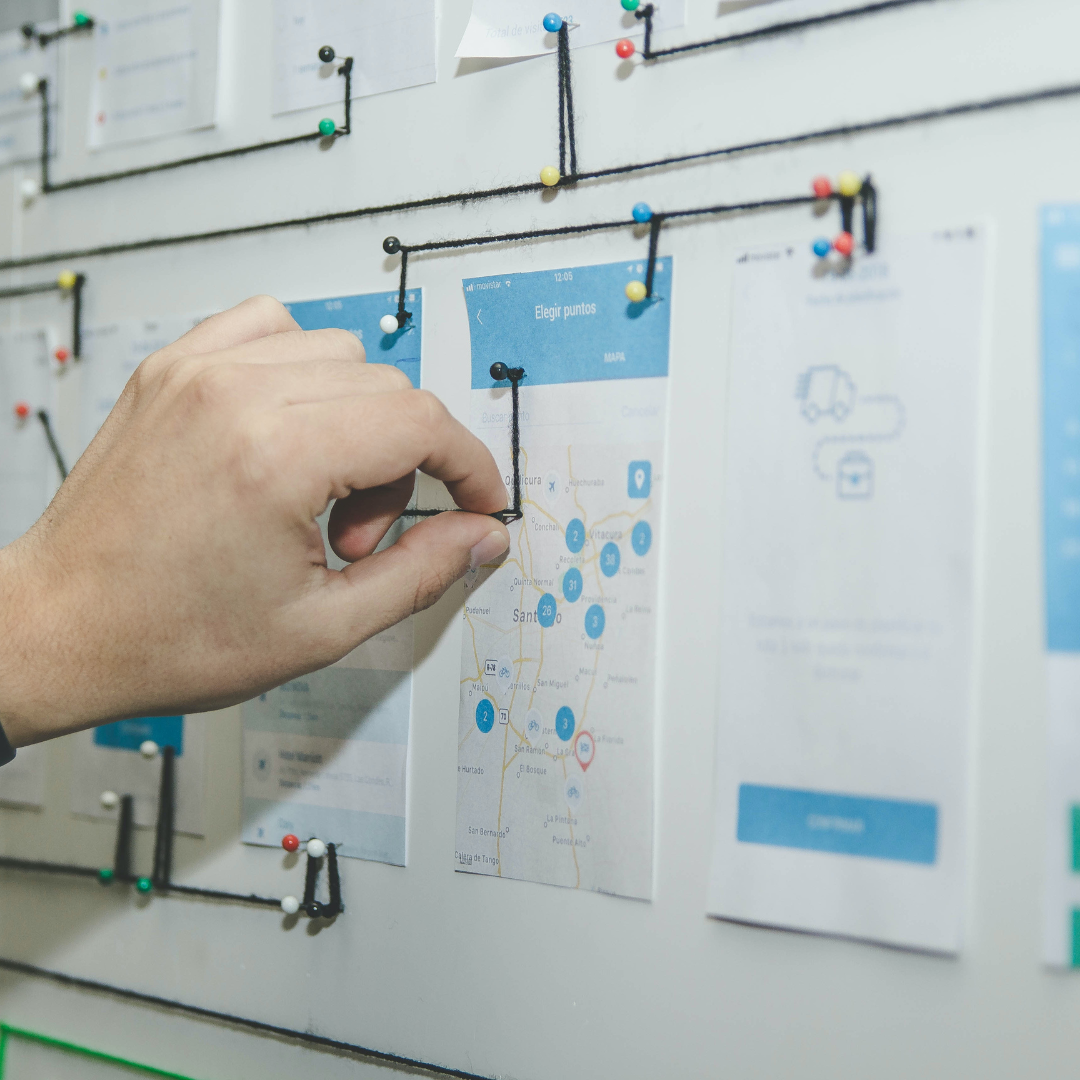
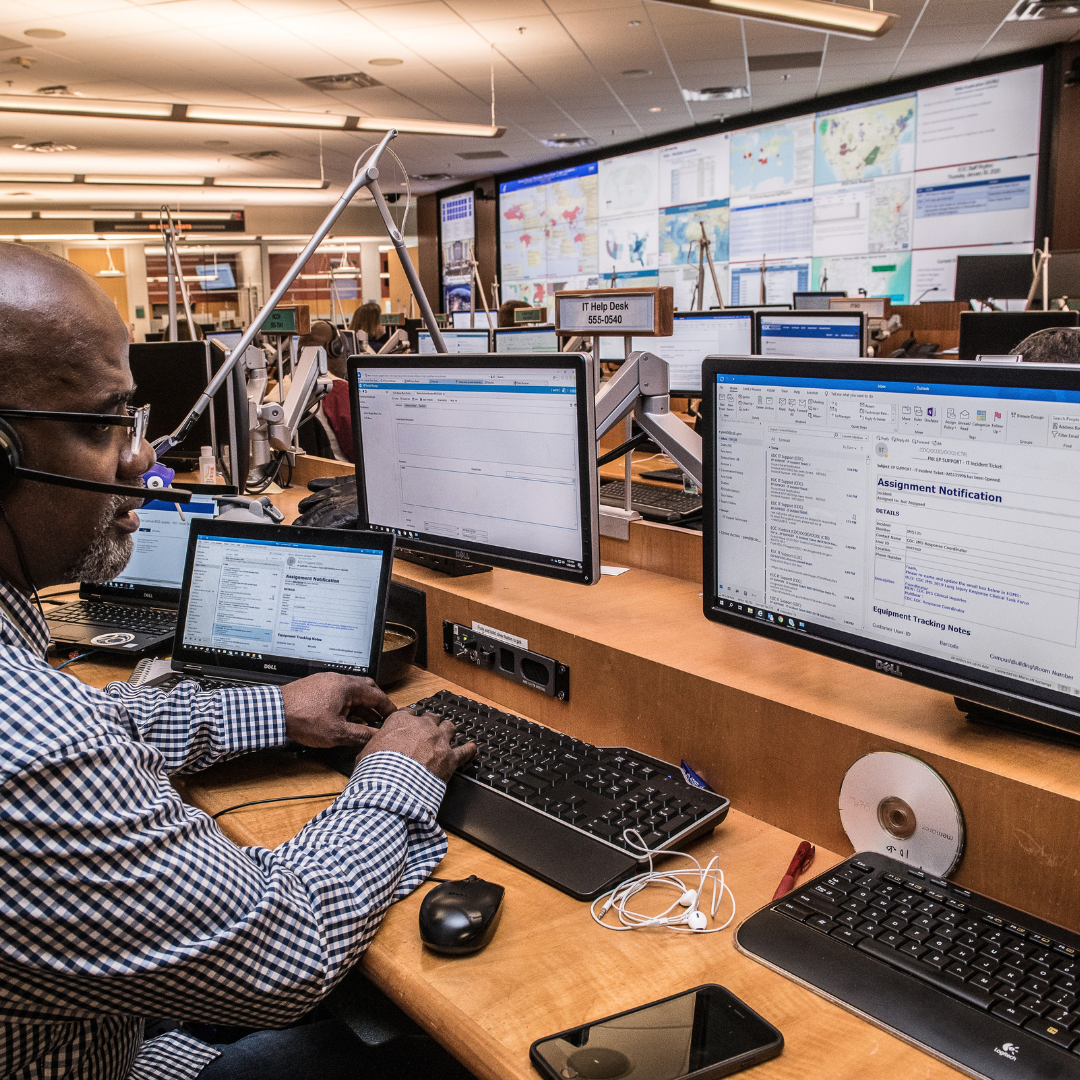
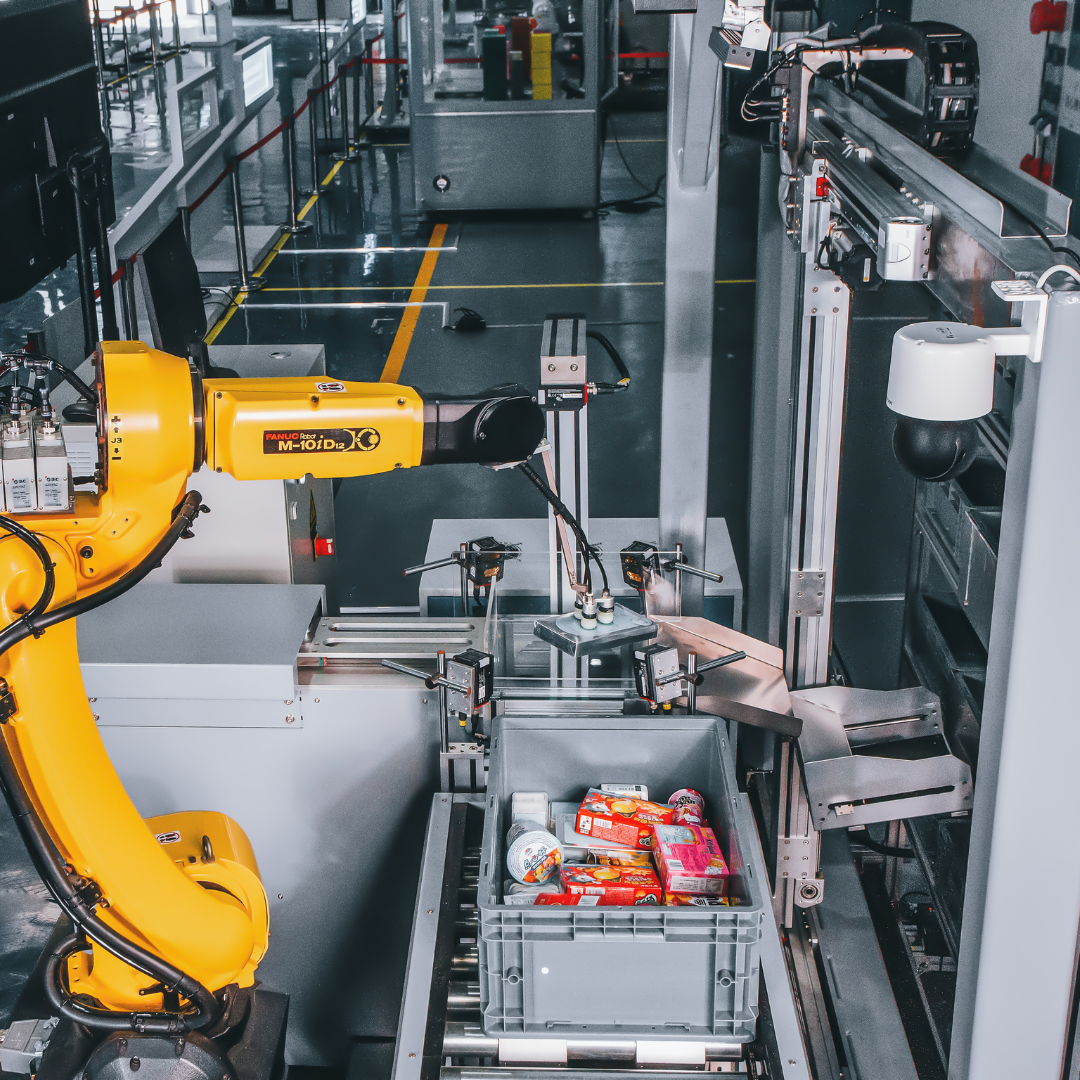
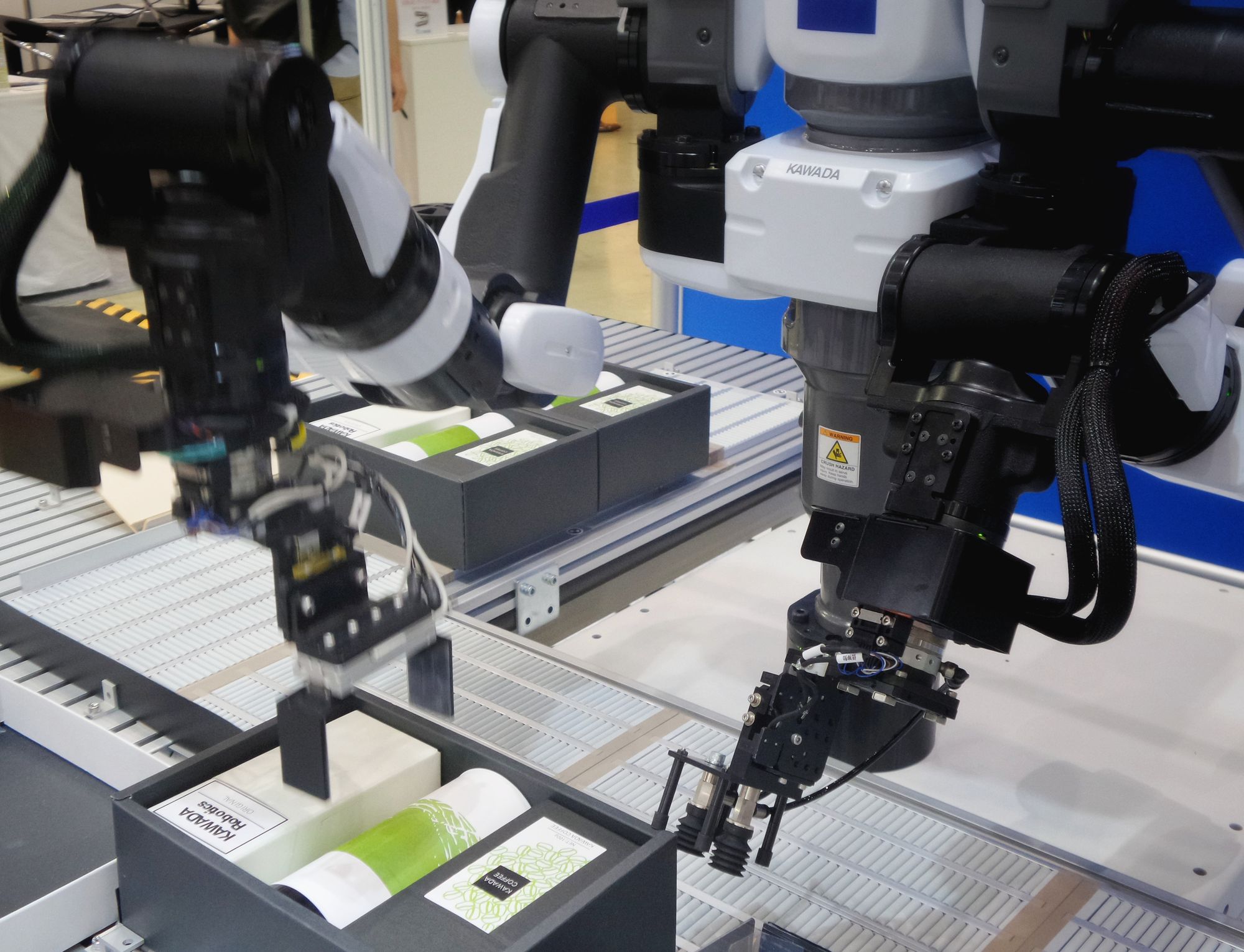