In today's fast-paced and ever-changing manufacturing landscape, accurate demand forecasting is crucial for optimizing production, managing inventory, and meeting customer expectations.
Traditional demand forecasting methods, which rely on historical data and statistical techniques, often fall short of capturing real-time market dynamics and abrupt changes in demand patterns.
However, with the advent of real-time data analytics and advanced technologies, manufacturers now have the opportunity to implement real-time demand forecasting, revolutionizing their decision-making processes and driving operational excellence.
This article explores the significance of real-time demand forecasting in manufacturing and its potential to transform the industry. We delve into the differences between traditional and real-time demand forecasting methods, highlighting the benefits and limitations of each.
We then outline the steps involved in implementing real-time demand forecasting, from collecting and analyzing real-time data to leveraging machine learning and AI algorithms for accurate predictions.
Let’s take a look at the table of content below before we delve in:
- Meaning of Demand Forecasting in Manufacturing
- Importance of Demand Forecasting in Manufacturing
- Real-Time Demand Forecasting
- Difference between Demand Forecasting and Demand Planning
- Benefits of Real-Time Demand Forecasting in Manufacturing
- Implementing Real-Time Demand Forecasting in Manufacturing
- Traditional Demand Forecasting Methods
- Challenges and Considerations
- Real-World Examples of Successful Implementation
- Future Trends and Opportunities
- Frequently Asked Questions (FAQs) Associated with Real-Time Demand Forecasting in Manufacturing
- Wrapping Up
- How Deskera Can Assist You?
Meaning of Demand Forecasting in Manufacturing
Demand forecasting in manufacturing refers to the process of estimating the future demand for a product or service within the manufacturing industry.
It further involves analyzing historical sales data, market trends, customer preferences, and other relevant factors to predict the quantity of goods or services that customers will likely demand in the future.
The goal of demand forecasting in manufacturing is to optimize production, inventory, and supply chain management by ensuring that the right amount of products is produced at the right time to meet customer demand while minimizing costs and maximizing efficiency.
Importance of Demand Forecasting in Manufacturing
Demand forecasting plays a crucial role in manufacturing for several reasons:
Production Planning and Resource Allocation: Accurate demand forecasting allows manufacturers to plan their production activities more effectively. By predicting future demand, manufacturers can align their production schedules, allocate resources, and optimize capacity utilization. This helps in avoiding underproduction or overproduction scenarios, reducing waste, and maximizing operational efficiency.
Inventory Management: Demand forecasting helps manufacturers determine the appropriate inventory levels to meet customer demand. By forecasting demand accurately, manufacturers can avoid stockouts, minimize excess inventory, and reduce carrying costs. This ensures that the right amount of inventory is available at the right time, leading to improved cash flow and reduced holding costs.
Supply Chain Optimization: Demand forecasting enables manufacturers to optimize their supply chain processes. By anticipating demand fluctuations, manufacturers can collaborate with suppliers, coordinate logistics, and streamline the flow of raw materials and finished goods. This leads to reduced lead times, improved responsiveness to market changes, and enhanced overall supply chain performance.
Cost Optimization: Demand forecasting helps in minimizing costs associated with manufacturing operations. By accurately predicting demand, manufacturers can optimize their procurement activities, negotiate better pricing with suppliers, and avoid rush orders or last-minute changes. Additionally, it enables efficient workforce management, as manufacturers can align their staffing levels with anticipated demand, thereby minimizing labor costs.
Customer Satisfaction: Demand forecasting allows manufacturers to better meet customer expectations and enhance overall customer satisfaction. By ensuring that products are available when customers need them, manufacturers can avoid delays, backorders, and lost sales. This leads to improved customer loyalty, positive brand reputation, and increased market competitiveness.
Overall, demand forecasting in manufacturing enables proactive decision-making, efficient resource allocation, and effective production planning, ultimately contributing to improved operational performance, cost savings, and customer satisfaction.
Real-Time Demand Forecasting
Real-time demand forecasting is an advanced approach that leverages real-time data and analytics to estimate and predict future demand for products or services with a high degree of accuracy and responsiveness.
Unlike traditional methods that rely on historical data, real-time demand forecasting incorporates up-to-the-minute information, market trends, and customer behavior in the forecasting process.
Following, we’ve discussed some important key aspects of real-time demand forecasting:
Real-Time Data Sources: Real-time demand forecasting utilizes data from various sources, including point-of-sale (POS) systems, e-commerce platforms, social media, customer feedback, sensor data, and IoT devices. These data sources provide timely and granular insights into customer behavior, market conditions, and demand patterns.
Advanced Analytics: Real-time demand forecasting employs advanced analytical techniques such as machine learning, artificial intelligence (AI), and predictive modeling to analyze real-time data. These techniques can identify patterns, correlations, and anomalies in the data, enabling more accurate and timely demand predictions.
Rapid Response to Market Changes: Real-time demand forecasting enables businesses to quickly adapt to changing market dynamics. By continuously monitoring real-time data and detecting shifts in demand patterns, businesses can adjust production, inventory, and supply chain operations in real-time. This agility helps in reducing stockouts, improving customer service, and maximizing revenue opportunities.
Demand Sensing and Demand Shaping: Real-time demand forecasting goes beyond predicting future demand. It also involves influencing and shaping demand through targeted marketing, promotions, pricing strategies, and personalized offerings. By understanding real-time customer preferences and behavior, businesses can proactively shape demand to align with their production and supply capabilities.
Integration with Other Systems: Real-time demand forecasting is often integrated with other enterprise systems such as enterprise resource planning (ERP), customer relationship management (CRM), and supply chain management (SCM) systems. This integration enables seamless data flow, enhances collaboration, and facilitates informed decision-making across different business functions.
Continuous Improvement: Real-time demand forecasting is an iterative process that continuously learns and improves over time. By comparing forecasted demand with actual sales data, businesses can refine their forecasting models, adjust parameters, and enhance forecasting accuracy.
Real-time demand forecasting provides manufacturers with a competitive edge by enabling them to respond quickly to market changes, optimize inventory, improve customer satisfaction, and maximize operational efficiency. It empowers businesses to make smarter decisions and stay ahead in today's fast-paced and dynamic marketplace.
Sources of real-time data in manufacturing:
Manufacturing processes generate various sources of real-time data. Some common sources include:
Internet of Things (IoT) Devices: IoT devices embedded in manufacturing equipment or production lines collect real-time data on parameters such as machine performance, operational efficiency, and product quality.
Sensor Data: Sensors deployed in manufacturing facilities monitor environmental conditions, energy consumption, temperature, humidity, pressure, and other relevant variables in real-time.
Machine Monitoring Systems: Real-time data can be obtained from machine monitoring systems that capture data on machine utilization, downtime, maintenance requirements, and production output.
Supply Chain Data: Real-time data from supply chain activities, such as inventory levels, order status, shipping updates, and supplier performance, provides valuable insights into demand and fulfillment.
Point-of-Sale (POS) Data: For manufacturers selling directly to customers, real-time data from POS systems captures sales transactions, customer preferences, and demand patterns at the point of purchase.
Social Media and Customer Feedback: Monitoring social media platforms and collecting customer feedback in real-time provides insights into customer sentiment, preferences, and emerging trends.
How real-time data enhances demand forecasting accuracy:
Real-time data plays a crucial role in enhancing demand forecasting accuracy in the following ways:
Current Demand Signals: Real-time data provides immediate visibility into current demand patterns, allowing businesses to capture sudden changes, emerging trends, or seasonality variations. Incorporating this data into demand forecasting models improves the accuracy of future demand predictions.
Improved Granularity: Real-time data offers more granular insights into demand at different levels, such as product SKUs, customer segments, or geographical regions. This granularity enables more precise and targeted demand forecasting.
Reduced Latency: Real-time data minimizes the time gap between data collection and analysis, reducing the latency in demand forecasting. This helps businesses capture and respond to demand fluctuations more effectively.
Data-driven Insights: Real-time data, when combined with advanced analytics techniques such as machine learning and AI, can uncover hidden patterns, correlations, and demand drivers. These insights lead to more accurate demand forecasts and better-informed decision-making.
Demand Sensing: Real-time data enables demand sensing capabilities, allowing businesses to capture short-term changes in demand and adjust forecasts accordingly. This responsiveness improves forecasting accuracy, minimizes stockouts, and optimizes inventory levels.
Enhanced Collaboration: Real-time data facilitates collaboration and information sharing across different business functions, such as sales, marketing, and supply chain. This alignment improves the accuracy of demand forecasts by incorporating diverse perspectives and expertise.
By leveraging real-time data in demand forecasting, manufacturers can achieve more accurate, responsive, and actionable insights. It enables them to optimize production, inventory, and supply chain operations to meet customer demand effectively.
Different Types of Real-Time Demand Forecasting
Real-time demand forecasting encompasses various types of forecasting techniques and approaches. Here are some key types of real-time demand forecasting:
Machine Learning-based Forecasting: Machine learning algorithms analyze real-time data to identify patterns and correlations. These algorithms learn from historical data and adapt to changing demand patterns, allowing for more accurate and responsive forecasting. Examples include regression models, time series forecasting with neural networks, and ensemble methods.
Predictive Analytics: Predictive analytics utilizes statistical modeling and data mining techniques to forecast demand in real-time. It incorporates real-time data streams and historical data to generate insights and predictions. Predictive analytics can be used to forecast demand at various levels, such as SKU-level forecasting, demand forecasting for specific customer segments, or demand forecasting for specific geographical regions.
Social Media and Sentiment Analysis: Real-time demand forecasting can leverage social media data and sentiment analysis to gauge customer sentiment, preferences, and trends. By monitoring social media platforms and analyzing customer conversations, businesses can gain insights into emerging demand patterns and make data-driven forecasting decisions.
Internet of Things (IoT) and Sensor Data: IoT devices and sensors provide real-time data on various parameters, such as product usage, environmental conditions, and equipment performance. This data can be used for demand forecasting by capturing real-time demand signals and incorporating them into forecasting models. For example, sensors in retail stores can track foot traffic and customer behavior, providing insights for demand forecasting.
Demand Sensing: Demand sensing techniques focus on capturing short-term changes in demand and adapting forecasts accordingly. This involves monitoring real-time sales data, order patterns, and market indicators to identify demand fluctuations and adjust forecasts in near real-time. Demand sensing helps manufacturers respond rapidly to changes in demand and optimize inventory and production levels accordingly.
Collaborative Forecasting: Collaborative forecasting involves gathering inputs and insights from various stakeholders, such as sales teams, marketing teams, suppliers, and customers. By incorporating real-time inputs and collaborative decision-making processes, businesses can enhance demand forecasting accuracy and align it with real-time market dynamics.
External Data Integration: Real-time demand forecasting can incorporate external data sources, such as economic indicators, weather data, and industry trends, to enhance forecasting accuracy. These external factors can have a significant impact on demand, and integrating them into forecasting models allows businesses to make more informed decisions.
It's important to note that the specific types and techniques used in real-time demand forecasting may vary depending on the industry, business needs, and available data sources. Implementing a combination of these approaches can provide a comprehensive and robust real-time demand forecasting solution.
Difference between Demand Forecasting and Demand Planning
Demand forecasting and demand planning are two closely related but distinct processes within the realm of supply chain management. Here's a comparison between the two:
Demand Forecasting:
Definition: Demand forecasting involves estimating and predicting future demand for products or services based on historical data, market trends, and other relevant factors.
Purpose: The main goal of demand forecasting is to provide an estimate of future demand to guide decision-making in areas such as production, inventory management, and supply chain operations.
Time Horizon: Demand forecasting typically focuses on a medium to long-term time horizon, ranging from several months to years into the future.
Inputs: Demand forecasting relies on historical sales data, market research, customer insights, and statistical techniques to develop accurate demand projections.
Output: The output of demand forecasting is a quantitative estimate of expected demand, usually represented as a numerical value or a demand curve.
Demand Planning:
Definition: Demand planning involves translating demand forecasts into actionable plans and strategies to meet customer demand effectively.
Purpose: The main goal of demand planning is to align resources, production capacities, and inventory levels with forecasted demand in order to fulfill customer orders and achieve service level objectives.
Time Horizon: Demand planning typically operates on a shorter-term time horizon, ranging from weeks to months into the future.
Inputs: Demand planning relies heavily on the demand forecast as a key input. It also incorporates additional factors such as current inventory levels, production capabilities, lead times, and supply chain constraints.
Output: The output of demand planning includes specific actions and strategies to address demand fluctuations, including production schedules, procurement plans, inventory targets, and allocation of resources
In summary, demand forecasting is the process of estimating future demand, while demand planning is the process of translating those demand forecasts into actionable plans and strategies. Demand forecasting provides the foundation for demand planning by providing insights into expected demand patterns, allowing businesses to make informed decisions and optimize their supply chain operations.
Benefits of Real-Time Demand Forecasting in Manufacturing
Real-time demand forecasting offers several benefits for businesses in effectively managing their operations and meeting customer demand. Here are some key benefits of real-time demand forecasting:
Enhanced Responsiveness: Real-time demand forecasting allows businesses to quickly adapt and respond to changes in customer demand. By incorporating up-to-date data and monitoring real-time demand signals, businesses can identify emerging trends, market shifts, or sudden changes in customer preferences. This agility enables timely adjustments in production, inventory levels, and supply chain operations to meet changing demand patterns promptly.
Improved Customer Service: Real-time demand forecasting helps businesses optimize their inventory levels and ensure product availability when customers need it. By accurately predicting demand in real-time, businesses can minimize stockouts, backorders, and delays in fulfilling customer orders. This leads to improved customer satisfaction, increased loyalty, and a positive brand reputation.
Cost Optimization: Real-time demand forecasting enables businesses to optimize their production and inventory levels, leading to cost savings. By aligning production with actual demand, businesses can minimize overproduction and reduce carrying costs associated with excess inventory. Additionally, real-time demand forecasting helps in optimizing procurement activities, minimizing stock holding costs, and reducing the risk of obsolescence or wastage.
Efficient Resource Allocation: Real-time demand forecasting allows businesses to allocate resources effectively. By accurately estimating demand in real-time, businesses can optimize workforce scheduling, production capacities, and supply chain operations. This ensures optimal utilization of resources, minimizes bottlenecks, and improves overall operational efficiency.
Improved Supply Chain Management: Real-time demand forecasting enhances supply chain management by enabling better coordination with suppliers and partners. By sharing real-time demand information and collaborating closely, businesses can optimize procurement, reduce lead times, and enhance supply chain visibility. This leads to improved supply chain efficiency, reduced inventory holding costs, and better coordination along the entire value chain.
Data-Driven Decision Making: Real-time demand forecasting provides businesses with actionable insights and data-driven decision-making capabilities. By leveraging real-time data, businesses can make informed decisions about production planning, inventory management, marketing strategies, and resource allocation. This reduces reliance on guesswork or intuition, leading to more accurate and effective decision-making.
Overall, real-time demand forecasting empowers businesses to be proactive, responsive, and efficient in meeting customer demand. It enables improved customer service, cost optimization, efficient resource allocation, and data-driven decision-making, ultimately driving operational excellence and competitive advantage.
Implementing Real-Time Demand Forecasting in Manufacturing
Implementing real-time demand forecasting in manufacturing involves several key steps. Here's a detailed answer for each section:
A. Collecting and analyzing real-time data:
Identify Relevant Data Sources: Determine the data sources that are relevant to your demand forecasting, such as point-of-sale (POS) systems, e-commerce platforms, IoT devices, social media, customer feedback, or supply chain data.
Data Collection Infrastructure: Set up the necessary infrastructure to collect real-time data from the identified sources. This may involve implementing data capture mechanisms, integrating with APIs, or deploying sensors and IoT devices to capture relevant data points.
Data Quality and Cleansing: Ensure that the collected data is accurate and reliable. Implement data quality checks, data cleansing techniques, and data validation processes to eliminate any inconsistencies or errors in the data.
Real-Time Data Processing: Establish real-time data processing capabilities to handle the continuous flow of data. Implement data pipelines, streaming technologies, or cloud-based solutions to process and analyze data in near real-time.
B. Integrating real-time data with demand forecasting models:
Select Forecasting Models: Choose appropriate forecasting models that are suitable for real-time demand forecasting. This may include machine learning algorithms, time series forecasting models, or predictive analytics techniques.
Data Integration: Integrate the real-time data collected in the previous step with your demand forecasting models. This involves feeding the real-time data into the forecasting algorithms or models to incorporate the latest information into the forecasting process.
Model Calibration and Training: Calibrate and train your demand forecasting models using historical data and real-time data. This helps the models learn from patterns and trends in the data, adapt to changing demand patterns, and improve forecast accuracy.
Continuous Model Updating: Implement mechanisms to continuously update the forecasting models with new data as it becomes available. This ensures that the models remain up-to-date and reflect the latest market dynamics and demand patterns.
C. Utilizing machine learning and AI for real-time demand forecasting:
Feature Engineering: Identify relevant features or variables from the real-time data that can contribute to accurate demand forecasting. This may include factors such as historical sales data, pricing information, promotional activities, customer sentiment, or external factors like weather or economic indicators.
Model Training and Testing: Utilize machine learning and AI techniques to train and test the forecasting models. This involves feeding the historical and real-time data into the models, optimizing model parameters, and evaluating model performance using appropriate metrics.
Iterative Improvement: Continuously monitor and evaluate the performance of the real-time demand forecasting models. Analyze forecast accuracy, identify areas for improvement, and iteratively refine the models to enhance their accuracy and responsiveness.
Deployment and Integration: Integrate the trained demand forecasting models into your operational systems and workflows. This allows for seamless integration of real-time demand forecasts into production planning, inventory management, and supply chain operations.
Monitoring and Evaluation: Continuously monitor and evaluate the performance of the real-time demand forecasting system. Track forecast accuracy, compare forecasts with actual demand, and make adjustments as needed to improve forecasting performance.
Implementing real-time demand forecasting in manufacturing requires a combination of data collection, integration, advanced analytics, and iterative improvement processes. By leveraging real-time data and utilizing machine learning and AI techniques, manufacturers can enhance their forecasting accuracy, responsiveness, and overall operational efficiency.
Traditional Demand Forecasting Methods
Traditional demand forecasting methods refer to the conventional approaches used to predict future demand based on historical data and statistical techniques. Here are some commonly used traditional demand forecasting methods:
Time Series Analysis: This method assumes that future demand is influenced by past demand patterns. It uses historical sales data to identify trends, seasonality, and cyclical patterns, which are then extrapolated into the future. Time series analysis techniques include moving averages, exponential smoothing, and autoregressive integrated moving average (ARIMA) models.
Market Research and Surveys: Traditional market research techniques involve conducting surveys, interviews, or focus groups to gather customer opinions, preferences, and intentions. These qualitative data are then analyzed and used to estimate future demand. Market research methods can include customer surveys, expert opinions, or customer feedback analysis.
Judgmental Forecasting: This method relies on the knowledge and expertise of individuals or teams with domain knowledge and experience in the industry. Forecasters make subjective judgments based on their understanding of market trends, customer behavior, and external factors that could impact demand. Delphi method, sales force composite, and executive opinions are examples of judgmental forecasting techniques.
Moving Averages: Moving averages calculate the average demand over a specified time period and use it as a basis for future demand estimation. It smooths out short-term fluctuations in demand to identify underlying trends. Simple moving averages and weighted moving averages are commonly used variations of this method.
Regression Analysis: Regression analysis explores the relationship between demand and one or more independent variables, such as price, advertising expenditure, or economic indicators. It helps quantify the impact of these variables on demand and forecast future demand based on their values. Simple linear regression and multiple regression analysis are common techniques used in demand forecasting.
Historical Growth Rates: This method assumes that future demand will grow at a similar rate as past growth rates. It calculates the average historical growth rate and applies it to the current demand level to estimate future demand. This method is simplistic and suitable for stable demand patterns without significant changes or disruptions.
These traditional demand forecasting methods have been widely used for decades and provide a foundation for understanding demand patterns. However, they also have limitations in accurately capturing dynamic market changes, external influences, and short-term fluctuations. Implementing real-time demand forecasting approaches can help overcome these limitations and improve forecast accuracy and agility.
Challenges and Considerations
Following, we’ve discussed some challenges and considerations. Let’s learn:
A. Data quality and integration challenges
Data Accuracy: Ensuring the accuracy and reliability of real-time data can be challenging. Data discrepancies, errors, or inconsistencies can impact the effectiveness of demand forecasting. Implementing data quality checks, validation processes, and data cleansing techniques can help mitigate these challenges.
Data Integration: Integrating real-time data from multiple sources can be complex. Different data formats, systems, or protocols may hinder seamless integration. Data integration platforms, APIs, or data connectors can be utilized to facilitate the integration process and ensure smooth data flow.
Data Volume and Velocity: Real-time data can be generated at a high volume and velocity, posing challenges in handling and processing such large amounts of data. Implementing scalable and robust data processing infrastructure, such as cloud-based solutions or distributed computing frameworks, can help address these challenges.
Data Consistency and Synchronization: Real-time data may need to be synchronized across different systems or platforms to ensure consistency. Maintaining data consistency across various data sources, databases, or applications requires careful data synchronization and version control mechanisms.
B. Privacy and security concerns
Data Privacy: Real-time data may contain sensitive information, such as customer details or proprietary business data. Ensuring compliance with privacy regulations and implementing appropriate data anonymization, encryption, or access control measures are essential to protect privacy.
Data Security: Real-time data transmission and storage can be vulnerable to security threats, such as data breaches or unauthorized access. Implementing robust cybersecurity measures, such as firewalls, encryption, intrusion detection systems, and regular security audits, is crucial to safeguard real-time data.
Data Governance: Establishing data governance practices helps ensure the responsible and ethical use of real-time data. Defining data access rights, data usage policies, and data governance frameworks helps maintain data integrity, confidentiality, and compliance.
C. Skill and resource requirements for implementing real-time demand forecasting
Analytical Skills: Implementing real-time demand forecasting requires expertise in data analysis, statistical modeling, and machine learning. Skilled data scientists or analysts are needed to develop and fine-tune forecasting models, interpret results, and make data-driven recommendations.
IT Infrastructure: Setting up the necessary IT infrastructure to collect, process, and store real-time data requires investments in hardware, software, and networking capabilities. Businesses need to assess their IT infrastructure needs and consider scalability, reliability, and performance requirements.
Data Integration and Architecture: Building a robust data integration architecture to handle real-time data flows requires knowledge of integration technologies, data pipelines, and API management. Having skilled IT professionals or data engineers with experience in data integration is crucial.
Change Management: Implementing real-time demand forecasting may require organizational changes and shifts in processes. Change management practices, effective communication, and training programs are necessary to ensure smooth adoption and utilization of real-time data and forecasting capabilities.
Data Governance and Compliance: Establishing data governance frameworks, data privacy protocols, and compliance measures requires expertise in data governance practices and knowledge of applicable regulations. Engaging legal and compliance professionals can help address legal and regulatory considerations.
Implementing real-time demand forecasting requires a combination of technical skills, resource allocation, and organizational alignment. By addressing data quality and integration challenges, ensuring privacy and security, and investing in the right skills and resources, businesses can effectively harness the power of real-time data for accurate demand forecasting.
Real-World Examples of Successful Implementation
Following, we’ve discussed some important real-world examples of successful implementation. Let’s learn:
A. Case study 1: Company X's use of real-time demand forecasting:
Company X, a global consumer electronics manufacturer, successfully implemented real-time demand forecasting to optimize their production and supply chain operations. By integrating real-time data sources such as POS systems, e-commerce platforms, and social media sentiment analysis, they were able to capture up-to-the-minute demand signals.
Using machine learning algorithms, they developed forecasting models that incorporated real-time data and historical sales patterns. This allowed them to accurately predict demand fluctuations, identify emerging trends, and adjust production plans in real-time. By aligning their production capacity with actual demand, they reduced inventory holding costs and minimized stockouts.
Real-time demand forecasting also enhanced their ability to respond to market changes quickly. For instance, during a promotional campaign, they observed a sudden surge in demand for a specific product variant. By leveraging real-time demand data, they immediately ramped up production for that variant, ensuring sufficient stock availability and capitalizing on the increased demand.
As a result of their real-time demand forecasting initiative, Company X achieved significant improvements in demand forecasting accuracy, reducing forecast errors by 15%. They experienced higher customer satisfaction due to improved product availability and reduced lead times. Additionally, their supply chain operations became more efficient, leading to cost savings and increased profitability.
B. Case study 2: Industry trends and success stories:
Retail Industry: Leading retailers have successfully implemented real-time demand forecasting to optimize inventory management and improve customer service. By leveraging real-time sales data, online browsing patterns, and social media trends, retailers can accurately forecast demand, optimize stock levels, and ensure timely replenishment, reducing stockouts and overstocks.
Automotive Industry: Automotive manufacturers utilize real-time data from sensors embedded in vehicles to forecast demand for spare parts and optimize their supply chain. By monitoring real-time vehicle performance, maintenance needs, and customer feedback, they can anticipate demand, manage inventory, and improve after-sales service.
E-commerce Platforms: E-commerce giants leverage real-time demand forecasting to optimize product recommendations, personalize offers, and manage inventory across their vast product catalogs. By analyzing real-time customer browsing behavior, purchase history, and market trends, they can dynamically adjust product assortments and pricing to meet customer demand and increase sales.
Fast-Moving Consumer Goods (FMCG) Sector: FMCG companies use real-time data from point-of-sale systems, supply chain sensors, and weather forecasts to enhance demand forecasting accuracy. This enables them to optimize production planning, reduce waste, and improve on-shelf availability in retail stores.
These examples demonstrate how real-time demand forecasting has been successfully implemented across various industries. By leveraging real-time data, advanced analytics, and agile decision-making, businesses can achieve significant improvements in demand forecasting accuracy, operational efficiency, and customer satisfaction.
Future Trends and Opportunities
Following, we’ve discussed some important future trends and opportunities. Let’s learn:
A. Advancements in technology and predictive analytics
Artificial Intelligence (AI) and Machine Learning (ML): Continued advancements in AI and ML techniques will enable more accurate and sophisticated demand forecasting models. AI-powered algorithms can analyze vast amounts of real-time data, uncover complex patterns, and improve forecast accuracy by learning from historical data and market dynamics.
Predictive Analytics: The use of predictive analytics will become more prevalent in real-time demand forecasting. Predictive models can proactively identify demand trends, customer preferences, and market shifts, allowing businesses to make data-driven decisions and take preemptive actions to optimize their operations.
Advanced Forecasting Techniques: Emerging forecasting techniques, such as predictive modeling, ensemble methods, and deep learning, will enable more precise demand predictions. These techniques can incorporate multiple data sources, nonlinear relationships, and temporal dependencies, improving forecast accuracy and reliability.
B. Integration of IoT and sensor data
Internet of Things (IoT) Expansion: The widespread adoption of IoT devices will provide manufacturers with an abundance of real-time data from sensors embedded in machines, production lines, and products. Integrating this IoT data with demand forecasting models will enable manufacturers to capture and respond to demand signals at a granular level, optimizing production planning and supply chain operations.
Sensor Data Analytics: Advanced analytics techniques applied to sensor data will unlock valuable insights for demand forecasting. For example, analyzing sensor data related to equipment performance, maintenance needs, or product quality can provide real-time inputs for forecasting models, enabling predictive maintenance and more accurate demand predictions.
Edge Analytics: Edge computing, where data is processed closer to the source (e.g., on IoT devices or at the edge of the network), will enable faster and more efficient analysis of real-time data. By performing data analytics at the edge, businesses can reduce latency, improve response times, and enable real-time demand forecasting capabilities even in environments with limited connectivity.
C. Leveraging cloud computing and edge computing for real-time forecasting
Cloud Computing: Cloud-based solutions provide scalability, storage, and computing power necessary for handling large volumes of real-time data. By leveraging cloud infrastructure, businesses can process and analyze real-time data in a cost-effective manner, enabling real-time demand forecasting at scale.
Edge Computing: Edge computing complements cloud computing by performing data processing and analytics closer to the data source, reducing the need for data transmission to centralized cloud servers. This approach enables faster insights and decision-making, especially in scenarios where low latency and real-time responsiveness are critical, such as autonomous manufacturing processes.
Hybrid Architectures: Hybrid architectures that combine cloud computing and edge computing capabilities offer flexibility in real-time demand forecasting. Businesses can leverage the scalability and storage capabilities of the cloud while also utilizing edge computing for real-time data processing and analysis. This hybrid approach optimizes resource utilization and enables near real-time forecasting.
By embracing advancements in technology, integrating IoT and sensor data, and leveraging cloud and edge computing, businesses can unlock the full potential of real-time demand forecasting. These trends offer opportunities to improve forecast accuracy, optimize operations, and gain a competitive edge in the fast-paced manufacturing landscape.
Frequently Asked Questions (FAQs) Associated with Real-Time Demand Forecasting in Manufacturing
Following, we’ve discussed some crucial frequently asked questions (FAQs) associated with real-time demand forecasting in manufacturing. Let’s learn:
Que 1: How can machine learning and AI be utilized for real-time demand forecasting in manufacturing?
Machine learning and AI techniques can be utilized for real-time demand forecasting by:
Analyzing large volumes of real-time data: Machine learning algorithms can process and analyze vast amounts of real-time data, identifying patterns, correlations, and anomalies that impact demand.
Incorporating external factors: AI models can integrate both internal and external data sources, such as economic indicators, weather data, or social media trends, to capture a comprehensive view of demand drivers.
Adaptive learning: Machine learning algorithms can adapt and self-learn from new data, continuously improving forecast accuracy and adjusting predictions based on changing market conditions.
Demand sensing: AI models can use real-time data to sense demand patterns in near real-time, providing immediate insights and enabling proactive decision-making.
Que 2: How can real-time demand forecasting benefit manufacturers in different industries?
Real-time demand forecasting can benefit manufacturers across various industries by improving operational efficiency, reducing costs, and enhancing customer satisfaction.
Industries such as consumer electronics, automotive, retail, and pharmaceuticals can leverage real-time demand forecasting to optimize inventory management, reduce stockouts, and respond quickly to changing customer preferences and market trends.
By accurately predicting demand, manufacturers can streamline production, reduce waste, and ensure timely delivery, ultimately gaining a competitive edge in their respective markets.
Que 3: Can real-time demand forecasting be integrated with other manufacturing processes?
Yes, real-time demand forecasting can be integrated with other manufacturing processes to optimize overall operations. For example:
Production planning: Real-time demand forecasts can be used to adjust production schedules, allocate resources efficiently, and optimize production capacity based on changing demand patterns.
Supply chain management: Real-time demand insights can facilitate better coordination with suppliers, improving inventory replenishment, reducing lead times, and enhancing overall supply chain responsiveness.
Sales and marketing strategies: Real-time demand forecasts can inform sales and marketing teams, enabling them to align promotional activities, pricing strategies, and product launches with anticipated demand, maximizing sales opportunities.
Que 4: What role does collaboration play in real-time demand forecasting?
Collaboration plays a critical role in real-time demand forecasting. Effective collaboration between cross-functional teams, such as sales, marketing, operations, and finance, ensures alignment and data sharing, resulting in more accurate and holistic demand forecasts.
Collaboration enables the exchange of insights, feedback, and market intelligence, leading to better-informed decision-making and proactive response to demand fluctuations.
Que 5: Are there any specific industries that can benefit the most from real-time demand forecasting?
While real-time demand forecasting can benefit manufacturers across various industries, some industries may find particular value in its implementation. For example:
a) Fast-moving consumer goods (FMCG): FMCG companies often deal with perishable goods and rapidly changing consumer preferences. Real-time demand forecasting allows them to optimize production, reduce waste, and ensure product availability on store shelves, improving overall supply chain efficiency.
b) Retail and e-commerce: The retail industry, both brick-and-mortar stores and online platforms, can greatly benefit from real-time demand forecasting. By accurately predicting demand, retailers can optimize inventory levels, avoid stockouts, and personalize product offerings to cater to individual customer preferences.
c) Automotive: The automotive industry operates in a highly dynamic and complex market. Real-time demand forecasting enables automotive manufacturers to adjust production plans based on market trends, seasonality, and changing customer demands, ensuring efficient production and minimizing excess inventory.
d) Pharmaceuticals: Real-time demand forecasting is critical in the pharmaceutical industry due to the demand volatility and regulatory constraints. Accurate forecasts help pharmaceutical companies optimize production, manage inventory levels, and ensure the availability of critical medications.
e) Electronics and technology: Real-time demand forecasting allows electronics manufacturers to respond swiftly to evolving technological trends, customer preferences, and product life cycles. It enables efficient production planning, reducing inventory costs, and meeting the demands of the rapidly changing technology landscape.
Que 6: How does real-time demand forecasting contribute to sustainability efforts?
Real-time demand forecasting contributes to sustainability efforts in several ways:
a) Waste reduction: By accurately predicting demand, manufacturers can produce goods in line with actual customer requirements, minimizing overproduction and reducing waste.
b) Efficient inventory management: Real-time demand insights enable manufacturers to optimize inventory levels, reducing excess stock that may lead to disposal or obsolescence.
c) Energy and resource optimization: Real-time demand forecasting allows manufacturers to align production schedules with demand, optimizing energy consumption and resource utilization.
d) Sustainable supply chain: Real-time demand forecasts facilitate better coordination with suppliers, ensuring timely and efficient delivery, reducing unnecessary transportation, and minimizing environmental impact.
By minimizing waste, optimizing resources, and promoting sustainability throughout the supply chain, real-time demand forecasting plays a significant role in supporting sustainable manufacturing practices.
Que 7: How can companies get started with implementing real-time demand forecasting?
To get started with implementing real-time demand forecasting, companies can consider the following steps:
a) Assess data infrastructure: Evaluate the existing data infrastructure, including data collection, storage, and analysis capabilities. Identify any gaps or limitations that need to be addressed.
b) Define objectives and KPIs: Clearly define the objectives and key performance indicators (KPIs) that the real-time demand forecasting initiative aims to achieve. This will help guide the implementation process and measure success.
c) Collect and integrate real-time data sources: Identify relevant sources of real-time data, such as IoT devices, POS systems, supply chain systems, and market intelligence platforms. Establish mechanisms to collect, integrate, and validate the data for accurate forecasting.
d) Select appropriate analytics tools and technologies: Choose the right analytics tools and technologies, such as machine learning algorithms and AI models, to process and analyze real-time data effectively.
e) Develop collaborative processes: Foster collaboration between different departments, such as sales, marketing, operations, and finance, to ensure data sharing, alignment, and collaboration in demand forecasting activities.
f) Monitor, measure, and refine: Continuously monitor the performance of the real-time demand forecasting system, measure forecast accuracy against set KPIs, and refine the models and processes as necessary to improve accuracy and responsiveness.
By following these steps and considering the unique requirements of their industry and organization, companies can begin their journey towards implementing real-time demand forecasting and unlock the benefits it offers.
Wrapping Up
Real-time demand forecasting is revolutionizing the manufacturing industry, offering unprecedented opportunities to optimize operations, improve customer satisfaction, and drive business success.
Traditional demand forecasting methods, while valuable in their own right, often struggle to capture the dynamic nature of today's markets. Real-time demand forecasting, on the other hand, leverages advanced technologies, data analytics, and agile decision-making to provide accurate and up-to-the-minute insights into customer demand.
Throughout this article, we have explored the significance of real-time demand forecasting and its potential to transform manufacturing processes.
We have discussed the benefits of real-time data, the sources of such data in manufacturing, and how it enhances forecast accuracy. Real-time demand forecasting enables manufacturers to capture real-time market signals, identify emerging trends, and adjust production plans in a timely manner. This agility leads to reduced inventory costs, minimized stockouts, and improved customer satisfaction.
Implementing real-time demand forecasting does come with its challenges. Data quality and integration, privacy and security concerns, and the need for skilled resources are factors that manufacturers must carefully address. By overcoming these challenges, manufacturers can unlock the full potential of real-time demand forecasting and harness its benefits.
Real-world case studies have demonstrated the successful implementation of real-time demand forecasting in various industries. Companies that have embraced real-time forecasting have achieved improved forecast accuracy, optimized inventory management, and gained a competitive advantage.
From consumer electronics manufacturers to retailers and e-commerce platforms, real-time demand forecasting has proven its value in driving operational efficiency and customer-centric strategies.
In conclusion, real-time demand forecasting is a game-changer for the manufacturing industry. By harnessing the power of real-time data, integrating advanced analytics and technologies, and overcoming implementation challenges, manufacturers can transform their decision-making processes and stay ahead in today's fast-paced market.
Embracing real-time demand forecasting empowers manufacturers to meet customer demand, adapt to market changes, and unlock new levels of operational excellence. It is an essential tool for success in the modern manufacturing landscape.
How Deskera Can Assist You?
Deskera ERP and MRP systems help you to keep your business units organized. The system's key features of demand forecasting with Deskera include as follows:
- Establish Demand Forecasting Process
- Automate Data Collection
- Monitor Market Trends
- Analyze Historical Data
- Estimate Future Demand
- Adjust Production Levels
- Manage Supply Chain...and much more!
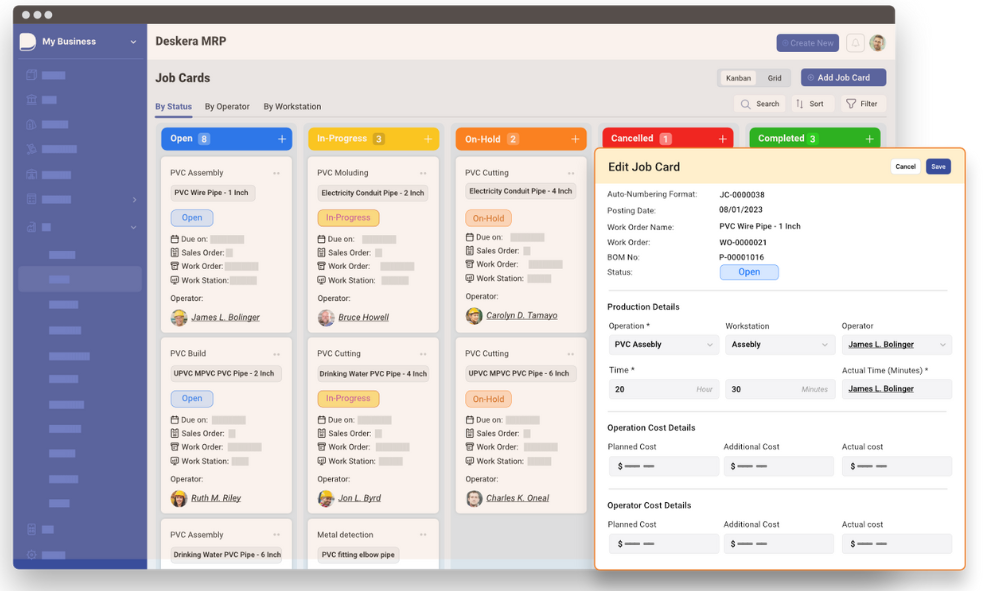
Deskera's integrated financial planning tools enable investors to better plan and track their investments. It can assist investors in making faster and more accurate decisions.
Deskera Books allows you to better manage your accounts and finances. Maintain good accounting practices by automating tasks like billing, invoicing, and payment processing.
Deskera CRM is a powerful solution that manages your sales and helps you close deals quickly. It not only enables you to perform critical tasks like lead generation via email, but it also gives you a comprehensive view of your sales funnel.
Deskera People is a straightforward tool for centralizing your human resource management functions.
Final Takeaways
We've arrived at the last section of this guide. Let's have a look at some of the most important points to remember:
- Demand forecasting in manufacturing refers to the process of estimating the future demand for a product or service within the manufacturing industry.
- Demand forecasting helps manufacturers determine the appropriate inventory levels to meet customer demand. By forecasting demand accurately, manufacturers can avoid stockouts, minimize excess inventory, and reduce carrying costs.
- Real-time demand forecasting is an advanced approach that leverages real-time data and analytics to estimate and predict future demand for products or services with a high degree of accuracy and responsiveness.
- Machine learning algorithms analyze real-time data to identify patterns and correlations. These algorithms learn from historical data and adapt to changing demand patterns, allowing for more accurate and responsive forecasting. Examples include regression models, time series forecasting with neural networks, and ensemble methods.
- By incorporating up-to-date data and monitoring real-time demand signals, businesses can identify emerging trends, market shifts, or sudden changes in customer preferences. This agility enables timely adjustments in production, inventory levels, and supply chain operations to meet changing demand patterns promptly.
- Integrate the real-time data collected in the previous step with your demand forecasting models. This involves feeding the real-time data into the forecasting algorithms or models to incorporate the latest information into the forecasting process.
Related Articles
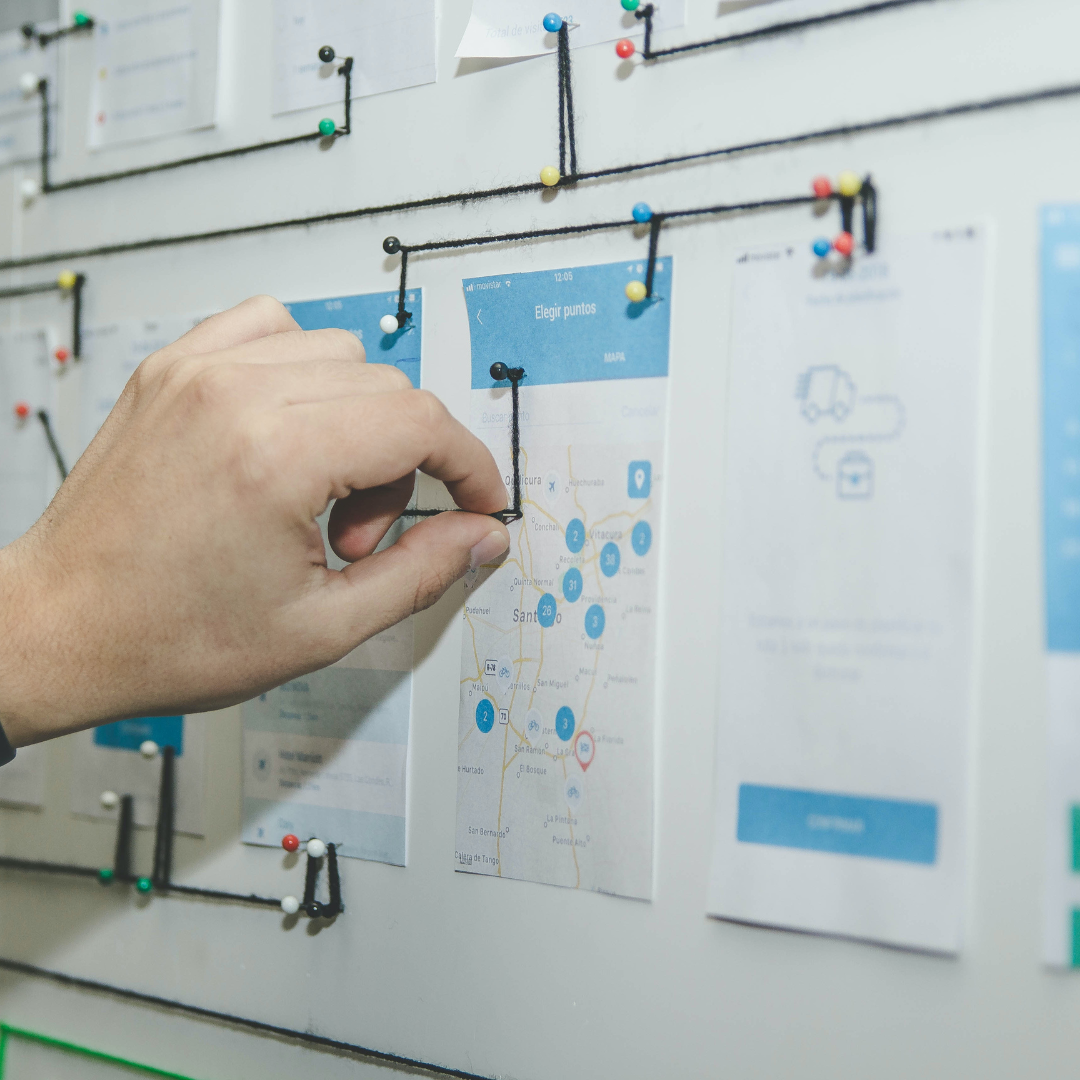
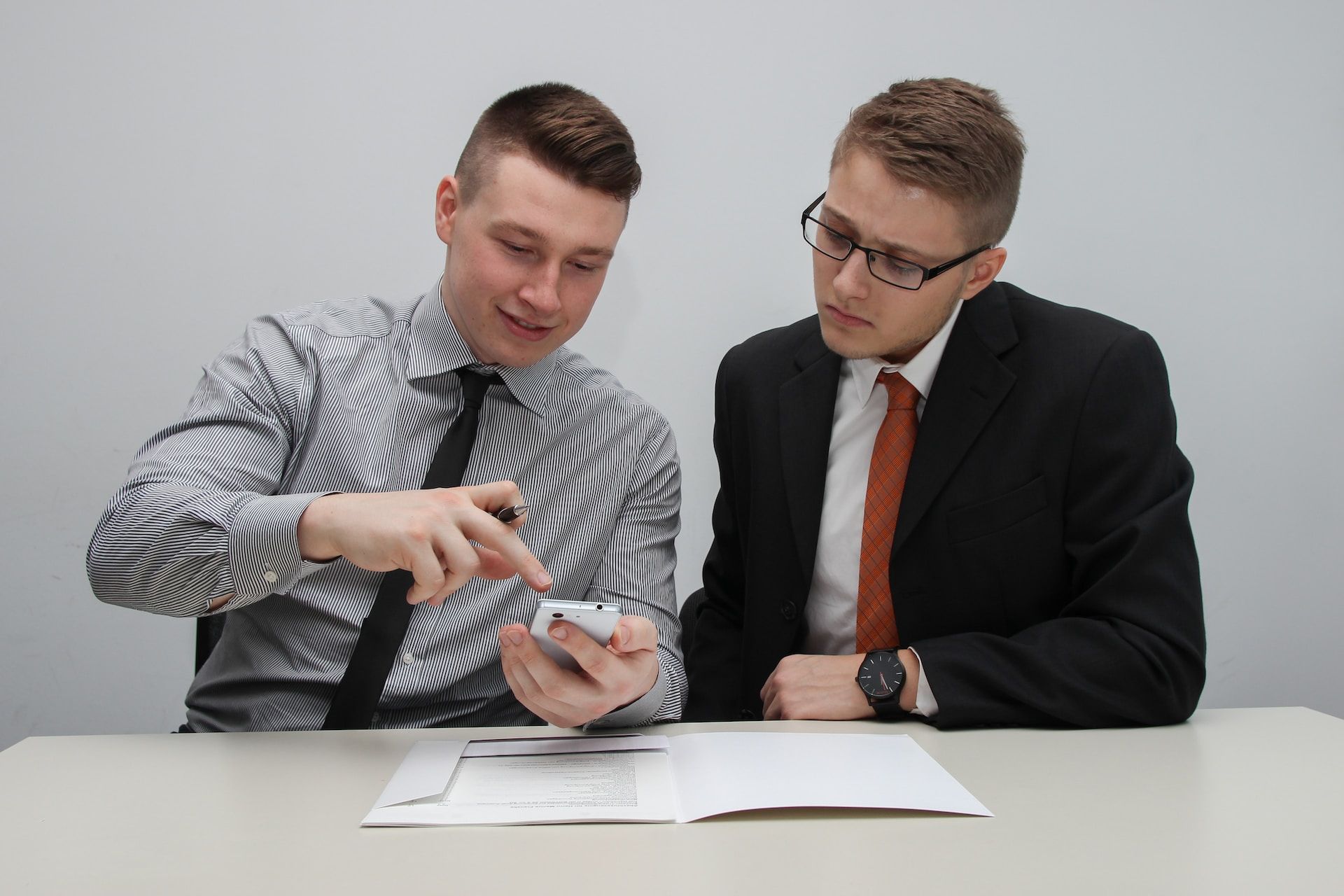
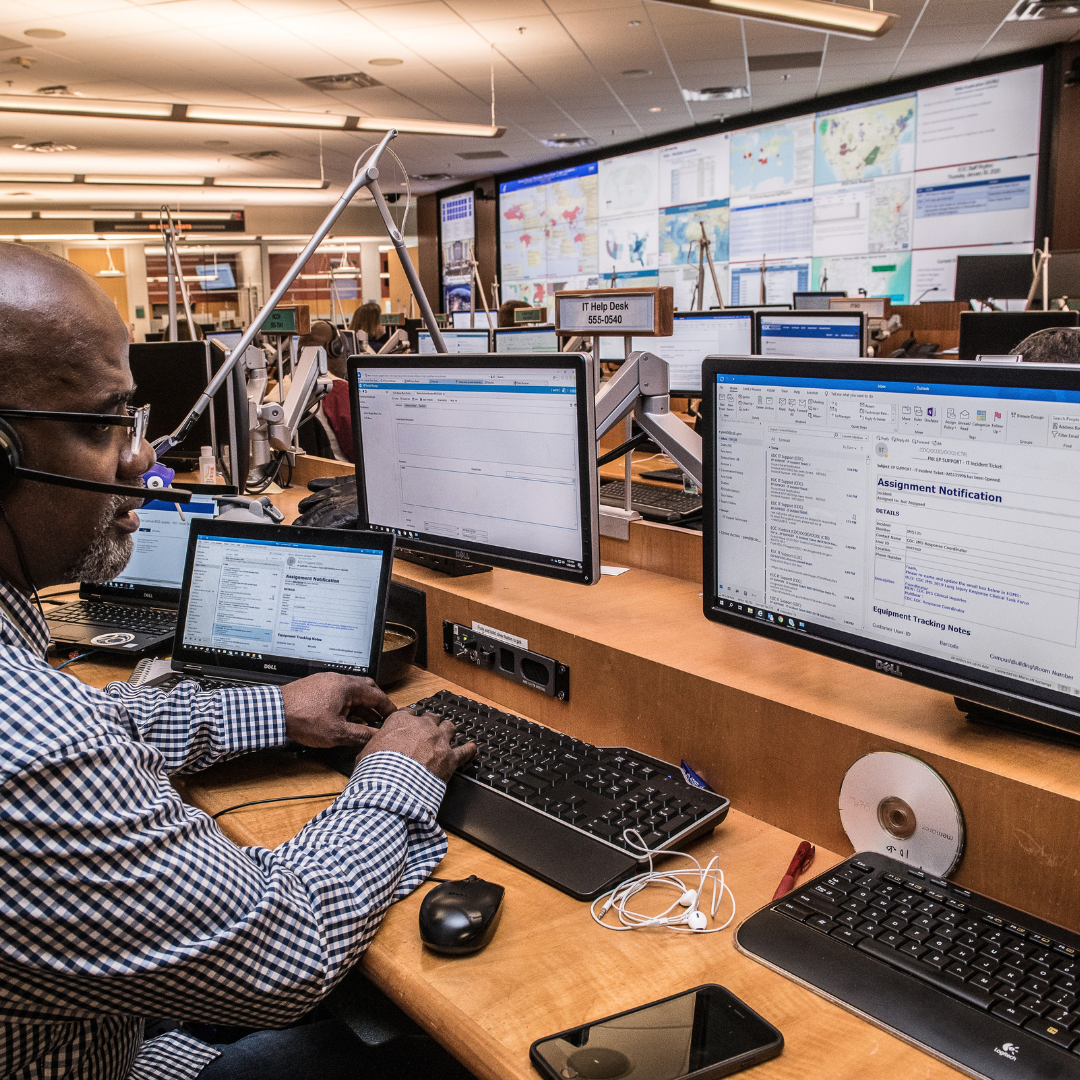
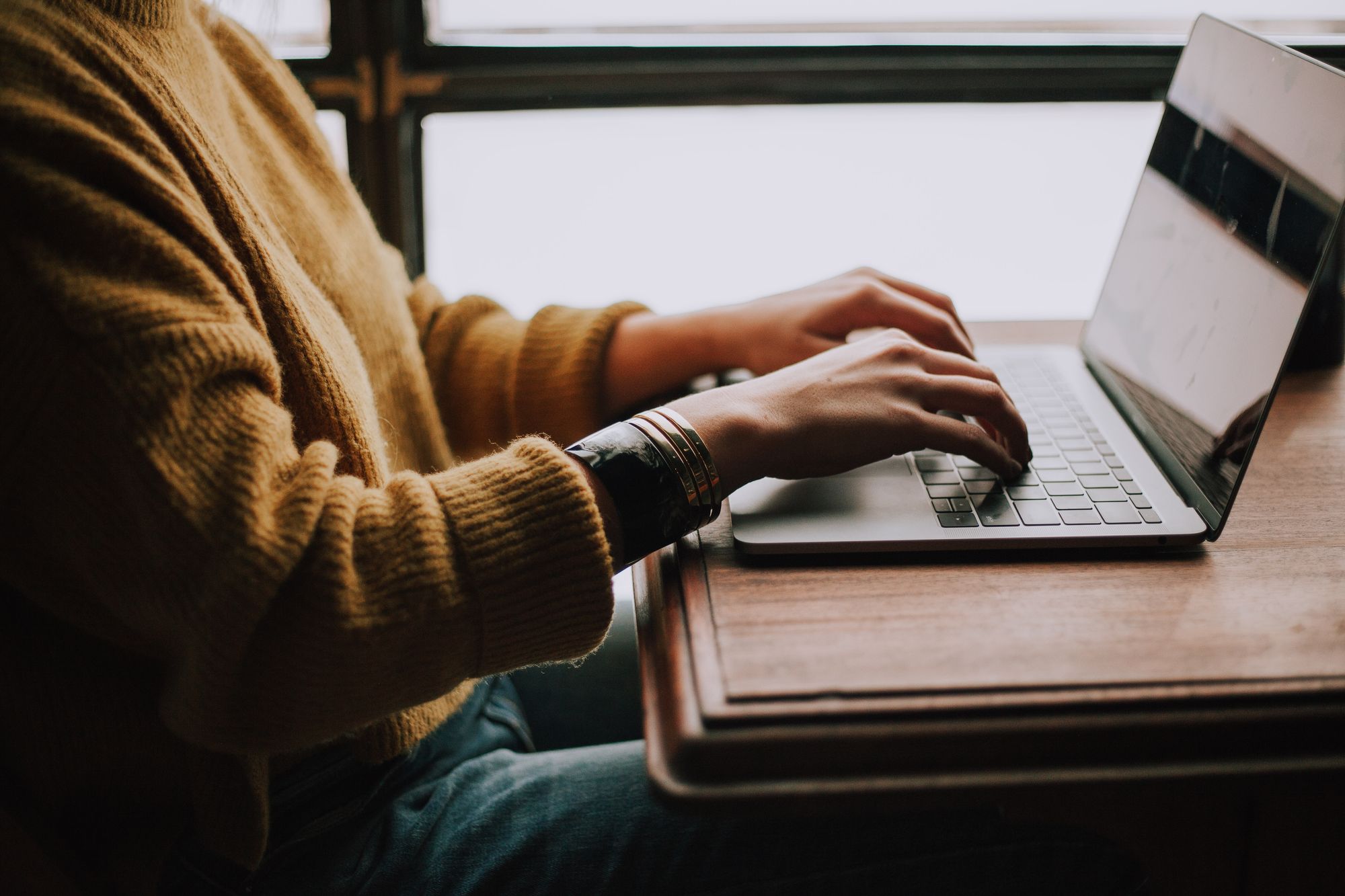