The manufacturing industry is undergoing a significant transformation due to technological advancements, shifting market trends, economic fluctuations, globalization, and regulatory changes.
In this rapidly evolving landscape, manufacturers face the challenge of forecasting demand accurately to align their production capabilities with customer expectations. The ability to anticipate and respond to changing demand patterns is crucial for maintaining competitiveness, optimizing resources, and ensuring customer satisfaction.
The purpose of this article is to provide strategies for forecasting demand in uncertain manufacturing environments. We will delve into the factors that influence demand in the changing manufacturing landscape. Let’s take a look at the table of content below:
- Evolving Nature of the Manufacturing Industry
- Importance of Accurate Demand Forecasting in a Changing Landscape
- Factors Influencing Demand in the Changing Manufacturing Landscape
- Data-Driven Demand Forecasting Techniques
- Scenario Planning and Sensitivity Analysis
- Customer and Market Intelligence
- Agile Manufacturing and Flexible Operations
- Collaboration and Communication across the Value Chain
- Continuous Learning and Adaptation
- How Can Technology and Digital Tools Support Demand Forecasting in a Changing Manufacturing Landscape?
- Wrapping Up
- How Deskera Can Assist You?
Let's get started!
Evolving Nature of the Manufacturing Industry
The manufacturing industry is undergoing significant transformations due to various factors. Technological advancements, such as automation, robotics, and the Internet of Things (IoT), are revolutionizing production processes and enabling greater efficiency and customization.
Industry 4.0, characterized by the integration of digital technologies and data-driven decision-making, is reshaping the manufacturing landscape.
Moreover, changing consumer preferences and market dynamics are driving manufacturers to adopt more flexible and agile production methods. Customers now demand personalized products, shorter lead times, and sustainable manufacturing practices. As a result, manufacturers are shifting from mass production to smaller batch sizes and lean manufacturing principles.
Globalization has also had a profound impact on the manufacturing industry. Companies are establishing global supply chains and sourcing materials and components from different countries to optimize costs and access new markets. However, this globalization also introduces challenges related to supply chain complexity, geopolitical uncertainties, and regulatory changes.
Furthermore, sustainability and environmental considerations are becoming increasingly important. Manufacturers are under pressure to reduce their carbon footprint, minimize waste, and adopt eco-friendly practices. Sustainable manufacturing and circular economy principles are gaining prominence as the industry seeks to balance economic growth with environmental responsibility.
Overall, the evolving manufacturing landscape is characterized by increased automation, digitalization, customer-centricity, global interconnectivity, and sustainability. Manufacturers must adapt to these changes and develop effective strategies to forecast demand in this dynamic and uncertain environment.
Importance of Accurate Demand Forecasting in a Changing Landscape
Accurate demand forecasting plays a crucial role in the success and profitability of manufacturing companies in a changing landscape. Here are some key reasons highlighting its importance:
Optimal Inventory Management: Accurate demand forecasting enables manufacturers to determine the right level of inventory to meet customer demands while avoiding excess stock or shortages. By aligning production and procurement plans with forecasted demand, manufacturers can optimize inventory levels, minimize carrying costs, and reduce the risk of stockouts or overstocking.
Efficient Production Planning: Demand forecasting provides valuable insights into expected customer demand patterns, allowing manufacturers to plan their production activities more effectively. By accurately predicting demand, manufacturers can adjust production schedules, allocate resources, and optimize capacity utilization. This helps reduce production lead times, streamline operations, and enhance overall efficiency.
Effective Supply Chain Management: Demand forecasting is crucial for supply chain management, as it allows manufacturers to synchronize their entire value chain based on anticipated demand. By sharing accurate forecasts with suppliers, distributors, and logistics partners, manufacturers can ensure timely and cost-effective procurement, production, and delivery processes. This leads to improved supply chain efficiency, reduced costs, and enhanced customer satisfaction.
Cost Optimization: Accurate demand forecasting helps manufacturers optimize costs across various aspects of their operations. By aligning production levels with forecasted demand, manufacturers can avoid unnecessary overtime or underutilization of resources, resulting in cost savings. Additionally, precise demand forecasts enable better negotiation with suppliers, leading to improved pricing agreements and reduced procurement costs.
Enhanced Customer Service: Meeting customer demands on time and in full is crucial for customer satisfaction and loyalty. Accurate demand forecasting allows manufacturers to fulfill customer orders promptly, reduce lead times, and deliver products reliably. By consistently meeting customer expectations, manufacturers can strengthen their brand reputation, increase customer loyalty, and gain a competitive edge.
Strategic Decision Making: Accurate demand forecasting provides manufacturers with valuable insights for strategic decision making. It helps in developing long-term plans, such as capacity expansion, new product introductions, market expansion, and investment decisions. By understanding future demand trends, manufacturers can make informed choices and adapt their strategies to the changing market dynamics, ensuring long-term business sustainability.
In a changing manufacturing landscape marked by technological advancements, shifting consumer preferences, globalization, and sustainability concerns, accurate demand forecasting empowers manufacturers to make proactive and data-driven decisions. It enables them to navigate uncertainties, optimize operations, and deliver value to customers, ultimately driving business growth and profitability.
Factors Influencing Demand in the Changing Manufacturing Landscape
Following, we've discussed several factors that influence demand in the changing manufacturing landscape. Let' learn:
A. Technological advancements and their impact on consumer behavior:
Technological advancements play a significant role in shaping consumer behavior and subsequently influencing demand in the manufacturing landscape. Advancements such as the Internet of Things (IoT), artificial intelligence, automation, and digitalization have transformed the way consumers interact with products and services. Here are some key impacts:
Product Innovation: Technological advancements enable manufacturers to develop innovative products that cater to changing consumer needs and preferences. For example, the rise of smartphones and mobile applications has led to an increased demand for smart devices, wearable technology, and connected homes.
Personalization and Customization: Consumers now expect personalized experiences and products tailored to their specific requirements. Technologies such as 3D printing and advanced data analytics allow manufacturers to offer customization options, leading to increased consumer satisfaction and demand.
Digital Channels and E-commerce: The proliferation of digital channels and e-commerce platforms has transformed the way consumers discover, evaluate, and purchase products. Manufacturers must adapt to these digital trends, optimize their online presence, and provide seamless purchasing experiences to meet consumer expectations.
Shift in Communication and Marketing: Technological advancements have shifted the way manufacturers communicate and market their products. Social media platforms, influencer marketing, and targeted advertising campaigns have become essential for reaching and engaging with consumers and influencing their purchasing decisions.
B. Shifts in Market Trends and Preferences:
Market trends and consumer preferences constantly evolve, driven by various factors such as societal changes, cultural influences, and emerging lifestyle trends. Manufacturers need to stay attuned to these shifts to accurately forecast and meet demand. Here are some key considerations:
Sustainability and Ethical Consumption: Consumers are increasingly conscious of environmental sustainability and ethical considerations. There is a growing demand for eco-friendly and socially responsible products. Manufacturers need to adapt their production processes, sourcing strategies, and product offerings to align with these preferences.
Health and Wellness: The increasing focus on health and wellness has led to rising demand for organic, natural, and functional products. Manufacturers need to respond by developing healthier alternatives, incorporating sustainable ingredients, and providing transparent labeling.
Convenience and On-Demand Services: Time-constrained lifestyles have fueled the demand for convenience and on-demand services. Manufacturers should explore options such as subscription-based models, fast delivery services, and hassle-free customer experiences to cater to these trends.
Experience-driven Consumption: Consumers are seeking experiences and emotional connections with products. Manufacturers should consider incorporating elements such as augmented reality, gamification, and interactive features to enhance the overall consumer experience and differentiate their offerings.
C. Economic factors and their effect on consumer purchasing power:
Economic factors significantly impact consumer purchasing power and, consequently, demand in the manufacturing industry. Fluctuations in the economy, income levels, and employment rates can influence consumer behavior. Here are some key economic factors to consider:
Disposable Income and Consumer Confidence: The disposable income available to consumers and their confidence in the economy affect their willingness and ability to make purchases. Manufacturers should monitor economic indicators, such as GDP growth, inflation rates, and unemployment levels, to anticipate changes in consumer spending patterns.
Price Sensitivity: Economic factors can impact consumer price sensitivity. During periods of economic uncertainty or recessions, consumers may become more price-conscious and opt for value-oriented products. Manufacturers should consider pricing strategies, promotions, and competitive positioning to capture demand in different economic scenarios.
Exchange Rates and International Markets: Exchange rate fluctuations can affect import costs, export competitiveness, and overall demand. Manufacturers engaged in international trade need to assess currency risks and adjust pricing strategies accordingly. Additionally, global economic trends and emerging markets can present opportunities or challenges for manufacturers seeking to expand their customer base.
D. Globalization and its impact on supply chain dynamics:
Globalization has profoundly influenced supply chain dynamics and, consequently, demand in the manufacturing industry. Manufacturers need to adapt to the opportunities and challenges presented by global markets. Key impacts include:
Increased Market Access: Globalization has expanded market reach, allowing manufacturers to access a broader customer base. It has opened up new growth opportunities and increased demand for products in previously untapped markets.
Supply Chain Complexity: Globalization has led to more complex and interconnected supply chains. Manufacturers must navigate logistical challenges, diverse regulatory requirements, and geopolitical risks to ensure a smooth flow of materials, components, and finished goods.
Outsourcing and Offshoring: Manufacturers often outsource production or source materials from different regions to optimize costs and access specialized expertise. This practice introduces dependencies on suppliers and requires effective demand forecasting to align production and procurement activities.
Competitive Pressure: Globalization has intensified competition in the manufacturing industry. Manufacturers need to continually innovate, differentiate their products, and enhance customer value propositions to maintain market share and meet evolving customer demands.
E. Regulatory changes and their influence on product demand:
Regulatory changes can significantly impact product demand in the manufacturing industry. Shifts in regulations related to safety standards, environmental compliance, trade policies, and consumer protection can affect market dynamics. Consider the following aspects:
Environmental Regulations: Increasing environmental concerns and regulations aimed at reducing carbon emissions and promoting sustainability can impact demand for certain products. Manufacturers need to assess the environmental impact of their products and align with regulations to meet changing consumer expectations.
Safety and Quality Standards: Regulatory changes in safety and quality standards can influence consumer confidence and demand for products. Compliance with evolving standards and certifications is crucial for manufacturers to ensure product acceptance and mitigate risks.
Trade Policies and Tariffs: Changes in trade policies, such as tariffs, import/export regulations, or trade agreements, can disrupt supply chains and affect demand. Manufacturers should closely monitor trade developments and evaluate the potential impact on production costs and market access.
Consumer Protection and Data Privacy: Regulations related to consumer protection and data privacy can impact consumer trust and preferences. Manufacturers should ensure compliance with relevant data protection laws and demonstrate transparency in handling consumer information.
By understanding and effectively responding to these factors influencing demand in the changing manufacturing landscape, manufacturers can adapt their strategies, anticipate market shifts, and make informed decisions to forecast and meet customer demands successfully.
Data-Driven Demand Forecasting Techniques
Following, we've discussed crucial data-driven demand forecasting techniques. Let's learn:
A. Historical Data Analysis and Trend Identification:
Historical data analysis is a fundamental technique for demand forecasting. It involves analyzing past sales data, market trends, and other relevant historical information to identify patterns and trends. This technique helps manufacturers understand the historical demand patterns of their products and make informed forecasts. Key steps in this process include:
Data Collection: Gather historical sales data, market data, and any other relevant information available from internal sources, such as sales records, customer databases, or ERP systems.
Data Cleaning and Preparation: Clean the data by removing outliers, correcting errors, and filling in missing values. Transform the data into a consistent format suitable for analysis.
Trend Identification: Use statistical techniques, such as moving averages or exponential smoothing, to identify underlying trends in the historical data. This helps to understand the overall direction and magnitude of demand changes over time.
Seasonality Analysis: Identify and analyze seasonal patterns in the data. Seasonality refers to regular, recurring patterns in demand that occur at specific times of the year or within shorter time periods, such as weekly or monthly cycles.
Cyclical Analysis: Explore longer-term cycles or fluctuations in the data that may be influenced by factors such as economic cycles, industry trends, or product life cycles.
Decomposition: Decompose the time series data into its various components, such as trend, seasonality, and irregularities (random variations), using techniques like seasonal decomposition of time series (STL) or X-12-ARIMA.
By analyzing historical data and identifying trends, manufacturers can gain valuable insights into demand patterns, seasonality, and cyclical variations, providing a basis for more accurate demand forecasting.
B. Statistical forecasting models (e.g., time series analysis, regression analysis):
Statistical forecasting models utilize statistical techniques to analyze historical data and make future demand predictions. These models are based on the assumption that historical patterns and relationships will continue into the future. Common statistical forecasting models include:
Time Series Analysis: Time series analysis involves analyzing and modeling data collected over time to forecast future demand. Techniques such as moving averages, exponential smoothing, autoregressive integrated moving average (ARIMA), or state space models can be applied to capture the patterns and dependencies within the time series data.
Regression Analysis: Regression analysis establishes relationships between the dependent variable (demand) and independent variables (such as price, promotion, or economic factors) to forecast future demand. Multiple linear regression, nonlinear regression, or logistic regression can be used to identify and quantify the impact of various factors on demand.
Seasonal Decomposition of Time Series: This technique decomposes the time series data into its different components (trend, seasonality, and irregularities) using statistical methods. It helps isolate and analyze the seasonal patterns and trends separately, providing more accurate demand forecasts.
Statistical forecasting models are widely used and provide a solid foundation for demand forecasting. They can be applied to both short-term and long-term forecasting, depending on the nature of the data and the forecasting requirements.
C. Machine Learning and Artificial Intelligence for Demand Forecasting:
Machine learning (ML) and artificial intelligence (AI) techniques have gained popularity for demand forecasting due to their ability to handle complex data sets and capture non-linear relationships.
Furthermore, ML and AI models can adapt and improve over time as they learn from new data. Some commonly used ML and AI techniques for demand forecasting include:
Neural Networks: Neural networks are models inspired by the human brain's functioning. They learn to recognize patterns and relationships within data, making them suitable for demand forecasting. Deep learning techniques, such as recurrent neural networks (RNNs) or long short-term memory (LSTM) networks, can capture sequential dependencies and handle time series data effectively.
Random Forests: Random forest models are an ensemble learning technique that combines multiple decision trees to make predictions. They can handle complex data sets with a large number of variables and capture non-linear relationships.
Gradient Boosting: Gradient boosting algorithms, such as XGBoost or LightGBM, sequentially build a predictive model by combining weak predictive models. They are known for their ability to handle large data sets, feature interactions, and nonlinearity.
Support Vector Machines (SVM): SVM is a supervised learning technique that analyzes data and separates it into distinct classes. It can be applied to demand forecasting by classifying demand patterns and predicting future demand based on historical data.
Machine learning and AI techniques offer the advantage of flexibility, scalability, and the ability to handle large and complex data sets. They can capture intricate patterns and relationships, leading to more accurate demand forecasts.
D. Predictive Analytics and Demand Sensing Techniques:
Predictive analytics combines historical and real-time data to make forecasts and predictions. It leverages advanced analytics techniques and algorithms to identify patterns and relationships that can impact future demand. Demand sensing techniques focus on capturing real-time data signals to adjust demand forecasts dynamically. Some key techniques in this category include:
Demand Sensing: Demand sensing techniques utilize real-time data sources, such as point-of-sale data, social media sentiment analysis, or sensor data, to capture current demand signals. By integrating these signals into the forecasting process, manufacturers can adjust demand forecasts rapidly and accurately in response to changing market conditions.
Predictive Modeling: Predictive modeling techniques use historical and real-time data to create models that predict future demand based on various factors and scenarios. These models can incorporate statistical techniques, machine learning algorithms, and domain knowledge to capture complex demand patterns and make accurate predictions.
Predictive Analytics Platforms: Predictive analytics platforms provide tools and frameworks to analyze historical and real-time data, build models, and generate demand forecasts. These platforms often include features such as data visualization, advanced analytics algorithms, and scenario analysis capabilities.
Predictive analytics and demand sensing techniques enable manufacturers to leverage both historical and real-time data to improve the accuracy of demand forecasts. By incorporating real-time signals into the forecasting process, manufacturers can respond more effectively to market dynamics and changing customer demands.
E. Collaborative forecasting with supply chain partners:
Collaborative forecasting involves sharing demand-related information and collaborating with supply chain partners to improve the accuracy of demand forecasts. By integrating the perspectives and insights of key stakeholders, manufacturers can enhance the forecasting process.
Key aspects of collaborative forecasting include:
Demand Data Sharing: Collaborative forecasting requires sharing demand-related data with supply chain partners, such as retailers, distributors, or suppliers. This data includes point-of-sale data, inventory levels, promotional plans, and market intelligence. Collaborative platforms or systems can facilitate secure data sharing and collaboration.
Joint Forecasting and Planning: Engage in joint forecasting and planning exercises with supply chain partners to align expectations and insights. Collaborative workshops, meetings, or virtual collaboration platforms enable stakeholders to share their perspectives, insights, and market knowledge to improve the accuracy of demand forecasts.
Demand Collaboration Tools: Utilize demand collaboration tools or software that enable real-time data sharing, scenario analysis, and collaborative forecasting capabilities. These tools facilitate effective communication, information exchange, and consensus building among supply chain partners.
Collaborative forecasting enhances demand forecasting accuracy by incorporating multiple perspectives, market insights, and domain knowledge. It promotes better alignment between supply and demand, reduces information asymmetry, and enables proactive decision-making across the supply chain.
By leveraging these data-driven demand forecasting techniques, manufacturers can improve the accuracy and reliability of their demand forecasts. The combination of historical data analysis, statistical models, machine learning, predictive analytics, and collaborative forecasting empowers manufacturers to navigate uncertainties and make informed decisions to meet customer demands effectively.
Scenario Planning and Sensitivity Analysis
Following, we've discussed scenario planning and sensitivity analysis. Let's learn:
A. Developing Multiple Demand Scenarios based on Different Assumptions:
Scenario planning involves creating multiple hypothetical situations or scenarios based on different assumptions about the future. In the context of demand forecasting, developing multiple demand scenarios helps manufacturers consider various potential outcomes and their corresponding impacts on demand. Here's how it can be done:
Identify Key Drivers: Identify the key factors or variables that significantly influence demand, such as economic conditions, market trends, consumer behavior, or regulatory changes. These drivers should be relevant to the specific industry and products being forecasted.
Define Assumptions: Develop different assumptions or narratives for each key driver. For example, in the case of economic conditions, assumptions could include a pessimistic scenario (economic recession), a moderate scenario (steady growth), and an optimistic scenario (economic boom).
Quantify Scenarios: Translate the assumptions into quantitative estimates or ranges for each scenario. This could involve assigning growth rates, market shares, or other relevant metrics to each scenario.
Create Scenarios: Combine the different assumptions for each driver to create multiple demand scenarios. For example, by combining the pessimistic economic scenario with a market trend assumption, a scenario could reflect a decline in demand due to a recession and changing consumer preferences.
By developing multiple demand scenarios, manufacturers can assess a range of potential outcomes, understand the risks and opportunities associated with each scenario, and make more robust and adaptive business plans.
B. Assessing the Sensitivity of Demand Forecasts to Key Variables:
Sensitivity analysis helps manufacturers understand how changes in key variables or assumptions affect demand forecasts. It allows for a quantitative assessment of the impact of different factors on the forecasted demand. Here's how to conduct sensitivity analysis:
Identify Key Variables: Identify the key variables or assumptions that are most critical to the demand forecast. These variables could include factors such as price, promotional activities, economic indicators, or competitor actions.
Define Range of Values: Determine a range of values for each key variable, representing different possible scenarios or changes. For example, you can vary the price by ±10%, assume a 5% increase or decrease in promotional activities, or consider different economic growth rates.
Analyze Impact: Modify the values of the key variables within their defined ranges and assess the resulting impact on the demand forecast. This can be done using statistical models, simulations, or scenario analysis tools.
Interpret Results: Analyze and interpret the sensitivity analysis results. Identify which variables have the most significant impact on the demand forecast and understand how changes in these variables can influence demand levels and patterns.
By conducting sensitivity analysis, manufacturers can identify the most influential variables, quantify their impact on demand, and gain insights into the potential risks and uncertainties associated with different scenarios.
C. Incorporating Risk Analysis and Contingency Planning:
Risk analysis involves identifying and assessing potential risks and uncertainties that may impact demand forecasts. Contingency planning, on the other hand, focuses on developing strategies and actions to mitigate risks and respond to unexpected events.
Here's how manufacturers can incorporate risk analysis and contingency planning into demand forecasting:
Identify Risks: Identify potential risks and uncertainties that could affect demand, such as supply chain disruptions, natural disasters, regulatory changes, or competitor actions. Consider both internal and external factors that may pose risks to the forecast.
Assess Probability and Impact: Evaluate the probability and potential impact of each identified risk on demand. This assessment can be subjective or based on historical data, expert opinions, or risk modeling techniques.
Develop Contingency Plans: Based on the identified risks and their potential impact, develop contingency plans to mitigate the risks and respond effectively. Contingency plans may include alternative sourcing options, inventory management strategies, diversification of product offerings, or agile production capabilities.
Monitor and Review: Continuously monitor the identified risks, market conditions, and relevant indicators. Regularly review and update the contingency plans to ensure their relevance and effectiveness in mitigating risks and adapting to changing circumstances.
By incorporating risk analysis and contingency planning, manufacturers can proactively address potential disruptions, minimize the negative impact on demand forecasts, and enhance their ability to respond swiftly and effectively to unforeseen events.
Overall, scenario planning, sensitivity analysis, and risk analysis with contingency planning provide manufacturers with a comprehensive framework to assess and prepare for different future scenarios, understand the potential impact of key variables on demand, and mitigate risks associated with demand forecasting in a changing manufacturing landscape.
Customer and Market Intelligence
Following, we've discussed customer and market intelligence. Let's learn in detail:
A. Conducting Market Research and Consumer Surveys:
Market research and consumer surveys are essential tools for gathering customer and market intelligence. They provide valuable insights into customer preferences, buying behavior, market trends, and competitor analysis. Here's how to effectively leverage market research and consumer surveys:
Define Research Objectives: Clearly define the objectives of the market research or consumer survey. Determine what specific information or insights you are seeking to gather, such as understanding customer needs, assessing product satisfaction, or identifying market trends.
Design the Study: Develop a research methodology that aligns with your objectives. This may include designing surveys, conducting focus groups, or utilizing qualitative or quantitative research techniques. Consider the target audience, sample size, data collection methods, and analysis techniques.
Data Collection: Implement the research methodology by collecting data from the target audience. This could involve administering surveys, conducting interviews, or using online research platforms. Ensure that the data collection process is structured, unbiased, and representative of the target market.
Data Analysis: Analyze the collected data to extract meaningful insights. This may involve quantitative analysis, such as statistical techniques or data mining, to identify patterns and trends. Qualitative analysis, such as thematic analysis or sentiment analysis, can provide deeper insights into customer opinions and preferences.
Interpretation and Action: Interpret the findings of the market research or consumer survey and translate them into actionable strategies. Use the insights to inform product development, marketing campaigns, pricing strategies, or customer engagement initiatives.
Market research and consumer surveys provide a direct understanding of customer needs, preferences, and market dynamics, enabling manufacturers to make informed decisions and tailor their strategies to meet customer demands effectively.
B. Leveraging Social Media and Online Analytics for Customer Insights:
Social media and online analytics offer a wealth of customer insights and real-time market intelligence. By monitoring social media platforms, online forums, and review sites, manufacturers can gather valuable information about customer sentiment, preferences, and emerging trends.
Here's how to leverage social media and online analytics effectively:
Social Listening: Monitor social media platforms, online forums, and review sites to understand customer conversations, opinions, and feedback related to your products or industry. Use social listening tools to track keywords, hashtags, or mentions relevant to your business.
Sentiment Analysis: Analyze social media and online content to gauge customer sentiment towards your brand, products, or competitors. Sentiment analysis techniques can help identify positive, negative, or neutral sentiments expressed by customers, providing insights into customer satisfaction and perception.
Trend Identification: Identify emerging trends, topics, or discussions within your industry by monitoring social media and online platforms. This can help you stay abreast of evolving customer preferences, new product demands, or emerging market opportunities.
Competitive Analysis: Track competitor activities and strategies through social media and online channels. Monitor their product launches, marketing campaigns, customer interactions, and customer feedback. This helps identify competitive advantages, potential gaps in the market, and areas for differentiation.
Online Surveys and Feedback: Use online surveys, polls, or feedback forms to gather direct input from customers. Leverage online platforms or email campaigns to reach a wider audience and collect valuable insights on customer preferences, satisfaction levels, or purchase behavior.
By leveraging social media and online analytics, manufacturers can gain real-time insights into customer perceptions, preferences, and market trends. This enables them to adapt quickly to changing customer needs and develop targeted strategies to engage and retain customers effectively.
C. Collaborating with Sales Teams and Channel Partners for Market Intelligence:
Sales teams and channel partners serve as valuable sources of market intelligence due to their direct interactions with customers and their knowledge of the sales landscape. By collaborating with them, manufacturers can gain valuable insights into customer needs, competitor activities, and market dynamics.
Here's how to effectively collaborate with sales teams and channel partners for market intelligence:
Regular Communication: Establish regular communication channels with sales teams and channel partners to exchange information and gather market intelligence. This can include weekly or monthly meetings, email updates, or online collaboration platforms.
Field Insights: Encourage sales teams and channel partners to share their field insights, including customer feedback, market trends, competitor activities, and emerging opportunities. Provide a structured framework or reporting format to capture and organize this information effectively.
Customer Feedback Loop: Establish a feedback loop between sales teams, channel partners, and other customer-facing personnel. Encourage them to collect and share customer feedback regarding product preferences, buying behavior, satisfaction levels, or unmet needs. This feedback can be invaluable in shaping future product development and marketing strategies.
Joint Market Research: Collaborate with sales teams and channel partners to conduct joint market research initiatives. This can include co-funded studies, customer surveys, or focus groups that involve both the manufacturer and the sales team/channel partner. This collaborative approach ensures that insights from the field are incorporated into the research process.
Training and Enablement: Provide training and enablement programs to sales teams and channel partners to enhance their market intelligence capabilities. This can include educating them about market research techniques, competitor analysis, or customer profiling. The better equipped they are, the more valuable their insights will be.
By collaborating with sales teams and channel partners, manufacturers can tap into their collective knowledge and experience, gaining valuable market intelligence from the front lines. This enables manufacturers to align their strategies with market realities, enhance customer satisfaction, and seize new business opportunities.
D. Monitoring Competitor Strategies and Industry Trends:
Keeping a close eye on competitor strategies and industry trends is crucial for understanding market dynamics and identifying potential opportunities or threats. Here's how to effectively monitor competitor strategies and industry trends:
Competitor Analysis: Conduct a thorough analysis of competitors, including their product offerings, pricing strategies, marketing campaigns, distribution channels, and customer engagement initiatives. Use a combination of primary research (such as gathering information from sales teams or channel partners) and secondary research (such as industry reports or news articles) to gather data.
Industry News and Publications: Stay updated with industry news, publications, and trade journals that provide insights into the latest trends, innovations, and market developments. This can help identify emerging technologies, changing consumer preferences, or regulatory changes that may impact demand.
Attend Industry Events and Conferences: Participate in industry events, conferences, and trade shows to network with peers, industry experts, and thought leaders. These events provide opportunities to gain insights into industry trends, hear about best practices, and engage in discussions that help shape market intelligence.
Online Monitoring: Utilize online tools, such as industry-specific websites, blogs, or newsletters, to monitor and track industry trends, competitor activities, and customer discussions. Set up alerts or subscribe to relevant online platforms to receive timely updates.
Competitive Intelligence Tools: Leverage competitive intelligence tools or software that provide automated monitoring and analysis of competitor activities. These tools can track competitor websites, social media accounts, advertising campaigns, or pricing changes, providing real-time insights.
By actively monitoring competitor strategies and industry trends, manufacturers can anticipate market shifts, identify competitive advantages, and align their own strategies accordingly. This proactive approach ensures that manufacturers stay ahead of the curve and make informed decisions to meet evolving customer demands.
Incorporating customer and market intelligence from various sources, including market research, social media analytics, collaboration with sales teams, and competitor analysis, empowers manufacturers to make data-driven decisions, respond effectively to customer needs, and stay competitive in the evolving manufacturing landscape.
Agile Manufacturing and Flexible Operations
Following, we've discussed agile manufacturing and flexible operations. Let's learn:
A. Implementing Agile Manufacturing Practices to Respond to Changing Demand:
Agile manufacturing refers to the ability of a manufacturing operation to quickly and effectively respond to changing customer demands, market dynamics, and unforeseen disruptions. It involves the adoption of flexible processes, technologies, and organizational structures. Here's how manufacturers can implement agile manufacturing practices:
Cross-Functional Teams: Establish cross-functional teams that bring together employees from different departments, such as production, engineering, sales, and marketing. These teams collaborate closely to enhance communication, share information, and make rapid decisions to adapt to changing demands.
Modular Production Systems: Implement modular production systems that allow for easy reconfiguration and flexibility in manufacturing processes. Modular systems enable manufacturers to quickly adjust production lines, accommodate changes in product designs or specifications, and optimize production efficiency.
Rapid Prototyping and Iterative Design: Adopt rapid prototyping techniques, such as 3D printing or computer-aided design (CAD), to accelerate the product development process. This enables manufacturers to quickly test and iterate product designs based on customer feedback, market trends, or changing requirements.
Demand-Driven Inventory Management: Implement demand-driven inventory management practices, such as just-in-time (JIT) or lean manufacturing, to minimize inventory levels while ensuring the timely availability of products. This approach reduces inventory holding costs, improves cash flow, and allows manufacturers to respond swiftly to fluctuating demand.
Collaborative Supply Chain Partnerships: Foster collaborative partnerships with suppliers, distributors, and logistics providers to create a responsive supply chain network. By sharing information, aligning production schedules, and implementing vendor-managed inventory (VMI) or consignment stock agreements, manufacturers can enhance their ability to quickly adjust production levels and meet changing demand.
Implementing agile manufacturing practices enables manufacturers to be more responsive, adaptive, and customer-centric. It allows them to quickly introduce new products, adjusts production volumes, and optimize operations to align with changing market conditions and customer requirements.
B. Investing in Flexible Production Systems and Supply Chain Capabilities:
Investing in flexible production systems and supply chain capabilities is essential for manufacturers to meet the challenges of a changing manufacturing landscape. Flexibility in production and supply chain operations allows manufacturers to quickly adapt to fluctuating demand, changing product requirements, and unforeseen disruptions. Here are key aspects to consider:
Modular and Scalable Production Equipment: Invest in modular and scalable production equipment that can be easily reconfigured or expanded to accommodate changing product specifications or production volumes. This flexibility enables manufacturers to ramp up or down production levels efficiently.
Multi-Purpose Manufacturing Facilities: Design manufacturing facilities with the flexibility to produce multiple product lines or adapt to changing production processes. This versatility allows manufacturers to switch production quickly and efficiently to meet shifting market demands.
Agile Supply Chain Network: Develop an agile supply chain network that can respond to changes in demand patterns and customer preferences. This may involve diversifying suppliers, establishing redundancy in critical components or materials, and implementing agile logistics strategies.
Supplier Collaboration and Integration: Foster close collaboration and integration with key suppliers to ensure a seamless flow of materials, components, and information. This collaboration enables manufacturers to quickly adjust procurement and production plans based on changing demand signals or supply disruptions.
Digitalization and Automation: Invest in digitalization and automation technologies to improve operational efficiency, reduce lead times, and enhance flexibility in production and supply chain processes. Technologies such as robotics, IoT sensors, and real-time data analytics enable manufacturers to monitor and optimize operations in real-time, identify bottlenecks, and make data-driven decisions.
By investing in flexible production systems and supply chain capabilities, manufacturers can effectively navigate changing demand patterns, mitigate supply chain risks, and maintain operational resilience in the face of uncertainty.
C. Leveraging Predictive Maintenance and Real-Time Data Analytics:
Predictive maintenance and real-time data analytics play a crucial role in ensuring efficient operations, minimizing downtime, and maximizing equipment utilization. By harnessing the power of data and analytics, manufacturers can proactively identify maintenance needs, optimize equipment performance, and respond quickly to potential disruptions.
Here's how to leverage these technologies effectively:
Condition Monitoring: Implement condition monitoring systems that utilize sensors and data analytics to continuously monitor the health and performance of critical equipment. These systems can detect anomalies, predict maintenance requirements, and enable proactive maintenance scheduling.
Predictive Analytics: Utilize predictive analytics algorithms and machine learning models to analyze historical and real-time data and forecast equipment failures or maintenance needs. This allows manufacturers to optimize maintenance schedules, minimize unplanned downtime, and reduce maintenance costs.
Real-time Data Integration: Integrate data from various sources, such as equipment sensors, production systems, and maintenance records, into a centralized data platform. This enables real-time monitoring, analysis, and visualization of key performance indicators, equipment health, and maintenance requirements.
Remote Monitoring and Diagnostics: Implement remote monitoring capabilities that allow maintenance teams to access real-time equipment data, conduct diagnostics, and perform predictive maintenance tasks remotely. This reduces the need for physical presence on the shop floor and enables quicker response times.
Continuous Improvement: Leverage data analytics insights to drive continuous improvement initiatives in maintenance practices. Analyze failure patterns, identify root causes of equipment breakdowns, and implement corrective actions to optimize equipment reliability and performance.
By leveraging predictive maintenance and real-time data analytics, manufacturers can enhance equipment uptime, optimize maintenance schedules, reduce costs, and improve overall operational efficiency. These technologies enable manufacturers to anticipate and respond to maintenance needs proactively, minimize production disruptions, and improve customer satisfaction.
Incorporating agile manufacturing practices, investing in flexible production systems and supply chain capabilities, and leveraging predictive maintenance and real-time data analytics empower manufacturers to navigate uncertainties in the changing manufacturing landscape. These strategies enable manufacturers to respond swiftly to changing demand, optimize operations, and maintain a competitive edge in the market.
Collaboration and Communication across the Value Chain
Following, we've discussed collaboration and communication across the value chain. Let's learn:
A. Strengthening Relationships with Suppliers for Better Demand Visibility:
Strong relationships with suppliers are crucial for manufacturers to gain better visibility into demand patterns and fluctuations. By collaborating closely with suppliers, manufacturers can gather valuable insights and ensure a smooth flow of information throughout the value chain. Here's how to strengthen relationships with suppliers for better demand visibility:
Transparent Communication: Establish open and transparent communication channels with suppliers. Regularly share relevant information such as sales forecasts, new product launches, or changes in customer demand. This helps suppliers align their production and inventory levels to meet the manufacturer's requirements.
Collaborative Demand Planning: Engage suppliers in collaborative demand planning processes. Share market intelligence, customer insights, and sales data to collectively forecast demand. This collaborative approach enhances accuracy in demand forecasting and reduces the risk of supply-demand mismatches.
Supplier Scorecards and Performance Metrics: Implement supplier scorecards and performance metrics to track and evaluate supplier performance. These metrics can include on-time delivery, product quality, responsiveness, and collaboration. Regularly review supplier performance and provide feedback for continuous improvement.
Joint Sales and Operations Planning (S&OP): Conduct joint Sales and Operations Planning meetings with suppliers to align production capacities, inventory levels, and lead times. This collaborative process allows manufacturers and suppliers to anticipate demand fluctuations, adjust production schedules, and optimize inventory levels.
Data Sharing and Integration: Share relevant data with suppliers, such as point-of-sale (POS) data, inventory levels, or production schedules. Explore opportunities to integrate data systems and technologies to enable real-time visibility and collaboration in supply chain operations.
By strengthening relationships with suppliers and improving demand visibility, manufacturers can enhance supply chain responsiveness, reduce lead times, and effectively manage demand fluctuations.
B. Collaborating with distributors and retailers for accurate demand forecasting:
Collaboration with distributors and retailers is essential for manufacturers to accurately forecast demand and align production and distribution plans. By working closely with these partners, manufacturers can gather market insights, understand customer preferences, and improve demand forecasting accuracy.
Here's how to collaborate effectively with distributors and retailers for accurate demand forecasting:
Regular Communication: Maintain regular communication channels with distributors and retailers. Stay informed about market trends, customer feedback, and changes in consumer preferences. This information provides valuable inputs for demand forecasting.
Joint Business Planning: Conduct joint business planning sessions with distributors and retailers. Share sales forecasts, promotional plans, and marketing strategies. Collaboratively analyze historical data and market trends to improve demand forecasting accuracy.
Point-of-Sale (POS) Data Sharing: Collaborate with distributors and retailers to share POS data. Furthermore, POS data provides real-time insights into product sales, customer buying behavior, and demand patterns. Analyze this data to identify demand trends, seasonality, or regional variations.
Collaborative Forecasting: Implement collaborative forecasting processes with distributors and retailers. This involves gathering inputs from all stakeholders, consolidating demand forecasts, and reconciling any differences or biases. By incorporating diverse perspectives, collaborative forecasting improves the accuracy of demand predictions.
Incentives and Rewards: Provide incentives or rewards to distributors and retailers for sharing accurate sales and demand data. This encourages active participation, data transparency, and a collaborative approach to demand forecasting.
By collaborating closely with distributors and retailers, manufacturers can gain insights into customer preferences, accurately forecast demand, optimize production levels, and enhance customer satisfaction.
C. Sharing data and insights with all stakeholders for improved decision-making:
Effective sharing of data and insights with all stakeholders across the value chain is vital for making informed decisions and driving collaborative decision-making processes. By providing relevant information to all parties, manufacturers can align strategies, improve operational efficiency, and respond swiftly to changing market dynamics. Here's how to share data and insights with stakeholders for improved decision-making:
Centralized Data Repository: Establish a centralized data repository or platform where relevant data from various sources can be stored, accessed, and shared securely. This repository serves as a single source of truth, ensuring that stakeholders have access to accurate and up-to-date information.
Data Visualization and Reporting: Use data visualization tools and reporting dashboards to present key insights and metrics in a clear and easily understandable format. Visual representations of data facilitate better decision-making by enabling stakeholders to identify trends, patterns, and anomalies quickly.
Collaborative Platforms: Implement collaborative platforms or software solutions that enable real-time sharing and collaboration among stakeholders. These platforms allow for seamless communication, data sharing, and document collaboration, fostering a collaborative decision-making culture.
Regular Meetings and Reviews: Conduct regular meetings, reviews, or workshops with stakeholders to share data insights, discuss performance metrics, and address challenges or opportunities. These interactions facilitate cross-functional collaboration, alignment of goals, and collective decision-making.
Training and Education: Provide training and education programs to stakeholders on data literacy, interpretation, and analysis. Enhancing stakeholders' data literacy empowers them to understand and leverage the shared data and insights for effective decision-making.
Data Security and Privacy: Ensure that appropriate data security and privacy measures are in place to protect sensitive information. Implement access controls, encryption, and other security protocols to safeguard data shared with stakeholders.
By sharing data and insights with all stakeholders, manufacturers can foster a culture of collaboration, transparency, and data-driven decision-making. This collaborative approach enhances the overall decision-making process, reduces information gaps, and enables stakeholders to make informed decisions that align with the overall business objectives.
In conclusion, collaboration and communication across the value chain are critical for manufacturers to navigate the changing manufacturing landscape successfully. Strengthening relationships with suppliers, collaborating with distributors and retailers, and sharing data and insights with stakeholders empower manufacturers to improve demand visibility, enhance forecast accuracy, and make informed decisions that drive operational efficiency and customer satisfaction.
Continuous Learning and Adaptation
Following, we've discussed continuous learning and adaptation. Let's learn:
A. Monitoring and Evaluating Demand Forecasting Accuracy:
Monitoring and evaluating demand forecasting accuracy is essential for manufacturers to assess the effectiveness of their forecasting processes and identify areas for improvement. By regularly tracking forecasted demand against actual demand, manufacturers can identify patterns, trends, and deviations, enabling them to refine their forecasting models and techniques. Here's how to effectively monitor and evaluate demand forecasting accuracy:
Define Key Performance Indicators (KPIs): Establish relevant KPIs to measure demand forecasting accuracy, such as forecast error, mean absolute percentage error (MAPE), or bias. These KPIs provide quantitative metrics to assess the accuracy of forecasts.
Regular Forecast Comparison: Conduct regular comparisons between forecasted demand and actual demand. This can be done on a weekly, monthly, or quarterly basis, depending on the product and industry. Analyze the variances and identify the causes of any significant discrepancies.
Root Cause Analysis: Perform root cause analysis to understand the factors contributing to forecasting inaccuracies. Evaluate the impact of variables such as seasonality, promotions, market trends, or external factors. This analysis helps identify areas for improvement in data collection, forecasting methods, or assumptions.
Cross-Functional Collaboration: Involve cross-functional teams, including sales, marketing, operations, and finance, in the evaluation process. Collaboratively review and analyze forecast accuracy to gain diverse perspectives and insights.
Statistical Analysis: Utilize statistical techniques and analytics to assess the accuracy of demand forecasts. Calculate forecast error metrics, generate forecast accuracy reports, and analyze trends over time. This analysis provides actionable insights for continuous improvement.
By monitoring and evaluating demand forecasting accuracy, manufacturers can identify areas of improvement, refine forecasting techniques, and enhance the accuracy of future demand predictions.
B. Incorporating Feedback Loops for Continuous Improvement:
Incorporating feedback loops into the demand forecasting process allows manufacturers to continuously learn from their experiences, gather insights from stakeholders, and refine their forecasting strategies. Feedback loops provide valuable information for course correction, process optimization, and continuous improvement. Here's how to effectively incorporate feedback loops for continuous improvement:
Feedback from Sales and Marketing Teams: Establish channels for sales and marketing teams to provide feedback on demand forecasts. Regularly gather input on market trends, customer behavior, or any changes in customer preferences. This feedback helps refine demand forecasts and improve their accuracy.
Feedback from Customers: Actively seek feedback from customers regarding their buying patterns, preferences, and satisfaction levels. Customer feedback can be collected through surveys, focus groups, or direct interactions. Incorporating customer feedback into demand forecasts ensures alignment with their needs and enhances forecast accuracy.
Collaboration with Supply Chain Partners: Engage in collaborative discussions and feedback exchanges with supply chain partners, such as suppliers, distributors, or retailers. Share demand forecasts and gather their input on market dynamics, inventory levels, or production capacities. This collaborative feedback enhances the accuracy and reliability of forecasts.
Post-Forecast Analysis: Conduct post-forecast analysis to assess the accuracy of forecasts and identify areas for improvement. Analyze the root causes of any significant variances and seek feedback from stakeholders involved in the forecasting process. Use these insights to refine forecasting methodologies and adjust future forecasts.
Continuous Learning Culture: Foster a culture of continuous learning and improvement within the organization. Encourage employees to share insights, lessons learned, and best practices related to demand forecasting. Regularly review and discuss feedback to drive continuous improvement in the forecasting process.
By incorporating feedback loops into the demand forecasting process, manufacturers can gather valuable insights, identify potential blind spots, and make iterative improvements to their forecasting strategies.
C. Learning from Past Forecasting Errors and Adjusting Strategies Accordingly:
Learning from past forecasting errors is crucial for manufacturers to avoid repeating the same mistakes and continuously improve their demand forecasting strategies. By analyzing and understanding the causes of past errors, manufacturers can refine their methodologies, adjust assumptions, and enhance forecasting accuracy. Here's how to effectively learn from past forecasting errors and adjust strategies accordingly:
Root Cause Analysis: Perform a thorough root cause analysis of past forecasting errors. Identify the factors that contributed to the inaccuracies, such as data quality issues, incorrect assumptions, or external market dynamics. This analysis helps pinpoint specific areas for improvement.
Data Quality Enhancement: Assess the quality and reliability of the data used in the forecasting process. Identify any data gaps, inconsistencies, or biases that may have affected the accuracy of past forecasts. Take steps to improve data collection, cleansing, and validation processes.
Forecasting Methodology Evaluation: Evaluate the forecasting methodologies and techniques used in the past. Assess their strengths and limitations in capturing demand patterns and changes. Consider alternative forecasting methods and explore new approaches to improve accuracy.
Adjusting Assumptions and Parameters: Review and adjust the assumptions, parameters, and variables used in the forecasting models. Consider changes in market dynamics, consumer behavior, or industry trends that may have influenced past errors. Incorporate these insights into revised forecasting strategies.
Continuous Improvement Plan: Develop a continuous improvement plan based on the lessons learned from past forecasting errors. Define actionable steps, timelines, and responsibilities for implementing improvements. Monitor the effectiveness of the changes and adjust strategies accordingly.
Collaboration and Knowledge Sharing: Encourage collaboration and knowledge sharing among the forecasting team and relevant stakeholders. Facilitate discussions, workshops, or training sessions to share insights, discuss lessons learned, and brainstorm ideas for improvement.
By learning from past forecasting errors and adjusting strategies accordingly, manufacturers can refine their forecasting models, improve accuracy, and enhance their ability to anticipate and meet customer demand effectively.
In conclusion, continuous learning and adaptation are essential for manufacturers to enhance their demand forecasting capabilities in a changing manufacturing landscape. By monitoring and evaluating demand forecasting accuracy, incorporating feedback loops, and learning from past forecasting errors, manufacturers can continuously improve their forecasting strategies, optimize operations, and maintain a competitive edge in the market.
How Can Technology and Digital Tools Support Demand Forecasting in a Changing Manufacturing Landscape?
Technology and digital tools offer valuable support for demand forecasting in a changing manufacturing landscape. Here's how they can assist:
Advanced analytics and big data processing: Leveraging advanced analytics and big data processing capabilities, manufacturers can analyze large volumes of historical and real-time data to identify patterns, correlations, and demand drivers. These insights help improve forecast accuracy and enable manufacturers to respond promptly to changes in demand.
Forecasting software and automation: Forecasting software equipped with statistical algorithms, machine learning capabilities, and automation features streamline the forecasting process. These tools enable manufacturers to generate forecasts quickly, run simulations, and perform scenario analysis to evaluate different demand scenarios.
Internet of Things (IoT) and real-time data integration: IoT devices and sensors provide real-time data on production levels, inventory status, and customer behavior. Integrating this data into demand forecasting models enhances accuracy and responsiveness to changing demand patterns.
Cloud-based platforms and collaboration tools: Cloud-based platforms facilitate data sharing, collaboration, and real-time updates across the supply chain. Manufacturers can collaborate with suppliers, distributors, and retailers, sharing demand forecasts and obtaining valuable input for more accurate predictions.
Artificial intelligence (AI) and machine learning: AI and machine learning algorithms can analyze vast amounts of data, identify complex patterns, and improve forecasting accuracy. These technologies can adapt to changing market conditions, identify anomalies, and provide insights for better demand forecasting.
By harnessing the power of technology and digital tools, manufacturers can leverage data-driven insights, automate processes, and enhance the accuracy and agility of their demand forecasting in a rapidly changing manufacturing landscape.
Wrapping Up
In conclusion, accurate demand forecasting is paramount for manufacturers operating in a changing manufacturing landscape. As technological advancements, market trends, economic factors, globalization, and regulatory changes shape the industry, manufacturers must adopt effective strategies to anticipate and meet customer demand. Throughout this article, we have explored various strategies and techniques for forecasting demand in uncertain manufacturing environments.
Data-driven demand forecasting techniques, such as historical data analysis, statistical models, machine learning, predictive analytics, and collaborative forecasting, provide manufacturers with valuable insights to make informed decisions. These techniques leverage data, patterns, and market intelligence to generate accurate demand forecasts.
Scenario planning and sensitivity analysis enable manufacturers to develop multiple demand scenarios and assess the impact of key variables on demand forecasts. By incorporating risk analysis and contingency planning, manufacturers can proactively respond to market fluctuations and mitigate potential disruptions.
Customer and market intelligence play a crucial role in demand forecasting. By conducting market research, leveraging social media and online analytics, collaborating with sales teams and channel partners, and monitoring competitor strategies, manufacturers gain valuable insights into customer preferences, trends, and market dynamics.
Agile manufacturing and flexible operations allow manufacturers to respond swiftly to changing demand. By implementing agile practices, investing in flexible production systems and supply chain capabilities, and leveraging predictive maintenance and real-time data analytics, manufacturers can adapt their operations to meet evolving customer needs.
Collaboration and communication across the value chain enhance demand visibility and forecasting accuracy. Strengthening relationships with suppliers, collaborating with distributors and retailers, and sharing data and insights with stakeholders facilitate better decision-making and alignment of supply and demand.
Continuous learning and adaptation are vital for improving demand forecasting accuracy. By monitoring and evaluating forecasting accuracy, incorporating feedback loops, and learning from past forecasting errors, manufacturers can continuously refine their forecasting strategies and optimize their operations.
In conclusion, navigating uncertainty in the changing manufacturing landscape requires a combination of data-driven techniques, scenario planning, market intelligence, agile operations, collaboration, and continuous learning. By adopting these strategies, manufacturers can effectively forecast demand, optimize resources, minimize risks, and gain a competitive edge in the dynamic manufacturing industry.
How Deskera Can Assist You?
Deskera ERP and MRP systems help you to keep your business units organized. The system's key features of demand forecasting with Deskera include as follows:
- Establish Demand Forecasting Process
- Automate Data Collection
- Monitor Market Trends
- Analyze Historical Data
- Estimate Future Demand
- Adjust Production Levels
- Manage Supply Chain...and much more!
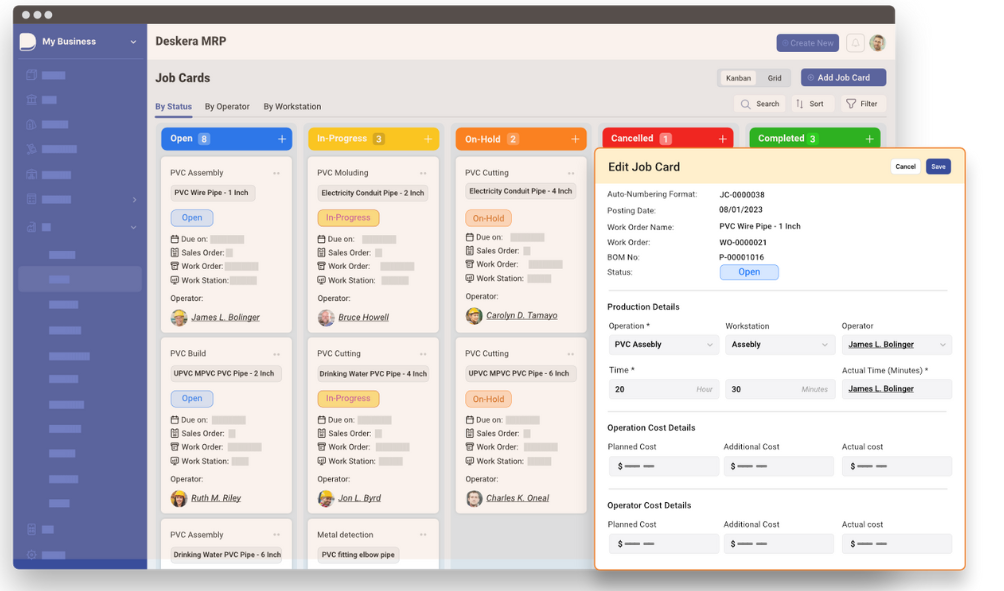
- Deskera Books allows you to better manage your accounts and finances. Maintain good accounting practices by automating tasks like billing, invoicing, and payment processing.
- Deskera's integrated financial planning tools enable investors to better plan and track their investments. It can assist investors in making faster and more accurate decisions.
- Deskera CRM is a powerful solution that manages your sales and helps you close deals quickly. It not only enables you to perform critical tasks like lead generation via email, but it also gives you a comprehensive view of your sales funnel.
- Deskera People is a straightforward tool for centralizing your human resource management functions.
Final Takeaways
We've arrived at the last section of this guide. Let's have a look at some of the most important points to remember:
- Accurate demand forecasting enables manufacturers to determine the right level of inventory to meet customer demands while avoiding excess stock or shortages.
- By aligning production and procurement plans with forecasted demand, manufacturers can optimize inventory levels, minimize carrying costs, and reduce the risk of stockouts or overstocking.
- Demand forecasting is crucial for supply chain management, as it allows manufacturers to synchronize their entire value chain based on anticipated demand.
- By sharing accurate forecasts with suppliers, distributors, and logistics partners, manufacturers can ensure timely and cost-effective procurement, production, and delivery processes.
- Technological advancements enable manufacturers to develop innovative products that cater to changing consumer needs and preferences. For example, the rise of smartphones and mobile applications has led to an increased demand for smart devices, wearable technology, and connected homes.
- Economic factors can impact consumer price sensitivity. During periods of economic uncertainty or recessions, consumers may become more price-conscious and opt for value-oriented products. Manufacturers should consider pricing strategies, promotions, and competitive positioning to capture demand in different economic scenarios.
- Regulatory changes in safety and quality standards can influence consumer confidence and demand for products. Compliance with evolving standards and certifications is crucial for manufacturers to ensure product acceptance and mitigate risks.
- Historical data analysis is a fundamental technique for demand forecasting. It involves analyzing past sales data, market trends, and other relevant historical information to identify patterns and trends. This technique helps manufacturers understand the historical demand patterns of their products and make informed forecasts.
Related Articles
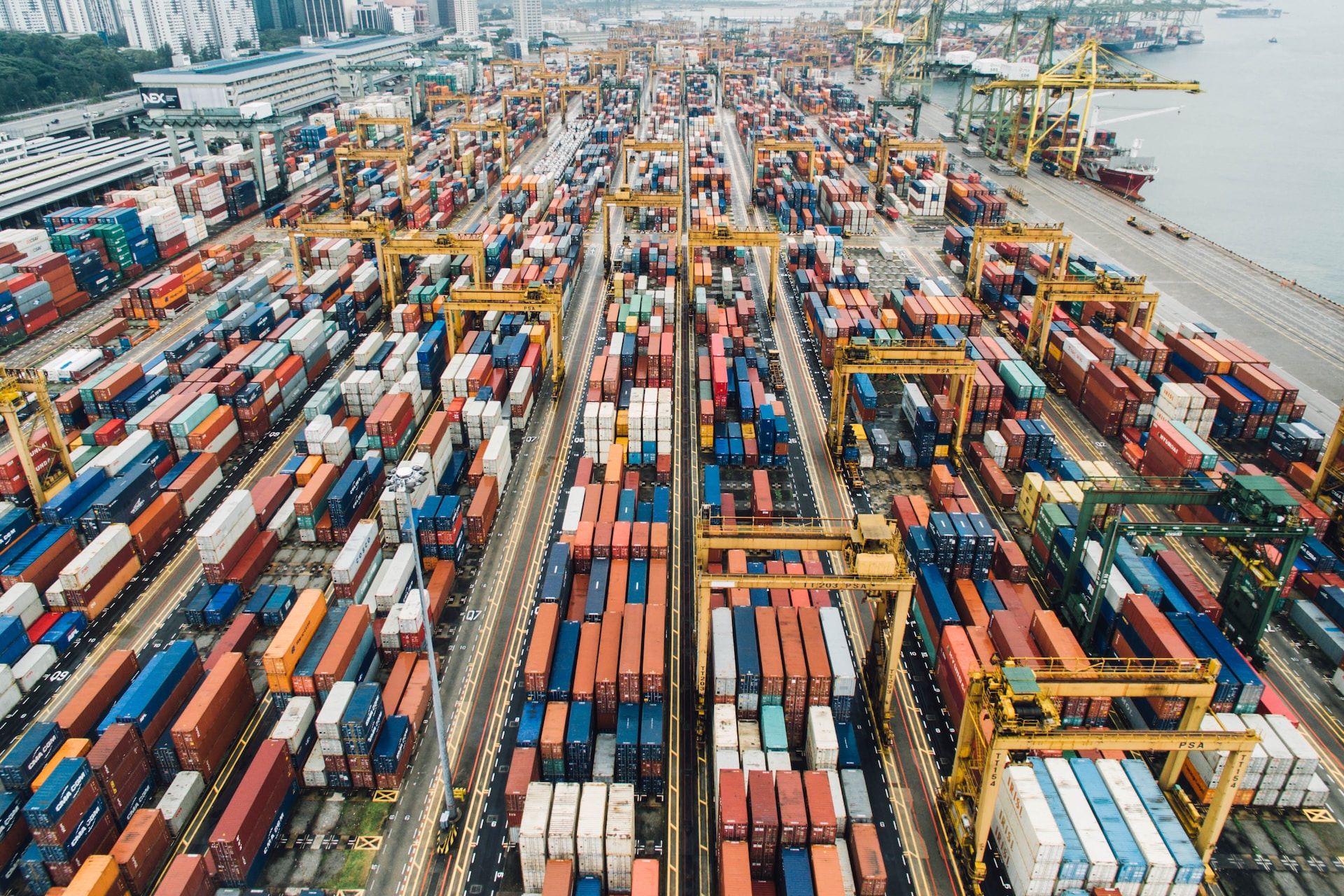
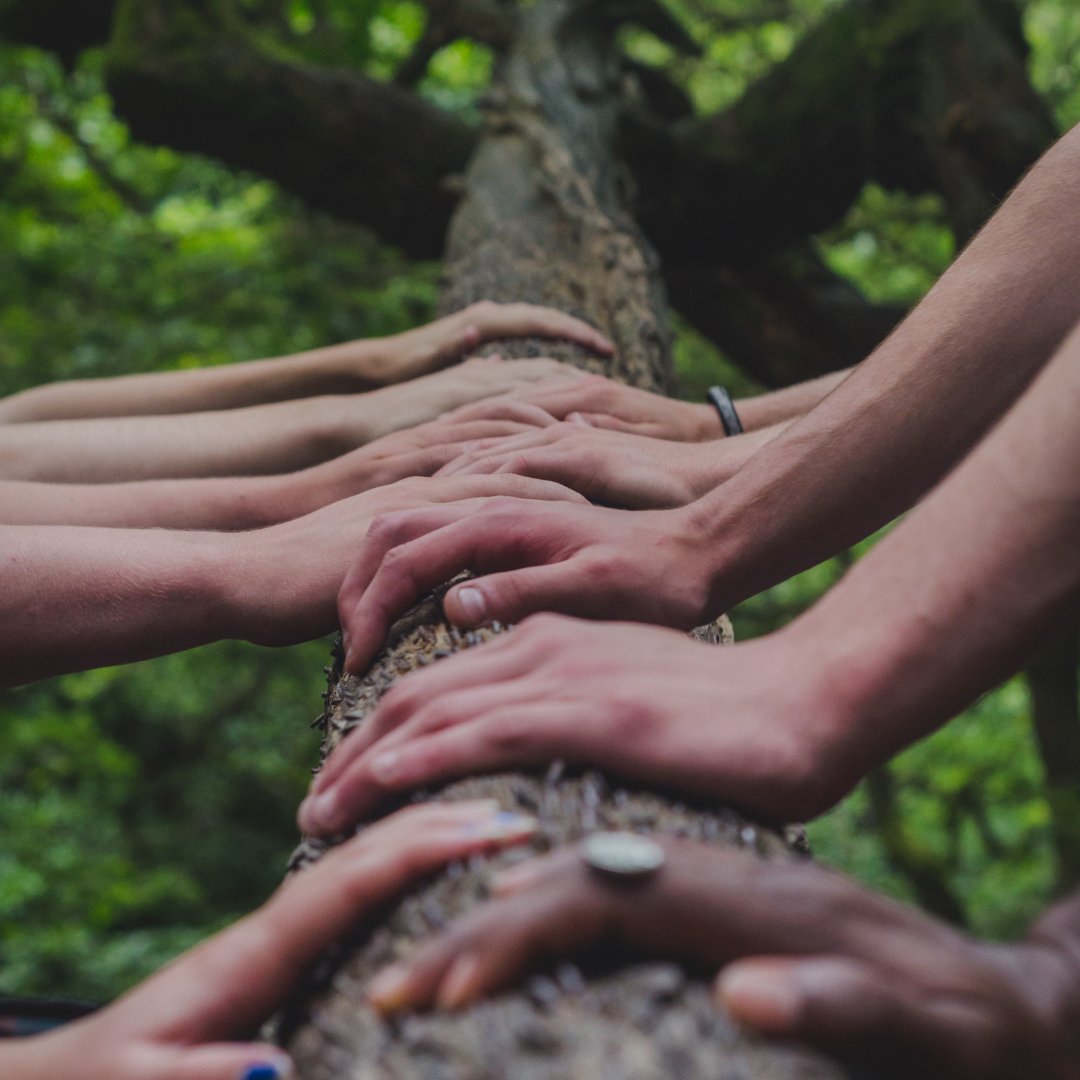
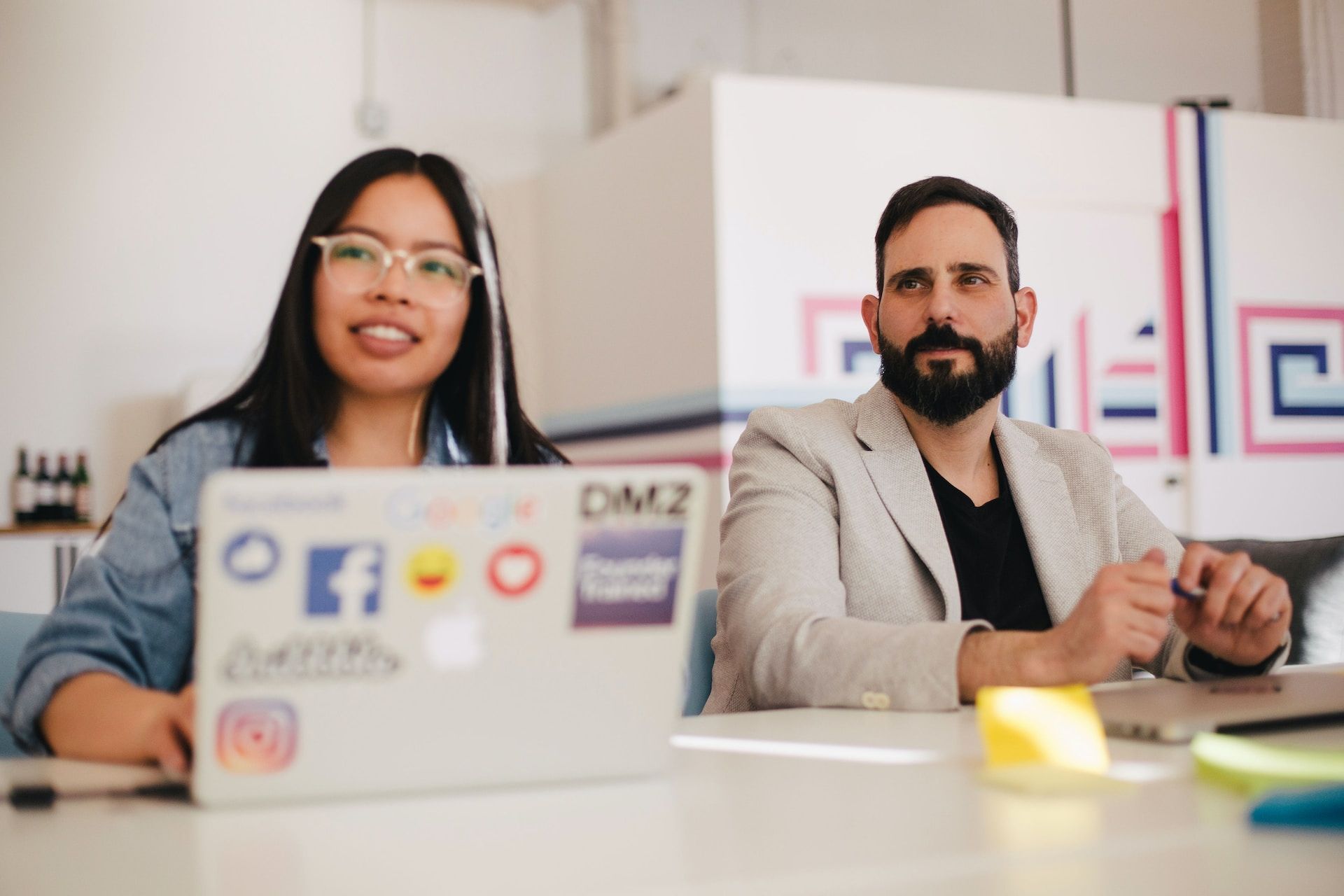
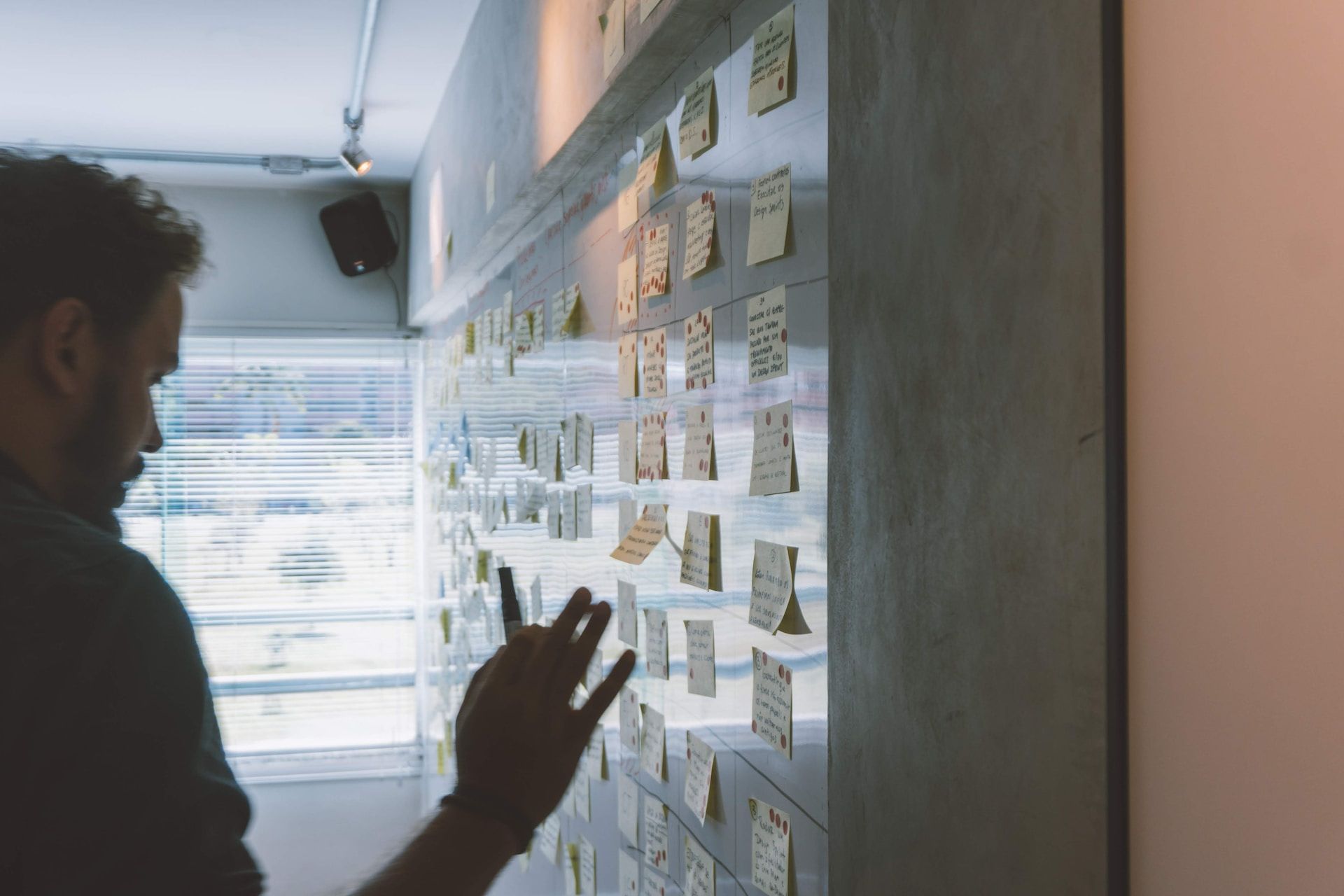