In today's rapidly evolving industrial landscape, where competition is fierce and consumer demands are ever-changing, the art of manufacturing has transformed into a science of precision. Amid this transformation, the integration of advanced technologies and data-driven strategies has become paramount, and one such strategy stands out: Demand Forecasting.
Did you know that inaccurate demand forecasts can lead to staggering financial losses? According to recent studies, businesses experience an average forecast error of 10-20%, resulting in excess inventory costs reaching up to 45% of total inventory value. These statistics underscore the critical role demand forecasting plays in modern manufacturing.
At its core, demand forecasting involves predicting future customer demand for products with a high degree of accuracy. This predictive power not only facilitates efficient inventory management but also empowers manufacturers to allocate resources effectively, streamline production, and enhance customer satisfaction.
From qualitative analysis of market trends to harnessing the power of advanced machine learning models, demand forecasting equips businesses with the tools they need to navigate the complex interplay between supply and demand.
As we embark on this enlightening journey, we'll delve into various facets of demand forecasting, exploring its different methodologies, benefits, and real-world applications. We'll also navigate the challenges that manufacturers face in implementing robust forecasting strategies and unveil best practices that can revolutionize manufacturing processes.
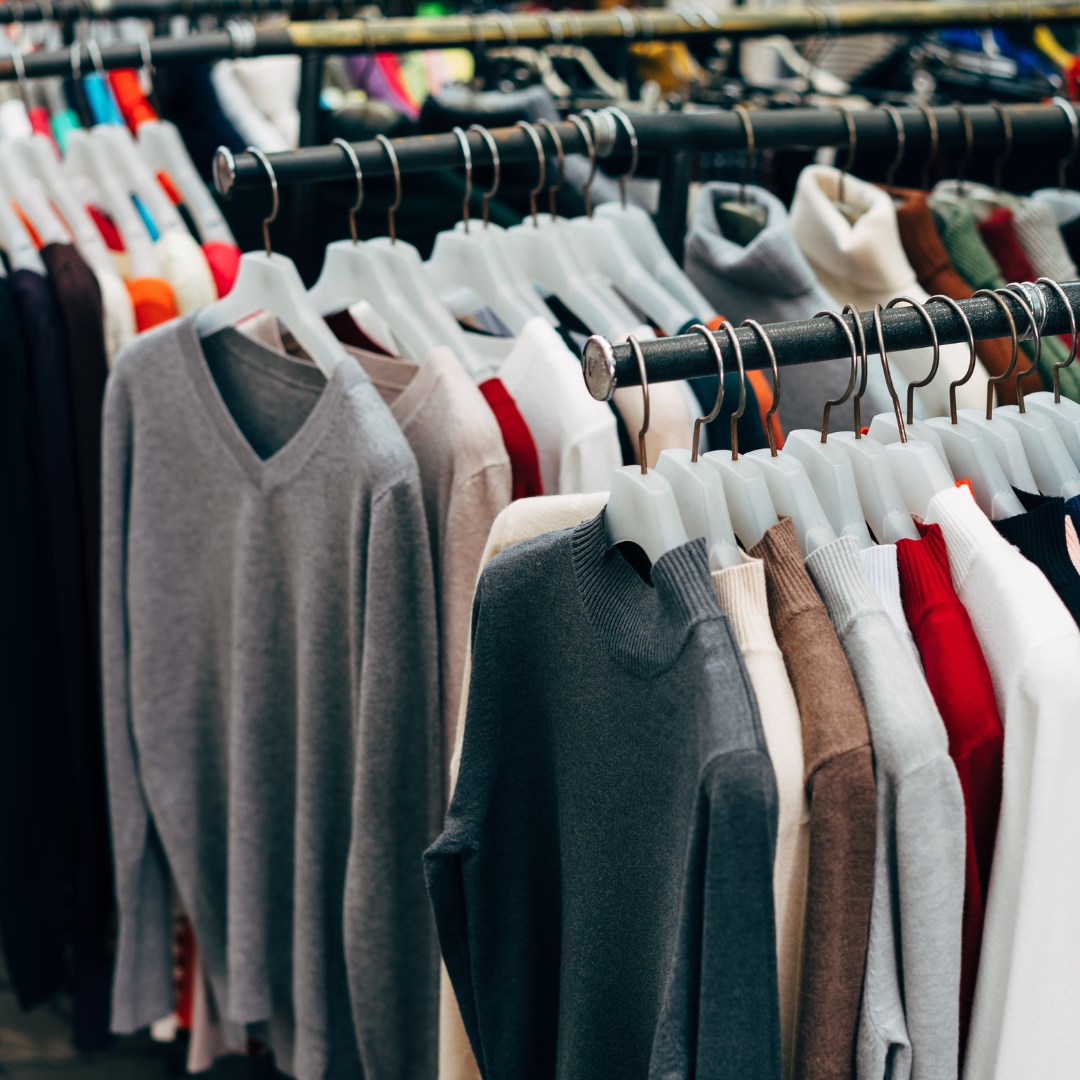
Get ready to uncover the secrets behind successful demand forecasting, where data-driven insights meet innovation, enabling manufacturers to not only meet market demands but also to stay ahead of the curve.
- Importance of Manufacturing Process Optimization
- Role of Demand Forecasting in Manufacturing
- Understanding Demand Forecasting
- Benefits of Demand Forecasting in Manufacturing
- Integrating Demand Forecasting into Manufacturing Processes
- Challenges in Demand Forecasting for Manufacturing
- Best Practices for Optimizing Manufacturing through Demand Forecasting
- Future Trends in Demand Forecasting and Manufacturing
- Conclusion
- Key Takeaways
- Related Articles
Importance of Manufacturing Process Optimization
In the realm of modern manufacturing, the quest for operational excellence has become an unending pursuit. The essence of this pursuit lies in the optimization of manufacturing processes, a strategic imperative that can make or break a company's competitive edge.
Manufacturing process optimization encompasses a systematic approach to refining every aspect of production, from resource utilization to workflow efficiency, with the ultimate goal of achieving higher productivity, reduced costs, and improved quality.
Intriguingly, statistics highlight the profound impact that optimized manufacturing processes can have on a business's bottom line. Research suggests that companies that effectively optimize their production processes can experience up to a 20% increase in overall efficiency and a 15% reduction in operational costs. Moreover, the ripple effects extend beyond the factory floor.
Streamlined processes lead to shorter lead times, faster order fulfillment, and heightened agility in responding to market fluctuations—a vital trait in today's dynamic business landscape.
The impact of manufacturing process optimization extends far beyond the confines of operational efficiency. It also contributes significantly to sustainable practices by minimizing waste, energy consumption, and environmental impact. In fact, companies that adopt environmentally conscious manufacturing processes often find themselves better aligned with evolving consumer preferences and regulatory standards, fostering long-term brand loyalty and goodwill.
However, the journey towards manufacturing process optimization is not without its challenges. Complex supply chains, intricate production networks, and the ever-present pressure to balance cost-effectiveness with innovation create a complex landscape. This is where the symbiotic relationship between demand forecasting and manufacturing optimization comes into play.
The ability to predict future demand accurately allows manufacturers to fine-tune their processes, align production capacities with actual market needs, and strategically allocate resources.
In the subsequent sections of this guide, we will explore a pivotal facet of this relationship: demand forecasting. By unraveling the intricacies of demand forecasting and its profound impact on manufacturing, we aim to equip you with the insights and tools needed to not only thrive in the market but also to lead the charge in shaping the future of manufacturing through intelligent optimization strategies.
Role of Demand Forecasting in Manufacturing
At the heart of successful manufacturing lies the ability to anticipate, adapt, and excel in meeting customer demands. This is where demand forecasting emerges as a guiding light, illuminating the path to efficient production, optimal resource allocation, and enhanced customer satisfaction. Demand forecasting is the compass that enables manufacturers to navigate the complex terrain of supply and demand, ensuring that production meets market needs with precision.
In an era characterized by rapid technological advancements and ever-evolving consumer preferences, manufacturers cannot afford to operate blindly, producing goods without a clear understanding of future demand. This is precisely where demand forecasting steps in, offering insights rooted in data analysis, historical patterns, and market trends. By harnessing this predictive power, manufacturers can make informed decisions that resonate across the entire supply chain.
Consider this: inaccurate forecasts can lead to overstocking, where surplus products accumulate dust on shelves, tying up valuable resources and capital. Conversely, underestimating demand can result in stockouts, leaving customers frustrated and potentially seeking alternatives. These scenarios are not mere hypotheticals; studies reveal that businesses often struggle with demand forecast errors as high as 10-20%.
The role of demand forecasting, however, extends beyond averting inventory woes. It acts as the bridge between various departments, aligning production, procurement, and distribution strategies. Accurate forecasts enable manufacturers to optimize inventory levels, fine-tune production schedules, and even pave the way for lean manufacturing practices. Ultimately, this synchronization leads to cost savings, improved operational efficiency, and a competitive edge in an increasingly demanding market.
In the context of globalization and interconnected supply chains, the role of demand forecasting takes on even greater significance. Fluctuations in demand in one part of the world can trigger a chain reaction that reverberates across the entire network. Here, demand forecasting provides the necessary foresight to adapt and respond swiftly, preventing bottlenecks and disruptions.
As we proceed through this guide, we will delve deeper into the intricacies of demand forecasting. We will explore the diverse methodologies and tools available, uncover the challenges manufacturers face in this endeavor, and highlight real-world examples where demand forecasting has been a game-changer.
By the time we conclude, you will be equipped with a comprehensive understanding of how demand forecasting is not just an ancillary process but a cornerstone of effective manufacturing process optimization.
Understanding Demand Forecasting
In the dynamic landscape of modern manufacturing, where staying ahead of market trends is essential, the art of predicting customer demand has become a critical cornerstone. This section delves into the core principles of demand forecasting, unveiling the methodologies that empower manufacturers to glimpse into the future and make informed decisions.
From qualitative insights to quantitative analytics, understanding demand forecasting is the key to unraveling the intricate dance between supply and demand.
A. Definition and concept of demand forecasting
At its essence, demand forecasting is the art and science of predicting future customer demand for products or services. It's a proactive approach that empowers manufacturers to peer into the horizon and anticipate market trends, enabling them to make well-informed decisions about production, resource allocation, and inventory management.
Demand forecasting is more than a mere statistical exercise; it's a dynamic process that combines historical data analysis, market intelligence, and predictive modeling. By examining past sales patterns, identifying trends, and considering external factors that influence demand, manufacturers can create forecasts that guide their operational strategies.
Imagine a retail business aiming to stock its shelves with the right amount of products during the holiday season. Through demand forecasting, they can analyze historical data from previous years, observe any seasonal spikes, and take into account factors like changing consumer preferences or economic conditions. Armed with these insights, they can ensure they have the right products available at the right time, avoiding the pitfalls of excess inventory or stockouts.
Demand forecasting operates on various time scales, ranging from short-term to long-term. Short-term forecasts might focus on weeks or months ahead, aiding in immediate production planning and inventory adjustments. On the other hand, long-term forecasts provide a broader view, helping manufacturers make strategic decisions about capacity expansion, new product introductions, and market positioning.
The concept of demand forecasting is rooted in the understanding that accurate predictions lead to optimized processes. It's a fusion of historical analysis and predictive analytics, powered by data-driven insights that bring manufacturers closer to aligning their offerings with market needs.
As we journey deeper into the realm of demand forecasting, we'll explore the different methodologies and techniques that enable manufacturers to refine their crystal ball and navigate the complexities of the manufacturing landscape with precision.
B. Types of demand forecasting methods
Demand forecasting methods can be broadly classified into two categories: qualitative methods and quantitative methods. These approaches vary in their complexity, data requirements, and the level of subjectivity involved. Let's delve into each category:
Qualitative Methods: Qualitative methods are employed when historical data might be scarce or when the business environment is highly uncertain, making statistical analysis less reliable. These methods rely on expert judgment, market knowledge, and subjective assessments to forecast demand. Some common qualitative methods include:
- Market Research: Gathering insights from customer surveys, focus groups, and interviews to gauge preferences, trends, and potential shifts in demand.
- Delphi Method: Utilizing a panel of experts who provide individual forecasts, which are then aggregated and refined through multiple iterations to arrive at a consensus.
- Scenario Analysis: Creating multiple scenarios based on different assumptions about future market conditions, helping to anticipate how demand might vary under various circumstances.
Qualitative methods are particularly useful when introducing new products or entering unfamiliar markets where historical data is limited. However, they can be prone to bias and subjectivity, making them most effective when combined with other forecasting techniques.
Quantitative Methods: Quantitative methods rely on historical data and mathematical models to predict future demand. These methods are well-suited for situations where there's a substantial volume of past sales data available. Some commonly used quantitative methods include:
- Time-Series Models: These models analyze historical data to identify patterns, trends, and seasonal variations. Examples include moving averages, exponential smoothing, and autoregressive integrated moving average (ARIMA) models.
- Causal Models: These models correlate demand with other factors that might influence it, such as economic indicators, marketing expenditures, or population growth. Linear regression is a common technique in this category.
- Machine Learning Models: Leveraging algorithms like random forests, support vector machines, or neural networks to extract patterns from historical data and make predictions. Machine learning methods can handle large datasets and capture complex relationships.
Quantitative methods offer the advantage of objectivity and the ability to provide statistically derived forecasts. However, they require accurate and sufficient historical data to produce reliable results.
The choice between qualitative and quantitative methods depends on the specific circumstances, data availability, and the level of accuracy required. Often, a combination of these approaches, known as hybrid forecasting, can provide a more robust and accurate forecast by leveraging the strengths of both qualitative and quantitative methods.
As we proceed, we'll explore these methods in greater detail, equipping you with the knowledge needed to choose the most appropriate forecasting approach for your manufacturing context.
C. Factors influencing demand forecasting accuracy
Demand forecasting accuracy hinges on a multitude of factors that shape the intricate dance between past, present, and future market dynamics. Understanding these factors is essential for manufacturers seeking to enhance their forecasting precision. Let's explore the key elements that influence demand forecasting accuracy:
Historical Data Analysis: Historical data forms the foundation of demand forecasting, serving as a compass for predicting future patterns. The accuracy of forecasts heavily relies on the quality, completeness, and relevance of this historical data. Important considerations include:
- Data Quality: Inaccurate or incomplete data can lead to flawed forecasts. Ensure that the historical data is clean, free from errors, and accurately recorded.
- Data Consistency: Consistency in data collection methods and time intervals is crucial for detecting meaningful patterns and trends.
- Data Relevance: Ensure that the historical data used for forecasting aligns with the current market conditions and product offerings. Outdated data might lead to misguided predictions.
Market Trends and External Factors: The business landscape is influenced by a myriad of external factors that impact consumer behavior and demand. These factors introduce a layer of complexity into forecasting that requires careful consideration:
- Economic Indicators: Economic conditions, such as GDP growth, inflation rates, and consumer spending, can significantly influence demand for products and services.
- Competitor Actions: Competitors' strategies, product launches, and market positioning can directly affect your product's demand trajectory.
- Regulatory Changes: Shifts in regulations, trade policies, or industry standards can lead to sudden changes in demand patterns.
- Technological Advancements: Technological breakthroughs or shifts in consumer preferences can lead to rapid changes in demand for certain products.
Seasonality and Cyclic Patterns: Many products experience recurring demand fluctuations tied to seasons, holidays, or other cyclic patterns. Recognizing and accounting for these variations is crucial for accurate forecasting:
- Seasonal Demand: Products like winter clothing or holiday-themed items often exhibit predictable peaks and troughs in demand tied to specific times of the year.
- Cyclic Patterns: Some products experience longer cycles of demand, which might be tied to economic cycles, fashion trends, or other non-seasonal influences.
- Failing to capture these seasonal and cyclic patterns can lead to underestimating demand during peak periods and overestimating it during slower times, resulting in costly inventory imbalances.
As manufacturers delve into demand forecasting, a holistic understanding of these influencing factors can guide the selection of appropriate forecasting methods and the fine-tuning of forecasting models. Embracing historical data, keeping a vigilant eye on market trends, and accounting for seasonality and cyclic patterns are the cornerstones of accurate demand predictions.
Benefits of Demand Forecasting in Manufacturing
This section unveils the far-reaching benefits of demand forecasting, showcasing how it transforms production processes, optimizes resource allocation, and propels businesses toward a future defined by efficiency and customer satisfaction.
From inventory management to customer loyalty, demand forecasting transcends its role as a prediction tool to become a cornerstone of manufacturing success. We shall uncover the myriad advantages that demand forecasting bestows upon the process of manufacturing.
A. Improved inventory management
Demand forecasting's impact on inventory management is akin to a finely tuned orchestra, where every note is orchestrated with precision. By accurately predicting future demand, manufacturers can optimize their inventory levels, striking a delicate balance between having enough stock to meet customer needs while minimizing excess carrying costs.
This delicate equilibrium translates into reduced holding costs, freeing up capital that can be reinvested in core business operations. Furthermore, an accurate forecast empowers inventory managers to plan replenishment cycles effectively, reducing the chances of costly stockouts or overstocking scenarios.
B. Efficient resource allocation
The art of manufacturing rests upon the optimal allocation of resources—materials, labor, equipment, and time. Demand forecasting lends a scientific edge to this allocation, allowing manufacturers to allocate resources based on predicted demand patterns.
With foresight into future demand, production schedules can be optimized, ensuring that resources are allocated when and where they're needed most. This leads to streamlined production processes, reduced downtime, and heightened operational efficiency.
C. Minimized production costs
Cost efficiency is a perpetual goal in manufacturing, and demand forecasting serves as a compass to navigate this pursuit. By accurately estimating future demand, manufacturers can adjust their production volumes to match actual market needs. This minimizes the risk of overproducing, which often results in excess inventory and associated costs.
Conversely, underproduction can lead to missed sales opportunities. Demand forecasting mitigates these risks, aligning production levels with actual demand, thereby curbing unnecessary production costs.
D. Enhanced customer satisfaction
Customer satisfaction is the bedrock upon which business success is built. Accurate demand forecasting ensures that manufacturers are equipped to meet customer expectations consistently.
By maintaining the right products in stock, manufacturers can fulfill orders promptly, minimize delays, and foster positive customer experiences. This, in turn, bolsters brand loyalty and encourages repeat business.
E. Reduction in stockouts and overstocking
Stockouts and overstocking are two sides of the same coin, both carrying financial implications and customer dissatisfaction. Demand forecasting acts as a guardian against these challenges. With accurate predictions, manufacturers can avoid stockouts by having adequate inventory to meet demand spikes.
Simultaneously, overstocking is mitigated as manufacturers refrain from producing excess units that might languish in storage. This balance ensures that products are available when customers want them, eliminating the revenue loss associated with stockouts and the cost burdens of overstocking.
In essence, demand forecasting transforms manufacturing from a reactive endeavor to a proactive, data-driven practice. It empowers manufacturers to anticipate and adapt, resulting in more efficient operations, cost savings, and ultimately, a satisfied customer base.
Integrating Demand Forecasting into Manufacturing Processes
In the intricate web of manufacturing operations, where precision and efficiency reign supreme, the fusion of demand forecasting emerges as a linchpin that binds strategy with execution. This section delves into the intricacies of seamlessly integrating demand forecasting into the very fabric of manufacturing processes. From data collection to model selection and forecast analysis, we unravel the steps that transform raw data into actionable insights.
By bridging the gap between foresight and production, demand forecasting empowers manufacturers to synchronize their efforts with market demands, optimizing resource allocation and ensuring products reach customers with impeccable timing.
A. Data collection and preparation
In the realm of demand forecasting, the accuracy and reliability of predictions hinge upon the quality of data used as raw material. The process of data collection and preparation lays the foundation for informed decision-making. This involves meticulous attention to detail and a strategic approach to gathering and refining data. Let's explore the key components of this process:
Gathering Historical Sales Data: Historical sales data is the bedrock of demand forecasting. It provides insights into past trends, seasonal variations, and market behavior. This data encompasses information on product sales, dates of purchase, and quantities sold. Accurate historical data facilitates the identification of patterns and cycles, helping to fine-tune forecasting models. This information is typically drawn from sales records, transaction logs, and databases.
For instance, a manufacturer aiming to forecast demand for a particular product can retrieve historical sales data over a specific period. This data forms the basis for analyzing demand fluctuations, identifying growth trends, and detecting any seasonality inherent to the product.
Incorporating Market Intelligence: Historical data, while crucial, often doesn't capture the full picture. External factors like market trends, economic conditions, and competitor activities play a significant role in shaping demand. Incorporating these external influences—often referred to as "market intelligence"—strengthens the accuracy of forecasts.
Market intelligence involves gathering information from diverse sources such as industry reports, economic indicators, consumer surveys, and social media trends. This supplementary data enriches the forecasting process by capturing contextual information that historical data might overlook.
For example, an electronics manufacturer might factor in trends in the tech industry, consumer sentiment regarding new technologies, and competitor announcements when forecasting demand for a new smartphone model.
Accurate data collection and preparation set the stage for robust demand forecasting. It ensures that forecasting models are well-informed and capable of capturing both historical patterns and real-time market dynamics. By amalgamating historical sales data and market intelligence, manufacturers empower themselves to make predictions that are attuned to the nuanced interplay between past trends and current external influences.
B. Selecting appropriate forecasting models
Selecting the right forecasting model is akin to choosing the perfect tool for a precise task. It requires a deep understanding of data characteristics, business context, and the nuances of different model types. The process of model selection is critical, as it determines the accuracy and reliability of the forecasts.
Let's delve into the two primary categories of forecasting models:
Time-Series Models (e.g., ARIMA, Exponential Smoothing): Time-series models are a staple in demand forecasting, particularly when dealing with historical data that exhibits patterns over time. These models focus on analyzing data points in chronological order to uncover trends, seasonality, and cyclic patterns. Two prominent time-series models are:
- ARIMA (AutoRegressive Integrated Moving Average): ARIMA models combine autoregressive and moving average components to capture linear relationships and remove seasonality and trends from data.
- Exponential Smoothing: This method assigns exponentially decreasing weights to older observations, prioritizing recent data. Various forms of exponential smoothing models exist, including simple, double, and triple exponential smoothing.
Time-series models are valuable when historical data showcases consistent patterns, making them suitable for short-term forecasts. However, they might struggle with highly irregular data or situations where external factors significantly impact demand.
Machine Learning Models (e.g., Random Forest, Neural Networks): Machine learning has injected new vigor into demand forecasting, especially when historical patterns are complex or influenced by multiple variables. These models leverage algorithms to identify intricate relationships in data and predict future patterns.
Two notable machine learning models used in demand forecasting are:
- Random Forest: This ensemble learning technique constructs multiple decision trees and combines their predictions to improve accuracy. It's adept at handling non-linear relationships and can incorporate various predictors.
- Neural Networks: Inspired by the human brain, neural networks consist of interconnected nodes that process information. They excel in capturing complex patterns and relationships but can be computationally intensive.
Machine learning models shine in scenarios where data relationships are intricate, nonlinear, or influenced by numerous external factors. They offer adaptability to changing conditions and can be fine-tuned to capture the subtleties of demand dynamics.
The choice between time-series and machine learning models hinges on factors such as data characteristics, forecasting horizon, and the level of complexity in demand patterns. A nuanced understanding of these model types and their applicability to different scenarios allows manufacturers to wield forecasting tools that align with their specific needs, ultimately driving accurate and actionable predictions.
C. Forecast generation and analysis
Forecast generation and analysis is the culmination of the demand forecasting process, where the insights derived from historical data and chosen models are transformed into actionable predictions. This step involves producing forecasts for different time horizons, evaluating their accuracy, and fine-tuning the forecasting models for ongoing improvement. Let's delve into the intricacies of this pivotal phase:
Generating Short-Term and Long-Term Forecasts: Demand forecasting operates on multiple time scales, each catering to different business needs. Short-term forecasts focus on immediate weeks or months, aiding in tactical decisions such as production scheduling and inventory management. Long-term forecasts extend further into the future, helping manufacturers shape strategic decisions like capacity planning, new product introductions, and market positioning.
For instance, a fashion retailer might generate a short-term forecast to ensure that the right clothing items are available during the upcoming holiday season. Simultaneously, a car manufacturer might generate long-term forecasts to align production capacities with anticipated demand shifts over the next few years.
Assessing Forecast Accuracy and Adjusting Models: The litmus test of a successful forecasting endeavor lies in assessing the accuracy of predictions against actual outcomes. Forecast accuracy metrics quantify the deviation between forecasted values and real data. Common accuracy metrics include Mean Absolute Error (MAE), Mean Squared Error (MSE), and Root Mean Squared Error (RMSE). These metrics provide a quantifiable measure of how well the forecasting model performs.
The process of assessing forecast accuracy doesn't stop at metrics; it involves a continuous loop of model refinement. If forecasts consistently deviate from actual values, adjustments are made to the forecasting model. This might involve recalibrating model parameters, incorporating new data, or even switching to a different model type.
Adjusting models based on forecast accuracy analysis ensures that forecasts remain aligned with changing market dynamics. It's a dynamic process that adapts to shifts in consumer behavior, economic conditions, and other influencing factors.
Forecast generation and analysis form the heartbeat of demand forecasting, transforming raw data and model outputs into actionable insights. It's the stage where the rubber meets the road, and manufacturers can harness the power of accurate predictions to steer their operational strategies.
By generating forecasts across different time horizons and iteratively improving models based on accuracy assessments, manufacturers lay the groundwork for agile and efficient decision-making in the face of ever-evolving market conditions.
Challenges in Demand Forecasting for Manufacturing
This section delves into the intricacies of demand forecasting's often unpredictable landscape, shedding light on the hurdles that manufacturers encounter in their pursuit of accurate predictions. From volatile market conditions to data quality dilemmas, we unravel the obstacles that underscore the need for robust forecasting methodologies. Join us as we navigate through the challenges that shape the demand forecasting landscape and explore strategies to overcome them.
A. Volatile market conditions
Volatile market conditions present a formidable challenge in demand forecasting, as they introduce rapid and often unpredictable shifts in consumer behavior. Economic downturns, geopolitical events, and unforeseen trends can swiftly disrupt demand patterns.
Navigating such volatility demands not only accurate historical data but also the agility to adapt to sudden changes. Manufacturers must implement forecasting models that can capture these abrupt fluctuations and identify underlying trends, allowing them to pivot swiftly in response to evolving market dynamics.
B. Data quality and availability
Demand forecasting hinges on the quality and availability of data. Inaccurate or incomplete historical data can lead to flawed predictions, while data scarcity can hinder the application of certain forecasting techniques. Ensuring data accuracy through consistent data collection methods, validation processes, and dealing with missing data points is crucial.
Manufacturers may also face challenges in integrating data from various sources, requiring data normalization and cleansing to ensure accurate insights for forecasting models.
C. Seasonal demand variations
Many industries experience seasonal demand variations that pose unique forecasting challenges. Predicting demand for products influenced by weather, holidays, or cultural events requires specialized models that account for these recurring patterns.
Accurate forecasting in such scenarios involves capturing the amplitude and timing of these seasonal variations, adjusting inventory and production schedules accordingly to prevent stockouts during peak demand and minimize overstocking during slower periods.
D. Incorporating new product launches
Introducing new products disrupts established demand patterns, and forecasting for these products can be particularly challenging due to a lack of historical data. Traditional methods might fall short when faced with this uncertainty.
Manufacturers must employ approaches that consider analogs or similarities to existing products, incorporate market research, and engage cross-functional teams to gather insights and inform forecasts. The challenge lies in accurately estimating how the market will respond to the new offering.
E. Addressing demand uncertainty
Demand uncertainty stems from factors such as rapidly changing consumer preferences, unforeseen events, or shifts in competition. Forecasting in the face of uncertainty requires flexibility and the ability to model various scenarios. Manufacturers might employ simulation techniques, sensitivity analysis, or scenario planning to gauge potential outcomes.
Balancing risk and opportunity becomes a critical aspect of forecasting, as addressing uncertainty involves making informed decisions while acknowledging the inherent unpredictability of certain market forces.
In the dynamic landscape of demand forecasting, these challenges underscore the need for a multi-faceted approach. From harnessing sophisticated models to embracing adaptive strategies, manufacturers can overcome these hurdles and attain accurate predictions that empower agile decision-making and efficient operations.
Best Practices for Optimizing Manufacturing through Demand Forecasting
This section unveils a blueprint for manufacturers seeking to harness the power of accurate predictions and transform them into actionable strategies. From fostering cross-functional collaboration to embracing cutting-edge analytics tools, these best practices form the linchpin that bridges foresight with execution. Let's explore a comprehensive guide to optimizing manufacturing processes through the strategic lens of demand forecasting, unlocking a realm of efficiency, cost savings, and customer satisfaction.
A. Collaborative approach between departments
A siloed approach within an organization can hinder accurate demand forecasting. A collaborative approach, on the other hand, fosters cross-functional communication and information sharing, enabling a more holistic view of demand drivers.
Departments like sales, marketing, operations, and finance must work in tandem, sharing insights, market trends, and customer feedback. This collaboration not only improves the accuracy of input data but also brings diverse perspectives that refine forecasting models and ensure alignment with overall business goals.
B. Continuous monitoring and model updating
Demand forecasting is not a one-time task; it's an ongoing process that demands vigilance. Continuous monitoring of forecast accuracy against actual outcomes is essential to identify deviations and trends.
If discrepancies arise, models need to be updated and refined accordingly. Incorporating new data points and adjusting parameters can enhance forecast accuracy, ensuring that models remain relevant in dynamic market conditions.
C. Sensitivity analysis for different scenarios
The future is inherently uncertain, making it imperative to consider various scenarios that might impact demand. Sensitivity analysis involves testing forecasts against different assumptions, such as changes in economic conditions or competitor behavior.
This technique helps manufacturers identify potential risks and opportunities, enabling them to prepare contingency plans and make informed decisions that withstand the unpredictability of the market.
D. Utilizing advanced analytics tools
The advent of advanced analytics tools empowers manufacturers to extract deeper insights from their data. Machine learning, artificial intelligence, and data visualization tools provide a more nuanced understanding of demand patterns.
These tools can capture complex relationships, identify hidden trends, and optimize model performance. Leveraging these tools can elevate forecasting accuracy and empower manufacturers to make more informed decisions.
E. Aligning forecasts with production capabilities
Accurate demand forecasting is only beneficial when it aligns with a manufacturer's production capabilities. Overestimating demand can lead to overproduction and unnecessary costs, while underestimating can result in stockouts and customer dissatisfaction.
Close collaboration between forecasting teams and production departments is crucial. Forecasters should consider production lead times, constraints, and capacities, ensuring that predicted demand aligns with the organization's operational capabilities.
By embracing these best practices, manufacturers can bridge the gap between anticipation and action. A collaborative culture, ongoing monitoring, scenario planning, advanced tools, and an alignment of forecasts with production capabilities create a harmonious ecosystem where demand forecasting is not just a prediction but a catalyst for optimized manufacturing processes.
Future Trends in Demand Forecasting and Manufacturing
As the manufacturing landscape continues to evolve, demand forecasting stands as a dynamic cornerstone poised to shape the future of production processes. This section ventures into the realm of what lies ahead, exploring the exciting trends that are poised to revolutionize the way manufacturers anticipate, adapt, and excel in meeting market demands.
From the integration of artificial intelligence and predictive analytics to the emergence of demand sensing technologies, we embark on a journey to unveil the trends that will define the intersection of demand forecasting and manufacturing innovation. Let's peer into the future and unravel the possibilities that await in this ever-evolving landscape.
A. AI and machine learning advancements
Artificial Intelligence (AI) and Machine Learning (ML) are poised to revolutionize demand forecasting and manufacturing processes. These technologies enable manufacturers to extract deeper insights from vast datasets, uncover complex patterns, and make more accurate predictions.
AI-driven models can adapt to changing market conditions, automatically adjusting parameters to reflect evolving trends. They can handle nonlinear relationships, capture nuances in consumer behavior, and identify correlations that might elude traditional methods.
Machine learning algorithms, such as neural networks and random forests, excel at recognizing patterns in data, making them particularly suited for forecasting tasks. Reinforcement learning techniques allow systems to learn and adjust strategies based on outcomes, enabling dynamic decision-making. With AI and ML, manufacturers can enhance demand forecasting accuracy, optimize production schedules, and proactively respond to shifts in consumer preferences.
B. Integration of IoT and Big Data
The Internet of Things (IoT) and Big Data have ushered in an era of unprecedented data availability. Smart sensors embedded in products, machinery, and supply chains generate a wealth of real-time data. This data, when harnessed effectively, provides manufacturers with granular insights into consumer behaviors, operational efficiencies, and production processes. By integrating IoT-generated data with demand forecasting, manufacturers can refine predictions based on real-time feedback, enhancing accuracy and adaptability.
Big Data analytics further amplify these benefits. The fusion of historical data with real-time insights offers a comprehensive view of market dynamics. Advanced analytics tools can process and analyze this vast data landscape, unveiling trends, anomalies, and correlations that impact demand. Manufacturers can proactively address demand fluctuations, optimize inventory management, and tailor production processes to align with real-world demand patterns.
C. Predictive analytics for supply chain optimization
Predictive analytics extends beyond demand forecasting to optimize the entire supply chain. By combining historical data, market intelligence, and real-time insights, manufacturers can predict not only demand but also potential supply chain disruptions. Predictive models can identify potential bottlenecks, resource shortages, and transportation delays. This proactive approach allows manufacturers to adjust their strategies in advance, mitigating the impact of unforeseen events.
Predictive analytics also enable manufacturers to identify optimal reorder points, reduce lead times, and enhance supplier collaboration. By utilizing predictive analytics to optimize the supply chain, manufacturers can streamline operations, reduce costs, and increase customer satisfaction through reliable and timely product delivery.
The integration of AI, IoT, Big Data, and predictive analytics reflects the forward momentum of manufacturing. These trends transcend conventional methods, propelling demand forecasting and manufacturing processes into a new era of adaptability, accuracy, and operational excellence.
Conclusion
Throughout this guide, we've navigated the terrain of demand forecasting, uncovering its essential role in guiding manufacturing operations toward excellence. From understanding the core concepts of demand forecasting to exploring its benefits and challenges, we've embarked on a journey that sheds light on the multifaceted nature of this practice.
Demand forecasting is not merely a predictive tool; it's a dynamic process that empowers manufacturers to anticipate and adapt to ever-changing market dynamics. Through collaborative efforts between departments, continuous monitoring, and the strategic use of advanced analytics tools, manufacturers can transcend traditional methods and embark on a path toward operational optimization.
The fusion of AI and machine learning, the integration of IoT and Big Data, and the utilization of predictive analytics promise to shape the future of demand forecasting, ensuring that manufacturers remain agile, accurate, and responsive in their pursuit of excellence.
As we conclude this guide, it's evident that demand forecasting is not a static concept but a living force that propels manufacturing into an era defined by data-driven insights, adaptive strategies, and customer-centric operations. By embracing the principles and practices outlined here, manufacturers can navigate the complex landscape of supply and demand with confidence, transforming challenges into opportunities and paving the way for a future where precision and efficiency thrive.
How can Deskera Help You?
Deskera ERP and MRP systems help you to keep your business units organized. The system's key features of demand forecasting with Deskera include as follows:
- Establish Demand Forecasting Process
- Automate Data Collection
- Monitor Market Trends
- Analyze Historical Data
- Estimate Future Demand
- Adjust Production Levels
- Manage Supply Chain and much more!
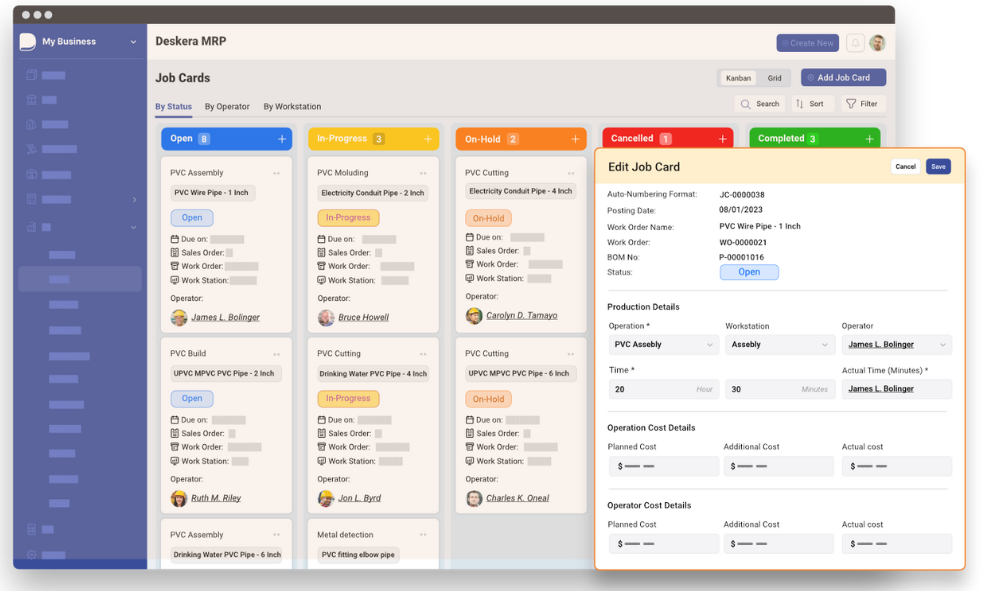
Deskera's integrated financial planning tools enable investors to better plan and track their investments. It can assist investors in making faster and more accurate decisions.
Deskera CRM is a powerful solution that manages your sales and helps you close deals quickly. It not only enables you to perform critical tasks like lead generation via email, but it also gives you a comprehensive view of your sales funnel.
Deskera Books allows you to better manage your accounts and finances. Maintain good accounting practices by automating tasks like billing, invoicing, and payment processing.
Deskera People is a straightforward tool for centralizing your human resource management functions.
Key Takeaways
- Strategic Imperative: Demand forecasting is a strategic imperative in manufacturing, guiding decisions across the supply chain by predicting future customer demand.
- Holistic View: Accurate demand forecasting requires a holistic view, considering historical data, market trends, and external factors that influence demand.
- Qualitative and Quantitative: Demand forecasting methods encompass qualitative approaches based on expert judgment and quantitative methods relying on historical data and statistical models.
- Data Quality Matters: The accuracy of forecasts hinges on the quality and relevance of historical data, requiring consistent data collection and validation processes.
- Collaboration is Key: Collaboration between departments fosters accurate forecasting, as cross-functional insights and knowledge refine predictions and align with business goals.
- Model Diversity: Selecting the right forecasting model is crucial, with time-series models capturing historical trends and machine learning models uncovering complex patterns.
- Real-time Insights: IoT and Big Data offer real-time insights, enhancing demand forecasts by capturing granular data from smart sensors and integrating historical and real-time information.
- Predictive Analytics: Predictive analytics extends beyond forecasting demand to optimizing the entire supply chain, mitigating disruptions and enhancing operational efficiency.
- Continuous Improvement: Demand forecasting is an ongoing process requiring continuous monitoring, model refinement, and adjustments based on forecast accuracy analysis.
- Future-ready Strategies: Embracing AI, machine learning, and advanced analytics tools prepares manufacturers for future trends, enabling adaptive responses to market changes and optimization of production processes.
Related Articles
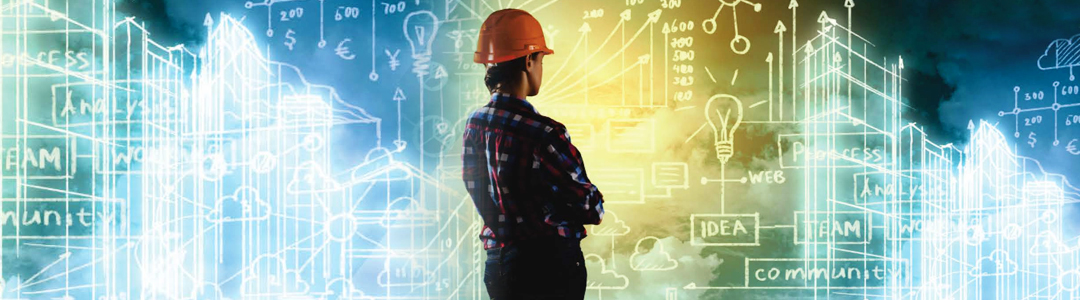
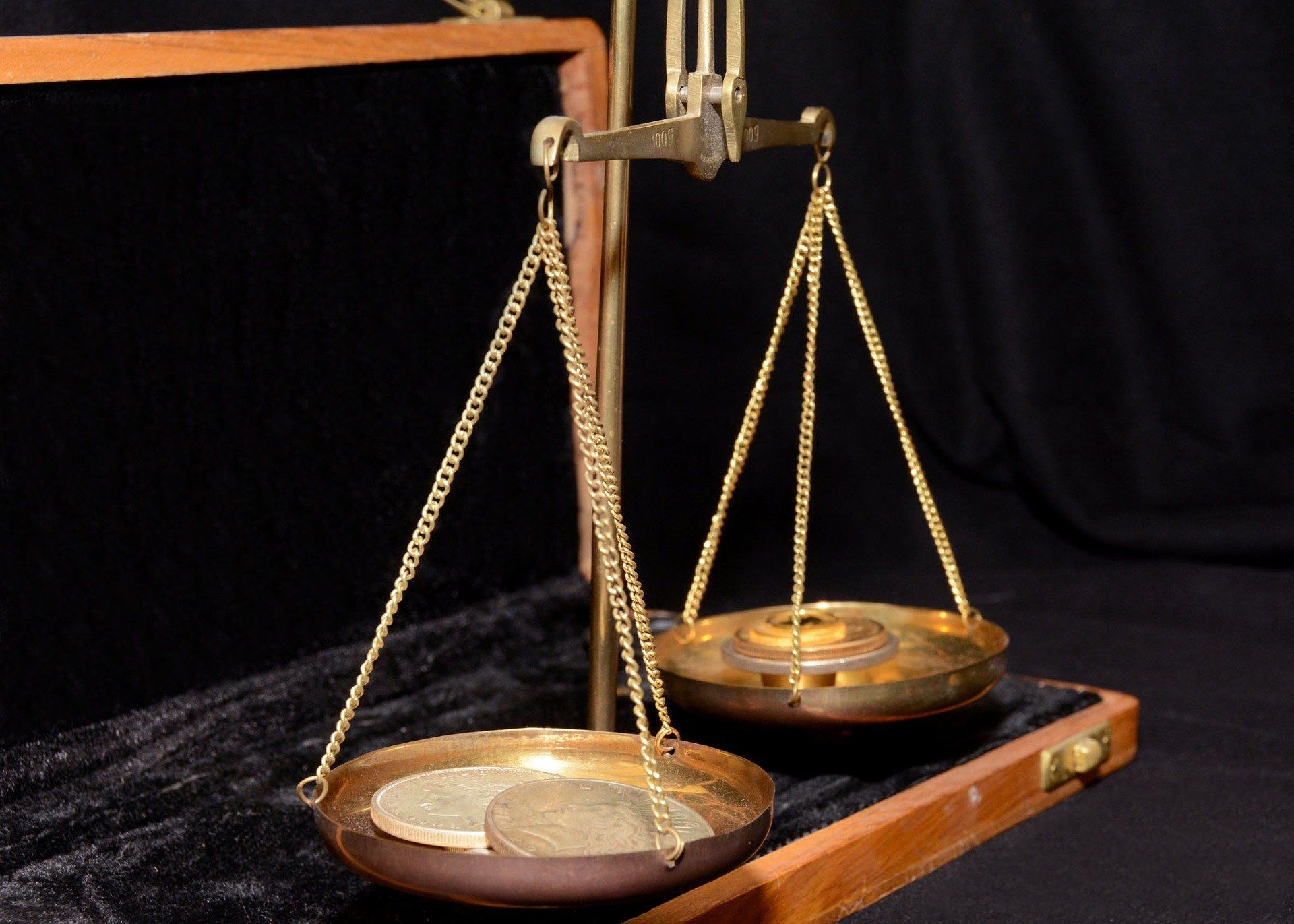
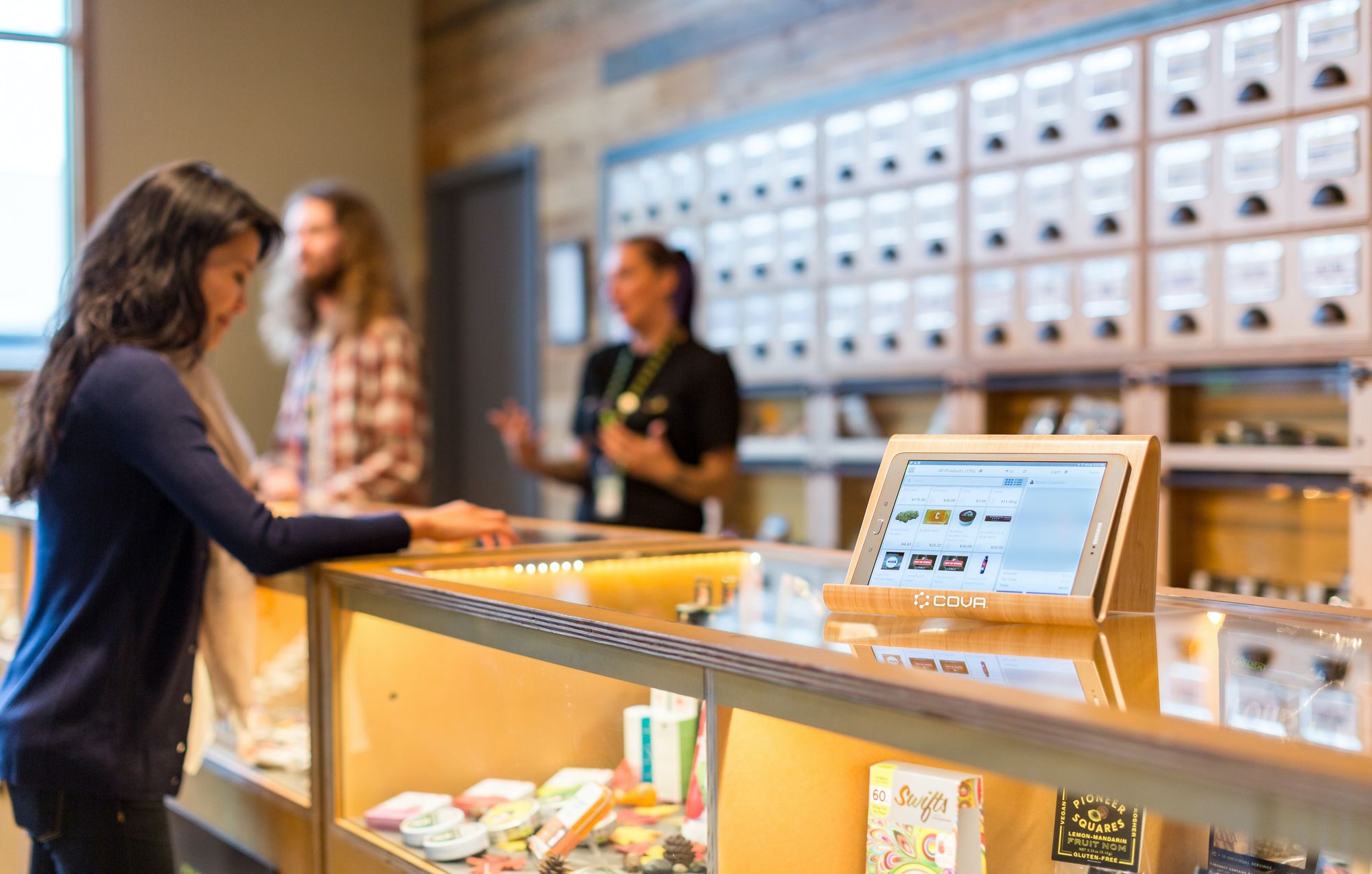
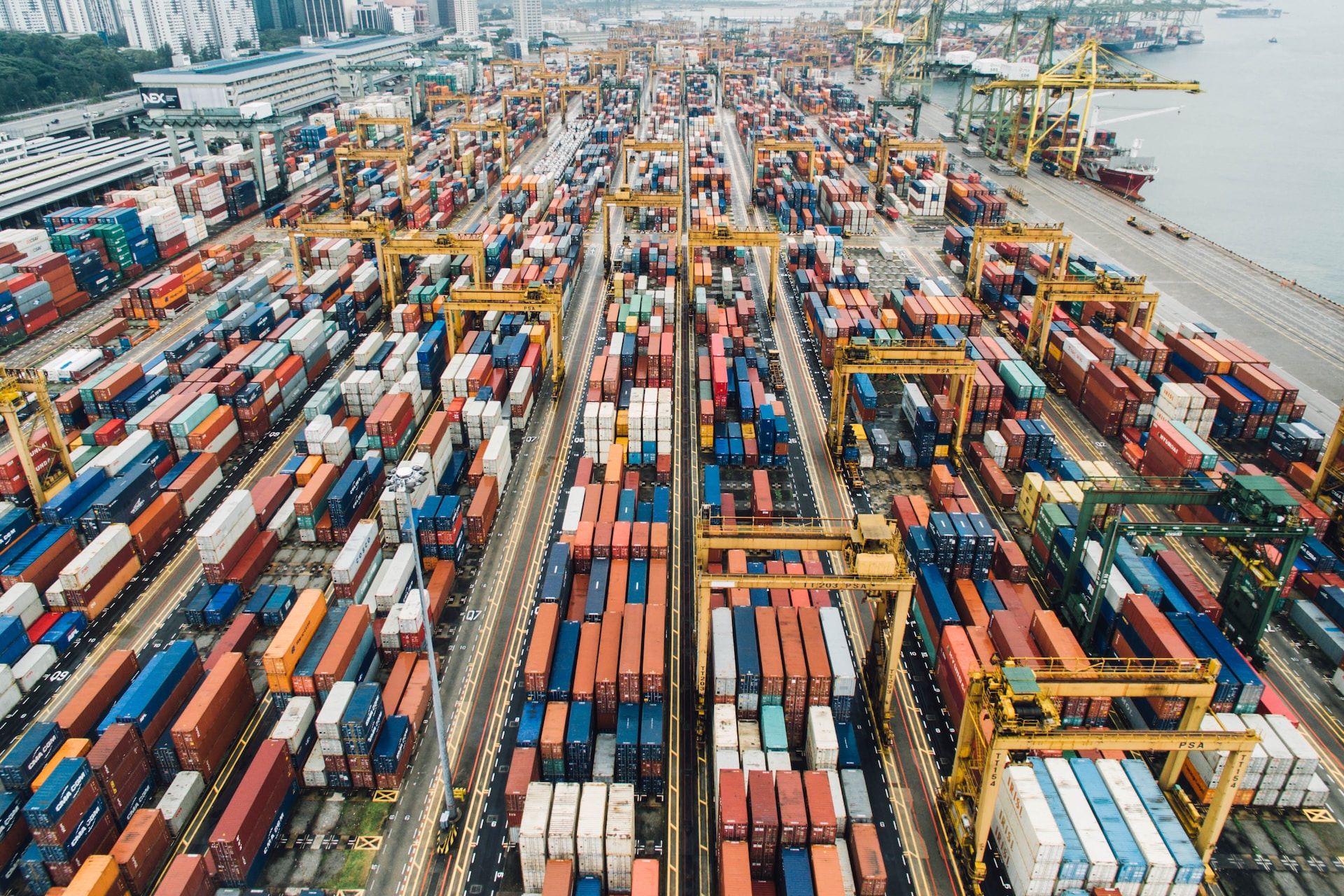
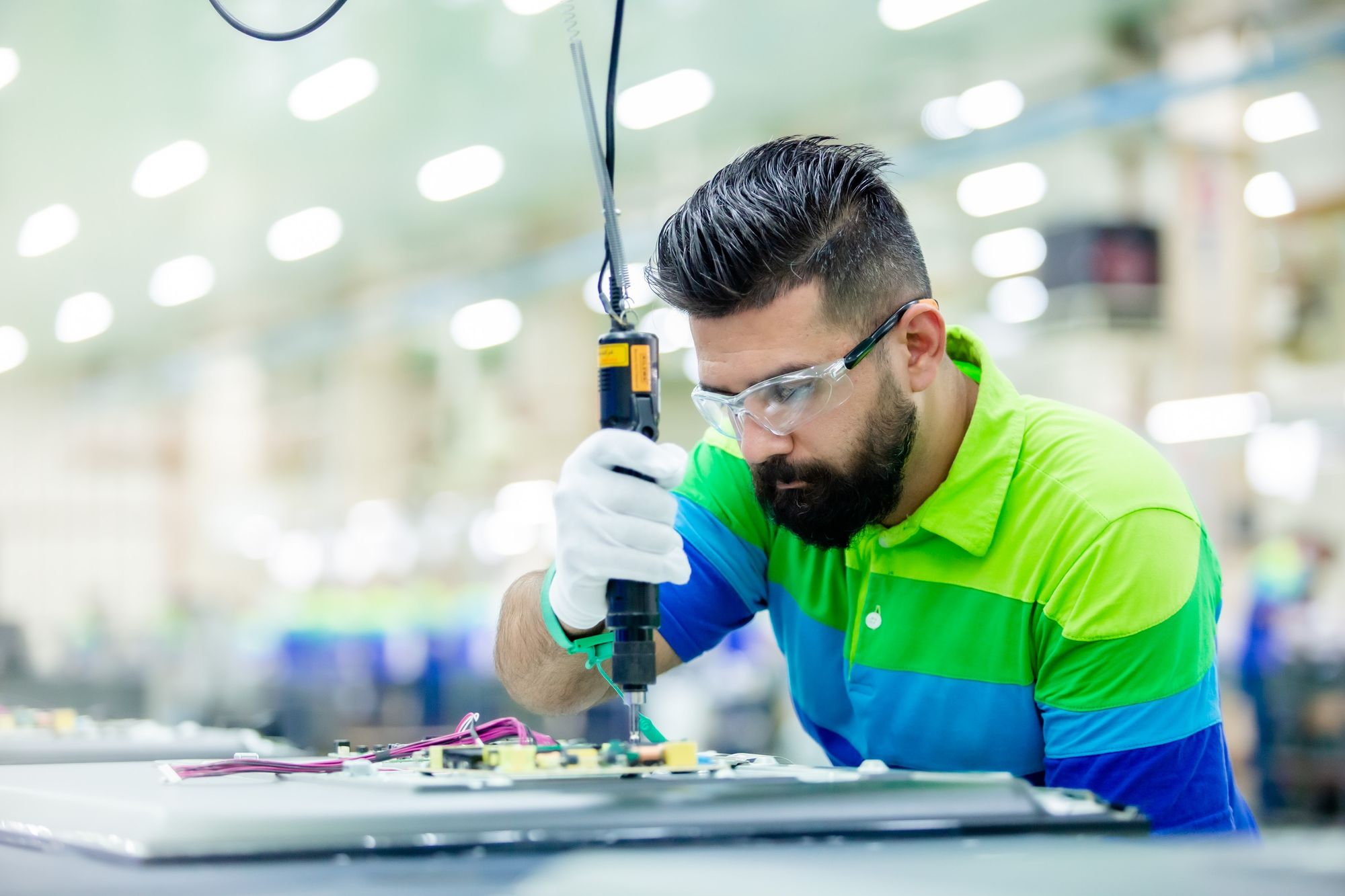