Effective demand forecasting is crucial for success in the manufacturing industry. Accurately predicting customer demand enables manufacturers to optimize their operations, minimize costs, and improve customer satisfaction.
However, there are common mistakes that manufacturers should avoid when forecasting demand. These mistakes can hinder the accuracy and effectiveness of the forecasting process, leading to suboptimal decisions and missed opportunities.
In this article, we will explore five common mistakes to avoid when forecasting demand in manufacturing. Let’s take a look at the table of content below:
- Meaning of Demand Forecasting Errors in Manufacturing?
- Causes of Demand Forecasting Errors
- Types of Demand Forecasting Errors
- Importance of Demand Forecasting in Manufacturing and its Impact
- 5 Common Mistakes to Avoid When Forecasting Demand in Manufacturing
- What are the Key Challenges in Demand Forecasting for Manufacturers?
- Benefits of Demand Forecasting in Manufacturing
- Wrapping Up
- How Deskera Can Assist You?
- Final Takeaways
Let's learn!
Meaning of Demand Forecasting Errors in Manufacturing?
Demand forecasting errors in manufacturing refer to the discrepancies between the predicted or forecasted demand for a product or service and the actual demand that occurs. These errors occur when the forecasted demand does not accurately align with the real-world demand experienced by the manufacturing company.
There are various types of demand forecasting errors that can occur in manufacturing:
Underestimation: This error happens when the forecasted demand is lower than the actual demand. As a result, the manufacturing company may not produce enough goods to meet customer demand, leading to stockouts, lost sales opportunities, and dissatisfied customers.
Overestimation: On the contrary, overestimation occurs when the forecasted demand is higher than the actual demand. This error can lead to excess inventory levels, increased storage costs, potential obsolescence, and financial losses for the manufacturing company.
Seasonality Errors: Seasonal demand forecasting errors occur when the predicted demand does not accurately capture the seasonal fluctuations in customer demand. If the forecasting models fail to account for the seasonality of certain products, the manufacturing company may face stockouts during peak periods or have excessive inventory during off-peak periods.
Random Errors: Random errors in demand forecasting are unpredictable and can occur due to unforeseen events, market fluctuations, or other external factors that affect demand. These errors are challenging to anticipate and can lead to deviations between the forecasted and actual demand.
Demand forecasting errors in manufacturing can have significant implications for a company's operations, inventory management, and financial performance.
Moreover, it is essential for manufacturing companies to continuously evaluate and improve their forecasting methods, consider historical data, market trends, and customer feedback, and incorporate the latest technologies, such as machine learning and artificial intelligence, to minimize these errors and enhance their forecasting accuracy.
By reducing demand forecasting errors, manufacturers can optimize production levels, improve customer satisfaction, and make informed decisions regarding resource allocation, procurement, and supply chain management.
Causes of Demand Forecasting Errors
Demand forecasting errors can occur due to a variety of factors. Understanding the causes of these errors is crucial for improving the accuracy of demand forecasts. Here are some common causes of demand forecasting errors in manufacturing:
Insufficient or Inaccurate Data:
Demand forecasting relies heavily on historical data, including sales records, customer behavior patterns, and market trends. If the available data is incomplete, outdated, or of poor quality, it can lead to forecasting errors.
Furthermore, insufficient data can result from limited data collection methods, inadequate data storage, and management systems, or gaps in data collection processes. Additionally, using inaccurate data or failing to account for data outliers can also contribute to forecasting errors.
Lack of Consideration for External Factors:
Demand forecasts should not solely rely on internal data but should also consider external factors that influence customer demand. Failure to account for external factors such as changes in the economy, market trends, competitive activity, and regulatory changes can lead to inaccurate forecasts. Ignoring these factors can result in missed opportunities or failure to anticipate shifts in customer preferences and market dynamics.
Inappropriate Forecasting Methods or Models:
Using inappropriate forecasting methods or models can also lead to errors. Different forecasting techniques, such as time series analysis, regression analysis, or machine learning algorithms, have different strengths and weaknesses.
Choosing the wrong method for a specific situation or applying a model that is not suited to the available data can result in inaccurate forecasts. It is essential to select the most appropriate forecasting method based on the nature of the data and the specific characteristics of the demand being forecasted.
Lack of Collaboration and Communication:
Forecasting errors can arise from a lack of collaboration and communication among stakeholders involved in the forecasting process. If key departments, such as sales, marketing, and supply chain, are not involved in providing inputs or validating the forecasts, critical insights may be missed. Collaboration and effective communication ensure that diverse perspectives, market intelligence, and ground-level information are considered, leading to more accurate forecasts
Failure to Adapt and Update Forecasting Models:
Demand forecasting is an ongoing process that requires continuous monitoring and adjustment. Failure to update forecasting models to reflect changes in market conditions, customer behavior, or internal business dynamics can result in forecasting errors. Demand patterns may evolve over time, and forecasting models should be regularly reviewed, refined, and recalibrated to reflect the latest trends and data.
Unforeseen Events and Volatility:
External events such as natural disasters, pandemics, economic crises, or sudden shifts in customer behavior can introduce volatility and unpredictability into demand patterns. These unforeseen events can significantly impact forecasting accuracy, as they may disrupt historical trends or introduce new demand dynamics that were not anticipated. Incorporating scenario planning and risk assessment into the forecasting process can help mitigate the impact of these unpredictable events.
Addressing these causes of demand forecasting errors requires a combination of robust data management, appropriate forecasting methods, stakeholder collaboration, continuous monitoring, and adaptability. By identifying and addressing these causes, manufacturers can improve the accuracy of their demand forecasts and make more informed decisions about production planning, inventory management, and customer satisfaction.
Types of Demand Forecasting Errors
Demand forecasting errors can occur due to various factors and can have significant consequences for manufacturing operations. Here are some common types of demand forecasting errors:
Underestimation Errors:
Underestimation errors occur when the forecasted demand is lower than the actual demand. This can lead to situations where manufacturers are unable to meet customer demand, resulting in stockouts, lost sales, and dissatisfied customers.
Additioanlly, underestimation errors can occur due to factors such as inaccurate historical data analysis, failure to consider external market factors, or not accounting for unexpected changes in customer behavior.
Overestimation Errors:
Overestimation errors happen when the forecasted demand exceeds the actual demand. This can lead to excess inventory, carrying costs, and potential wastage. Overestimation errors can arise from factors such as overreliance on historical data without considering changing market dynamics, failure to incorporate seasonality or cyclical patterns, or improper understanding of customer preferences.
Bias Errors:
Bias errors occur when there is a consistent overestimation or underestimation of demand over time. These errors can lead to long-term imbalances in production planning and inventory management.
Further, bias errors can be caused by factors such as inherent biases in forecasting models, incomplete or inadequate data, or a lack of continuous monitoring and adjustment of forecasting models.
Random Errors:
Random errors are unpredictable variations between forecasted and actual demand. These errors can result from factors such as unforeseen events, market volatility, or inaccurate assumptions about customer behavior.
While random errors are difficult to eliminate completely, they can be minimized through the use of statistical techniques and by continuously refining forecasting models based on real-time data and feedback.
Systematic Errors:
Systematic errors occur when there are consistent inaccuracies in the forecasting process. These errors can be caused by flawed methodologies, outdated models, or improper consideration of external factors.
Furthermore, systematic errors can have a long-lasting impact on manufacturing operations and can lead to inefficiencies, excess costs, and missed business opportunities. It is crucial to identify and rectify systematic errors through regular evaluation and improvement of forecasting techniques and models.
Addressing and minimizing these types of demand forecasting errors requires a combination of robust data analysis, consideration of external factors, the use of advanced forecasting techniques, and continuous monitoring and adjustment. By understanding these potential errors, manufacturers can enhance the accuracy and reliability of their demand forecasts, leading to improved operational efficiency, optimized inventory management, and increased customer satisfaction.
5 Common Mistakes to Avoid When Forecasting Demand in Manufacturing
Following, we’ve discussed some important common mistakes that you need to avoid when forecasting demand in manufacturing. Let’s learn:
1. Neglecting Historical Data Analysis
Neglecting historical data analysis is a common mistake in demand forecasting that can significantly impact the accuracy of forecasts. Here's why analyzing past sales and demand patterns is crucial and how it helps in identifying seasonal variations, demand trends, and potential anomalies:
Significance of Analyzing Historical Data:
Analyzing historical data provides valuable insights into past sales patterns, customer behavior, and market trends. It serves as a foundation for understanding the dynamics of demand and forms the basis for forecasting future demand accurately. Here are the key reasons why historical data analysis is significant:
Seasonal Variations:
Historical data analysis helps identify seasonal variations in demand. Many industries experience fluctuations in demand based on factors like holidays, weather conditions, or cultural events. By analyzing historical data, manufacturers can detect recurring patterns and adjust their forecasts accordingly. This enables them to optimize production and inventory levels to meet expected demand during peak and off-peak seasons.
Demand Trends:
Analyzing historical data allows manufacturers to identify long-term demand trends. It helps them understand how demand has evolved over time, whether it's increasing, decreasing, or remaining stable. Recognizing demand trends is vital for making informed decisions regarding production capacity, resource allocation, and product development. It also assists in predicting future growth or decline in demand, enabling manufacturers to align their strategies accordingly.
Potential Anomalies:
Historical data analysis helps uncover potential anomalies or irregularities in demand. These anomalies could be caused by various factors such as one-time events, changes in market conditions, or outliers in customer behavior. By examining historical data, manufacturers can identify these anomalies and assess their impact on future demand forecasts.
This knowledge allows them to make necessary adjustments to the forecasting models, account for unusual circumstances, and improve the accuracy of their predictions.
Mistake of Disregarding Historical Data:
Disregarding historical data due to assumptions of market volatility or changing trends is a significant mistake in demand forecasting. Here's why:
Hidden Insights:
Historical data contains valuable insights that might not be immediately apparent. Dismissing it without thorough analysis can lead to missed opportunities for understanding customer preferences, detecting demand patterns, or identifying potential risks and challenges. Even in volatile or rapidly changing markets, historical data can provide a foundation for making informed forecasts and strategic decisions.
Validation of Assumptions:
Assumptions of market volatility or changing trends should be validated using historical data analysis. While markets can be unpredictable, historical data can reveal patterns, cycles, and trends that persist despite short-term fluctuations. Analyzing historical data allows manufacturers to evaluate the validity of their assumptions and adjust their forecasting models accordingly.
Baseline for Comparison:
Historical data serves as a baseline for comparison when assessing the accuracy of forecasts. By comparing forecasted demand with actual demand, manufacturers can measure the effectiveness of their forecasting models and make necessary improvements. Disregarding historical data deprives manufacturers of this crucial benchmark for evaluating their forecasting performance.
In conclusion, neglecting historical data analysis is a common mistake in demand forecasting. It is essential to recognize the significance of historical data in identifying seasonal variations, demand trends, and potential anomalies. By leveraging historical data, manufacturers can improve the accuracy of their forecasts, optimize production and inventory planning, and make more informed business decisions.
2. Ignoring External Factors
Ignoring external factors in demand forecasting is a significant error that can lead to inaccurate predictions and hinder effective decision-making. Here's why solely relying on internal data without considering external factors is problematic, the impact of external factors, and the importance of integrating them into demand forecasting models:
Error of Solely Relying on Internal Data:
Solely relying on internal data without considering external factors limits the scope and accuracy of demand forecasting. Internal data, such as historical sales records or customer data, provides insights into past performance but may not capture the full picture of market dynamics. Ignoring external factors can result in missed opportunities, incorrect demand projections, and inadequate preparation for changing market conditions.
Impact of External Factors:
External factors significantly influence customer demand and market trends. Here are some key external factors that impact demand forecasting:
Economic Conditions:
Changes in the overall economic climate, including factors like GDP growth, inflation rates, employment levels, and consumer confidence, have a direct impact on customer purchasing power and demand. Failing to consider economic conditions can lead to inaccurate forecasts, especially during economic downturns or periods of rapid growth.
Industry Trends:
Understanding industry-specific trends is vital for accurate demand forecasting. Factors such as emerging technologies, evolving customer preferences, industry regulations, and new market entrants can shape demand patterns. Ignoring these trends may result in missed opportunities or inadequate preparation for shifts in customer demand.
Market Competition:
Competitive dynamics greatly influence demand. Factors such as pricing strategies, product launches, promotional activities, and market share of competitors impact customer preferences and purchasing behavior. Neglecting competition can lead to inaccurate demand forecasts and inadequate response to market challenges.
Regulatory Changes:
Changes in regulations, standards, or legal requirements can significantly impact demand for certain products or industries. Ignoring regulatory changes can lead to incorrect demand forecasts, compliance issues, and potential disruptions to supply chains.
Importance of Integrating External Data Sources:
Integrating external data sources into demand forecasting models enhances accuracy and responsiveness. Here's why it is crucial:
Holistic View of the Market:
External data provides a broader perspective on market dynamics, enabling manufacturers to capture the full range of factors influencing demand. By incorporating external data sources, such as market research reports, industry publications, or economic indicators, manufacturers can better understand market trends, anticipate shifts in demand, and align their forecasts accordingly.
Improved Forecasting Accuracy:
Considering external factors allows manufacturers to refine their demand forecasting models and incorporate additional variables. By leveraging external data sources, such as economic forecasts, competitor analysis, or social media sentiment analysis, manufacturers can improve the accuracy of their predictions and enhance the reliability of their forecasts.
Enhanced Adaptability:
External factors are often dynamic and subject to rapid changes. Integrating external data sources into demand forecasting models enables manufacturers to adapt quickly to shifting market conditions. This adaptability helps them respond to emerging trends, mitigate risks, and seize opportunities.
Better Strategic Decision-Making:
Incorporating external factors into demand forecasting provides a foundation for informed decision-making. By understanding the external environment, manufacturers can make strategic choices regarding production capacity, resource allocation, pricing strategies, and new product development. This leads to more effective planning, improved customer satisfaction, and increased competitiveness.
In conclusion, ignoring external factors in demand forecasting is an error that can hinder accuracy and strategic decision-making. By considering economic conditions, industry trends, market competition, and regulatory changes, manufacturers can enhance the reliability of their forecasts, optimize resource allocation, and better respond to market dynamics. Integrating external data sources into demand forecasting models ensures a holistic view of the market and improves overall forecasting performance.
3. Lack of Collaboration and Communication
Excluding key stakeholders, such as sales teams, marketing personnel, and supply chain managers, from the demand forecasting process is a mistake that can hinder the accuracy and effectiveness of forecasting. Here's why collaboration and communication are crucial, the benefits they bring, and the significance of establishing a feedback loop:
Mistake of Excluding Key Stakeholders:
Demand forecasting involves multiple functions within an organization, and excluding key stakeholders can lead to incomplete or biased forecasts. Each department holds valuable insights and data that contribute to a comprehensive understanding of customer demand. Failing to involve stakeholders like sales teams, marketing personnel, and supply chain managers can result in limited perspectives, missed opportunities, and inaccurate forecasts
Benefits of Cross-Functional Collaboration and Communication:
Cross-functional collaboration and effective communication play a pivotal role in demand forecasting. Here are the key benefits they bring:
Comprehensive Insights:
Each department holds unique insights and data about customer preferences, market trends, and supply chain capabilities. Collaborating and sharing information among stakeholders ensures that all relevant factors are considered, leading to a more comprehensive understanding of demand dynamics. This holistic approach allows for more accurate forecasting and better-informed decision-making.
Real-Time Market Intelligence:
Sales teams and marketing personnel are in direct contact with customers and have a deep understanding of their preferences, feedback, and purchasing behavior. By involving them in the forecasting process, manufacturers gain access to real-time market intelligence. This up-to-date information helps identify emerging trends, changing customer needs, and market opportunities, enhancing the accuracy of demand forecasts.
Enhanced Data Accuracy:
Effective collaboration facilitates the sharing of data and ensures its accuracy. By involving different stakeholders, inconsistencies or errors in data can be identified and rectified promptly. Collaborative efforts also enable the integration of diverse data sources, improving the quality and reliability of the data used in demand forecasting models.
Improved Forecasting Accuracy:
Cross-functional collaboration allows for a more holistic and accurate demand forecasting process. By leveraging the collective knowledge and expertise of various departments, manufacturers can capture a more comprehensive picture of demand drivers, potential risks, and opportunities. This leads to improved forecasting accuracy and minimizes the chances of overlooking critical factors that can impact demand.
Significance of Establishing a Feedback Loop:
Establishing a feedback loop is crucial for continuous improvement in the demand forecasting process. Here's why it is significant:
Continuous Learning:
A feedback loop enables continuous learning and improvement. By collecting feedback and insights from various departments, manufacturers can identify areas of improvement in their forecasting models, methodologies, and data collection processes. This iterative approach ensures that the forecasting process evolves over time, becoming more accurate and reliable.
Timely Adjustments:
A feedback loop allows for timely adjustments to forecasts based on real-time information and market changes. By regularly updating and refining forecasts based on inputs from stakeholders, manufacturers can adapt their production plans, inventory management, and resource allocation to align with changing demand patterns.
Alignment of Strategies:
Effective communication and feedback help align strategies across departments. This alignment ensures that forecasts are consistent with sales targets, marketing plans, and supply chain capabilities. It facilitates coordination and enables cross-functional teams to work together towards common goals, maximizing operational efficiency and customer satisfaction.
In conclusion, excluding key stakeholders from the demand forecasting process is a mistake that can impact the accuracy and effectiveness of forecasts. Cross-functional collaboration and effective communication bring numerous benefits, including comprehensive insights, real-time market intelligence, enhanced data accuracy, and improved forecasting accuracy.
Establishing a feedback loop ensures continuous learning, timely adjustments, and strategic alignment. By involving key stakeholders and establishing effective collaboration channels, manufacturers can enhance the accuracy of their demand forecasts and make more informed business decisions.
4. Overlooking Demand Segmentation:
Overlooking demand segmentation is an error that occurs when manufacturers treat all customers and products as a single entity without considering their inherent differences. Here's why demand segmentation is important, the benefits it brings, and how it enables targeted forecasting, improved inventory planning, and customized marketing strategies:
Error of Treating All Customers and Products as a Single Entity:
Treating all customers and products as a homogeneous group without segmenting demand overlooks the fact that different customers have varying preferences, behaviors, and purchasing patterns.
Similarly, products can have diverse characteristics, demand drivers, and market dynamics. Failing to recognize these differences can lead to inaccurate forecasts, suboptimal inventory management, and ineffective marketing strategies.
Benefits of Demand Segmentation:
Demand segmentation involves dividing customers and products into distinct segments based on specific criteria. Here are the key benefits it brings:
Targeted Forecasting:
Demand segmentation enables more precise forecasting by analyzing demand patterns within each segment. By considering factors such as customer preferences, geographic locations, product categories, and market segments, manufacturers can identify unique demand drivers and tailor their forecasts accordingly. This targeted approach improves the accuracy of predictions and allows for more efficient resource allocation and production planning.
Improved Inventory Planning:
Segmenting demand helps in optimizing inventory levels. Different customer segments and product categories may exhibit varying demand volatility, seasonality, or lead time requirements. By understanding these patterns, manufacturers can develop inventory strategies that align with specific segment needs. This leads to improved inventory turnover, reduced stockouts, and minimized carrying costs.
Customized Marketing Strategies:
Demand segmentation enables customized marketing strategies that resonate with specific customer segments. By understanding the preferences, behaviors, and needs of different segments, manufacturers can tailor their messaging, promotions, and product offerings. This targeted marketing approach enhances customer engagement, increases conversion rates, and fosters brand loyalty.
Identification of Growth Opportunities:
Demand segmentation helps identify untapped market segments or emerging trends. By analyzing customer segments that exhibit higher growth rates, increasing demand, or unique requirements, manufacturers can uncover new business opportunities.
Furthermore, this insight allows for strategic investments, product development, and market expansion strategies targeted at specific segments, driving overall business growth.
Enhanced Customer Satisfaction:
By segmenting demand, manufacturers can better understand the specific needs and preferences of different customer groups. This understanding allows for the development of customer-centric strategies, personalized experiences, and tailored solutions. Meeting the unique demands of each segment enhances customer satisfaction, fosters long-term relationships, and increases customer loyalty.
In conclusion, overlooking demand segmentation is an error that can hinder forecasting accuracy, inventory management, and marketing effectiveness. Demand segmentation brings several benefits, including targeted forecasting, improved inventory planning, customized marketing strategies, identification of growth opportunities, and enhanced customer satisfaction.
By recognizing the inherent differences among customers and products and segmenting demand accordingly, manufacturers can optimize their operations, drive growth, and deliver value to diverse customer segments.
5. Failing to Adapt and Iterate:
Failing to adapt and iterate in demand forecasting is a mistake that occurs when manufacturers treat forecasting as a one-time task rather than an ongoing process. Here's why demand forecasting should be viewed as an iterative process, the importance of continuously monitoring forecast accuracy, and the benefits of leveraging advanced analytics techniques, machine learning, and AI for improved forecasting accuracy and responsiveness:
Mistake of Considering Demand Forecasting as a One-Time Task:
Considering demand forecasting as a one-time task can lead to stagnant and outdated forecasting models. Market dynamics, customer preferences, and external factors are constantly evolving, and a one-time forecast quickly becomes outdated. Failing to adapt and iterate based on real-time feedback can result in inaccurate forecasts, missed opportunities, and operational inefficiencies.
Importance of Continuously Monitoring Forecast Accuracy:
Continuous monitoring of forecast accuracy is essential for improving forecasting performance and adapting to changing market conditions. Here's why it is important:
Real-Time Feedback:
Monitoring forecast accuracy provides real-time feedback on the performance of forecasting models. By comparing forecasted demand with actual demand, manufacturers can identify any discrepancies or deviations. This feedback enables them to assess the effectiveness of their forecasting models and make necessary adjustments.
Identification of Trends and Patterns:
Continuous monitoring helps identify emerging trends and patterns that may impact demand. By analyzing forecast errors over time, manufacturers can uncover recurring patterns, seasonal variations, or shifts in customer behavior. This knowledge allows for the refinement of forecasting models to better capture these trends and improve forecast accuracy.
Proactive Decision-Making:
Monitoring forecast accuracy allows for proactive decision-making based on real-time information. When discrepancies are identified, manufacturers can take immediate action, such as adjusting production plans, revising inventory levels, or reallocating resources. This proactive approach helps in mitigating risks, minimize disruptions, and optimizing operations.
Benefits of Leveraging Advanced Analytics, Machine Learning, and AI:
Advanced analytics techniques, machine learning, and AI can significantly enhance demand forecasting accuracy and responsiveness. Here's how they bring benefits:
Improved Forecasting Accuracy:
Advanced analytics techniques, such as time series analysis, regression models, and predictive algorithms, can capture complex demand patterns and relationships more accurately.
Furthermore, machine learning and AI algorithms can identify nonlinear patterns, detect anomalies, and adapt to changing market conditions. By leveraging these technologies, manufacturers can achieve higher forecasting accuracy and reduce forecast errors.
Enhanced Responsiveness:
Advanced analytics, machine learning, and AI enable faster and more responsive forecasting. These techniques can process and analyze large volumes of data in real-time, enabling manufacturers to react quickly to changing market conditions or demand patterns. This agility helps in adjusting forecasts promptly, optimizing production plans, and improving customer satisfaction.
Intelligent Insights and Recommendations:
Machine learning and AI algorithms can provide intelligent insights and recommendations based on historical data, market trends, and external factors. These technologies can automatically identify relevant variables, uncover hidden relationships, and generate more accurate forecasts. They can also provide actionable recommendations for inventory management, pricing strategies, and resource allocation, helping manufacturers make data-driven decisions.
Scalability and Automation:
Advanced analytics, machine learning, and AI techniques allow for scalable and automated demand forecasting processes. These technologies can handle large and diverse datasets, automate data cleaning and preprocessing, and streamline the forecasting workflow. This scalability and automation reduce manual effort, increase efficiency, and enable manufacturers to focus on strategic decision-making.
In conclusion, failing to adapt and iterate in demand forecasting is a mistake that hampers accuracy and responsiveness. Demand forecasting should be viewed as an iterative process, with continuous monitoring of forecast accuracy and adjustments based on real-time feedback.
Leveraging advanced analytics techniques, machine learning, and AI brings benefits such as improved forecasting accuracy, enhanced responsiveness, intelligent insights, and automation. By embracing these technologies and adopting an iterative approach, manufacturers can optimize their demand forecasting capabilities, respond effectively to market changes, and drive operational excellence.
Importance of Demand Forecasting in Manufacturing and its Impact
Demand forecasting plays a crucial role in manufacturing as it helps organizations anticipate and plan for future customer demand. By accurately predicting demand, manufacturers can optimize their operations, streamline production processes, and ensure efficient inventory management.
Following, we’ve discussed some major key impacts of demand forecasting in manufacturing:
Operational Efficiency: Demand forecasting allows manufacturers to align their production capacities, labor resources, and equipment utilization with expected demand. By having a clear understanding of future demand patterns, manufacturers can plan their production schedules, optimize resource allocation, and minimize production bottlenecks. This leads to improved operational efficiency, reduced downtime, and better utilization of resources.
Inventory Management: Accurate demand forecasting helps manufacturers optimize their inventory levels. By knowing the expected demand for their products, manufacturers can avoid overstocking or understocking situations. Maintaining the right level of inventory helps reduce carrying costs, prevent stockouts, and minimize wastage. Effective demand forecasting also enables manufacturers to plan for raw material procurement, reducing lead times and improving supply chain efficiency.
Customer Satisfaction: Meeting customer demand is critical for maintaining high levels of customer satisfaction. By accurately forecasting demand, manufacturers can ensure product availability and timely delivery to customers.
This further prevents situations where customers have to face delays or unavailability of desired products. Improved customer satisfaction leads to repeat business, positive brand reputation, and increased customer loyalty.
Cost Reduction: Demand forecasting helps manufacturers optimize their production and inventory management processes, leading to cost savings. By avoiding excessive inventory holding costs, manufacturers can reduce storage expenses, write-offs, and obsolescence.
Additionally, efficient production planning based on accurate demand forecasts helps minimize production overruns, reduces waste, and optimizes resource utilization, resulting in cost efficiencies.
Market Adaptability: Demand forecasting enables manufacturers to adapt to market changes and shifts in customer preferences. By analyzing demand trends and customer behavior, manufacturers can identify emerging opportunities or potential risks in the market.
This allows them to proactively adjust their production plans, introduce new products, or modify existing ones to meet evolving customer demands. Staying agile and responsive to market dynamics helps manufacturers maintain a competitive edge and seize growth opportunities.
In summary, demand forecasting in manufacturing significantly impacts operational efficiency, inventory management, and customer satisfaction. It enables manufacturers to optimize resources, reduce costs, improve product availability, and adapt to changing market conditions, ultimately driving business success.
What are the Key Challenges in Demand Forecasting for Manufacturers?
Manufacturers face several challenges when it comes to demand forecasting. These challenges can impact the accuracy and effectiveness of their forecasting efforts. Here are some key challenges in demand forecasting for manufacturers:
Volatile Market Conditions: Market dynamics can be unpredictable, with changes in economic conditions, consumer behavior, and external factors. Fluctuations in market demand make it challenging to accurately forecast future demand, particularly in industries with rapid technological advancements or changing customer preferences.
Seasonality and Demand Variations: Many industries experience seasonal variations in demand, where demand levels fluctuate throughout the year. Predicting these variations accurately can be complex, requiring robust forecasting models that capture seasonal patterns, holidays, and other factors that influence demand.
Limited Data Availability: Accurate demand forecasting relies on historical data. However, there may be instances where historical data is limited or incomplete, especially for new products, emerging markets, or during times of significant industry or market changes. Insufficient data can make it difficult to establish reliable forecasting models.
External Factors: External factors such as regulatory changes, industry disruptions, and competitive actions can significantly impact demand. Manufacturers need to consider these external factors and their potential effects on demand patterns. Failure to account for these factors can lead to inaccurate forecasts and misaligned production plans.
Forecast Bias and Errors: Bias and errors can arise from various sources, including inaccurate assumptions, data inconsistencies, or human judgment. These biases and errors can lead to inaccurate demand forecasts and subsequent operational inefficiencies, such as excess inventory or stockouts.
Collaboration and Information Sharing: Demand forecasting should involve cross-functional collaboration and information sharing. However, coordinating and integrating inputs from different departments, such as sales, marketing, and supply chain, can be a challenge. Lack of collaboration can result in incomplete or biased forecasts, missing out on valuable insights from key stakeholders.
Forecasting New Products or Markets: Forecasting demand for new products or entering new markets presents additional challenges. Limited historical data, uncertainty about customer acceptance, and unknown market dynamics make it difficult to accurately predict demand. Manufacturers need to rely on market research, consumer insights, and iterative forecasting approaches to address these challenges.
Forecast Accuracy Monitoring: Assessing forecast accuracy and monitoring deviations from actual demand is crucial for improving forecasting models. However, tracking forecast accuracy can be challenging due to data availability, measuring the right performance metrics, and implementing effective feedback loops for continuous improvement.
Addressing these challenges requires manufacturers to adopt a combination of data-driven approaches, collaboration, advanced analytics, and continuous monitoring. By acknowledging these challenges and implementing strategies to mitigate them, manufacturers can enhance the accuracy and effectiveness of their demand forecasting processes.
Benefits of Demand Forecasting in Manufacturing
Demand forecasting in manufacturing brings several benefits to businesses. Here are some key advantages:
Production Planning and Efficiency: Accurate demand forecasts help manufacturers plan their production schedules more effectively. By knowing the expected demand, they can allocate resources, adjust production capacity, and optimize their manufacturing processes. This leads to improved efficiency, reduced wastage, and better utilization of resources.
Inventory Management: Demand forecasting plays a critical role in inventory management. By accurately predicting future demand, manufacturers can maintain optimal inventory levels. This helps in avoiding stockouts, minimizing excess inventory, reducing holding costs, and improving cash flow. Effective inventory management ensures that the right products are available when customers need them, resulting in increased customer satisfaction.
Supply Chain Optimization: Demand forecasting enables manufacturers to streamline their supply chain operations. With accurate forecasts, they can align procurement, production, and distribution activities to meet anticipated demand.
Furthermore, this helps in reducing lead times, improving logistics planning, and optimizing transportation and warehousing. A well-optimized supply chain enhances operational efficiency, reduces costs, and improves overall customer service.
Financial Planning and Budgeting: Demand forecasting provides valuable insights for financial planning and budgeting. By knowing the expected demand for their products, manufacturers can estimate revenue projections, plan their expenditures, and allocate budgets accordingly. This helps in effective resource allocation, avoiding cash flow constraints, and making informed financial decisions.
Customer Satisfaction and Retention: Demand forecasting helps manufacturers meet customer expectations more effectively. By accurately predicting demand, they can ensure product availability, timely delivery, and excellent customer service. Meeting customer demand leads to higher customer satisfaction, increased loyalty, and positive brand reputation.
Market Expansion and Growth Opportunities: Accurate demand forecasts can identify market trends, emerging opportunities, and new customer segments. This knowledge allows manufacturers to identify growth areas, develop new products or services, and expand into new markets. By proactively responding to market demand, manufacturers can seize growth opportunities and stay ahead of the competition.
Efficient Resource Allocation: Demand forecasting enables manufacturers to allocate resources efficiently. By having visibility into future demand, they can optimize labor, raw material procurement, machinery, and other resources. This helps in minimizing waste, reducing costs, and improving overall resource utilization.
In summary, demand forecasting in manufacturing provides numerous benefits, including improved production planning and efficiency, optimized inventory management, streamlined supply chain operations, enhanced financial planning, increased customer satisfaction, identification of growth opportunities, and efficient resource allocation. By leveraging accurate demand forecasts, manufacturers can make informed decisions, optimize their operations, and achieve sustainable business growth.
Wrapping Up
In conclusion, demand forecasting is a critical process in manufacturing that enables businesses to anticipate and plan for future customer demand. However, there are several common mistakes that manufacturers should avoid to ensure accurate and effective forecasting.
Neglecting historical data analysis can lead to missed opportunities to identify seasonal variations, demand trends, and anomalies. Ignoring external factors such as economic conditions, industry trends, and regulatory changes can result in inaccurate forecasts that do not align with market realities. Excluding key stakeholders from the forecasting process can limit valuable insights and hinder accuracy.
Treating all customers and products as a single entity without demand segmentation overlooks opportunities for targeted forecasting, optimized inventory planning, and customized marketing strategies.
Finally, considering demand forecasting as a one-time task rather than an iterative process can hinder the ability to adapt to market changes and leverage advanced analytics techniques.
By avoiding these mistakes and adopting best practices, manufacturers can improve operational efficiency, enhance inventory management, optimize resource allocation, and ultimately enhance customer satisfaction.
It is crucial to analyze historical data, integrate external factors, promote collaboration and communication, leverage demand segmentation, and embrace continuous adaptation and iteration in the forecasting process. Additionally, leveraging advanced analytics techniques, machine learning, and AI can enhance forecast accuracy and responsiveness to dynamic market conditions.
Demand forecasting should be viewed as a strategic and ongoing process that involves collaboration, data analysis, and continuous improvement. By recognizing and addressing the challenges in demand forecasting, manufacturers can make informed decisions, optimize their operations, and gain a competitive edge in the market. With accurate demand forecasts, manufacturers can align their production capabilities with customer needs, minimize risks, and seize growth opportunities.
How Deskera Can Assist You?
Deskera ERP and MRP systems help you to keep your business units organized. The system's key features of demand forecasting with Deskera include as follows:
- Establish Demand Forecasting Process
- Automate Data Collection
- Monitor Market Trends
- Analyze Historical Data
- Estimate Future Demand
- Adjust Production Levels
- Manage Supply Chain...and much more!
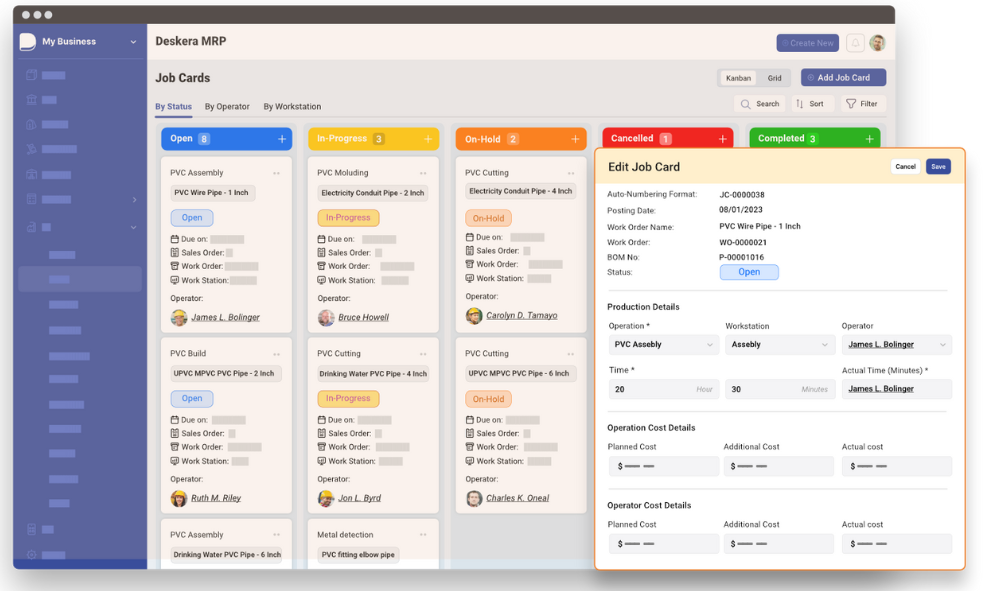
- Deskera Books allows you to better manage your accounts and finances. Maintain good accounting practices by automating tasks like billing, invoicing, and payment processing.
- Deskera's integrated financial planning tools enable investors to better plan and track their investments. It can assist investors in making faster and more accurate decisions.
- Deskera CRM is a powerful solution that manages your sales and helps you close deals quickly. It not only enables you to perform critical tasks like lead generation via email, but it also gives you a comprehensive view of your sales funnel.
- Deskera People is a straightforward tool for centralizing your human resource management functions.
Final Takeaways
We've arrived at the last section of this guide. Let's have a look at some of the most important points to remember:
- Demand forecasting errors in manufacturing refer to the discrepancies between the predicted or forecasted demand for a product or service and the actual demand that occurs. These errors occur when the forecasted demand does not accurately align with the real-world demand experienced by the manufacturing company.
- Underestimation errors occur when the forecasted demand is lower than the actual demand. This can lead to situations where manufacturers are unable to meet customer demand, resulting in stockouts, lost sales, and dissatisfied customers.
- Assumptions of market volatility or changing trends should be validated using historical data analysis. While markets can be unpredictable, historical data can reveal patterns, cycles, and trends that persist despite short-term fluctuations.
- Understanding industry-specific trends is vital for accurate demand forecasting. Factors such as emerging technologies, evolving customer preferences, industry regulations, and new market entrants can shape demand patterns.
- Incorporating external factors into demand forecasting provides a foundation for informed decision-making. By understanding the external environment, manufacturers can make strategic choices regarding production capacity, resource allocation, pricing strategies, and new product development.
- Sales teams and marketing personnel are in direct contact with customers and have a deep understanding of their preferences, feedback, and purchasing behavior. By involving them in the forecasting process, manufacturers gain access to real-time market intelligence. This up-to-date information helps identify emerging trends, changing customer needs, and market opportunities, enhancing the accuracy of demand forecasts.
- Demand segmentation enables more precise forecasting by analyzing demand patterns within each segment. By considering factors such as customer preferences, geographic locations, product categories, and market segments, manufacturers can identify unique demand drivers and tailor their forecasts accordingly.
Related Articles
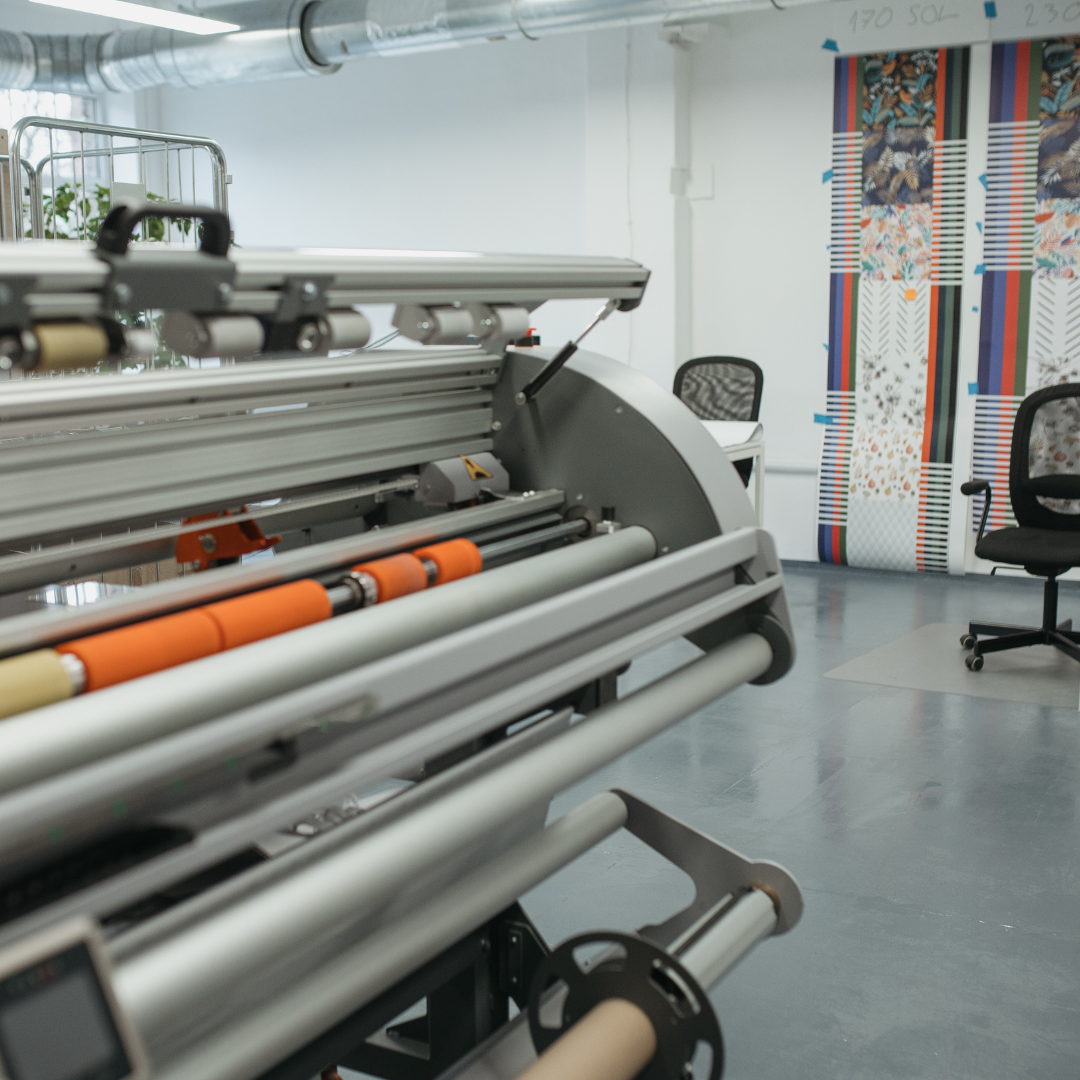
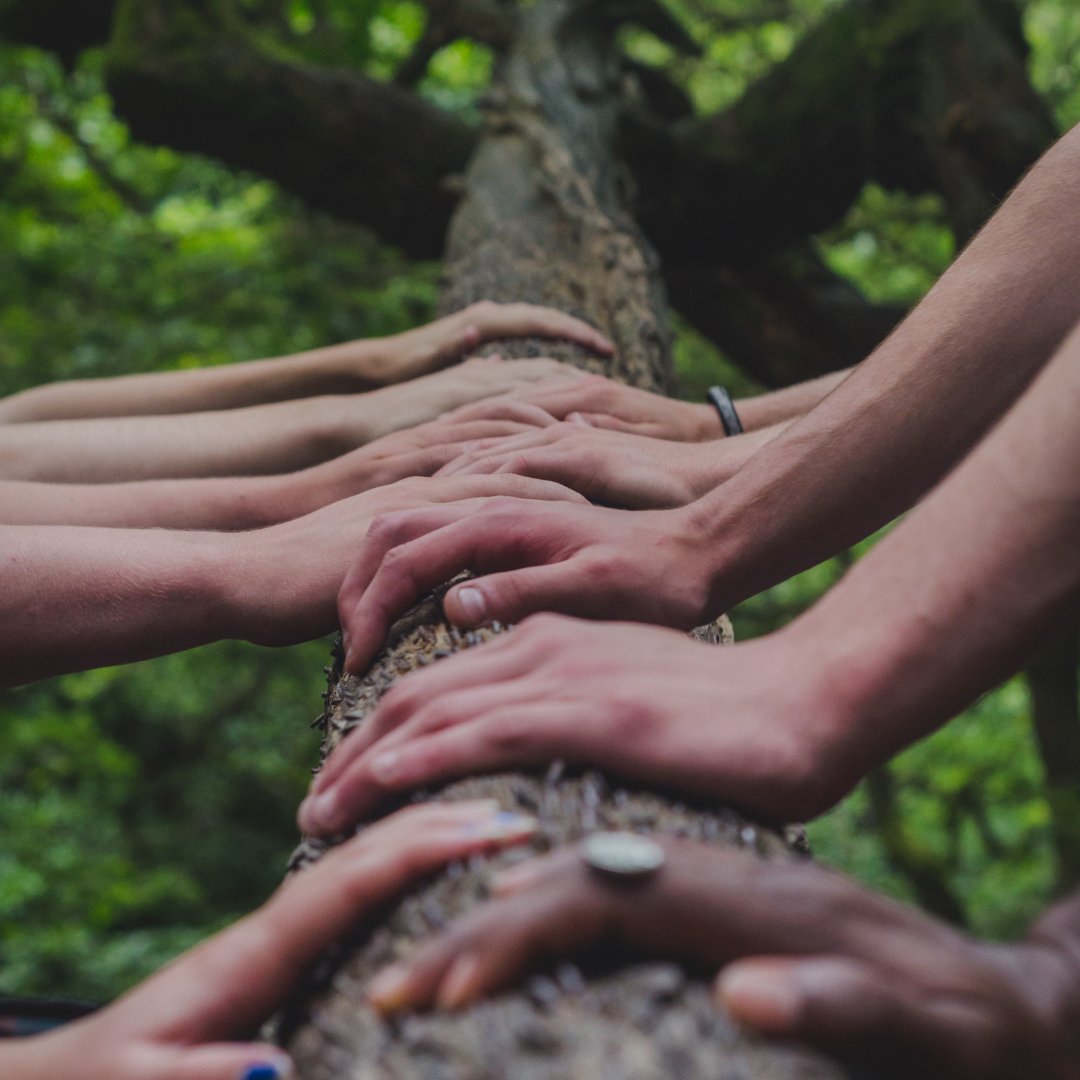
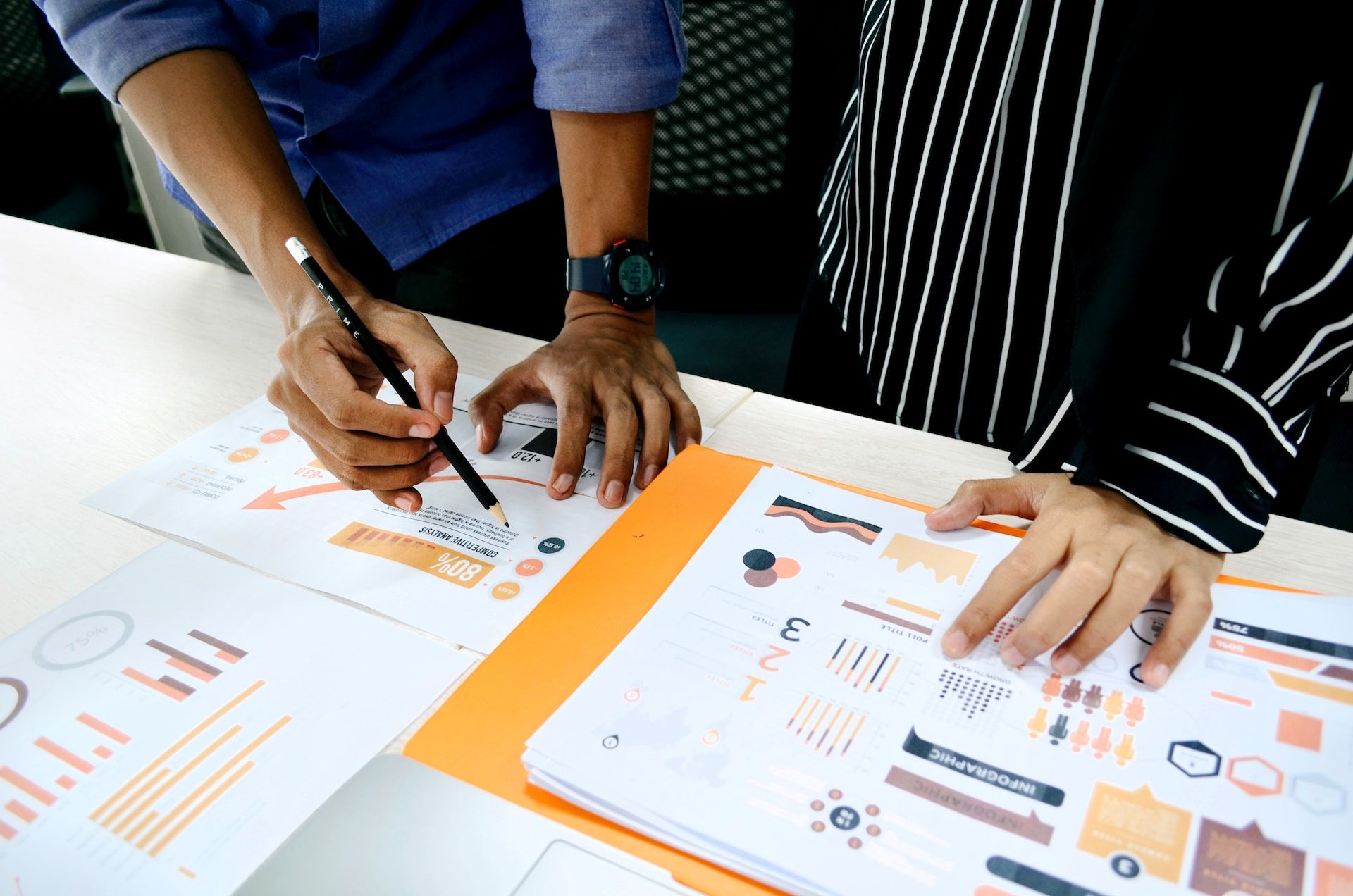
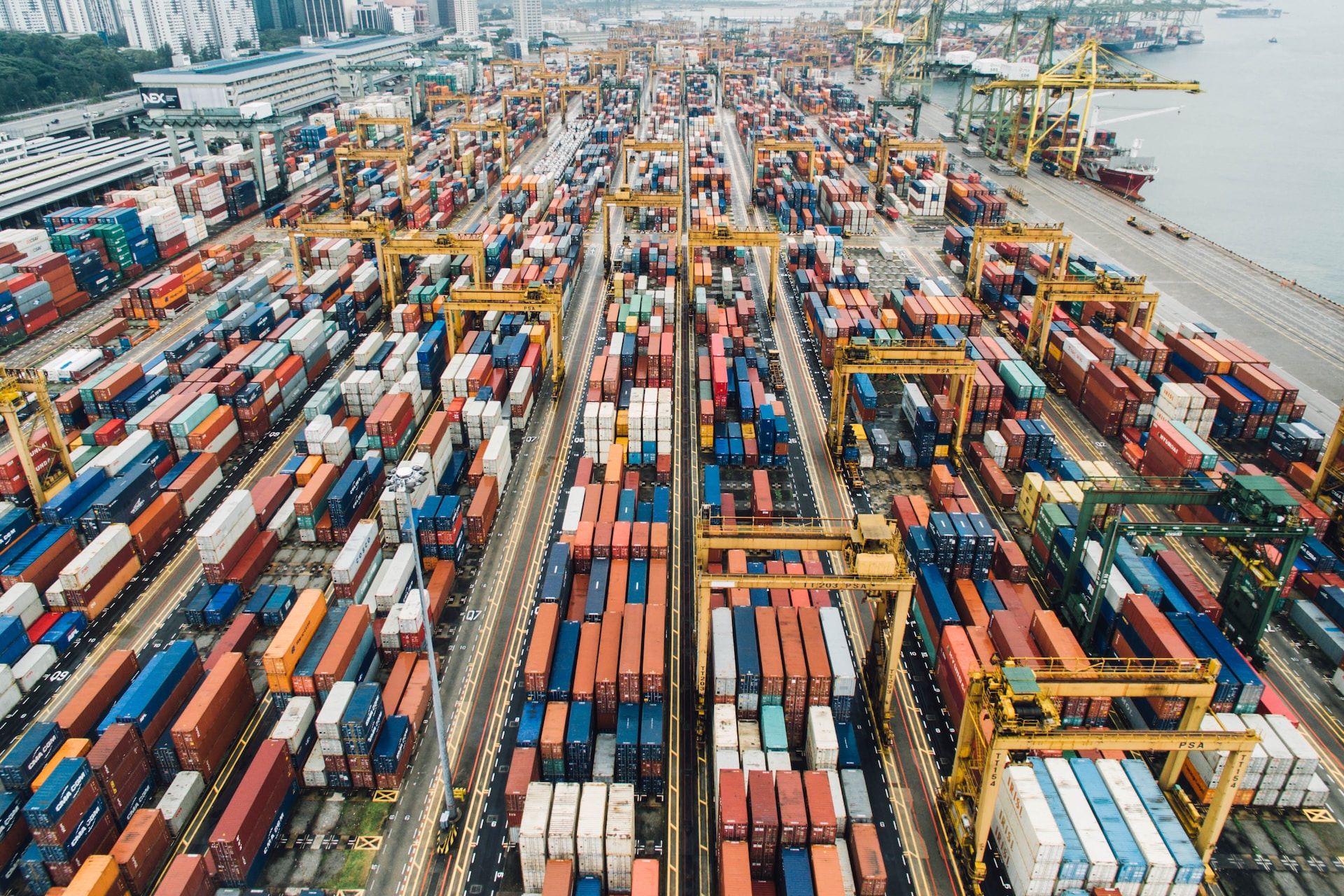
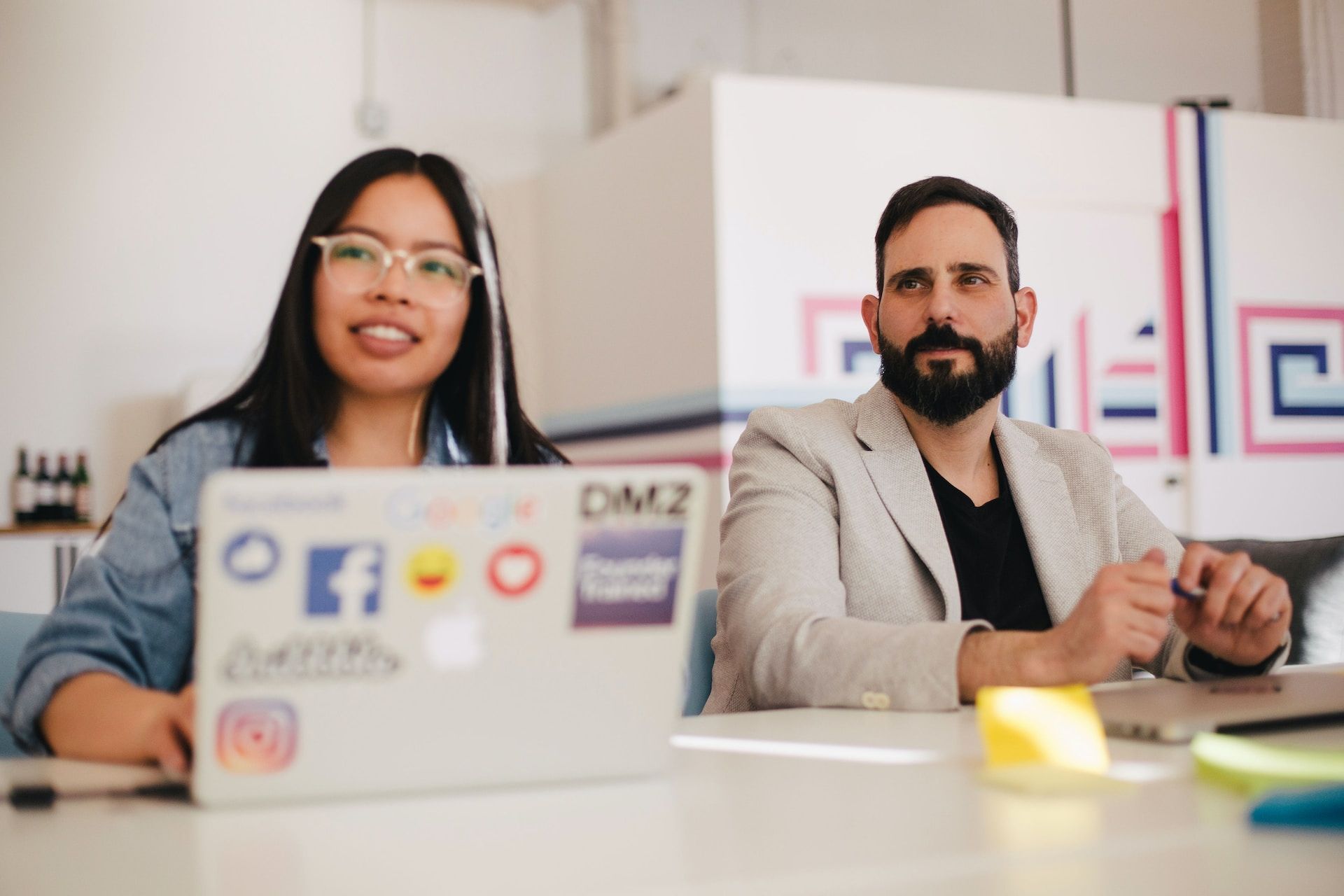