Understanding the significance of demand forecasting is crucial. Manufacturers can optimize their production schedules, align inventory levels with anticipated demand, and allocate resources effectively. This leads to reduced costs, improved customer satisfaction, enhanced decision-making, and ultimately, increased profitability.
However, there are challenges that manufacturers face when it comes to accurately predicting future demand. These challenges highlight the need for robust forecasting methodologies that can handle the dynamic nature of demand patterns.
Historical data plays a pivotal role in overcoming these challenges and improving demand forecasting accuracy. It provides valuable insights into the historical demand patterns, enabling manufacturers to identify trends, seasonality, and other factors that influence demand. By leveraging historical data, manufacturers can develop forecasting models that take into account these factors, leading to more accurate predictions of future demand.
In this article, we will explore the different types of historical data used in demand forecasting. Let’s take a look at the table of content below:
- Historical Data in Accurate Demand Forecasting for Manufacturers
- Importance of Demand Forecasting for Manufacturers
- Demand Forecasting Impact on Business Success
- Challenges Faced by Manufacturers in Accurately Predicting Future Demand
- Role of Historical Data in Improving Demand Forecasting Accuracy
- Factors Influencing Demand Forecasting Accuracy
- Historical Data as a Foundation for Accurate Forecasting
- Leveraging Historical Data for Demand Forecasting
- Challenges and Considerations
- Case Studies
- Frequently Asked Questions (FAQs) Associated with Historical Data in Accurate Demand Forecasting for Manufacturers
- Wrapping Up
- How Deskera Can Assist You?
- Final Takeaways
Let's get started!
Historical Data in Accurate Demand Forecasting for Manufacturers
Historical data refers to past information and records related to demand patterns, sales, customer behavior, market trends, and other relevant factors that impact product demand. In the context of accurate demand forecasting and planning for manufacturers, historical data serves as the foundation for developing forecasting models and making informed predictions about future demand.
Historical data provides valuable insights into past demand patterns, trends, and fluctuations. By analyzing historical data, manufacturers can identify recurring patterns, seasonality, and changes in consumer behavior over time. This understanding helps in developing forecasting models that can capture and predict future demand with a higher degree of accuracy.
The historical data used in demand forecasting can include various types of data, such as sales data, customer data, market data, and external data sources. Sales data provides information on past product demand, including quantities sold, revenue generated, and sales channels.
Furthermore, customer data offers insights into consumer preferences, buying behavior, and demographics. Market data provides information on market trends, competitor actions, and industry dynamics. External data sources can include economic indicators, social media trends, industry reports, and other contextual data that may influence demand.
By leveraging historical data, manufacturers can uncover important insights, such as seasonal variations, product lifecycle patterns, promotional impacts, and the influence of external factors. These insights enable manufacturers to make more accurate forecasts, optimize production schedules, manage inventory levels efficiently, allocate resources effectively, and make informed business decisions.
Overall, historical data serves as a crucial component in accurate demand forecasting for manufacturers. It provides a basis for understanding past demand patterns, identifying trends and fluctuations, and developing forecasting models that can help manufacturers anticipate and meet future demand effectively. By leveraging historical data, manufacturers can enhance their forecasting accuracy, streamline operations, and ultimately achieve better business outcomes.
Importance of Demand Forecasting for Manufacturers
Accurate demand forecasting is a critical aspect of success for manufacturers in today's competitive marketplace. The ability to anticipate and predict customer demand enables manufacturers to optimize their operations, streamline production, minimize inventory costs, and make informed business decisions.
Demand forecasting serves as a compass, guiding manufacturers towards meeting customer expectations and achieving sustainable growth. In this article, we will delve into the role of historical data in improving demand forecasting accuracy and its impact on business success.
Manufacturers operate in dynamic markets influenced by various factors such as evolving consumer preferences, market trends, economic conditions, and competitor actions. Failing to accurately forecast demand can lead to costly consequences, such as overstocking, stockouts, production inefficiencies, and missed sales opportunities.
On the other hand, accurate demand forecasting empowers manufacturers to align their production capacities, optimize inventory levels, ensure timely delivery, and ultimately satisfy customer demand while maximizing profitability.
Demand Forecasting Impact on Business Success
The impact of effective demand forecasting extends beyond operational efficiencies. Manufacturers can strategically plan their resource allocation, procurement, and supply chain activities based on reliable demand forecasts.
This proactive approach helps prevent bottlenecks, reduce lead times, enhance product quality, and strengthen relationships with suppliers and distributors (supplier management).
Furthermore, accurate demand forecasting enables manufacturers to make data-driven decisions regarding pricing, promotions, product development, and market expansion, fostering a competitive edge in the industry.
By leveraging historical data to forecast demand, manufacturers can gain valuable insights into past consumption patterns, seasonal variations, and demand fluctuations.
This historical perspective helps identify trends, understand customer behavior, and detect emerging patterns, providing a solid foundation for future predictions.
Moreover, historical data serves as a compass, guiding manufacturers to navigate through uncertain market conditions, anticipate changes, and adapt their strategies accordingly.
Challenges Faced by Manufacturers in Accurately Predicting Future Demand
Following, we’ve discussed some serious challenges faced by manufacturers in accurately predicting future demand. Let’s learn:
Market Volatility: Manufacturers operate in markets that are often subject to rapid changes and volatility. Market trends, consumer preferences, and economic conditions can shift unexpectedly, making it challenging to accurately forecast future demand. Factors such as new product introductions, changing consumer behavior, and disruptive technologies can significantly impact demand patterns.
Seasonality and Cyclical Trends: Many industries experience seasonal fluctuations in demand, where consumer preferences and purchasing patterns vary throughout the year. Seasonal products or services require accurate forecasting to ensure appropriate inventory levels, production capacity, and resource allocation. Additionally, cyclical trends, influenced by economic cycles and industry-specific factors, can complicate demand forecasting efforts.
New Product Introductions: Launching new products or services poses a unique challenge in demand forecasting. Limited or no historical data is available for new products, making it difficult to predict their market reception and future demand. Manufacturers must rely on market research, consumer insights, and competitive analysis to estimate initial demand and adjust forecasts as real-time data becomes available.
Short Product Lifecycles: Some industries, such as technology or fashion, experience short product lifecycles due to rapid innovation and changing trends. Forecasting demand accurately becomes more challenging in such cases, as manufacturers need to predict demand within a limited timeframe before the product becomes obsolete or loses market appeal.
Supply Chain Complexity: Manufacturers operate within complex supply chains, involving multiple suppliers, distributors, and intermediaries. Demand forecasting becomes more challenging when manufacturers must consider the dynamics of the entire supply chain, including lead times, transportation, inventory levels, and production capacities across various nodes. Collaboration and data sharing with supply chain partners become essential for accurate forecasting.
Limited Historical Data: Historical data is crucial for accurate demand forecasting, but obtaining a sufficient amount of relevant and high-quality data can be challenging. In some cases, manufacturers may lack access to comprehensive historical data due to data unavailability, data gaps, or inadequate data collection and storage practices. Insufficient historical data can lead to less reliable forecasts and increased forecasting errors.
External Factors: Manufacturers must consider external factors that can influence demand, such as economic indicators, government policies, natural disasters, and geopolitical events. These external factors introduce uncertainties and increase the complexity of demand forecasting. Manufacturers need to assess and incorporate these factors into their forecasting models to improve accuracy.
Consumer Behavior and Preferences: Understanding and predicting consumer behavior is crucial for accurate demand forecasting. Consumer preferences, purchasing habits, and brand loyalty can change over time, making it challenging to accurately anticipate future demand. Factors such as shifting demographics, cultural changes, and evolving consumer expectations further complicate the forecasting process.
Limited Forecast Horizon: Manufacturers often face the challenge of forecasting demand over different time horizons, ranging from short-term tactical planning to long-term strategic decision-making. Accuracy requirements vary depending on the time horizon, and balancing short-term operational needs with long-term planning can be demanding.
Addressing these challenges requires manufacturers to adopt robust demand forecasting methodologies, leverage advanced analytics and technology, gather market intelligence, and establish effective collaboration with supply chain partners and customers. By overcoming these challenges, manufacturers can improve their forecasting accuracy and gain a competitive edge in the marketplace.
Role of Historical Data in Improving Demand Forecasting Accuracy
Demand forecasting refers to the process of estimating future customer demand for a product or service. It plays a crucial role in manufacturing operations as it helps manufacturers anticipate the quantity and timing of customer orders, enabling them to efficiently plan and manage their resources. Here's a detailed explanation of the key points in this section:
Demand forecasting involves analyzing historical data, market trends, and other relevant factors to predict future customer demand accurately. In manufacturing operations, demand forecasting serves as a foundation for effective planning, production planning and scheduling, inventory management, and resource allocation. It provides manufacturers with insights into the expected demand for their products, enabling them to align their operations accordingly.
Key objectives of demand forecasting:
Demand forecasting in manufacturing serves several objectives that contribute to operational efficiency and business success. Following, we've discussed these objectives:
Optimizing Production: Accurate demand forecasts help manufacturers determine the right production levels to meet customer demand without excessive overproduction or underproduction. This prevents unnecessary inventory holding costs, reduces production bottlenecks, and improves overall production efficiency.
Inventory Management: By forecasting demand accurately, manufacturers can maintain optimal inventory levels. This ensures sufficient stock to fulfill customer orders promptly while minimizing excess inventory and associated holding costs.
Resource Allocation: Demand forecasting guides manufacturers in allocating their resources effectively, such as labor, raw materials, and production capacities. It enables them to plan their resource utilization based on anticipated demand, leading to efficient resource allocation and cost optimization.
Benefits of accurate demand forecasting:
Accurate demand forecasting offers numerous benefits to manufacturers, positively impacting various aspects of their operations. Some key benefits include:
Improved Customer Satisfaction: Accurate demand forecasting allows manufacturers to meet customer demand promptly, avoiding stockouts and delayed deliveries. This enhances customer satisfaction, builds trust, and strengthens customer relationships.
Reduced Costs: By forecasting demand accurately, manufacturers can avoid overproduction and excess inventory. This helps reduce storage costs, minimize wastage, and optimize resource utilization, leading to cost savings.
Enhanced Decision-Making: Reliable demand forecasts provide manufacturers with valuable insights for making informed business decisions. They can strategize pricing, promotional activities, and product launches based on projected demand, improving the effectiveness of their decision-making process.
Efficient Supply Chain Management: Accurate demand forecasting enables manufacturers to collaborate effectively with suppliers, distributors, and other partners in the supply chain. This facilitates better coordination, streamlined inventory management, and improved overall supply chain performance.
Competitive Advantage: Manufacturers that excel in demand forecasting gain a competitive edge by aligning their operations with market demand. They can respond quickly to changing market conditions, anticipate trends, and seize opportunities, giving them an advantage over competitors.
By focusing on the role of historical data in improving demand forecasting accuracy, manufacturers can harness the power of data-driven insights to optimize their operations, enhance customer satisfaction, and drive business success.
Factors Influencing Demand Forecasting Accuracy
Demand forecasting accuracy is influenced by various factors that manufacturers must consider when predicting future customer demand. These factors introduce uncertainties and challenges, making it crucial to account for their impact on forecasting. Here's a detailed explanation of the key factors and their effects:
Market Trends:
Market trends encompass the shifts and changes in consumer preferences, industry dynamics, and emerging market conditions. These trends can significantly impact demand forecasting accuracy. For example, changing consumer preferences may lead to sudden shifts in demand for specific products or variations in product features. Manufacturers need to closely monitor and analyze market trends to adjust their forecasts accordingly and remain competitive.
Seasonality:
Seasonality refers to the predictable patterns of demand that repeat on a seasonal basis. Many industries experience fluctuations in demand due to factors such as weather, holidays, or cultural events. Accurate forecasting requires recognizing these seasonal patterns and adjusting forecasts accordingly. Failure to account for seasonality can result in either overproduction during peak seasons or insufficient stock during low-demand periods.
Product Lifecycle:
The product lifecycle, consisting of the introduction, growth, maturity, and decline stages, has a significant impact on demand forecasting accuracy. Different stages of the lifecycle present varying levels of demand and require distinct forecasting approaches. Early in the lifecycle, forecasting relies heavily on market research and customer insights, while during the maturity and decline stages, historical data and market trends become more crucial.
Consumer Behavior:
Consumer behavior, including purchasing habits, preferences, and buying patterns, directly affects demand forecasting accuracy. Consumer behavior can be influenced by various factors such as changing demographics, cultural shifts, marketing campaigns, or social trends. Manufacturers need to continually monitor and analyze consumer behavior to understand demand drivers and anticipate changes that could impact future demand.
External Factors:
External factors encompass a range of variables that lie outside the control of manufacturers but can significantly influence demand. Economic conditions, such as inflation, interest rates, or unemployment rates, can impact consumer spending and overall demand for products. Competitor actions, such as pricing strategies or product launches, can also affect demand patterns. Manufacturers need to incorporate these external factors into their forecasting models to account for potential demand fluctuations.
These factors create uncertainties and challenges for manufacturers in demand forecasting:
Increased Complexity: The combination of multiple factors, such as market trends, seasonality, and consumer behavior, adds complexity to demand forecasting models. Manufacturers must analyze and interpret a wide range of data sources to gain a comprehensive understanding of demand drivers.
Data Variability: Factors influencing demand are often dynamic and subject to change. This variability introduces challenges in accurately capturing and incorporating these factors into forecasting models. Manufacturers need to continuously update and adapt their models to reflect changing conditions.
Limited Information: In some cases, manufacturers may have limited information or data availability, particularly for new products or emerging markets. Insufficient data hampers forecasting accuracy and requires reliance on assumptions or market research to estimate demand.
Unforeseen Events: External factors such as natural disasters, political instability, or sudden shifts in consumer sentiment can disrupt demand patterns. These unforeseen events introduce uncertainties and make it challenging to accurately predict future demand.
To address these challenges, manufacturers must employ robust forecasting methodologies, leverage advanced analytics tools, and continuously monitor and update their models based on the changing market dynamics. By accounting for the factors influencing demand forecasting accuracy, manufacturers can improve the reliability of their forecasts and make better-informed decisions.
Historical Data as a Foundation for Accurate Forecasting
Historical data plays a crucial role as a foundation for demand forecasting models. By analyzing past demand patterns and trends, manufacturers can gain valuable insights that serve as a basis for predicting future demand. Here's a detailed explanation of the importance of historical data and the types of data used in demand forecasting:
Importance of Historical Data:
Historical data serves as a reference point for understanding past demand patterns and behavior. It provides manufacturers with a basis for identifying trends, seasonality, and fluctuations in customer demand over time.
By leveraging historical data, manufacturers can make informed decisions about production levels, inventory management, and resource allocation. Historical data allows for a data-driven approach to demand forecasting, enabling manufacturers to improve accuracy and optimize their operations.
Types of Historical Data Used in Demand Forecasting:
Several types of historical data are utilized in demand forecasting. These types include:
Sales Data: Sales data is one of the most fundamental types of historical data used in forecasting. It includes information about the quantities sold, revenue generated, and customer purchasing patterns over a specific period. Sales data provides insights into historical demand levels and helps identify any recurring patterns or seasonality.
Customer Data: Customer data encompasses information about individual customers, such as their purchase history, preferences, demographics, and behavior.
Furthermore, analyzing customer data allows manufacturers to segment their customer base, understand demand variations across different customer segments, and tailor their forecasts accordingly.
Market Data: Market data refers to information about the broader market conditions, industry trends, and competitor performance. It includes data on market size, market share, pricing trends, and market dynamics. Analyzing market data helps manufacturers understand the external factors that influence demand and incorporate them into their forecasting models.
External Data Sources: In addition to internal data sources, manufacturers can leverage external data sources to enhance their forecasting accuracy. This includes data from industry reports, market research studies, government statistics, social media trends, and other relevant external sources. External data provides additional context and insights into market trends, consumer behavior, and emerging patterns.
Insights from Historical Data:
Historical data provides valuable insights into past demand patterns, trends, and fluctuations, which serve as a foundation for forecasting future demand. Here's how historical data contributes to accurate demand forecasting:
Identifying Demand Patterns: Historical data helps manufacturers identify recurring demand patterns, such as seasonality, cyclicality, or trend-based variations. By understanding these patterns, manufacturers can adjust their forecasts to account for expected fluctuations in demand.
Trend Analysis: Historical data allows for the identification and analysis of long-term trends in demand. It helps manufacturers understand shifts in customer preferences, market dynamics, and industry trends. By recognizing these trends, manufacturers can adapt their strategies and anticipate changes in future demand.
Forecast Validation: Historical data can be used to validate the accuracy of demand forecasts. By comparing the forecasted values with the actual historical data, manufacturers can assess the reliability of their forecasting models, identify areas of improvement, and refine their future forecasts.
Demand Fluctuation Analysis: Historical data enables manufacturers to analyze past demand fluctuations and understand their causes. By identifying factors that lead to demand volatility, manufacturers can adjust their forecasting models and develop contingency plans to mitigate the impact of future fluctuations.
Incorporating historical data into demand forecasting models allows manufacturers to make data-driven predictions and improve the accuracy of their forecasts. It provides a solid foundation for understanding past demand patterns, identifying trends, and making informed decisions to optimize operations and resource allocation.
Leveraging Historical Data for Demand Forecasting
The process of leveraging historical data for demand forecasting involves several key steps to ensure accurate and reliable forecasts. Here's a detailed explanation of the process, including data cleansing and preprocessing techniques, statistical and machine learning techniques, and the role of advanced analytics and artificial intelligence:
Data Cleansing and Preprocessing:
Data cleansing and preprocessing are essential steps to ensure the quality and consistency of historical data. This involves:
Data Cleaning: Removing any outliers, missing values, or inconsistent data points that can skew the analysis and forecasting results. Techniques such as imputation or interpolation can be used to handle missing data.
Data Transformation: Transforming data into a suitable format for analysis. This may involve aggregating data into appropriate time intervals (e.g., daily, weekly, monthly) or converting data into a standardized scale.
Handling Seasonality and Trends: Identifying and removing seasonality or trend components from the historical data to isolate the underlying patterns. This can involve techniques such as seasonal decomposition or differencing.
Statistical and Machine Learning Techniques:
Various statistical and machine learning techniques can be employed to analyze historical data and generate accurate demand forecasts. Some commonly used techniques include:
Time Series Analysis: Time series analysis involves analyzing historical data to identify patterns, trends, and seasonality. Techniques such as moving averages, exponential smoothing, and autoregressive integrated moving average (ARIMA) models are commonly used in time series analysis for demand forecasting.
Regression Analysis: Regression analysis is used to model the relationship between demand and other variables that influence it, such as price, promotions, or marketing campaigns. Multiple linear regression or nonlinear regression models can be employed to capture these relationships and forecast demand.
Predictive Modeling: Predictive modeling techniques, including machine learning algorithms, can be used to analyze historical data and identify complex patterns and relationships. Algorithms such as random forest, gradient boosting, or neural networks can be trained on historical data to forecast future demand accurately.
Advanced Analytics and Artificial Intelligence:
Advanced analytics techniques, combined with artificial intelligence (AI), offer powerful tools for extracting valuable insights from historical data. These techniques include:
Predictive Analytics: By leveraging AI algorithms and advanced analytics techniques, predictive analytics can uncover hidden patterns and relationships within historical data to generate accurate demand forecasts. Additionally, these methods can handle large and complex datasets, identify nonlinear relationships, and adapt to changing market conditions.
Forecasting Optimization: Advanced analytics can be used to optimize demand forecasting by incorporating various factors such as pricing, promotions, capacity constraints, and supply chain considerations. Optimization models can find the optimal balance between demand and available resources to improve forecasting accuracy.
AI-based Forecasting: AI techniques such as deep learning, recurrent neural networks (RNNs), or long short-term memory (LSTM) networks can be employed to capture complex temporal dependencies and nonlinear relationships in demand data. These algorithms can learn from historical patterns and make accurate forecasts even in dynamic and uncertain environments.
By leveraging advanced analytics and AI techniques, manufacturers can gain deeper insights from historical data, uncover hidden patterns, and generate more accurate demand forecasts. These methods enable manufacturers to make data-driven decisions, optimize their operations, and respond effectively to changing market conditions.
Challenges and Considerations
Using historical data for demand forecasting comes with several challenges that manufacturers must address to ensure accurate and reliable forecasts. Additionally, selecting appropriate forecasting models requires careful consideration of various factors. Let's explore the common challenges and considerations:
Challenges with Historical Data:
Following, we've discussed these challenges thoroughly. Let's learn:
Data Availability: Historical data may not always be readily available, especially for new products or emerging markets. Limited data availability can hinder accurate forecasting, requiring manufacturers to rely on alternative data sources or employ techniques such as market research or expert opinions.
Data Quality Issues: Historical data may contain outliers, missing values, or inconsistent entries that can impact forecasting accuracy. Data cleansing and preprocessing techniques, as discussed earlier, help address these issues by removing or imputing problematic data points.
Incorporating Dynamic Factors: Historical data alone may not capture the impact of dynamic factors such as evolving customer preferences, technological advancements, or regulatory changes. Manufacturers need to consider how to incorporate these dynamic factors into their forecasting models, such as by leveraging external data sources, expert opinions, or qualitative insights.
Considerations for Selecting Forecasting Models:
Following, we've discussed important considerations for selecting forecasting methods. Let's learn:
Nature of the Product: The characteristics of the product, such as perishability, seasonality, or product lifecycle stage, influence the choice of forecasting models. For example, time series analysis may be suitable for products with strong seasonality, while predictive modeling techniques might be more appropriate for products with complex demand patterns.
Industry Dynamics: Different industries have unique characteristics that impact demand forecasting. Considerations such as product substitutability, competitive landscape, or industry-specific trends need to be accounted for when selecting forecasting models. For instance, demand for fashion apparel may require more advanced modeling techniques due to rapidly changing trends.
Market Dynamics: The nature of the market, including market size, market share, competitive intensity, or market maturity, can influence the choice of forecasting models. Market-driven factors such as pricing strategies, promotional activities, or supply chain dynamics need to be integrated into the forecasting models to capture their impact on demand.
Data Availability and Complexity: The availability and complexity of historical data impact the choice of forecasting models. Some models, such as regression analysis, require a sufficient amount of historical data with relevant variables, while others, like machine learning algorithms, can handle large and complex datasets but may require more computational resources.
Forecasting Horizon: The forecasting horizon, i.e., the time frame for which forecasts are needed, is another consideration. Short-term forecasts may rely more on recent historical data and immediate market trends, while long-term forecasts may require a combination of historical patterns and macroeconomic indicators.
It's important for manufacturers to carefully evaluate these considerations and select forecasting models that best suit their specific needs and circumstances. It may also be beneficial to employ a combination of models or utilize advanced analytics techniques to leverage the strengths of different approaches.
By acknowledging the challenges associated with historical data and considering the appropriate forecasting models, manufacturers can enhance the accuracy of their demand forecasts and make more informed decisions regarding production, inventory, and resource allocation.
Enhancing Demand Forecasting Accuracy
To enhance demand forecasting accuracy using historical data, manufacturers can employ various strategies and techniques. Here's a detailed explanation of each strategy:
Incorporating External Data Sources and Market Intelligence:
Augmenting historical data with external data sources and market intelligence can provide additional insights and improve forecasting accuracy. Manufacturers can gather data from industry reports, market research studies, social media trends, or economic indicators. By incorporating these external factors into their forecasting models, manufacturers can capture market dynamics, competitor actions, and emerging trends that influence demand.
Applying Advanced Forecasting Techniques and Algorithms:
Utilizing advanced forecasting techniques and algorithms can improve the accuracy of demand forecasts. Some techniques include:
Machine Learning: Employing machine learning algorithms, such as random forests, support vector machines, or neural networks, enables the identification of complex patterns and relationships in historical data. These algorithms can adapt to changing demand patterns and incorporate a wide range of variables, leading to more accurate forecasts.
Time Series Analysis: Leveraging advanced time series analysis techniques, such as exponential smoothing, ARIMA models, or state space models, allows manufacturers to capture seasonality, trends, and other temporal dependencies present in historical data. These techniques are particularly effective for demand forecasting with strong time-based patterns.
Ensemble Forecasting: Utilizing ensemble forecasting techniques combines the predictions of multiple forecasting models to improve accuracy. By aggregating the forecasts from various models, manufacturers can reduce biases, increase robustness, and capture a wider range of demand scenarios.
Implementing Real-Time Data Analytics and Monitoring:
Real-time data analytics and monitoring enable manufacturers to capture up-to-date information and adjust forecasts accordingly. By integrating real-time data streams, such as point-of-sale data, customer feedback, or online sales data, manufacturers can gain insights into demand fluctuations and make timely adjustments to their forecasts.
Utilizing Predictive Analytics Tools and Software:
Leveraging predictive analytics tools and software can enhance demand forecasting accuracy. These tools enable manufacturers to automate data processing, apply advanced algorithms, and generate forecasts efficiently. They often include features such as data visualization, statistical modeling, and scenario analysis, allowing manufacturers to explore different forecasting scenarios and assess their impact.
Collaborating with Suppliers, Distributors, and Customers:
Collaborating with supply chain partners, including suppliers, distributors, and customers, can provide valuable insights for demand forecasting. By sharing data, market intelligence, and customer feedback, manufacturers can gain a holistic view of the demand ecosystem.
Furthermore, collaborative forecasting, demand sensing, and demand-driven supply chain approaches can improve accuracy by incorporating diverse perspectives and reducing information gaps.
These strategies, when combined, can significantly enhance demand forecasting accuracy by leveraging historical data effectively. By incorporating external data sources, applying advanced techniques, implementing real-time analytics, utilizing predictive analytics tools, and fostering collaboration, manufacturers can make more informed decisions, optimize their operations, and improve customer satisfaction.
Case Studies
Following, we’ve discussed some important case studies. Let’s learn:
Case Study 1: Walmart
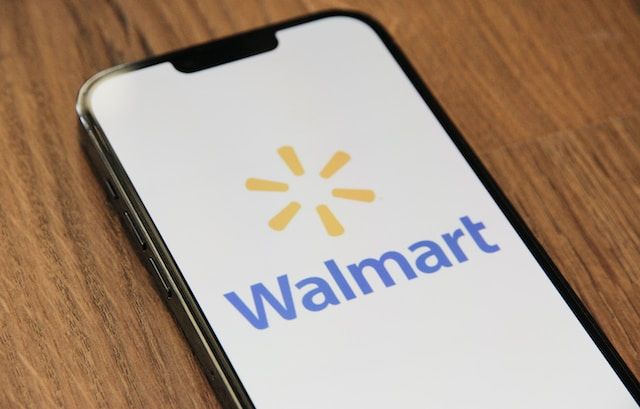
Walmart, one of the world's largest retailers, has implemented historical data-driven demand forecasting to optimize its operations and improve customer satisfaction. By analyzing historical sales data, customer data, and market trends, Walmart has been able to achieve the following benefits:
Efficient Inventory Management: Walmart uses historical data to accurately forecast demand for its extensive product range. By aligning inventory levels with anticipated demand, they reduce stockouts, minimize excess inventory, and improve overall inventory turnover. This optimization leads to improved efficiency, cost savings, and better product availability for customers.
Demand-Sensitive Replenishment: Leveraging historical data, Walmart has developed demand-sensitive replenishment strategies. By analyzing past demand patterns, they adjust replenishment schedules and quantities to match customer demand. This enables them to maintain optimal stock levels, reduce lead times, and improve product availability, resulting in higher customer satisfaction.
Enhanced Supply Chain Collaboration: Walmart collaborates with its suppliers and utilizes historical data to improve forecasting accuracy across the entire supply chain. By sharing historical sales data with suppliers, they facilitate joint forecasting and align production and inventory levels more effectively. This collaborative approach minimizes supply chain disruptions, reduces stockouts, and enhances overall operational efficiency.
Check out the inventory management software for your business to optimize inventory and reduce costs.
Case Study 2: Amazon
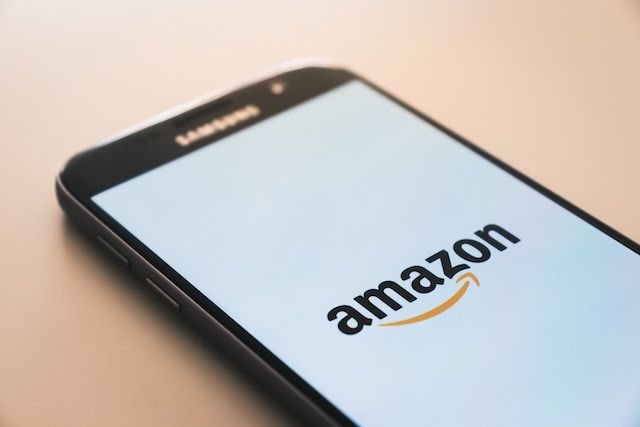
Amazon, the leading global e-commerce company, extensively utilizes historical data-driven demand forecasting to drive its operations and customer experience. By harnessing historical data, Amazon has achieved the following benefits:
Personalized Recommendations: Amazon uses historical customer data, including purchase history, browsing behavior, and reviews, to provide personalized product recommendations. By analyzing historical data patterns, they can suggest relevant products to customers, leading to increased sales and customer satisfaction.
Dynamic Pricing: Historical data analysis allows Amazon to dynamically adjust prices based on demand patterns, competitor actions, and market trends. By continuously monitoring historical data and market conditions, Amazon can optimize pricing strategies to maximize revenue and stay competitive.
Demand Planning and Fulfillment Optimization: Amazon leverages historical data to optimize its demand planning and fulfillment operations. By analyzing historical sales patterns, seasonal trends, and customer behavior, they can accurately forecast demand and adjust inventory levels and distribution networks accordingly. This leads to improved order fulfillment, reduced delivery times, and enhanced customer satisfaction.
Benefits of Accurate Demand Forecasting:
Accurate demand forecasting based on historical data can have significant benefits for manufacturers:
Improved Customer Satisfaction: Accurate demand forecasting ensures product availability, reduces stockouts, and minimizes order delays. This enhances customer satisfaction by meeting customer demands promptly and delivering a positive shopping experience.
Optimal Inventory Management: Historical data-driven demand forecasting enables manufacturers to optimize inventory levels, reducing carrying costs while ensuring sufficient stock availability. This results in efficient inventory management, improved cash flow, and reduced wastage or obsolescence.
Cost Reduction: Accurate demand forecasting helps manufacturers streamline their production and procurement processes. By aligning production schedules and purchase orders with anticipated demand, they can reduce overproduction, minimize excess inventory, and lower costs associated with storage and obsolescence.
Enhanced Decision-Making: Historical data-driven demand forecasting provides manufacturers with valuable insights for making informed decisions. By analyzing historical patterns and trends, manufacturers can make strategic choices regarding pricing, promotions, product launches, capacity planning, and resource allocation.
Increased Profitability: Accurate demand forecasting directly impacts profitability by optimizing production, minimizing costs, and maximizing revenue. Manufacturers can allocate resources efficiently, reduce waste, and capture market opportunities, leading to improved profitability and financial performance.
These case studies and benefits illustrate the importance of historical data-driven demand forecasting for manufacturers. By leveraging historical data effectively, manufacturers can optimize their operations, improve customer satisfaction, and drive business success.
Frequently Asked Questions (FAQs) Associated with Historical Data in Accurate Demand Forecasting for Manufacturers
Following, we’ve discussed some important frequently asked questions (faqs) associated with historical data in accurate demand forecasting for manufacturers. Let’s learn:
Q1: What is demand forecasting?
A1: Demand forecasting is the process of estimating the future demand for a product or service. It involves analyzing historical data, market trends, and other relevant factors to predict the quantity of goods or services that customers will demand over a specific time period.
Q2: Why is demand forecasting significant in manufacturing operations?
A2: Demand forecasting is crucial in manufacturing operations for several reasons:
Production Optimization: Accurate forecasting helps manufacturers optimize their production processes, ensuring that the right quantity of products is manufactured to meet customer demand.
Inventory Management: Demand forecasting enables manufacturers to maintain optimal inventory levels, reducing the risk of stockouts or excess inventory.
Resource Allocation: By forecasting demand, manufacturers can allocate resources efficiently, such as labor, raw materials, and equipment, to meet projected demand and avoid bottlenecks or underutilization.
Q3: How can manufacturers incorporate external data sources and market intelligence in demand forecasting?
A 3: Incorporating external data sources and market intelligence involves gathering and analyzing data from external sources such as industry reports, economic indicators, social media trends, and customer feedback. This additional information enhances demand forecasting accuracy by providing insights into market dynamics, customer preferences, and external factors that influence demand.
Q4: What factors can affect demand forecasting accuracy?
A4: Several factors can influence demand forecasting accuracy, including:
Market Trends: Changes in market trends, consumer preferences, and competitive dynamics can impact demand patterns and make accurate forecasting challenging.
Seasonality: Products that exhibit seasonal demand variations require specific forecasting techniques to account for these patterns.
Product Lifecycle: Different stages of a product's lifecycle may have varying demand patterns, and accurate forecasting requires understanding the product's lifecycle stage.
Consumer Behavior: Changes in consumer behavior, purchasing habits, or preferences can affect demand patterns and forecasting accuracy.
External Factors: Economic conditions, competitor actions, government policies, or other external factors can introduce uncertainties and influence demand patterns.
Q5: How can historical data improve demand forecasting accuracy?
A5: Historical data provides a foundation for demand forecasting by offering insights into past demand patterns, trends, and fluctuations. It helps identify seasonal variations, understands the impact of marketing activities, and capture the effects of external factors on demand. By analyzing historical data, manufacturers can identify patterns, establish forecasting models, and make more accurate predictions about future demand.
Q6: What are some techniques used for demand forecasting?
A6: Various techniques can be used for demand forecasting, including:
Time Series Analysis: This technique analyzes historical data to identify patterns, seasonality, and trends and applies forecasting models like exponential smoothing or ARIMA.
Regression Analysis: Regression models use historical data and related variables (such as price, promotions, or economic indicators) to estimate the relationship between demand and these factors.
Machine Learning: Machine learning algorithms, such as random forests, support vector machines, or neural networks, can identify complex patterns in historical data and make accurate predictions.
Predictive Modeling: Predictive models utilize statistical or machine learning techniques to forecast demand based on historical data and other relevant factors.
Q7: How can manufacturers ensure data quality when using historical data for forecasting?
A7: To ensure data quality, manufacturers should employ data cleansing and preprocessing techniques. This involves removing outliers, addressing missing values, and correcting inconsistencies in the historical data.
Furthermore, data validation methods, such as cross-checking with other sources or manual review, can also help verify the accuracy and reliability of the data used for forecasting.
Q8: What are some challenges in using historical data for demand forecasting?
A8: Challenges in using historical data for demand forecasting include:
Data Availability: Historical data may not be readily available or may have gaps, especially for new products or emerging markets.
Data Quality Issues: Historical data can contain errors, inconsistencies, or missing values, which can impact the accuracy of forecasting models.
Incorporating Dynamic Factors: Historical data may not capture recent market changes, shifts in consumer behavior, or other dynamic factors, requiring additional adjustments or the inclusion of real-time data sources.
Q9: How can manufacturers select appropriate forecasting models for their products and industry?
A9: Selecting the right forecasting model involves considering the nature of the product, industry dynamics, and the available historical data. Manufacturers should assess whether the demand patterns exhibit seasonality, trends, or other specific characteristics.
Additionally, understanding the industry's competitive landscape, market dynamics, and product lifecycle stages can help determine the appropriate forecasting techniques or algorithms to apply.
Q10: How can collaboration with suppliers, distributors, and customers improve demand forecasting accuracy?
A10: Collaboration with supply chain partners allows manufacturers to gather additional insights and improve forecasting accuracy.
By sharing data, market intelligence, and customer feedback, manufacturers can better understand demand patterns, anticipate changes, and align their forecasting models with the collective knowledge of the supply chain.
Furthermore, collaborative forecasting and demand sensing approaches enable manufacturers to incorporate diverse perspectives, reduce information gaps, and achieve more accurate forecasts.
Wrapping Up
Accurate demand forecasting is crucial for manufacturers as it plays a pivotal role in optimizing production, inventory management, and resource allocation. By leveraging historical data effectively, manufacturers can improve their forecasting accuracy and reap several benefits.
Historical data serves as a valuable foundation for demand forecasting models, providing insights into past demand patterns, trends, and fluctuations. It allows manufacturers to understand market dynamics, consumer behavior, and external factors that influence demand. However, challenges such as data availability, data quality issues, and the need to incorporate dynamic factors must be addressed to ensure accurate forecasting.
To enhance demand forecasting accuracy, manufacturers can incorporate external data sources and market intelligence, apply advanced forecasting techniques and algorithms, implement real-time data analytics and monitoring, utilize predictive analytics tools and software, and collaborate with supply chain partners. Furthermore, these strategies enable manufacturers to make data-driven decisions, optimize their operations, and respond effectively to changing market conditions.
Successful implementation of historical data-driven demand forecasting can be observed through case studies of companies like Walmart and Amazon. These companies have achieved benefits such as efficient inventory management, personalized recommendations, dynamic pricing, and improved customer satisfaction by leveraging historical data effectively.
In conclusion, historical data plays a crucial role in accurate demand forecasting for manufacturers. By leveraging historical data, implementing advanced analytics techniques, and incorporating external factors, manufacturers can enhance their forecasting accuracy, make informed decisions, improve customer satisfaction, and increase profitability.
As the manufacturing landscape continues to evolve, harnessing the power of historical data will be essential for manufacturers to stay competitive and thrive in the market.
How Deskera Can Assist You?
Deskera ERP and MRP systems help you to keep your business units organized. The system's key features of demand forecasting with Deskera include as follows:
- Establish Demand Forecasting Process
- Automate Data Collection
- Monitor Market Trends
- Analyze Historical Data
- Estimate Future Demand
- Adjust Production Levels
- Manage Supply Chain...and much more!
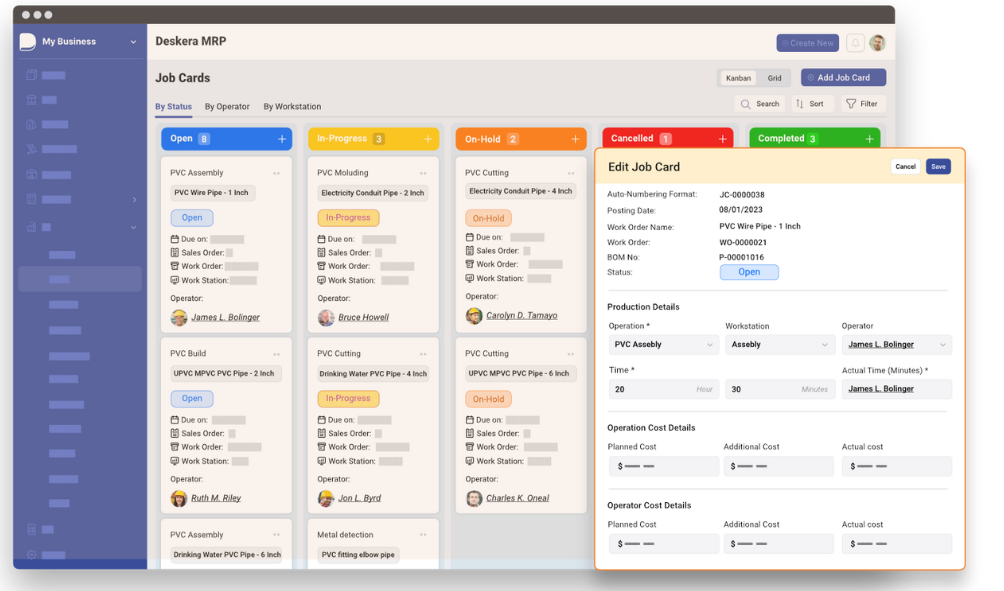
- Deskera's integrated financial planning tools enable investors to better plan and track their investments. It can assist investors in making faster and more accurate decisions.
- Deskera Books allows you to better manage your accounts and finances. Maintain good accounting practices by automating tasks like billing, invoicing, and payment processing.
- Deskera CRM is a powerful solution that manages your sales and helps you close deals quickly. It not only enables you to perform critical tasks like lead generation via email, but it also gives you a comprehensive view of your sales funnel.
- Deskera People is a straightforward tool for centralizing your human resource management functions.
Final Takeaways
We've arrived at the last section of this guide. Let's have a look at some of the most important points to remember:
- Accurate demand forecasting is a critical aspect of success for manufacturers in today's competitive marketplace. The ability to anticipate and predict customer demand enables manufacturers to optimize their operations, streamline production, minimize inventory costs, and make informed business decisions.
- The impact of effective demand forecasting extends beyond operational efficiencies. Manufacturers can strategically plan their resource allocation, procurement, and supply chain activities based on reliable demand forecasts.
- Manufacturers operate within complex supply chains, involving multiple suppliers, distributors, and intermediaries. Demand forecasting becomes more challenging when manufacturers must consider the dynamics of the entire supply chain, including lead times, transportation, inventory levels, and production capacities across various nodes.
- Real-time data analytics and monitoring enable manufacturers to capture up-to-date information and adjust forecasts accordingly. By integrating real-time data streams, such as point-of-sale data, customer feedback, or online sales data, manufacturers can gain insights into demand fluctuations and make timely adjustments to their forecasts.
- Demand forecasting guides manufacturers in allocating their resources effectively, such as labor, raw materials, and production capacities. It enables them to plan their resource utilization based on anticipated demand, leading to efficient resource allocation and cost optimization.
- Seasonality refers to the predictable patterns of demand that repeat on a seasonal basis. Many industries experience fluctuations in demand due to factors such as weather, holidays, or cultural events. Accurate forecasting requires recognizing these seasonal patterns and adjusting forecasts accordingly.
- Sales data is one of the most fundamental types of historical data used in forecasting. It includes information about the quantities sold, revenue generated, and customer purchasing patterns over a specific period. Sales data provides insights into historical demand levels and helps identify any recurring patterns or seasonality.
- Historical data enables manufacturers to analyze past demand fluctuations and understand their causes. By identifying factors that lead to demand volatility, manufacturers can adjust their forecasting models and develop contingency plans to mitigate the impact of future fluctuations.
Related Articles
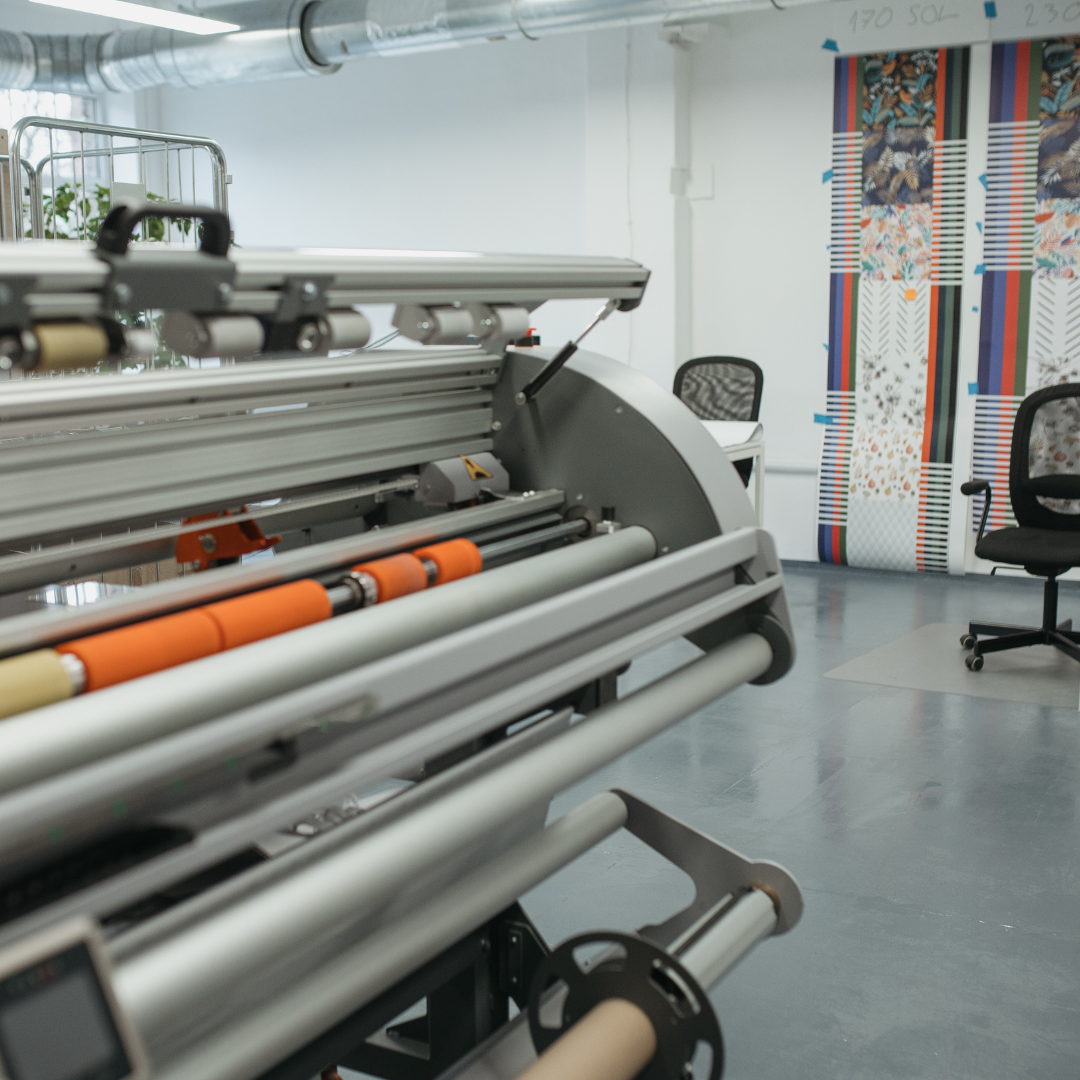
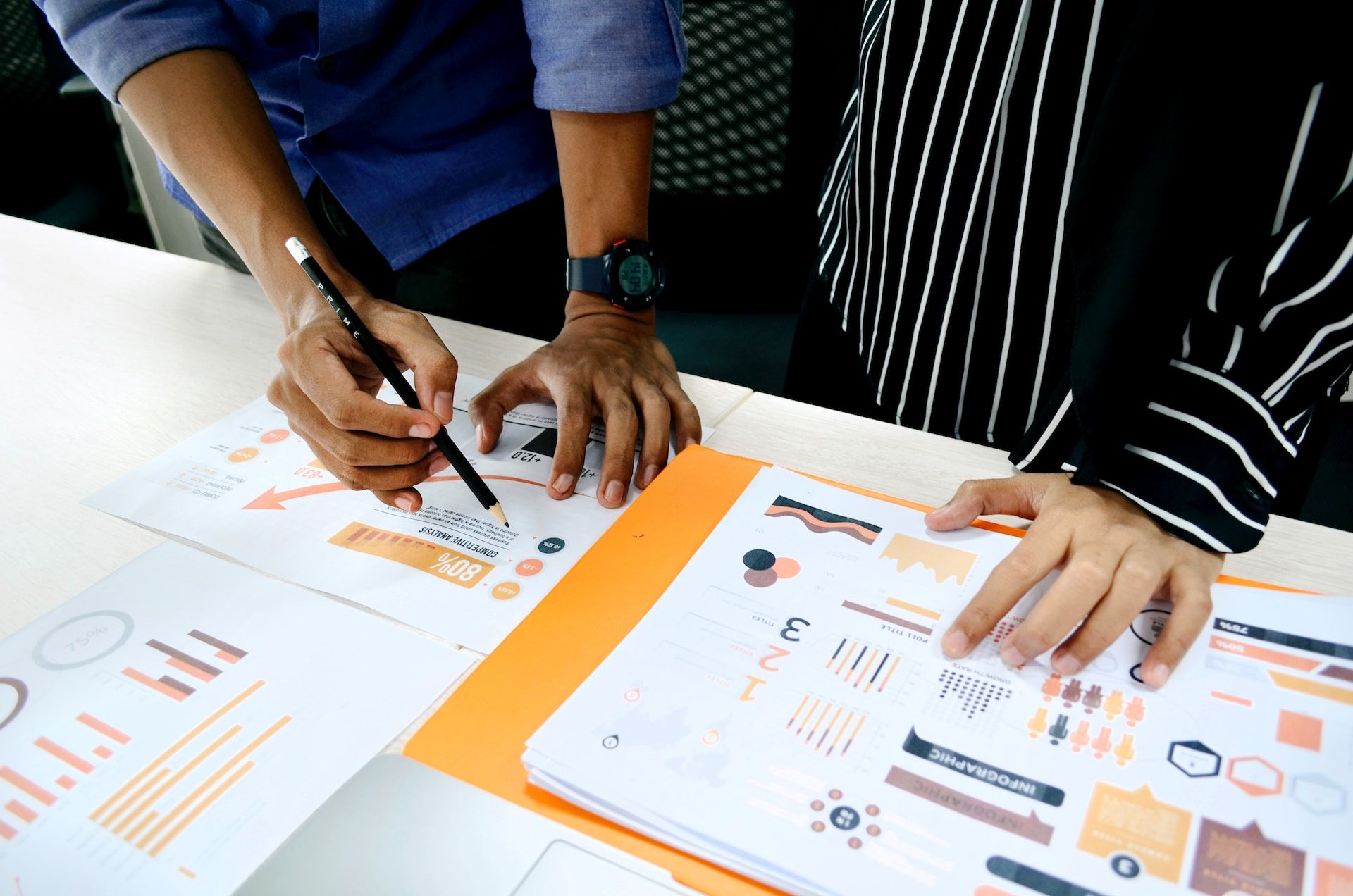
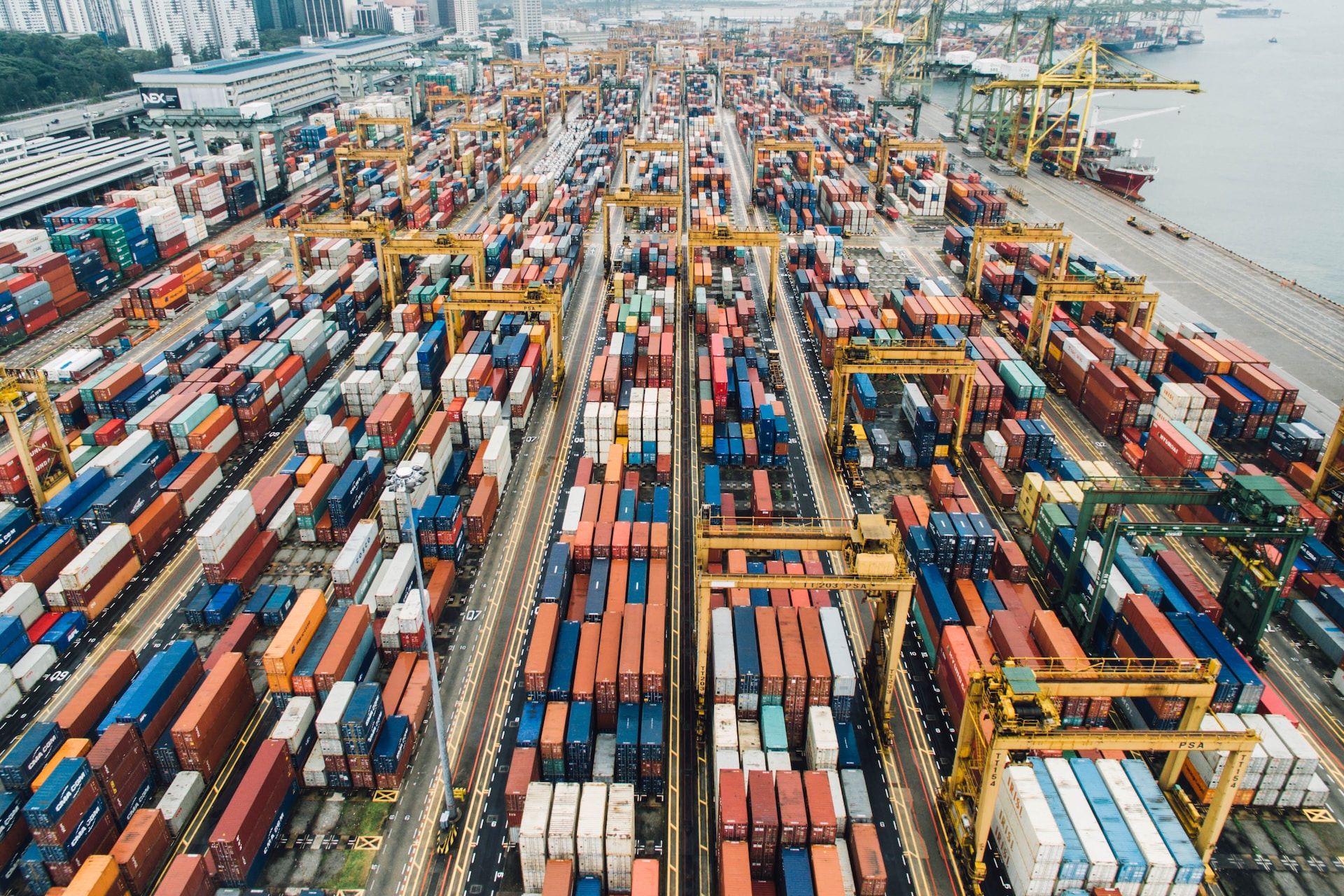
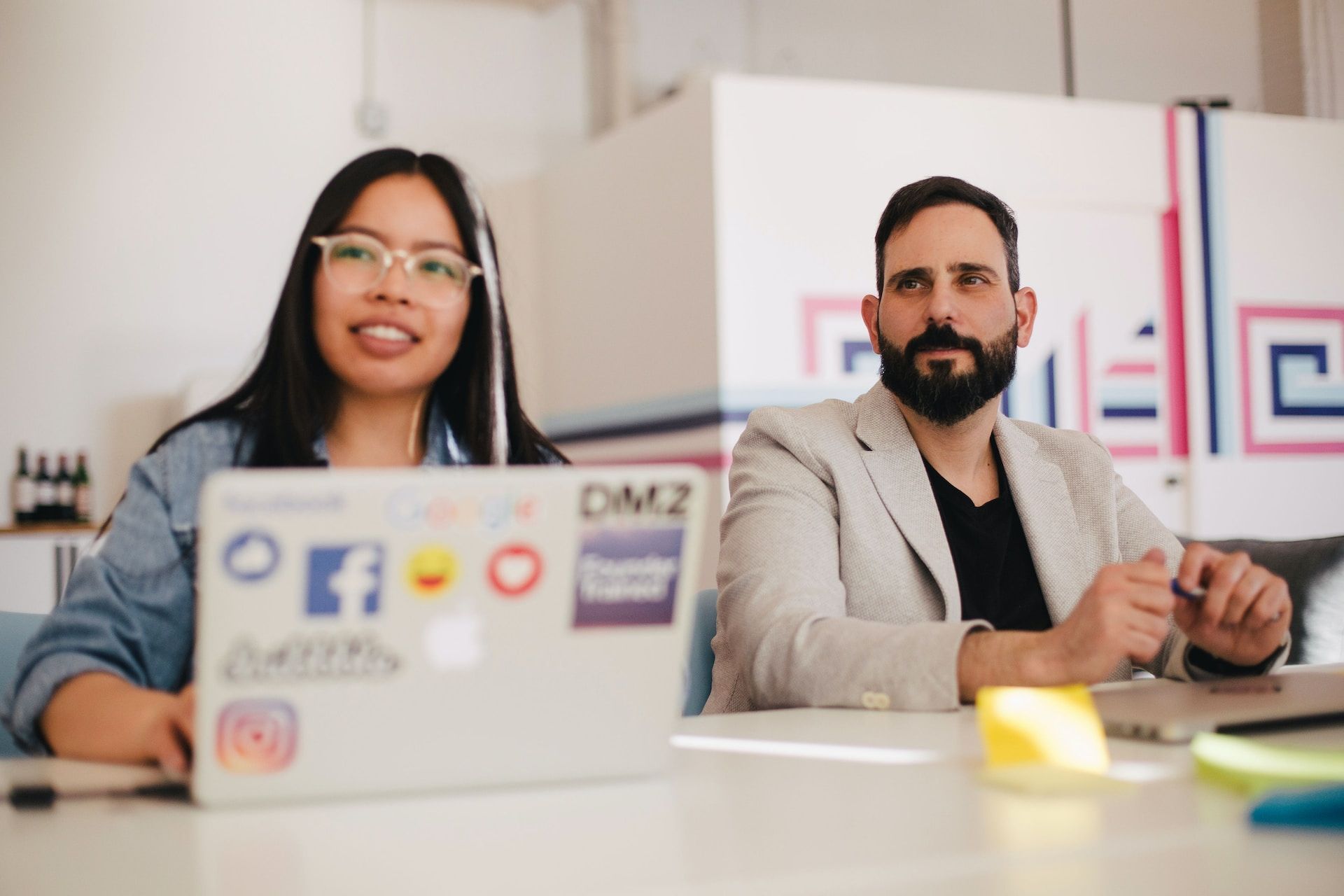
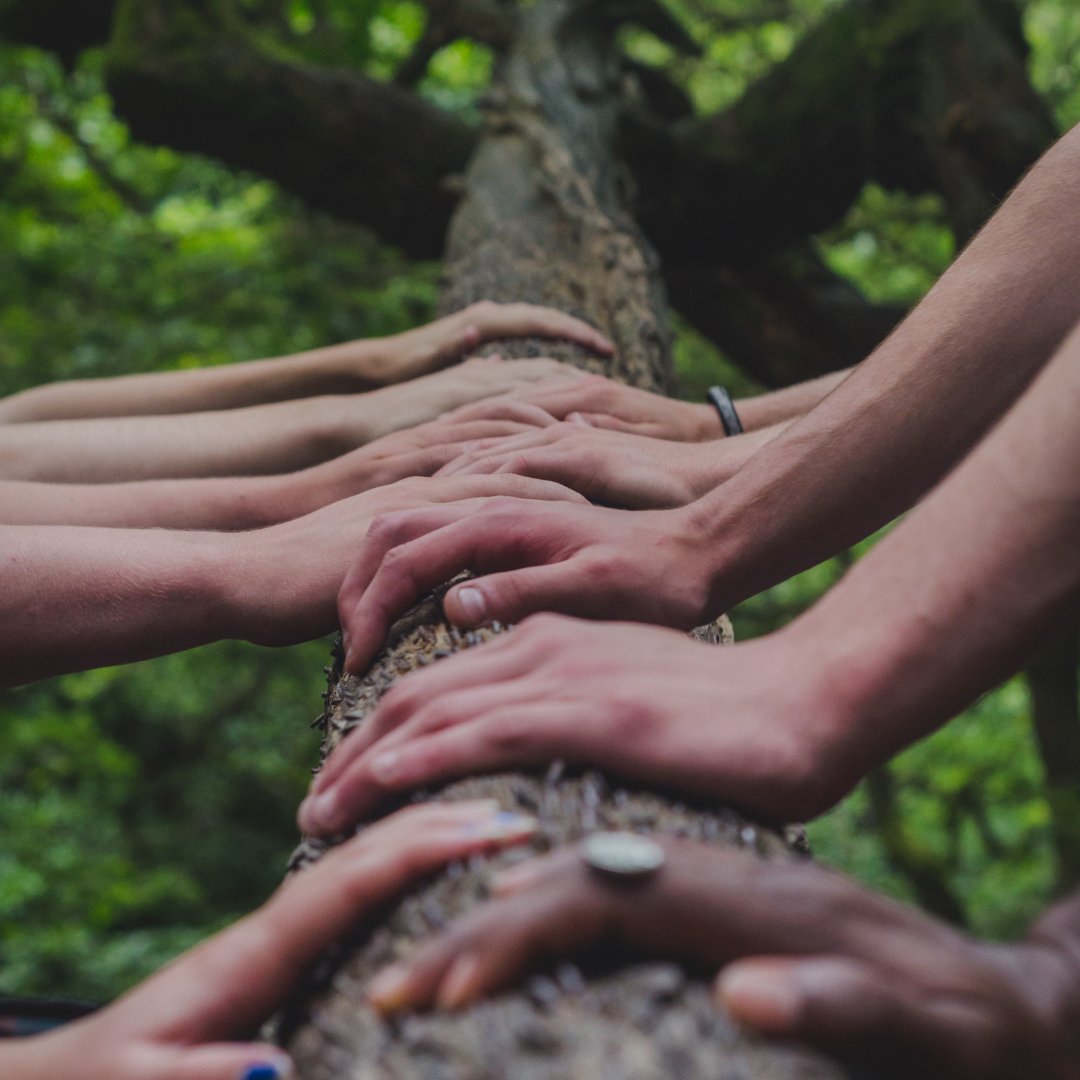