The intersection of sales forecasting and demand forecasting in manufacturing is a crucial nexus that drives operational efficiency and business success. Sales forecasting focuses on predicting future sales based on historical data, market trends, and customer behavior, providing valuable insights to guide sales strategies and revenue projections.
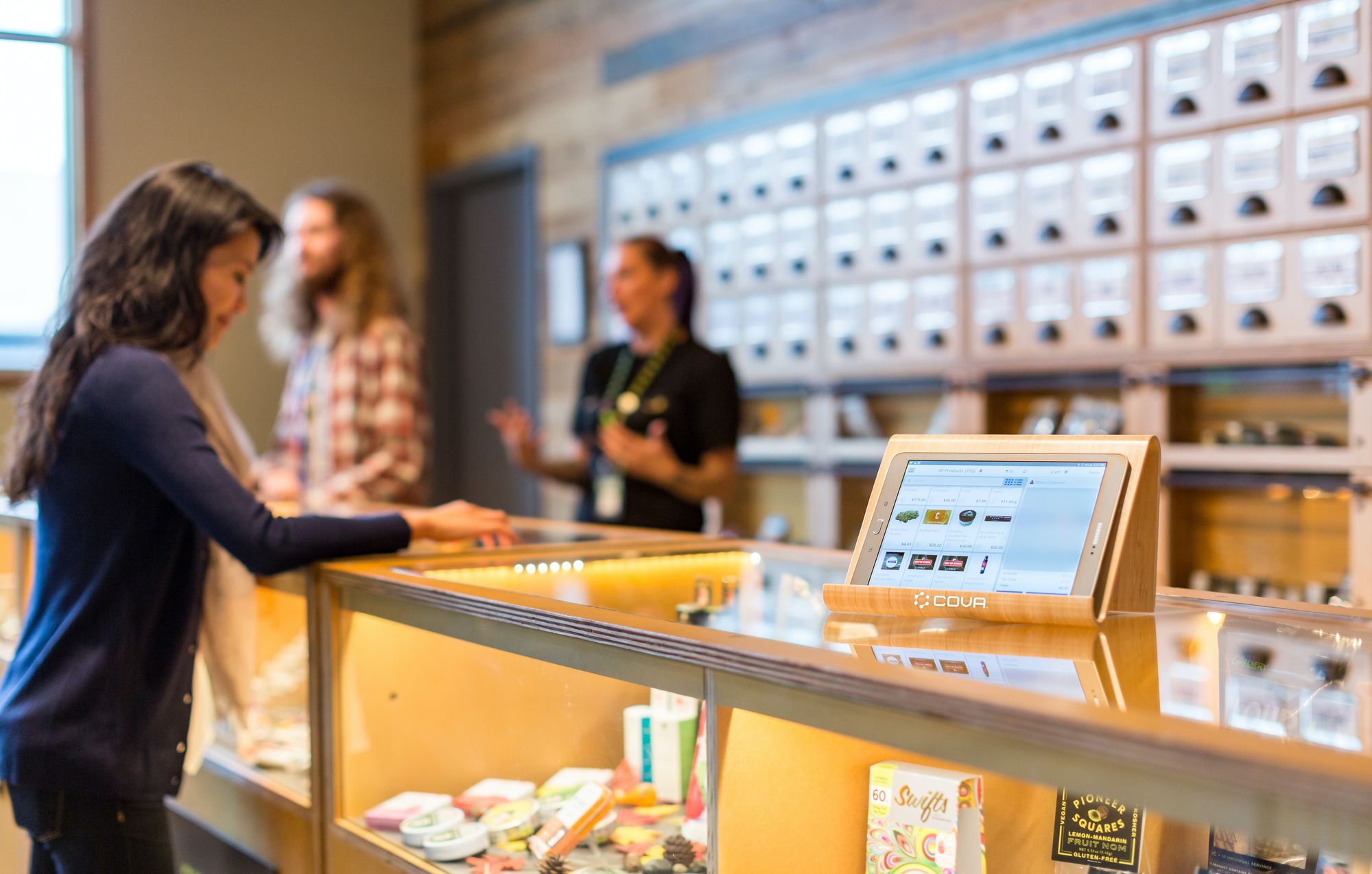
On the other hand, demand forecasting aims to anticipate the quantity and type of products customers will require, assisting manufacturing units in optimizing production schedules, inventory management, and resource allocation.
By integrating these two forecasting approaches, manufacturers can align production capabilities with anticipated market demand, minimize stockouts, reduce excess inventory costs, and enhance customer satisfaction through timely deliveries.
This symbiotic relationship fosters agile and responsive supply chains, ensuring companies can meet consumer needs while maintaining a competitive edge in the dynamic manufacturing landscape.
This article covers the following:
- Understanding Sales Forecasting and Demand Forecasting
- The Crucial Role of Forecasting in Manufacturing
- Differentiating Sales Forecasting from Demand Forecasting
- Sales Forecasting Techniques for Manufacturers
- Demand Forecasting Techniques for Manufacturers
- Data Integration: Bridging the Gap between Sales and Demand Forecasts
- Challenges in Forecasting for Manufacturers
- The Role of Technology in Forecasting
- Collaborative Forecasting
- Benefits of Accurate Forecasting in Manufacturing
- Real-Life Case Studies: Success Stories in Manufacturing Forecasting
- The Future of Forecasting in Manufacturing
- Best Practices for Integrating Sales and Demand Forecasting
- Mitigating Forecasting Risks: Strategies for Manufacturers
- The Human Factor: Balancing Intuition with Data-Driven Forecasting
Understanding Sales Forecasting and Demand Forecasting
Sales forecasting and demand forecasting are essential components of business planning and management. They involve predicting future sales and customer demand to make informed decisions about inventory management, production levels, resource allocation, and overall business strategy. Let's explore each concept in detail:
Sales Forecasting: Sales forecasting is the process of estimating the future sales of a product, service, or an entire business for a specific period, typically a month, quarter, or year. The goal of sales forecasting is to project the revenue a company expects to generate based on historical sales data, market trends, and other relevant factors.
Key aspects of sales forecasting:
a. Historical Data: Sales data from past periods is analyzed to identify trends, seasonality, and other patterns that can help in making future predictions.
b. Market Analysis: Understanding the market dynamics, consumer behavior, and competitors' activities can provide insights into potential demand for the product or service.
c. External Factors: Economic conditions, industry trends, changes in regulations, and other external factors can influence sales, and they need to be taken into account.
d. Internal Factors: Factors like marketing initiatives, new product launches, pricing changes, and distribution strategies can impact sales performance.
e. Statistical Models: Various quantitative methods, such as time-series analysis, regression analysis, and machine learning algorithms, may be used to create sales forecasts.
f. Collaboration: Sales teams, marketing teams, and other relevant stakeholders should collaborate to provide valuable input for a more accurate forecast.
Demand Forecasting: Demand forecasting is a broader term that refers to the estimation of customer demand for products or services. It encompasses not only sales but also the entire demand-supply chain, including raw materials, production capacity, and inventory levels.
Key aspects of demand forecasting:
a. Sales Forecasting: Predicting future sales is one part of demand forecasting, as it focuses on the final output of the supply chain.
b. Supply Chain Management: Demand forecasting helps businesses optimize their supply chain operations by aligning production, inventory, and distribution with projected demand.
c. Inventory Management: Accurate demand forecasts aid in maintaining optimal inventory levels, reducing excess inventory costs or stockouts.
d. Production Planning: Manufacturers use demand forecasts to plan their production schedules and avoid underutilization or overutilization of resources.
e. Resource Allocation: Businesses can allocate resources effectively based on anticipated demand, which may include human resources, raw materials, and capital.
f. New Product Planning: When launching new products, demand forecasting can assist in estimating potential sales and planning production accordingly.
Both sales forecasting and demand forecasting are vital for businesses to make informed decisions, especially in industries with seasonality, fluctuating demand, and dynamic market conditions.
Accurate forecasting enables businesses to minimize risks, optimize operations, and remain competitive in their respective markets. However, it's essential to recognize that forecasting is not an exact science and may be subject to unforeseen events or changes in the market, so regular reviews and adjustments are necessary.
The Crucial Role of Forecasting in Manufacturing
Forecasting plays a crucial role in manufacturing for various reasons. It involves predicting future demands, trends, and events to help manufacturers make informed decisions and optimize their production processes. Here are some key aspects that highlight the importance of forecasting in manufacturing:
- Demand Planning: Accurate forecasting allows manufacturers to anticipate customer demand and plan their production schedules accordingly. By understanding future demand patterns, they can avoid stockouts, reduce excess inventory, and optimize their supply chain to ensure products are available when needed.
- Production Planning and Scheduling: Forecasts help manufacturers determine the required production capacity and allocate resources efficiently. With this information, they can create production schedules that minimize downtime, reduce lead times, and improve overall production efficiency.
- Inventory Management: Overstocking or understocking can lead to significant financial losses. Forecasting aids in maintaining optimal inventory levels by predicting demand fluctuations and preventing excess stock or shortages. This, in turn, minimizes carrying costs and maximizes inventory turnover.
- Resource Allocation: Manufacturing involves various resources such as labor, raw materials, and equipment. Forecasting helps manufacturers allocate these resources effectively, avoiding wastage and ensuring smooth operations during peak demand periods.
- Supply Chain Optimization: Manufacturers often rely on complex supply chains with multiple suppliers and distributors. Forecasting enables better coordination and collaboration across the supply chain, reducing lead times and enhancing overall efficiency.
- New Product Introductions: When launching new products or variants, forecasting provides insights into potential market acceptance and demand. This helps manufacturers avoid overproduction of untested products and minimizes financial risks.
- Cost Reduction: Efficient forecasting can lead to cost reductions in multiple areas. By aligning production with demand, manufacturers can minimize production and storage costs. Additionally, better inventory management and reduced wastage contribute to cost savings.
- Risk Management: Forecasts help manufacturers anticipate potential risks and uncertainties, such as changes in market conditions or supply chain disruptions. With this information, they can develop contingency plans to mitigate the impact of adverse events.
- Budgeting and Financial Planning: Reliable forecasts are vital for developing realistic budgets and financial plans. Manufacturers can allocate funds appropriately for production, marketing, and other expenses based on anticipated sales and revenue.
- Continuous Improvement: By analyzing past forecasting accuracy, manufacturers can learn from their successes and mistakes. This information helps refine forecasting models and improve decision-making processes over time.
In summary, forecasting plays a pivotal role in enabling manufacturers to optimize their operations, reduce costs, and adapt to changing market conditions. It allows them to make data-driven decisions, stay competitive, and deliver products efficiently to meet customer demands.
Differentiating Sales Forecasting from Demand Forecasting
Sales forecasting and demand forecasting are related but distinct concepts used in business and supply chain management. They both involve predicting future customer behavior and market trends, but they serve different purposes and focus on different aspects of the business. Let's differentiate them:
- Sales Forecasting: Sales forecasting is the process of estimating the future sales of a specific product or service offered by a company. It involves predicting the quantities or revenues that a company is expected to achieve over a defined period, typically on a short-term basis. The primary purpose of sales forecasting is to assist in planning and managing the company's sales operations, inventory, and production.
Key points about sales forecasting:
- Focus: It concentrates on individual products or services and projects the actual sales a company is likely to achieve.
- Scope: Typically, sales forecasting deals with a shorter time frame, often on a monthly, quarterly, or annual basis.
- Factors: Sales forecasting considers factors such as historical sales data, current sales trends, marketing initiatives, sales team efforts, and the company's competitive position.
- Aim: The main objective is to set achievable sales targets, allocate resources effectively, and optimize inventory levels based on anticipated demand for specific products.
- Demand Forecasting: Demand forecasting, on the other hand, is a broader process that involves predicting the overall demand for a category of products or services in the market. It aims to estimate the total customer demand for a particular product type or industry, considering various market factors, customer preferences, and economic conditions.
Key points about demand forecasting:
- Focus: It looks at the overall demand for a group of products or services, often covering an entire industry or market segment.
- Scope: Demand forecasting typically spans a longer time frame, including long-term projections to help businesses make strategic decisions.
- Factors: Demand forecasting takes into account various external variables, such as economic trends, consumer behavior, population growth, technological advancements, and market competition.
- Aim: The primary goal is to guide businesses in making strategic decisions related to capacity planning, production levels, expansion, and market positioning based on anticipated market demand.
In summary, sales forecasting focuses on predicting the future sales of specific products or services to assist in operational planning and resource allocation. Demand forecasting, on the other hand, deals with estimating the overall market demand for a group of products or services to guide strategic decision-making and long-term planning. Both types of forecasting are crucial for businesses to effectively manage their operations and stay competitive in the market.
Sales Forecasting Techniques for Manufacturers
Sales forecasting is a crucial aspect of the manufacturing industry as it helps manufacturers plan their production, inventory, and resource allocation effectively. There are various techniques that manufacturers can use to forecast sales. Here are some common ones:
- Time Series Analysis: This method involves analyzing historical sales data to identify patterns and trends over time. Time series forecasting techniques, such as moving averages, exponential smoothing, and autoregressive integrated moving averages (ARIMA), can help predict future sales based on past performance.
- Market Research and Surveys: Conducting market research and surveys can provide valuable insights into customer preferences, buying behavior, and market trends. Gathering data from potential customers and industry experts can help manufacturers estimate future sales demand more accurately.
- Customer Segmentation: Manufacturers can group customers based on their characteristics, preferences, and buying behaviors. By analyzing sales patterns within each segment, manufacturers can make more precise forecasts for different customer groups.
- Leading Indicators: Identify leading indicators that have a strong correlation with sales performance. For example, in the manufacturing industry, the number of inquiries or requests for quotations might be a leading indicator. By monitoring these leading indicators, manufacturers can anticipate changes in demand and adjust their forecasts accordingly.
- Seasonality and Trends: Consider the seasonal patterns and long-term trends in the manufacturing industry. Some products might experience higher sales during specific periods, like holidays or seasonal trends, which need to be factored into the sales forecasting process.
- Collaborative Planning, Forecasting, and Replenishment (CPFR): CPFR is a supply chain management approach that involves collaboration between manufacturers and retailers to improve forecasting accuracy. By sharing data and insights, manufacturers can enhance their sales forecasts and align production with actual demand.
- Machine Learning and AI: Advanced techniques like machine learning algorithms and artificial intelligence can be utilized to analyze vast amounts of data and identify patterns that might be difficult to spot using traditional methods. These techniques can improve forecasting accuracy by considering multiple variables and their complex interactions.
- Scenario Analysis: Manufacturers can use scenario analysis to assess different possible situations and their impact on sales. By considering various scenarios, manufacturers can prepare contingency plans and respond proactively to changes in the market.
- Industry and Economic Analysis: Monitoring economic indicators and industry-specific trends can provide valuable context for sales forecasting. For instance, changes in GDP, interest rates, or raw material prices can influence consumer behavior and, consequently, sales.
- Sales Team Input: The sales team's on-the-ground knowledge and experience with customers can provide valuable qualitative insights that complement quantitative forecasting techniques. Regular communication with the sales team can help refine the sales forecast based on their observations and feedback.
It's essential for manufacturers to combine multiple forecasting techniques to increase accuracy and mitigate risks associated with relying solely on one approach. The choice of technique(s) will depend on the nature of the manufacturing business, the available data, and the industry's complexity.
Demand Forecasting Techniques for Manufacturers
Demand forecasting is a crucial process for manufacturers to estimate the future demand for their products accurately. Accurate forecasting enables manufacturers to optimize their production, inventory management, and supply chain operations. Several demand forecasting techniques are commonly used by manufacturers:
- Time Series Analysis: Time series analysis involves studying historical data to identify patterns and trends in demand over time. Techniques like moving averages, exponential smoothing, and autoregressive integrated moving averages (ARIMA) models can be used to make short-term and long-term forecasts based on past demand patterns.
- Regression Analysis: Regression analysis helps manufacturers understand the relationship between demand and various influencing factors, such as price, marketing efforts, seasonality, or economic indicators. By using regression models, manufacturers can predict future demand based on the changes in these variables.
- Machine Learning Algorithms: Machine learning techniques, such as neural networks, decision trees, and random forests, can analyze large and complex datasets to identify patterns and correlations that might be missed by traditional statistical methods. These algorithms can be used to make more accurate demand forecasts.
- Consumer Surveys and Market Research: Conducting consumer surveys and market research helps gather valuable feedback and insights from potential customers, providing qualitative data that can supplement quantitative forecasting techniques.
- Collaborative Forecasting: Collaborative forecasting involves gathering input from various stakeholders, including sales teams, distributors, retailers, and suppliers. Their combined knowledge can lead to a more comprehensive and accurate demand forecast.
- Leading Indicators: Some manufacturers use leading indicators, such as economic indicators, social trends, or competitor activities, to forecast future demand. These indicators can provide early signals of potential changes in demand patterns.
- Seasonality and Trend Analysis: Manufacturers often deal with seasonal demand fluctuations. Analyzing historical data to identify seasonal patterns and trends can help adjust forecasts for future periods accurately.
- New Product Introduction (NPI) Forecasting: When introducing new products, manufacturers can use techniques like analog forecasting (comparing with similar existing products) or conjoint analysis (measuring customer preferences for different product attributes) to estimate demand.
- Simulation and Scenario Analysis: Using simulation techniques and scenario analysis allows manufacturers to model different demand scenarios based on changing market conditions, new product launches, or other variables affecting demand.
- Collaborative Planning, Forecasting, and Replenishment (CPFR): CPFR is a collaborative approach that involves sharing data and insights between manufacturers and their supply chain partners to create a more accurate and synchronized demand forecast.
Manufacturers often employ a combination of these techniques to get a holistic view of demand patterns and make more informed decisions about production, inventory levels, and overall business strategy. The choice of the appropriate technique depends on the specific industry, product characteristics, data availability, and the manufacturer's level of sophistication in forecasting methods.
Data Integration: Bridging the Gap between Sales and Demand Forecasts
Data integration plays a crucial role in bridging the gap between sales and demand forecasts in various industries. It involves combining data from multiple sources and systems to provide a comprehensive and accurate view of an organization's performance, market trends, and customer behavior. By integrating data from sales and demand forecasting processes, businesses can achieve several benefits:
- Accurate Demand Forecasts: Integrating sales data with demand forecasts helps companies gain a better understanding of customer demand patterns. This enables them to adjust their production schedules, inventory levels, and supply chain operations accordingly, reducing the risk of stockouts or overstocking.
- Real-Time Visibility: Data integration facilitates real-time access to sales and demand data, enabling decision-makers to react quickly to changing market conditions and make informed decisions based on up-to-date information.
- Identification of Sales Trends: Integrating sales data with demand forecasts can reveal valuable insights into sales trends and customer preferences. Analyzing this data can help businesses identify best-selling products, customer segments, and regions to optimize sales and marketing strategies.
- Improved Sales Forecast Accuracy: By integrating historical sales data with demand forecasting algorithms, organizations can enhance the accuracy of their predictions. This, in turn, allows them to optimize inventory levels, streamline production, and reduce operational costs.
- Enhanced Collaboration: Data integration promotes better collaboration between sales teams, demand planners, and other stakeholders involved in the forecasting process. Having a unified view of data ensures that everyone is working with the same information, fostering more effective communication and decision-making.
- Reduced Silos: Integrating data from different departments or systems breaks down data silos and fosters a holistic approach to business planning. Sales and demand data can be combined with other relevant data sources, such as marketing, finance, and supply chain, to gain a comprehensive view of the organization's overall performance.
- Demand-Driven Supply Chain: Integrating sales and demand forecast data can lead to a demand-driven supply chain, where inventory levels and production are aligned with actual customer demand. This reduces excess inventory carrying costs and minimizes the risk of stockouts.
- Improved Sales and Marketing Strategies: By understanding demand patterns, businesses can develop more targeted sales and marketing strategies. This includes tailoring promotions, pricing, and advertising efforts to specific customer segments, leading to increased sales and customer satisfaction.
Overall, data integration serves as a foundation for effective sales and demand forecasting, enabling businesses to make data-driven decisions, respond to market changes proactively, and achieve better alignment between supply and demand.
Challenges in Forecasting for Manufacturers:
Forecasting for manufacturers can be a complex and challenging task due to various factors that can influence demand and production. Some of the key challenges in forecasting for manufacturers include:
Demand Variability: Manufacturers often face fluctuating demand patterns, influenced by changing customer preferences, market trends, seasonal variations, and economic conditions. Predicting these fluctuations accurately can be difficult, leading to overstocking or stockouts, both of which can have significant cost implications.
Supply Chain Complexity: Manufacturers typically operate within intricate supply chains involving multiple suppliers, vendors, and logistics partners. The uncertainty in the availability and reliability of these components can make forecasting challenging.
Lead Times and Production Constraints: The time it takes to procure raw materials, produce goods, and deliver them to customers can significantly impact forecasting accuracy. Long lead times, production bottlenecks, and capacity constraints can make it difficult to respond quickly to changes in demand.
New Product Introductions: Forecasting for newly launched products is particularly challenging since historical data is limited or non-existent. Estimating the demand for new products requires market research, customer insights, and a thorough understanding of the product's potential.
Data Quality and Accessibility: Reliable and up-to-date data is crucial for accurate forecasting. Manufacturers may encounter difficulties in obtaining relevant data, especially when dealing with external factors like changes in regulations or economic conditions.
Seasonality and Trends: Seasonal demand patterns and evolving trends add complexity to forecasting models. Incorporating these patterns and trends into the forecasting process requires sophisticated analytics.
Forecasting Horizon: The period for which forecasts are needed can vary significantly depending on the industry. Short-term forecasting might focus on daily or weekly sales, while long-term forecasting could involve planning for years ahead.
Globalization and Geopolitical Factors: Manufacturers operating on a global scale must consider geopolitical uncertainties, trade policies, and currency fluctuations, which can impact demand, costs, and profitability.
Demand Forecasting for Spare Parts and After-Sales Services: Forecasting for spare parts and after-sales services can be particularly challenging due to irregular demand patterns and the need to maintain a balance between inventory costs and customer service.
Integration of Advanced Technologies: Implementing and integrating advanced technologies like artificial intelligence, machine learning, and big data analytics for forecasting requires expertise, resources, and cultural adaptation.
To address these challenges, manufacturers often leverage a combination of historical data analysis, collaborative planning with partners in the supply chain, real-time data monitoring, and advanced forecasting models. Additionally, maintaining flexibility and agility in production and inventory management can help manufacturers adapt to changing conditions and improve forecast accuracy.
The Role of Technology in Forecasting:
The role of technology in forecasting is critical, as it has revolutionized the way we collect, analyze, and interpret data to predict future events, trends, and outcomes. Technological advancements have significantly enhanced forecasting capabilities across various fields, including weather forecasting, financial forecasting, sales forecasting, supply chain forecasting, and more. Here are some key ways technology influences forecasting:
Data collection and storage: Technology allows for the efficient and extensive collection of data from various sources, including sensors, satellites, social media, and online platforms. These vast amounts of data are stored and processed in sophisticated databases, enabling analysts to access historical information and real-time data for more accurate and up-to-date forecasts.
Data analysis and modeling: Advanced data analysis tools, machine learning algorithms, and artificial intelligence (AI) techniques have transformed the way we analyze data for forecasting purposes. These technologies can detect patterns, identify correlations, and make predictions based on historical data, allowing for more precise forecasting models.
Computational power: The increasing computational power of computers has accelerated the speed at which complex forecasting models can be processed. It enables the handling of large datasets and intricate mathematical algorithms, leading to more sophisticated and accurate predictions.
Real-time forecasting: Technology allows for real-time data collection and processing, which is crucial for forecasting in rapidly changing environments. Real-time forecasts are particularly vital in industries like finance, logistics, and emergency response.
Predictive analytics: Predictive analytics tools use historical data and statistical algorithms to anticipate future trends and events. These technologies can identify potential risks, opportunities, and anomalies, aiding decision-makers in making informed choices.
Visualization and communication: Advanced data visualization tools facilitate the presentation of complex forecast results in a visually appealing and understandable manner. This enhances communication and enables stakeholders to grasp the implications of forecasts easily.
Internet of Things (IoT): IoT devices and sensors provide continuous data streams from various sources, such as weather conditions, traffic patterns, and consumer behavior. Integrating this data into forecasting models enhances accuracy and enables real-time adjustments.
Cloud computing: Cloud-based solutions offer scalable storage and computing resources, enabling organizations to handle massive datasets and run complex forecasting models without investing in expensive hardware infrastructure.
Mobile applications: Mobile technology enables access to forecasting information on the go. Users can receive real-time updates, alerts, and insights, empowering them to make informed decisions anytime and anywhere.
Scenario analysis: Technology allows forecasters to simulate different scenarios based on various inputs and assumptions. This helps decision-makers understand the potential outcomes of different strategies, enhancing preparedness for various situations.
In summary, technology plays a vital role in forecasting by improving data collection, analysis, and modeling, providing real-time insights, enhancing communication, and enabling more accurate predictions. As technology continues to evolve, forecasting capabilities will likely become even more advanced and impactful across numerous industries and domains.
Collaborative Forecasting: Aligning Sales and Operations Teams
Benefits of Accurate Forecasting in Manufacturing
Collaborative forecasting is a business strategy that aims to align the sales and operations teams within an organization. The goal is to create a more efficient and effective supply chain management process by integrating sales forecasts with operational plans. By doing so, companies can better meet customer demand, optimize inventory levels, and improve overall business performance.
Here are some key elements of collaborative forecasting and how it can benefit an organization:
- Information Sharing: One of the critical aspects of collaborative forecasting is ensuring that both the sales and operations teams share relevant information. The sales team provides insights into customer demand, market trends, and upcoming promotions or marketing activities. The operations team, on the other hand, shares information about production capabilities, inventory levels, and lead times. This exchange of data allows both teams to make more informed decisions.
- Shared Objectives: Collaborative forecasting encourages the sales and operations teams to work together towards a common goal. Instead of operating in silos, the teams align their objectives, focusing on achieving targets that benefit the entire organization, rather than optimizing their individual department's performance.
- Regular Collaboration Meetings: Holding regular meetings between sales and operations teams is crucial for collaborative forecasting success. During these meetings, teams can review and analyze the forecast accuracy, discuss any changes in the market or production capacity, and adjust plans accordingly.
- Accurate Demand Forecasting: By incorporating insights from the sales team into the forecasting process, the organization can create a more accurate demand forecast. This ensures that the right amount of products or services is produced or stocked, reducing inventory costs and avoiding stockouts.
- Improved Customer Service: When sales and operations are aligned, the organization can respond more effectively to changes in customer demand. Meeting customer expectations and ensuring timely deliveries enhance customer satisfaction and loyalty.
- Efficient Resource Allocation: Collaborative forecasting helps in optimizing resource allocation. For example, the operations team can adjust production schedules based on the sales forecast, preventing overproduction or underutilization of resources.
- Risk Mitigation: By having a more comprehensive understanding of market trends and sales projections, companies can identify potential risks and challenges in advance. This allows them to develop contingency plans to mitigate disruptions to the supply chain.
- Enhanced Agility: In dynamic markets, collaboration between sales and operations teams fosters a culture of adaptability. The ability to respond quickly to changing circumstances becomes a competitive advantage.
Overall, collaborative forecasting is a powerful tool that facilitates communication, cooperation, and coordination between sales and operations teams. By working together, organizations can achieve better forecasting accuracy, optimize their supply chain, and drive improved business performance.
It's important to note that successful implementation of collaborative forecasting requires commitment from top management, proper training, and the use of appropriate technology and analytics tools to process and analyze data effectively.
Real-Life Case Studies: Success Stories in Manufacturing Forecasting
As of my last knowledge update in September 2021, there were several real-life case studies showcasing success stories in manufacturing forecasting. While I don't have access to the most recent data, I can share some historical examples of successful manufacturing forecasting implementations. Keep in mind that newer case studies might have emerged since then. Here are a few notable ones:
- Ford Motor Company: In the early 2000s, Ford implemented a sophisticated forecasting system that helped them predict demand for various car models accurately. The system used historical sales data, market trends, and economic indicators to optimize production schedules and inventory management. As a result, Ford reduced production costs, minimized stockouts, and improved customer satisfaction.
- Cisco: In the mid-2000s, Cisco implemented an advanced demand forecasting system to manage its complex supply chain efficiently. By leveraging real-time data and analytics, they could anticipate demand fluctuations, optimize inventory levels, and ensure the timely delivery of products to customers. Cisco's forecasting success led to significant cost savings and improved operational efficiency.
- Procter & Gamble: P&G, a consumer goods giant, invested in predictive analytics and machine learning technologies for forecasting demand across its extensive product portfolio. By combining historical sales data, market trends, and external factors like weather patterns, P&G improved its inventory management, reduced stockouts, and enhanced production planning.
- Amazon: The e-commerce giant is known for its effective use of data analytics and machine learning to forecast demand accurately. Their algorithms analyze vast amounts of customer data, purchasing behavior, and other variables to anticipate demand and optimize their inventory management. This approach has played a vital role in Amazon's ability to deliver products quickly and maintain high customer satisfaction levels.
- Siemens: Siemens, a global industrial conglomerate, implemented advanced forecasting techniques to optimize its manufacturing processes. By using predictive analytics, they were able to anticipate equipment failures and maintenance needs, leading to reduced downtime and increased efficiency in their production facilities.
- Honeywell: Honeywell, a multinational conglomerate, implemented an AI-driven demand forecasting system that considered variables like economic conditions, customer behavior, and historical sales data. The system helped Honeywell optimize production planning and inventory management, leading to cost savings and improved customer service.
Please note that each of these success stories involved a combination of technologies, data analysis, and skilled personnel to achieve the desired results. As technology continues to evolve, more recent case studies may showcase even more sophisticated and successful approaches to manufacturing forecasting.
The Future of Forecasting in Manufacturing:
As of my last update in September 2021, I can provide insights into the potential future of forecasting in manufacturing. Keep in mind that the field of manufacturing and technology evolves rapidly, so these predictions might have evolved since then. Here are some potential trends and advancements in manufacturing forecasting:
- Artificial Intelligence and Machine Learning Integration: AI and machine learning algorithms will play an increasingly significant role in manufacturing forecasting. These technologies can analyze vast amounts of historical data, identify patterns, and make predictions with higher accuracy and speed than traditional methods. Manufacturers will rely on AI-powered tools to optimize production schedules, anticipate demand fluctuations, and manage inventory more efficiently.
- Predictive Maintenance: As manufacturing processes become more digitized and interconnected through the Industrial Internet of Things (IIoT), predictive maintenance will gain prominence. Advanced sensors and analytics will continuously monitor equipment health, enabling manufacturers to predict maintenance needs accurately. This approach minimizes downtime, reduces repair costs, and enhances overall equipment efficiency.
- Supply Chain Integration and Transparency: Future forecasting in manufacturing will involve closer collaboration and data-sharing within supply chains. Manufacturers will integrate their forecasting processes with suppliers and customers, leading to more accurate demand forecasting and optimized inventory management. Blockchain technology may also be employed to increase transparency and traceability across the supply chain.
- Real-time Data Analytics: With the growth of IIoT and data connectivity, manufacturers will have access to real-time data from various sources. Advanced analytics platforms will enable them to process this data on the fly and gain immediate insights into production performance, demand shifts, and other critical factors. Real-time analytics will allow for quicker decision-making and more agile responses to market changes.
- Simulation and Digital Twins: Digital twin technology will become more prevalent in manufacturing forecasting. Manufacturers will create virtual replicas (digital twins) of their production processes, allowing them to simulate different scenarios, test new strategies, and optimize operations in a risk-free virtual environment. This approach will help reduce costly errors and improve the efficiency of manufacturing processes.
- Human-Machine Collaboration: As AI and automation continue to advance, human-machine collaboration will become the norm in manufacturing. Forecasting tools will enhance the capabilities of human experts rather than replace them. This symbiotic relationship will lead to better decision-making, improved productivity, and more innovative approaches to problem-solving.
- Eco-friendly and Sustainable Forecasting: Sustainability will play a crucial role in the future of manufacturing. Forecasting methods will consider environmental impacts, resource availability, and circular economy principles. Manufacturers will strive to minimize waste, optimize energy usage, and design products with longer lifespans, all of which will be integrated into their forecasting models.
- Advanced Sensing Technologies: The use of advanced sensing technologies, such as LIDAR, 3D cameras, and robotics, will enable better real-time data collection from manufacturing processes. These technologies will contribute to enhanced forecasting accuracy and improved quality control.
In conclusion, the future of forecasting in manufacturing is likely to be heavily influenced by AI, machine learning, IoT, and real-time data analytics. Collaboration, sustainability, and the integration of cutting-edge technologies will be the key drivers of success for manufacturers in an ever-changing global marketplace. However, to get the most accurate and up-to-date insights on the topic, it's always essential to consult the latest industry reports and experts.
Best Practices for Integrating Sales and Demand Forecasting
Integrating sales and demand forecasting is crucial for any business to effectively plan and optimize its operations. By aligning these two processes, companies can make more accurate predictions, improve inventory management, and enhance overall business performance. Here are some best practices for integrating sales and demand forecasting:
Collaboration between Sales and Forecasting Teams: Foster a close working relationship between the sales and forecasting teams. Encourage regular meetings and open communication to share insights, market trends, and feedback on forecasting accuracy. This collaboration will enable both teams to understand each other's perspectives and make more informed decisions.
Data Sharing and Integration: Ensure seamless data sharing and integration between sales data and demand forecasting systems. This can be achieved by using integrated software solutions, APIs, or data connectors that allow the forecasting team to access real-time sales data easily.
Historical Data Analysis: Analyze historical sales data alongside demand forecasting data. Look for patterns, seasonality, and any other factors that may impact demand. Past sales trends can offer valuable insights for forecasting future demand.
Incorporate External Factors: Consider external factors like economic conditions, industry trends, competitive landscape, and marketing initiatives that can influence sales and demand patterns. Integrate these factors into the forecasting model to make predictions more accurate.
Use Advanced Analytics and AI: Leverage advanced analytics and artificial intelligence (AI) techniques to enhance demand forecasting accuracy. AI algorithms can process vast amounts of data, identify patterns, and make predictions that human analysts may miss.
Real-Time Monitoring and Adjustments: Continuously monitor sales and demand trends in real time. Make adjustments to the forecast as new information becomes available. This agile approach ensures that forecasts stay relevant and accurate in dynamic market conditions.
Promotion and Seasonality Planning: Coordinate sales promotions and seasonality planning with demand forecasting. Aligning promotional activities with anticipated demand can prevent stockouts or overstock situations and improve overall sales performance.
Collaborative Sales Forecasting: Implement a collaborative sales forecasting process that involves input from sales representatives and other stakeholders. They can provide valuable insights and on-the-ground knowledge, leading to more accurate forecasts.
Scenario Planning: Perform scenario planning to evaluate various potential outcomes. This exercise helps businesses prepare for different demand scenarios, such as supply chain disruptions or unexpected market shifts.
Regular Forecast Evaluation: Continuously assess the accuracy of forecasts and compare them to actual sales data. Use this feedback to refine and improve the forecasting model and the integration process over time.
Education and Training: Provide training and education to both sales and forecasting teams on the importance of integration and how it benefits the organization. This will help create a shared sense of responsibility and commitment to achieving accurate forecasts.
Executive Buy-In: Obtain buy-in from top-level management for the integrated sales and demand forecasting strategy. When executives support the initiative, it becomes easier to implement necessary changes and allocate resources effectively.
By following these best practices, businesses can create a more efficient and accurate sales and demand forecasting process, leading to better decision-making, improved inventory management, and increased customer satisfaction.
Mitigating Forecasting Risks: Strategies for Manufacturers
Mitigating forecasting risks is essential for manufacturers to ensure smooth operations, minimize inventory costs, and meet customer demands effectively. Here are some strategies that can help manufacturers address forecasting risks:
- Data-driven Forecasting: Use historical sales data, market trends, and other relevant data sources to create more accurate demand forecasts. Utilize statistical methods, machine learning algorithms, and forecasting software to identify patterns and trends in your sales data. Regularly update and fine-tune your forecasting models to reflect changing market conditions.
- Collaborative Forecasting: Engage with key stakeholders, including sales teams, distributors, and suppliers, to gather insights and inputs for the forecasting process. Collaborative forecasting helps capture a more comprehensive picture of the market and reduces the chances of overlooking critical factors that could impact demand.
- Safety Stock Management: Maintain safety stocks of raw materials or finished goods to act as a buffer against demand variability and supply chain disruptions. Safety stock can help manufacturers continue production and fulfill customer orders even when there are unexpected spikes in demand or delays in supply.
- Diversified Supplier Base: Relying on a single supplier can pose risks, especially if they encounter production issues or disruptions. Diversify your supplier base to spread the risk and have alternative sources to procure raw materials or components.
- Continuous Monitoring and Visibility: Implement a robust supply chain visibility system to monitor demand and supply fluctuations in real time. Early identification of deviations from forecasts allows manufacturers to adjust production schedules, inventory levels, and logistics operations promptly.
- Demand Sensing: Use technologies like IoT sensors, point-of-sale data, and social media monitoring to gain real-time insights into changing customer preferences and demand patterns. Demand sensing can help you adapt your production and inventory strategies more dynamically.
- Scenario Planning: Develop contingency plans and conduct scenario-based forecasting to prepare for different potential outcomes. Consider scenarios like supply chain disruptions, economic downturns, or sudden shifts in customer preferences. Having plans in place for different situations allows manufacturers to respond swiftly and effectively.
- Supplier Risk Assessment: Regularly assess the risks associated with your suppliers, including their financial health, operational capabilities, and geographic locations. Understanding supplier risks enables manufacturers to proactively address potential issues and seek alternative options if necessary.
- Agile Manufacturing: Implement agile manufacturing practices that allow for quick adjustments to production schedules and product mix in response to changing market conditions. This flexibility helps manufacturers adapt to unforeseen changes in demand more effectively.
- Continuous Improvement: Regularly review and analyze the forecasting process's performance and identify areas for improvement. Seek feedback from stakeholders and consider incorporating new technologies and best practices to enhance forecasting accuracy and risk mitigation.
By combining these strategies, manufacturers can significantly reduce forecasting risks and create a more resilient supply chain that can adapt to various market challenges and opportunities.
The Human Factor: Balancing Intuition with Data-Driven Forecasting
In the world of decision-making and forecasting, there has always been a delicate balance between relying on human intuition and data-driven analysis. Both approaches have their strengths and limitations, and finding the right blend of the two can lead to more accurate and insightful predictions. This balance is often referred to as "The Human Factor."
Intuition, sometimes called gut feeling or instinct, is the ability to make decisions based on subconscious knowledge and past experiences without explicit reasoning. It allows individuals to draw on their expertise and tacit knowledge to quickly assess a situation and make judgments.
Intuition can be a valuable asset, especially in rapidly changing and uncertain environments, where data might be limited or incomplete. It can help decision-makers identify patterns and connections that might not be immediately evident from data alone.
On the other hand, data-driven forecasting relies on quantitative analysis, statistical models, and machine learning algorithms to make predictions. This approach involves collecting and analyzing vast amounts of data to uncover trends, correlations, and causal relationships.
Data-driven forecasts are often seen as more objective and less susceptible to bias than human intuition. However, they can be limited by the quality of data available and may not capture the full complexity of certain situations.
The key to effective decision-making and forecasting lies in striking a balance between these two approaches:
Acknowledge the Value of Intuition: Decision-makers should recognize the importance of intuition and the insights it can provide. This is especially true in situations where historical data is scarce or unreliable. Experienced professionals with domain expertise can often "read between the lines" and make informed judgments based on subtle cues.
Complement Intuition with Data: While intuition is valuable, it should not be the sole basis for decision-making. Incorporating data-driven analysis can help validate or challenge intuitive judgments, providing a more comprehensive understanding of the situation. Data can also help decision-makers identify blind spots and potential biases in their intuition.
Data Quality and Interpretation: To make effective use of data-driven forecasting, it is crucial to ensure the quality, relevance, and accuracy of the data being used. Additionally, data should be interpreted in the right context, considering domain-specific knowledge and the limitations of the models used.
Embrace Uncertainty: Both intuition and data-driven approaches have inherent uncertainties. Decision-makers should be willing to accept that no forecast is entirely certain and be prepared to adapt their strategies when new information becomes available.
Continuous Learning and Adaptation: Balancing intuition and data-driven forecasting requires an open mindset and a willingness to learn from successes and failures. Decision-makers should continuously evaluate their approaches and update their methods as new insights emerge.
Collaborative Decision-Making: Encouraging interdisciplinary collaboration can enhance the balance between intuition and data-driven analysis. Bringing together experts from different fields can lead to more holistic and robust decision-making processes.
In conclusion, "The Human Factor" involves harmonizing intuition and data-driven forecasting to make well-informed decisions. Embracing the strengths of both approaches while being aware of their limitations can lead to more accurate, insightful, and adaptable forecasts in an ever-changing world.
How Can Deskera Assist You?
Deskera ERP and MRP system can help you:
- Manage production plans
- Maintain Bill of Materials
- Generate detailed reports
- Create a custom dashboard
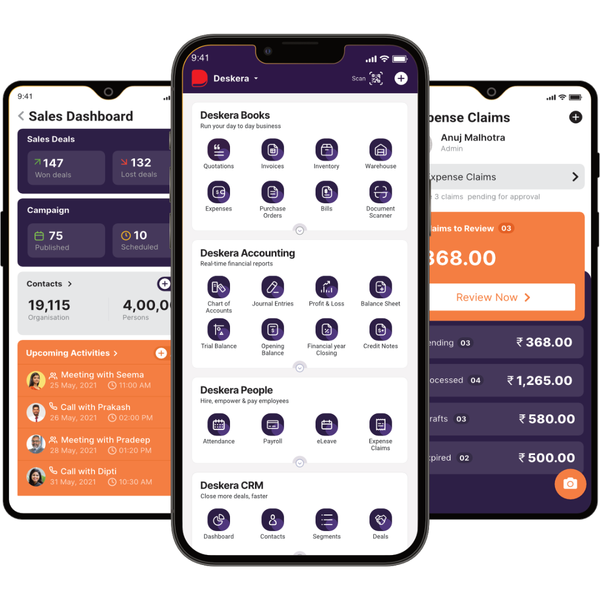
Deskera ERP is a comprehensive system that allows you to maintain inventory, manage suppliers, and track supply chain activity in real-time, as well as streamline a variety of other corporate operations.
Deskera MRP allows you to closely monitor the manufacturing process. From the bill of materials to the production planning features, the solution helps you stay on top of your game and keep your company's competitive edge.
Deskera Books enables you to manage your accounts and finances more effectively. Maintain sound accounting practices by automating accounting operations such as billing, invoicing, and payment processing.
Deskera CRM is a strong solution that manages your sales and assists you in closing agreements quickly. It not only allows you to do critical duties such as lead generation via email, but it also provides you with a comprehensive view of your sales funnel.
Deskera People is a simple tool for taking control of your human resource management functions. The technology not only speeds up payroll processing but also allows you to manage all other activities, such as overtime, benefits, bonuses, training programs, and much more. This is your chance to grow your business, increase earnings, and improve the efficiency of the entire production process.
Related Articles:
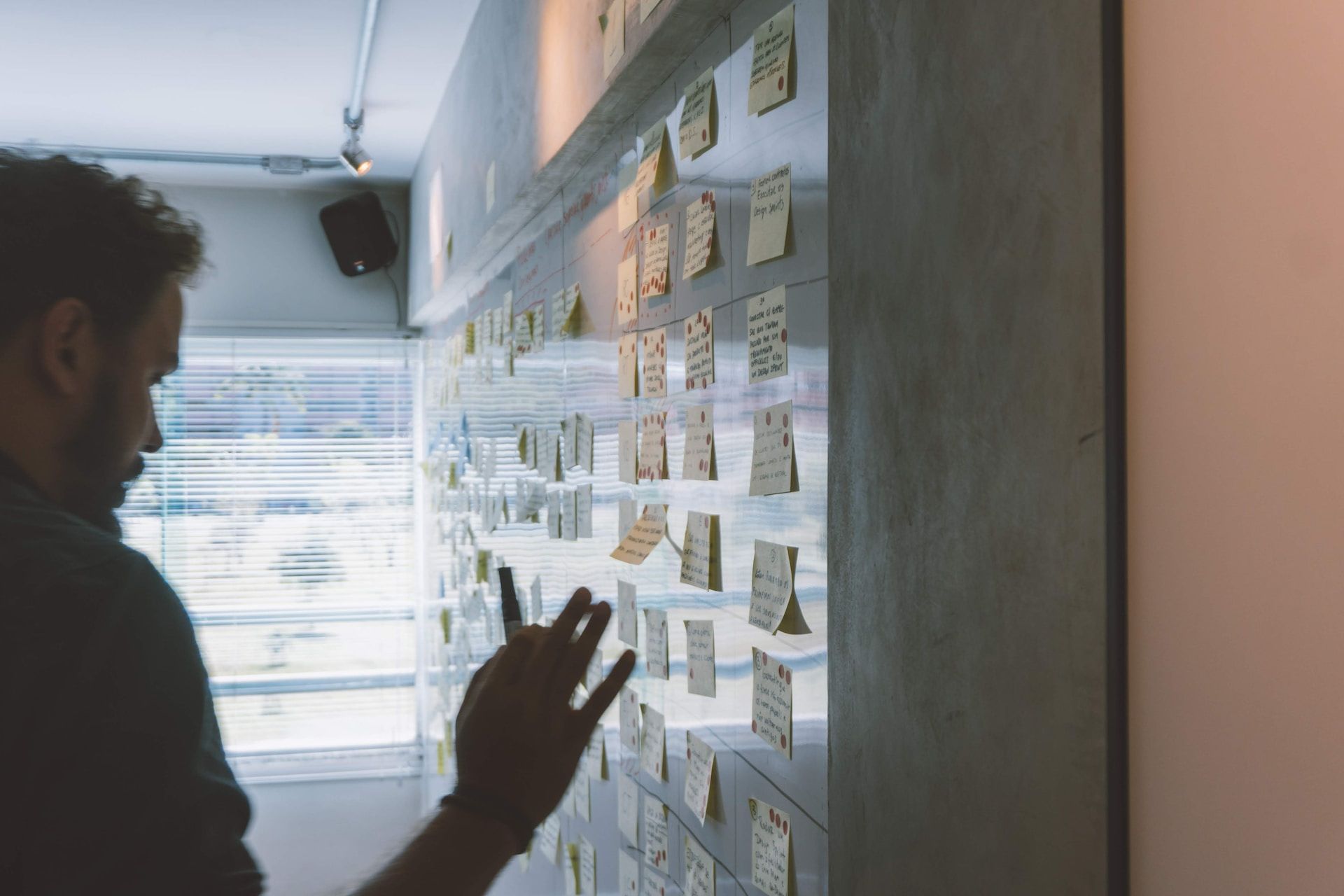
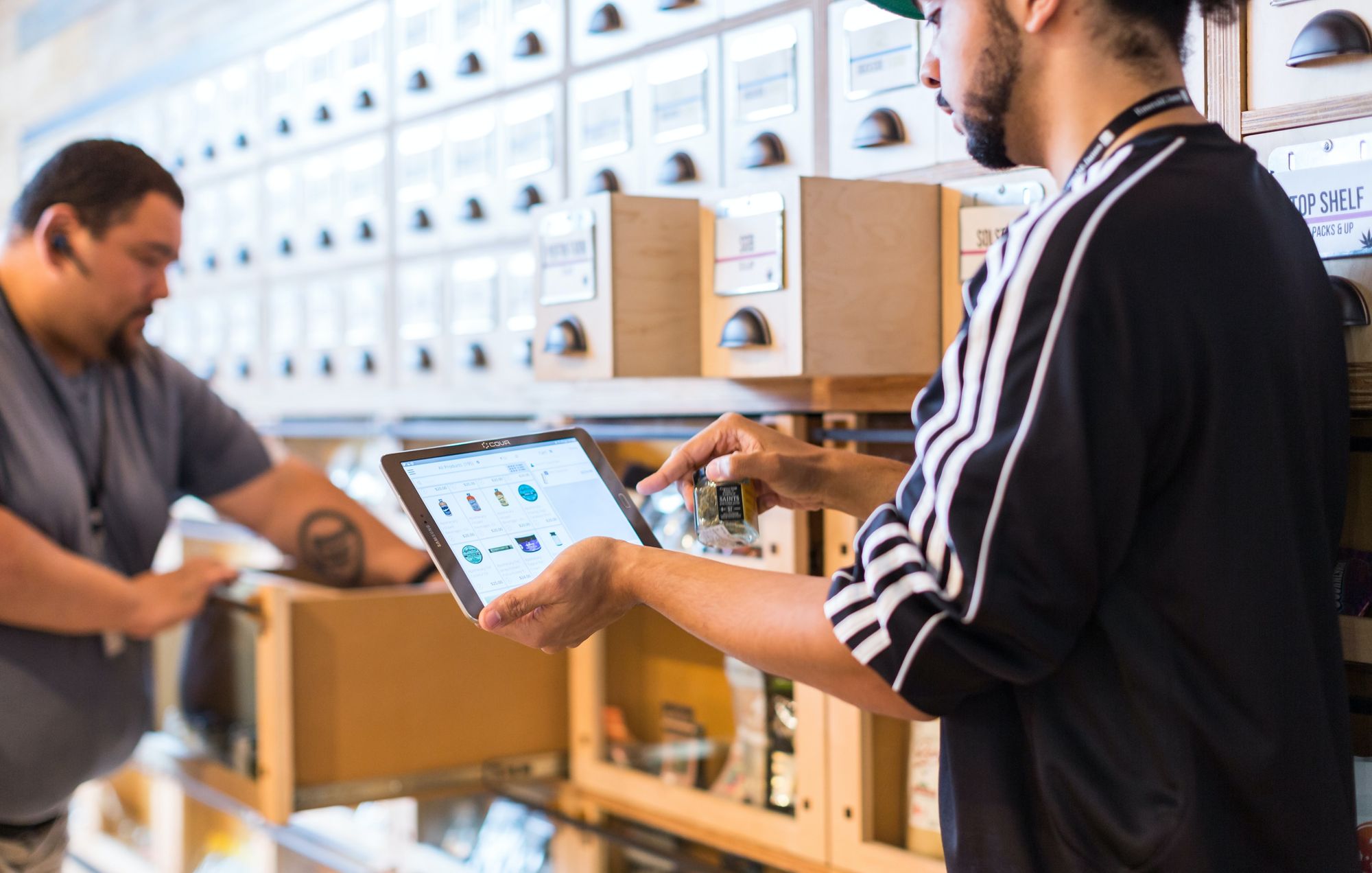
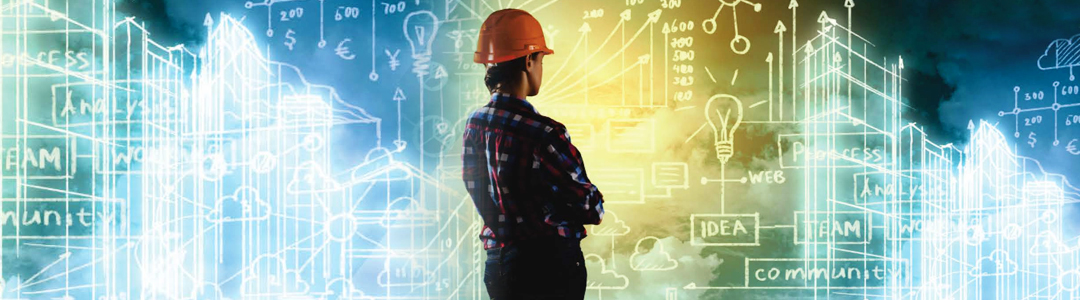