In this era of rapid technological advancements, leveraging big data has emerged as a game-changer for businesses seeking to optimize their inventory control decision-making processes.
According to a study by IDC, the global datasphere is projected to reach a staggering 175 zettabytes by 2025, highlighting the immense volume of data available for analysis.
Big data, characterized by its high volume, velocity, and variety, holds tremendous potential in revolutionizing inventory control practices. By harnessing this vast amount of data, businesses can gain valuable insights into customer behavior, market trends, and supply chain dynamics.
Furthermore, a survey conducted by Deloitte revealed that 64% of companies that implemented big data analytics for inventory management experienced a significant improvement in forecasting accuracy and demand planning. These statistics underscore the transformative power of big data analytics in optimizing inventory control, helping businesses make data-driven decisions to reduce costs, minimize stockouts, and enhance customer satisfaction.
In this article, we will delve into the concept of leveraging big data for enhanced inventory control decision-making. We will explore the challenges faced in traditional methods, examine the potential benefits of big data analytics, and provide insights into best practices for effective implementation.
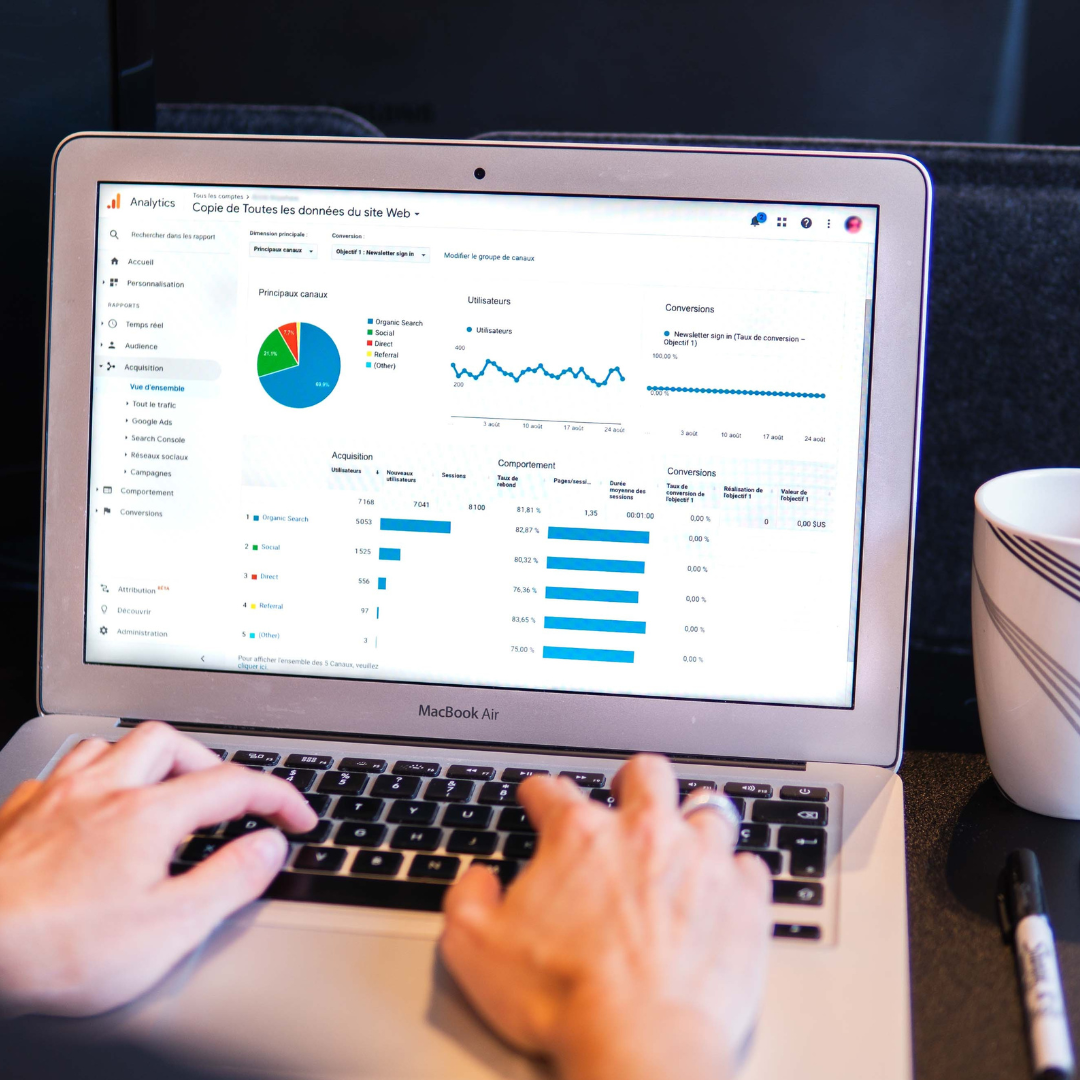
The following list presents the points that are covered in this article:
- Significance of Inventory Control in Businesses
- Introduction to the Concept of Big Data and its Growing Importance in Decision-making Processes
- Understanding Inventory Control and its Challenges
- Exploring Big Data in Inventory Control
- Leveraging Big Data Analytics for Inventory Control
- Overcoming Challenges in Implementing Big Data for Inventory Control
- Best Practices for Effective Implementation
- Why Should Businesses Embrace Big Data Analytics in their Inventory Management Strategies
- Conclusion
- How can Deskera Help You?
- Key Takeaways
- Related Articles
Significance of Inventory Control in Businesses
Inventory control plays a vital role in the success and profitability of businesses across various industries. It involves the management and optimization of a company's inventory levels to meet customer demand while minimizing costs and maximizing operational efficiency.
The significance of inventory control can be understood through the following key points:
- Customer Satisfaction: Maintaining optimal inventory levels ensures that businesses can fulfill customer orders promptly. Timely delivery improves customer loyalty and satisfaction, builds trust, and enhances the overall customer experience.
- Cash Flow Management: Effective inventory control helps businesses strike a balance between stocking enough inventory to meet demand and avoiding excessive inventory holding costs. By managing inventory levels efficiently, companies can optimize their cash flow, reduce carrying costs, and allocate resources more effectively.
- Cost Reduction: Inventory control enables businesses to minimize costs associated with excess inventory, such as storage, obsolescence, and carrying costs. It also helps in reducing the risk of stockouts and emergency replenishments, which can result in additional expenses and lost sales opportunities.
- Demand Planning and Forecasting: Accurate inventory control facilitates better demand planning and forecasting. By analyzing historical data and market trends, businesses can make informed decisions about inventory replenishment, production schedules, and new product introductions.
- Supply Chain Optimization: Inventory control is closely tied to supply chain management. It allows businesses to optimize their supply chain by ensuring timely and efficient movement of goods, minimizing lead times, and enhancing collaboration with suppliers.
- Competitive Advantage: Effective inventory control can provide a competitive edge to businesses. It enables them to respond quickly to market fluctuations, adapt to changing customer demands, and maintain an optimal product mix, thereby outperforming competitors.
In summary, inventory control is crucial for businesses to meet customer demands, manage costs, optimize cash flow, and gain a competitive advantage. By implementing robust inventory control strategies and leveraging innovative technologies like big data analytics, companies can achieve improved operational efficiency, higher customer satisfaction, and increased profitability.
Introduction to the Concept of Big Data and its Growing Importance in Decision-making Processes
In today's digital age, the concept of big data has emerged as a powerful tool for businesses seeking to gain valuable insights and make informed decisions. Big data refers to large and complex datasets that are generated at an unprecedented volume, velocity, and variety. These datasets contain a wealth of information, including customer preferences, market trends, social media interactions, sensor data, and more.
The growing importance of big data in decision-making processes stems from its transformative potential. Traditional decision-making relied heavily on limited data samples and intuition. However, with the advent of big data, businesses can now leverage advanced analytics and machine learning algorithms to extract meaningful patterns, correlations, and trends from vast amounts of information.
By harnessing big data, organizations can gain a deeper understanding of customer behavior, identify emerging market trends, optimize operations, and enhance overall performance. It allows businesses to make data-driven decisions based on real-time insights rather than relying on guesswork or outdated information.
The impact of big data in decision-making is reflected in various industries. For instance, in retail, big data analytics can help identify customer preferences, personalize marketing campaigns, and optimize inventory levels. In healthcare, it can aid in disease prediction, drug discovery, and patient care optimization. In finance, big data analysis can identify fraudulent activities, improve risk management, and enhance investment strategies.
The importance of big data in decision-making is further emphasized by statistics. According to IBM, approximately 2.5 quintillion bytes of data are generated every day, and 90% of the world's data has been generated in the past two years alone. These figures highlight the immense potential for businesses to tap into this vast data pool and uncover valuable insights that can drive strategic decision-making.
In other words, big data has become an integral part of decision-making processes due to its ability to provide organizations with a wealth of valuable information. By leveraging advanced analytics and techniques, businesses can unlock the power of big data to gain a competitive edge, enhance operational efficiency, and drive innovation across various sectors.
Leveraging big data for enhancing inventory control decision-making
Inventory control decision-making plays a crucial role in optimizing supply chain operations and meeting customer demands. Traditional inventory control methods often struggle to adapt to the dynamic nature of today's markets. However, by leveraging big data, businesses can revolutionize their inventory control decision-making processes and gain a competitive advantage.
One of the primary benefits of leveraging big data in inventory control is improved forecasting accuracy. Big data analytics enable businesses to analyze large volumes of historical sales data, market trends, and other relevant factors to generate more precise demand forecasts. By understanding customer preferences, seasonal patterns, and market fluctuations, businesses can make informed decisions regarding inventory levels, production schedules, and replenishment strategies.
Furthermore, big data analytics empowers businesses to optimize their inventory management by identifying potential risks and opportunities. Real-time data feeds, such as point-of-sale data, social media sentiment analysis, and supplier performance metrics, can be integrated with inventory control systems to monitor demand fluctuations.
These could also be utilized to identify supply chain bottlenecks, and proactively respond to market changes. This ensures that businesses can maintain optimal stock levels, reduce stockouts, and minimize excess inventory.
Additionally, big data analytics facilitates enhanced demand planning. By analyzing customer behavior, browsing patterns, and purchase histories, businesses can segment their customer base and tailor their inventory strategies accordingly. This enables businesses to allocate resources effectively, improve product availability, and deliver personalized customer experiences.
Moreover, big data analytics supports supply chain optimization by providing insights into supplier performance, transportation efficiencies, and overall operational effectiveness. By leveraging this information, businesses can streamline their supply chain processes, identify cost-saving opportunities, and improve collaboration with suppliers and logistics partners.
The benefits of leveraging big data for enhanced inventory control decision-making are evident across industries. Retailers can optimize stock levels, reduce out-of-stock situations, and improve customer satisfaction. Manufacturers can optimize production schedules, minimize lead times, and enhance supply chain visibility. Service-based industries can streamline resource allocation, improve scheduling accuracy, and meet customer demands efficiently.
Thus, leveraging big data for enhanced inventory control decision-making offers businesses a wealth of opportunities to optimize their supply chain operations, reduce costs, and deliver exceptional customer experiences.
Understanding Inventory Control and its Challenges
In order to fully appreciate the potential impact of leveraging big data for enhanced inventory control decision-making, it is essential to first understand the fundamentals of inventory control and the challenges associated with traditional methods.
Effective inventory control is crucial for businesses to optimize their supply chain, meet customer demands, and maximize profitability. However, traditional inventory control methods often struggle to keep pace with the complexities of modern markets.
From inventory carrying costs to stockouts and demand forecasting accuracy, businesses face a range of challenges in maintaining optimal inventory levels. By delving into the intricacies of inventory control and examining its inherent challenges, we can better grasp the transformative potential of leveraging big data analytics as a solution to these limitations.
Inventory control and its role in business operations
Inventory control plays a crucial role in the smooth functioning of business operations. It involves managing and overseeing the inventory levels of a company's products or materials to ensure optimal availability while minimizing costs and maximizing efficiency. The role of inventory control can be understood through the following key aspects:
Meeting Customer Demand: Inventory control ensures that businesses have the right amount of inventory to fulfill customer orders in a timely manner. By maintaining appropriate stock levels, businesses can meet customer demands promptly, prevent stockouts, and avoid lost sales opportunities.
Cash Flow Management: Efficient inventory control allows businesses to strike a balance between stocking enough inventory to meet customer demand and minimizing the costs associated with excess inventory. By optimizing inventory levels, businesses can free up valuable working capital and improve cash flow management.
Cost Optimization: Inventory control helps businesses optimize costs associated with inventory management. By minimizing carrying costs, such as warehousing, storage, and insurance expenses, businesses can reduce overall operational costs. Additionally, effective inventory control minimizes the risk of stock obsolescence, reducing financial losses due to outdated or unsold inventory.
Supply Chain Efficiency: Inventory control is closely tied to supply chain management. By maintaining optimal inventory levels, businesses can improve supply chain efficiency by reducing lead times, enhancing supplier relationships, and optimizing production and distribution processes. This leads to improved coordination and collaboration among various stakeholders in the supply chain.
Demand Forecasting and Planning: Inventory control involves analyzing historical sales data, market trends, and customer behavior to forecast future demand accurately. This helps businesses plan production schedules, procurement, and inventory replenishment strategies. Accurate demand forecasting reduces the risk of overstocking or stockouts.
Risk Management: Inventory control helps businesses mitigate risks associated with supply chain disruptions, changes in customer demand, or unexpected events. By closely monitoring inventory levels and implementing risk management strategies, businesses can respond effectively to market uncertainties and mitigate the impact on operations.
Challenges faced in traditional inventory control methods
Traditional inventory control methods often encounter several challenges that can hinder their effectiveness in meeting the demands of modern business operations. These challenges include:
- Inaccurate Demand Forecasting: Traditional methods often rely on historical sales data and simple forecasting techniques, which may not capture the complexities of fluctuating customer demands accurately. This can lead to overstocking or stockouts, impacting customer satisfaction and increasing costs.
- Limited Visibility and Tracking: Traditional inventory control methods may lack real-time visibility into inventory levels, making it challenging to monitor stock movement, identify potential bottlenecks, and respond quickly to changes in demand or supply.
- Manual and Time-Consuming Processes: Traditional methods often involve manual tracking, data entry, and order processing, which can be time-consuming and prone to errors. This can result in delays, inaccuracies, and inefficiencies in inventory management.
- Siloed Information and Lack of Integration: Traditional inventory control methods may rely on separate systems or spreadsheets for different aspects of inventory management, leading to fragmented data and a lack of integration. This can hinder accurate decision-making and create challenges in data sharing and collaboration across departments.
- Limited Supplier Collaboration: Traditional methods may lack effective communication and collaboration channels with suppliers, leading to difficulties in managing supplier relationships, coordinating procurement, and optimizing replenishment processes.
- Changing Market Dynamics: Traditional methods may struggle to adapt to rapidly changing market trends, customer preferences, and supply chain disruptions. They may not incorporate real-time market data or external factors that influence inventory management decisions.
- Cost and Inventory Holding Challenges: Traditional methods may result in higher inventory carrying costs due to excess stock, increased obsolescence risks, and holding costs associated with warehousing, insurance, and storage.
- Lack of Scalability: Traditional methods may face limitations in handling large volumes of data or managing complex inventory networks, hindering scalability as businesses grow or expand into new markets.
Need for advanced techniques to optimize inventory management
In today's fast-paced and competitive business landscape, the need for advanced techniques to optimize inventory management is more critical than ever. Traditional inventory management methods often fall short in meeting the challenges posed by dynamic customer demands, market volatility, and supply chain complexities.
Here are the key reasons why advanced techniques are essential to optimize inventory management:
- Improved Demand Forecasting: Advanced techniques, such as predictive analytics and machine learning algorithms, enable businesses to analyze vast amounts of data, including historical sales data, market trends, and external factors, to generate more accurate demand forecasts. This helps in aligning inventory levels with anticipated demand, reducing the risk of stockouts or overstocking.
- Enhanced Supply Chain Visibility: Advanced inventory management techniques provide real-time visibility into the entire supply chain, enabling businesses to monitor inventory levels, track stock movement, and identify potential bottlenecks or inefficiencies. This visibility facilitates proactive decision-making, efficient order fulfillment, and better coordination with suppliers and partners.
- Optimal Inventory Levels: By leveraging advanced techniques, businesses can determine the optimal level of inventory to meet customer demand while minimizing carrying costs. These techniques consider factors like lead times, order frequency, order size, and service-level requirements to strike the right balance between customer satisfaction and cost optimization.
- Agile and Responsive Operations: Advanced techniques enable businesses to respond quickly and effectively to changing market dynamics, customer preferences, and supply chain disruptions. By analyzing real-time data, businesses can make agile decisions regarding inventory replenishment, production scheduling, and allocation of resources, ensuring timely and efficient operations.
- Integration and Automation: Advanced inventory management techniques leverage technology to integrate various aspects of inventory control, such as demand forecasting, order management, and supply chain optimization. This integration eliminates data silos, streamlines processes, and reduces manual effort, resulting in improved accuracy, efficiency, and productivity.
- Optimization of Order Fulfillment: Advanced techniques help businesses optimize order fulfillment processes by considering factors such as order prioritization, inventory availability, shipping costs, and customer service levels. This ensures that orders are fulfilled in the most cost-effective and timely manner, enhancing customer satisfaction and loyalty.
- Continuous Improvement: Advanced techniques facilitate data-driven analysis and measurement of key performance indicators (KPIs) related to inventory management. This allows businesses to identify areas for improvement, monitor the effectiveness of inventory control strategies, and implement continuous improvement initiatives.
Exploring Big Data in Inventory Control
The application of big data in inventory control offers unprecedented opportunities to optimize supply chain operations, improve demand forecasting accuracy, and make data-driven decisions. By exploring the potential of big data in inventory control, businesses can unlock valuable insights hidden within vast and complex datasets, revolutionizing their approach to inventory management.
In this section, we delve into the realm of big data and its implications for inventory control. We will explore the benefits, challenges, and key considerations involved in leveraging big data analytics to enhance inventory control decision-making.
Big Data and its relevance in inventory control
Big data has become increasingly relevant in inventory control due to its ability to provide businesses with valuable insights, improve decision-making, and optimize supply chain operations. Here are key reasons highlighting the relevance of big data in inventory control:
Improved Demand Forecasting: Big data analytics enables businesses to analyze large volumes of data, including historical sales data, customer behavior, market trends, social media interactions, and external factors. By leveraging this data, businesses can generate more accurate demand forecasts, identify patterns and trends, and make informed decisions regarding inventory levels and replenishment strategies.
Real-Time Data Insights: Big data allows businesses to tap into real-time data feeds, such as point-of-sale data, online transactions, and sensor data. This provides up-to-date information on inventory levels, customer demand, and supply chain performance. By leveraging real-time insights, businesses can proactively manage inventory, respond quickly to market changes, and prevent stockouts or excess inventory.
Enhanced Supply Chain Optimization: Big data analytics enables businesses to optimize their supply chain by analyzing data across multiple touchpoints, such as suppliers, production facilities, distribution centers, and transportation. By integrating and analyzing this data, businesses can identify bottlenecks, optimize logistics, streamline processes, and improve overall supply chain efficiency.
Personalized Inventory Management: Big data enables businesses to segment their customer base and customize inventory strategies based on individual preferences and behaviors. By analyzing customer data, businesses can tailor product offerings, optimize stock allocation, and deliver personalized experiences. This helps in reducing excess inventory and increasing customer satisfaction.
Risk Management and Predictive Analytics: Big data analytics can help businesses identify and mitigate supply chain risks. By analyzing historical data and external factors, businesses can detect patterns and anomalies.
In addition to the aforementioned benefits, businesses can leverage Change Data Capture (CDC) technology to efficiently replicate real-time data changes from operational databases to data warehouses or data lakes, facilitating Extract, Load, Transform (ELT) pipelines. This ensures that the inventory control system stays updated with the latest information, enabling businesses to make more accurate and timely decisions based on the most current data insights.
Furthermore, they can better predict potential disruptions and implement proactive risk management strategies. This helps in minimizing the impact of unforeseen events on inventory control and overall business operations.
Cost Optimization: Big data analytics allows businesses to identify cost-saving opportunities within the inventory management process. By analyzing data related to inventory carrying costs, transportation costs, and order fulfillment, businesses can optimize inventory levels, reduce costs associated with excess inventory or stockouts, and improve overall profitability.
Characteristics of big data in the context of inventory management
In the context of inventory management, big data exhibits several key characteristics that distinguish it from traditional data sources. These characteristics include:
Volume: Big data refers to vast volumes of data generated from various sources, including sales transactions, customer interactions, supply chain activities, and sensor data. In inventory management, this volume of data allows businesses to capture a comprehensive view of their inventory levels, demand patterns, and supply chain dynamics.
Velocity: Big data is characterized by its high velocity, which refers to the speed at which data is generated, collected, and processed. Real-time data feeds from sources like point-of-sale systems, online sales platforms, and supply chain sensors provide immediate insights into inventory levels, customer demand, and market trends.
This velocity allows businesses to make agile decisions and respond quickly to changes in demand or supply.
Variety: Big data encompasses diverse data types, including structured, semi-structured, and unstructured data. In the context of inventory management, this variety includes data from sales records, customer feedback, social media interactions, sensor data, and more.
By analyzing this variety of data, businesses gain a comprehensive understanding of inventory performance, customer preferences, and market dynamics.
Veracity: Veracity refers to the accuracy, reliability, and quality of the data. In the context of big data in inventory management, ensuring data veracity is crucial. By using data cleansing techniques, validation processes, and quality assurance measures, businesses can enhance the reliability of the data used for inventory analysis and decision-making.
Value: The ultimate objective of leveraging big data in inventory management is to derive actionable insights and create value for the business. By analyzing large and diverse datasets, businesses can uncover patterns, trends, and correlations that were previously unseen.
These insights enable businesses to optimize inventory levels, improve demand forecasting, reduce costs, enhance customer satisfaction, and drive overall operational efficiency.
Variability: Big data exhibits variability in terms of its sources, formats, and frequency of generation. Inventory management requires handling data from various sources, such as sales systems, supply chain sensors, and customer feedback channels.
Additionally, data formats may differ, including structured databases, unstructured text, images, or audio files. Managing this variability requires flexible data integration and processing approaches.
Complexity: Big data in inventory management is inherently complex due to its volume, velocity, variety, and interdependencies. Analyzing and interpreting large and diverse datasets require advanced analytics techniques, such as machine learning algorithms, data visualization, and predictive modeling.
Extracting meaningful insights from complex data sets allows businesses to make informed decisions and optimize inventory control strategies.
Sources of big data for inventory control, including internal and external data
Big data for inventory control can be sourced from various internal and external data channels. These data sources provide valuable insights to optimize inventory management strategies. Here are some common sources of big data for inventory control:
Internal Data Sources:
- Sales and Transaction Data: Internal sales data, including point-of-sale (POS) systems, e-commerce platforms, and order management systems, provide information on product sales, customer behavior, and order fulfillment.
- Inventory and Stock Data: Internal inventory systems and warehouse management systems (WMS) offer data on inventory levels, stock movements, SKU information, and storage conditions.
- Supply Chain Data: Data from supply chain systems, such as enterprise resource planning (ERP) systems, transportation management systems (TMS), and procurement systems, provide insights into supplier performance, lead times, delivery schedules, and order statuses.
- Customer Data: Customer relationship management (CRM) systems and customer databases hold valuable data on customer preferences, purchase history, feedback, and loyalty programs, which can help in demand forecasting and personalized inventory management.
- Production Data: Data from production systems, manufacturing execution systems (MES), and production planning systems offer insights into production schedules, capacity utilization, work-in-progress inventory, and production lead times.
External Data Sources:
- Market Data: External market data includes industry reports, market research data, competitor analysis, and economic indicators. This data helps in understanding market trends, demand patterns, and competitive dynamics that can impact inventory management decisions.
- Social Media and Online Platforms: Social media platforms, review websites, and online marketplaces generate vast amounts of data related to customer sentiments, product reviews, and purchasing behavior. This data can provide insights into product popularity, customer preferences, and emerging trends.
- Weather and Seasonal Data: Weather data from meteorological sources helps businesses understand how weather conditions impact demand patterns and inventory requirements. Seasonal data provides information on seasonal demand fluctuations and helps in planning inventory levels accordingly.
- Supplier Data: Data shared by suppliers, such as product availability, lead times, and inventory levels, can help in collaborative inventory planning and management.
- Sensor Data: Internet of Things (IoT) devices and sensors embedded in supply chain infrastructure, such as warehouses, vehicles, and production facilities, generate real-time data on inventory levels, temperature, humidity, and transportation conditions.
- Government and Public Data: Publicly available data, such as economic indicators, demographic data, and trade statistics, can provide insights into market conditions, consumer behavior, and industry trends.
Integrating and analyzing data from these internal and external sources allows businesses to gain a holistic view of their inventory, demand patterns, and supply chain dynamics, enabling data-driven decision-making for optimized inventory control.
Leveraging Big Data Analytics for Inventory Control
In the realm of inventory control, the advent of big data analytics has opened up new avenues for businesses to optimize their inventory management strategies. By harnessing the power of advanced analytics techniques and leveraging vast and diverse datasets, businesses can gain valuable insights, improve demand forecasting accuracy, enhance supply chain visibility, and make data-driven decisions.
In this section, we explore the transformative potential of big data analytics in inventory control. We delve into the key methodologies, tools, and techniques used to extract meaningful insights from large datasets. From predictive analytics to machine learning algorithms, this section uncovers how businesses can leverage big data analytics to drive efficiency, reduce costs, and deliver exceptional customer experiences.
Role of big data analytics in inventory control decision-making
Big data analytics plays a crucial role in inventory control decision-making by providing businesses with actionable insights derived from vast and diverse datasets. Here are key ways in which big data analytics enhances inventory control decision-making:
- Accurate Demand Forecasting: Big data analytics leverages historical sales data, market trends, customer behavior, and external factors to generate more accurate demand forecasts. By analyzing large volumes of data, businesses can identify patterns, seasonality, and trends to anticipate future demand, allowing for optimized inventory levels and improved customer satisfaction.
- Real-Time Inventory Visibility: Big data analytics enables real-time monitoring and visibility into inventory levels, stock movement, and order fulfillment. By integrating data from various sources, such as point-of-sale systems, warehouse management systems, and supply chain sensors, businesses can make timely decisions regarding inventory replenishment, allocation, and fulfillment, reducing the risk of stockouts or overstocking.
- Supply Chain Optimization: Big data analytics provides insights into the entire supply chain, allowing businesses to optimize logistics, identify bottlenecks, and enhance overall supply chain efficiency. By analyzing data on suppliers, transportation, and production processes, businesses can make informed decisions regarding sourcing, lead times, and distribution, improving inventory management and reducing costs.
- Inventory Classification and Segmentation: Big data analytics helps in classifying and segmenting inventory based on various parameters such as product characteristics, demand patterns, profitability, and seasonality. This enables businesses to adopt different inventory strategies for different segments, optimizing stock levels, and allocation based on specific requirements and performance metrics.
- Risk Management and Scenario Analysis: Big data analytics facilitates risk management by identifying potential risks and evaluating the impact on inventory control. By analyzing historical data, external factors, and supply chain vulnerabilities, businesses can conduct scenario analysis, predict the effects of disruptions, and implement proactive strategies to mitigate risks.
- Optimization of Replenishment Strategies: Big data analytics aids in optimizing inventory replenishment strategies by considering factors such as lead times, supplier performance, demand variability, and service-level requirements. By analyzing data on supplier reliability, order frequency, and economic order quantities, businesses can establish optimal reorder points, minimize stockouts, and reduce carrying costs.
- Continuous Improvement and Performance Measurement: Big data analytics enables businesses to measure key performance indicators (KPIs) related to inventory control, such as inventory turnover, stock accuracy, and fill rates. By analyzing data on these metrics, businesses can identify areas for improvement, implement performance improvement initiatives, and track the effectiveness of inventory control strategies over time.
Benefits of using big data analytics, such as improved forecasting accuracy, demand planning, and supply chain optimization
The utilization of big data analytics in inventory control offers several benefits, revolutionizing forecasting accuracy, demand planning, and supply chain optimization. Here are the key advantages:
Improved Forecasting Accuracy: Big data analytics leverages vast datasets, including historical sales data, customer behavior, market trends, and external factors, to enhance forecasting accuracy.
By analyzing large volumes of data, businesses can identify patterns, trends, and seasonality, leading to more accurate predictions of future demand. This helps in optimizing inventory levels, reducing stockouts, and minimizing excess inventory.
Enhanced Demand Planning: Big data analytics enables businesses to gain a deeper understanding of customer preferences, purchasing behavior, and market dynamics. By analyzing data from multiple sources, such as sales transactions, social media interactions, and online reviews, businesses can anticipate changes in demand, identify emerging trends, and align their inventory strategies accordingly.
This leads to improved demand planning, better inventory allocation, and increased customer satisfaction.
Optimized Supply Chain Management: Big data analytics provides insights into the entire supply chain, enabling businesses to optimize various aspects of supply chain management. By analyzing data on suppliers, transportation, production, and inventory levels, businesses can identify inefficiencies, streamline processes, and enhance overall supply chain performance. This results in improved inventory control, reduced lead times, and enhanced operational efficiency.
Proactive Inventory Management: Big data analytics allows businesses to move from reactive to proactive inventory management. By leveraging real-time data feeds, such as point-of-sale information and supply chain sensors, businesses can monitor inventory levels, identify trends, and respond quickly to changes in demand.
This proactive approach helps in avoiding stockouts, minimizing excess inventory, and maximizing sales opportunities.
Cost Optimization: Big data analytics helps in optimizing costs associated with inventory control. By analyzing data on inventory carrying costs, transportation costs, and order fulfillment, businesses can identify cost-saving opportunities. This includes optimizing order quantities, reducing excess inventory, minimizing stock obsolescence, and improving inventory turnover. Ultimately, this leads to cost reductions and improved profitability.
Risk Mitigation: Big data analytics enables businesses to mitigate risks associated with inventory control. By analyzing historical data, external factors, and supply chain vulnerabilities, businesses can identify potential risks, such as supply disruptions or demand fluctuations.
This allows businesses to implement proactive risk management strategies, such as alternative sourcing options, safety stock management, or scenario planning, to minimize the impact of unforeseen events on inventory control.
Examples of real-world applications where big data analytics have enhanced inventory control
Big data analytics has found numerous real-world applications that have enhanced inventory control in various industries. Here are a few examples:
Retail Industry: Retailers leverage big data analytics to optimize inventory control and improve customer satisfaction. By analyzing sales data, customer behavior, and market trends, retailers can forecast demand more accurately, reduce stockouts, and avoid excess inventory.
Additionally, they use real-time data from point-of-sale systems and online platforms to monitor inventory levels, optimize replenishment strategies, and personalize offers based on customer preferences.
E-commerce Sector: E-commerce platforms utilize big data analytics to manage their vast inventory and fulfill customer orders efficiently. By analyzing customer browsing patterns, purchase history, and real-time website interactions, e-commerce companies can optimize their product offerings, ensure sufficient stock levels, and provide personalized recommendations.
Furthermore, they leverage data on shipping, logistics, and order tracking to streamline fulfillment processes, reduce delivery times, and minimize inventory holding costs.
Manufacturing Sector: Manufacturers apply big data analytics to enhance inventory control and streamline production processes. By integrating data from various sources such as production systems, supply chain sensors, and customer feedback, manufacturers can gain real-time visibility into inventory levels, monitor equipment performance, and identify bottlenecks.
This enables them to optimize production schedules, minimize stockouts, and improve overall operational efficiency.
Pharmaceutical Industry: Pharmaceutical companies utilize big data analytics to optimize inventory management in drug development, manufacturing, and distribution. By analyzing data on clinical trials, regulatory requirements, and market demand, they can make informed decisions on inventory levels, product launches, and distribution strategies.
Real-time data from supply chain sensors ensures proper storage conditions and minimizes wastage of temperature-sensitive medications.
Automotive Sector: Automotive manufacturers and dealerships employ big data analytics to manage their vast inventories of vehicles and spare parts. By analyzing data on customer preferences, market trends, and vehicle performance, they can optimize inventory levels, forecast demand accurately, and ensure the timely availability of parts for repairs and servicing. Real-time data on vehicle sales and usage patterns also aid in inventory planning and optimizing production schedules.
Food and Beverage Industry: Big data analytics helps food and beverage companies optimize inventory control in areas such as perishable goods management, supply chain efficiency, and demand planning.
By analyzing data on expiry dates, shelf life, and storage conditions, businesses can minimize food wastage, optimize replenishment strategies, and reduce carrying costs. They also utilize external data sources such as weather patterns and seasonal demand trends to adjust inventory levels and meet changing consumer preferences.
These examples demonstrate how big data analytics has significantly enhanced inventory control in various industries, enabling businesses to optimize inventory levels, improve forecasting accuracy, streamline supply chain operations, and deliver better customer experiences.
Overcoming Challenges in Implementing Big Data for Inventory Control
Implementing big data for inventory control comes with its own set of challenges that organizations must address to fully harness its potential. In this section, we explore the common challenges faced when integrating big data analytics into inventory control processes. From data quality and integration issues to the need for skilled personnel and infrastructure, these challenges can hinder the successful implementation of big data solutions.
However, by understanding and overcoming these obstacles, businesses can unlock the transformative benefits of big data analytics and drive effective inventory control decision-making.
This section delves into the key challenges faced in implementing big data for inventory control and provides insights and strategies to overcome them, enabling businesses to leverage the power of data-driven insights for enhanced inventory management.
Challenges and limitations of leveraging big data for inventory control
Leveraging big data for inventory control presents organizations with both challenges and limitations that need to be addressed to ensure successful implementation. Here are some of the key challenges and limitations associated with utilizing big data for inventory control:
Data Quality and Integration: The quality and reliability of data can pose significant challenges. Big data analytics requires clean, accurate, and consistent data from various sources. Ensuring data integrity, data cleansing, and integrating data from disparate systems can be complex and time-consuming.
Scalability and Infrastructure: Managing and processing large volumes of data requires robust infrastructure and scalable systems. Organizations may need to invest in advanced technology, such as high-performance servers, storage, and processing capabilities, to handle the computational requirements of big data analytics.
Skill Set and Expertise: Extracting valuable insights from big data requires skilled personnel with expertise in data analytics, statistical modeling, and programming. Acquiring and retaining talent with these specialized skills can be a challenge, especially considering the high demand for data professionals in today's market.
Privacy and Security Concerns: Handling sensitive data, such as customer information or proprietary business data, raises privacy and security concerns. Organizations must ensure compliance with data protection regulations and implement robust security measures to safeguard data from unauthorized access or breaches.
Cost and Return on Investment (ROI): Implementing big data solutions can involve significant upfront costs, including infrastructure, software, and talent acquisition. It is crucial to carefully assess the expected ROI and align it with the organization's strategic objectives to justify the investment in big data analytics for inventory control.
Complexity and Interpretation: Big data analytics generates complex outputs that require interpretation and actionable insights. The challenge lies in translating the data into meaningful information and making informed decisions based on the analysis. Organizations must develop data visualization and reporting tools to facilitate decision-making for inventory control.
Data Accessibility and Availability: Availability and accessibility of relevant data from external sources can be a limitation. Some data, such as market trends or competitor information, may not be readily available or may come with a cost. Organizations need to identify and establish partnerships or data-sharing agreements to access the necessary external data sources.
Cultural and Organizational Readiness: Embracing a data-driven culture and ensuring organizational readiness for big data implementation is crucial. It may require organizational change management, training, and creating a data-driven mindset throughout the organization to effectively utilize big data for inventory control.
While these challenges and limitations exist, organizations that address them proactively can overcome hurdles and reap the benefits of leveraging big data for inventory control.
With careful planning, strategic investments, and the right expertise, organizations can harness the power of big data analytics to drive informed decision-making, optimize inventory levels, and gain a competitive advantage in today's dynamic business landscape.
Data quality issues and the importance of data governance
Poor data quality can lead to inaccurate insights, flawed decision-making, and compromised inventory management strategies. Here are some common data quality issues and the importance of data governance in addressing them:
- Inaccurate or Incomplete Data: Incomplete or inaccurate data can distort the analysis and lead to flawed conclusions. For example, missing or incorrect product information, sales records, or inventory levels can result in inaccurate demand forecasting or inefficient inventory replenishment decisions. Data governance ensures that data is complete, accurate, and up to date by implementing data validation checks and data quality controls.
- Inconsistent Data: Inconsistencies in data occur when the same attribute is represented differently across different systems or data sources. For instance, inconsistent product codes or unit of measurement can lead to confusion and errors in inventory control processes. Data governance establishes standardization rules and data integration processes to ensure consistency across the data landscape.
- Data Integration Challenges: Integrating data from multiple sources can be complex, particularly when dealing with diverse data formats, systems, and databases. Inconsistent data structures, incompatible data models, or data redundancy can hinder data integration efforts. Data governance provides guidelines and frameworks for data integration, including data mapping, data transformation, and data cleansing techniques, to ensure seamless integration of disparate data sources.
- Data Timeliness: Outdated or delayed data can limit the effectiveness of inventory control decisions. Real-time or near-real-time data is crucial for accurate demand forecasting, timely replenishment, and agile inventory management. Data governance establishes data capture and data update processes to ensure the availability of timely data for inventory control analysis.
- Data Security and Privacy: Data governance is also essential for ensuring data security and protecting sensitive information. Inventory data may include customer details, pricing information, or supplier contracts that need to be safeguarded against unauthorized access or breaches. Implementing data governance practices, including data encryption, access controls, and data privacy policies, helps maintain data security and compliance with regulations.
The importance of data governance cannot be overstated in addressing data quality issues. Data governance encompasses a set of policies, procedures, and guidelines that govern the overall management, quality, and usage of data within an organization. It establishes accountability, ownership, and responsibilities for data management, ensuring data accuracy, consistency, and integrity.
By implementing effective data governance practices, organizations can:
- Define data quality standards and establish data quality controls to ensure accurate and reliable inventory data.
- Establish data governance roles and responsibilities to enforce data quality and accountability.
- Implement data validation and cleansing processes to identify and rectify data quality issues.
- Create data integration strategies and standards to ensure consistent and seamless data integration across systems.
- Enforce data security and privacy measures to protect sensitive inventory-related information.
- Facilitate data collaboration and communication across departments, ensuring a shared understanding of data and its usage.
In conclusion, data quality issues can undermine the value and reliability of big data analytics for inventory control. Implementing robust data governance practices is crucial to address these issues, ensuring accurate, consistent, and timely data that serves as a solid foundation for effective inventory control decision-making.
Need for data integration and advanced analytics capabilities
In the realm of leveraging big data for inventory control, the need for data integration and advanced analytics capabilities is paramount. Here's why:
Comprehensive View of Inventory
Data integration enables organizations to consolidate data from various sources, such as sales transactions, supply chain systems, and customer interactions, into a unified view of their inventory. By integrating data from different systems and departments, businesses can gain a holistic and accurate understanding of their inventory levels, locations, and movement.
Real-Time Insights
Data integration facilitates the availability of real-time or near-real-time data for inventory control. This allows organizations to make informed decisions based on the most up-to-date information, enabling timely inventory replenishment, demand forecasting, and proactive management of stock levels. Real-time insights provide agility and responsiveness in addressing changing customer demands and market dynamics.
Enhanced Data Accuracy and Consistency
Integrating data eliminates inconsistencies and discrepancies that may arise from separate data sources. By aligning data formats, standards, and definitions, organizations can ensure the accuracy and consistency of inventory-related information. This enhances the reliability of analytics and decision-making processes, reducing errors and increasing confidence in inventory control strategies.
Advanced Analytics Capabilities
Data integration lays the foundation for advanced analytics techniques in inventory control. Once data is consolidated and integrated, organizations can apply sophisticated analytical methods, such as machine learning, predictive modeling, and data mining, to extract meaningful insights and patterns. These advanced analytics capabilities help identify trends, forecast demand, optimize inventory levels, and detect anomalies for proactive inventory management.
Granular and Actionable Insights
Integration of diverse data sources allows organizations to analyze inventory data at a granular level, revealing valuable insights that were previously hidden. Advanced analytics techniques can uncover patterns, correlations, and causality in inventory data, enabling organizations to take targeted actions. For example, identifying slow-moving items, detecting seasonality patterns, or optimizing warehouse layout based on historical movement patterns.
Optimization of Inventory Control Strategies
Data integration and advanced analytics empower organizations to optimize their inventory control strategies. By leveraging a comprehensive view of inventory data and applying advanced analytical models, businesses can determine optimal reorder points, safety stock levels, and order quantities. This leads to improved inventory turnover, reduced carrying costs, and enhanced operational efficiency.
Integration with Other Business Processes
Data integration and advanced analytics capabilities enable organizations to align inventory control with other business processes. Integrated data can be used to optimize supply chain management, production planning, and sales forecasting. To support this level of data integration, using a Postgres API call helps ensure real-time data flows smoothly between systems, enabling faster and more cohesive decision-making across departments. This cross-functional integration enhances the overall coordination and efficiency of the organization's operations.
Best Practices for Effective Implementation
Implementing big data for enhanced inventory control requires careful planning, execution, and adherence to best practices. In this section, we delve into the key best practices that organizations should consider to ensure a successful implementation.
From establishing clear goals and data governance frameworks to investing in the right technology and fostering a data-driven culture, these best practices provide a roadmap for organizations to maximize the benefits of leveraging big data for inventory control. By following these practices, businesses can navigate the complexities of implementation, overcome challenges, and unlock the full potential of big data analytics for efficient and optimized inventory management.
This section explores the best practices that organizations should embrace to drive effective implementation and achieve tangible results in their inventory control decision-making processes.
Guidelines for effective implementation of big data in inventory control decision-making
Implementing big data in inventory control decision-making can be a complex process, but with the right guidelines, organizations can navigate the challenges and maximize the benefits. Here are some key guidelines for effectively implementing big data in inventory control decision-making:
- Define Clear Goals and Objectives: Clearly define the goals and objectives you want to achieve through the implementation of big data in inventory control. Whether it's reducing stockouts, optimizing inventory levels, or improving demand forecasting accuracy, having well-defined goals helps align the implementation process and measure success.
- Establish Data Governance Framework: Implement a robust data governance framework to ensure data quality, integrity, and security. Define data standards, data ownership, and data management processes to maintain the accuracy and consistency of inventory data. Establish data governance roles and responsibilities to ensure accountability and adherence to data governance practices.
- Invest in the Right Technology: Select the appropriate technology infrastructure and tools to support big data analytics for inventory control. Consider factors such as scalability, data processing capabilities, data storage, and integration capabilities. Evaluate different software solutions and platforms that align with your organization's needs and objectives.
- Integrate Data from Multiple Sources: Integrate data from various internal and external sources to create a comprehensive view of inventory. This includes data from sales systems, supply chain management systems, point-of-sale systems, and external sources such as market data and customer sentiment analysis. Ensure data integration is seamless, accurate, and up to date.
- Build a Skilled Team: Assemble a skilled team with expertise in data analytics, statistical modeling, programming, and domain knowledge in inventory control. Invest in training and development programs to enhance the team's capabilities in working with big data analytics tools and techniques.
- Leverage Advanced Analytics Techniques: Utilize advanced analytics techniques such as machine learning, predictive modeling, and data mining to derive actionable insights from inventory data. Apply these techniques to optimize demand forecasting, inventory replenishment, and supply chain management.
- Embrace Data Visualization and Reporting: Implement data visualization and reporting tools to present inventory data in a visually appealing and easy-to-understand manner. Dashboards and reports with relevant metrics and key performance indicators (KPIs) help decision-makers quickly grasp insights and make informed inventory control decisions.
- Foster a Data-Driven Culture: Cultivate a data-driven culture within the organization by promoting the use of data in decision-making processes. Encourage cross-functional collaboration and communication, where stakeholders from different departments leverage data insights to drive inventory control strategies.
- Continuously Monitor and Refine: Implementing big data in inventory control is an iterative process. Continuously monitor the performance of inventory control decisions based on big data analytics and make necessary adjustments. Regularly assess the impact and effectiveness of big data-driven strategies and refine them based on changing market dynamics and business requirements.
- Ensure Data Security and Compliance: Implement robust data security measures to protect sensitive inventory-related information. Adhere to data privacy regulations and ensure compliance with industry standards. Regularly conduct security audits and assessments to identify and address any vulnerabilities.
By following these guidelines, organizations can effectively implement big data in inventory control decision-making and unlock the full potential of data-driven insights. This leads to improved inventory management, optimized operations, and ultimately, enhanced business performance.
Importance of data security and privacy in handling large-scale data
In today's digital landscape, where large-scale data is generated and utilized at unprecedented levels, data security and privacy have become paramount concerns. Here's why data security and privacy are of utmost importance when handling large-scale data:
- Protecting Sensitive Information: Large-scale data often contains sensitive and confidential information, such as customer details, financial records, or proprietary business data. Ensuring data security safeguards this information from unauthorized access, breaches, or misuse, preventing financial and reputational damages.
- Compliance with Regulations: Numerous data protection and privacy regulations, such as the General Data Protection Regulation (GDPR) and the California Consumer Privacy Act (CCPA), impose legal obligations on organizations to safeguard personal data. Adhering to these regulations not only helps avoid legal consequences but also fosters trust among customers, partners, and stakeholders.
- Mitigating Data Breach Risks: Large-scale data poses an attractive target for cybercriminals seeking to exploit vulnerabilities and gain unauthorized access. Robust data security measures, including encryption, access controls, and secure data storage, are crucial for mitigating the risk of data breaches and ensuring data remains confidential and protected.
- Preserving Data Integrity: Data integrity is crucial for large-scale data, as any unauthorized modifications or tampering can undermine the accuracy and reliability of insights and decisions. Implementing data security measures helps maintain data integrity, ensuring that the data remains unchanged and trustworthy throughout its lifecycle.
- Maintaining Customer Trust: Data breaches or mishandling of large-scale data can severely impact customer trust and loyalty. Demonstrating a commitment to data security and privacy instills confidence among customers that their personal information is being handled responsibly, fostering stronger customer relationships and brand reputation.
- Safeguarding Competitive Advantage: Large-scale data often contains proprietary business information, trade secrets, or intellectual property. Protecting this data from unauthorized access or theft is crucial to preserving a competitive advantage in the market. Data security measures help safeguard critical business information, ensuring it remains confidential and exclusive to the organization.
- Data Governance and Accountability: Data security and privacy considerations go hand in hand with effective data governance. Implementing data governance frameworks establishes accountability, ownership, and responsibilities for data management, ensuring that proper security measures are in place and data privacy practices are adhered to throughout the organization.
- Ethical Considerations: Responsible handling of large-scale data includes ethical considerations regarding data privacy and the fair and transparent use of data. Respecting individual privacy rights and ensuring data is collected and used with consent and transparency are essential for ethical data practices.
- Business Continuity and Resilience: Data breaches or privacy incidents can disrupt business operations and lead to financial losses. Proactive data security measures, including disaster recovery plans, data backups, and incident response protocols, help ensure business continuity and resilience in the face of unforeseen events.
- Reputation and Brand Protection: Data security incidents can significantly damage an organization's reputation and brand image. Proactively investing in data security and privacy measures demonstrates a commitment to protecting sensitive information, enhancing trust among customers, partners, and stakeholders, and safeguarding the organization's reputation.
Prioritizing data security and privacy is essential for successful data management and leveraging the full potential of large-scale data while mitigating associated risks.
Need for a collaborative and interdisciplinary approach within organizations
In today's complex and data-driven business environment, a collaborative and interdisciplinary approach within organizations is crucial. Here's why such an approach is needed:
- Holistic Problem-Solving: Complex challenges, including those related to inventory control, often require multiple perspectives and expertise. A collaborative and interdisciplinary approach allows diverse teams to come together, bringing their unique skills and knowledge to the table. This promotes holistic problem-solving by considering various aspects of inventory control, such as data analysis, supply chain management, finance, and customer demand.
- Integration of Specialized Knowledge: Different departments and individuals within an organization possess specialized knowledge and expertise in their respective domains. A collaborative approach allows for the integration of this knowledge, enabling cross-pollination of ideas and insights. For example, combining the expertise of data analysts, inventory managers, and sales teams can lead to a more comprehensive understanding of inventory control challenges and innovative solutions.
- Comprehensive Data Analysis: Leveraging big data for inventory control requires expertise in data analysis and interpretation. By fostering collaboration between data analysts, statisticians, and subject matter experts, organizations can ensure comprehensive data analysis. This collaboration helps uncover hidden patterns, correlations, and insights in large-scale data, leading to more accurate and meaningful inventory control decision-making.
- Effective Communication and Knowledge Sharing: Collaboration breaks down silos within an organization and encourages effective communication and knowledge sharing. When individuals from different departments collaborate, they share their expertise, perspectives, and insights. This enhances the collective understanding of inventory control challenges and facilitates the exchange of best practices, ultimately leading to improved decision-making and optimized inventory management.
- Innovation and Creativity: Interdisciplinary collaboration sparks innovation and creativity. By bringing together individuals with diverse backgrounds, organizations can foster a culture of innovation and out-of-the-box thinking. Different perspectives can lead to novel approaches and solutions for inventory control, enabling organizations to stay ahead of the competition and adapt to changing market dynamics.
- Enhanced Problem Ownership and Accountability: Collaborative approaches empower teams to take ownership and accountability for solving inventory control challenges. When individuals from different departments collaborate, they develop a shared responsibility for achieving common goals. This collective ownership fosters a sense of accountability, leading to more proactive and collaborative efforts to improve inventory control processes.
- Flexibility and Adaptability: The business landscape is dynamic, and inventory control needs to adapt to changing market conditions, customer demands, and supply chain disruptions. A collaborative approach allows organizations to quickly respond to these changes by leveraging the expertise of various stakeholders. Collaboration enhances flexibility and adaptability, enabling organizations to make agile decisions and implement effective inventory control strategies.
- Employee Engagement and Satisfaction: Collaboration promotes employee engagement and satisfaction by creating opportunities for individuals to contribute their expertise and ideas. When employees feel valued and involved in decision-making processes, their job satisfaction and motivation increase. This, in turn, leads to higher productivity and a positive work environment.
- Improved Organizational Alignment: Collaborative and interdisciplinary approaches foster better alignment within organizations. When teams from different departments work together, they develop a shared understanding of organizational goals, strategies, and priorities. This alignment ensures that inventory control decisions are in line with the overall business objectives, leading to a more coordinated and effective approach.
- Continuous Learning and Growth: Collaboration encourages continuous learning and growth among employees. By working together, individuals can learn from each other's expertise and experiences, expanding their knowledge and skills. This continuous learning culture strengthens the organization's capacity for innovation and improvement in inventory control practices.
Why should Businesses Embrace Big Data Analytics in their Inventory Management Strategies?
Today, embracing big data analytics in inventory management strategies is no longer an option but a necessity. Here are compelling reasons why businesses should adopt big data analytics in their inventory management strategies:
Enhanced Decision-Making: Big data analytics provides businesses with valuable insights into their inventory data. By analyzing large volumes of data from various sources, businesses can make informed decisions regarding demand forecasting, inventory optimization, and supply chain management.
These insights enable businesses to respond quickly to market changes, reduce stockouts, minimize excess inventory, and improve overall operational efficiency.
Improved Forecasting Accuracy: Big data analytics enables businesses to improve their forecasting accuracy by considering multiple variables and historical data patterns. By leveraging advanced analytics techniques, businesses can identify trends, seasonality, and other demand patterns, allowing for more accurate demand forecasting.
This accuracy helps businesses optimize inventory levels, reduce carrying costs, and meet customer demands more effectively.
Efficient Demand Planning: Big data analytics provides businesses with a comprehensive understanding of customer behavior, preferences, and buying patterns. By analyzing customer data, businesses can segment their customer base, identify demand trends, and tailor their inventory management strategies accordingly.
This personalized approach to demand planning helps businesses optimize their product assortments, pricing strategies, and promotional activities, leading to increased customer satisfaction and loyalty.
Optimal Supply Chain Management: Big data analytics enables businesses to optimize their supply chain management processes. By analyzing data related to supplier performance, lead times, transportation costs, and inventory levels, businesses can identify bottlenecks, streamline operations, and improve overall supply chain efficiency. This optimization results in reduced costs, improved delivery times, and better collaboration with suppliers.
Real-time Monitoring and Insights: Big data analytics allows businesses to monitor their inventory in real-time, providing up-to-date insights into inventory levels, sales trends, and stock replenishment needs. This real-time visibility enables businesses to proactively manage inventory, respond to demand fluctuations, and prevent stockouts or overstocks. It also facilitates timely decision-making, reducing the risk of lost sales or excess holding costs.
Competitive Advantage: Businesses that embrace big data analytics gain a competitive edge in the market. By leveraging data-driven insights, businesses can optimize inventory management, improve customer service levels, and differentiate themselves from competitors. This competitive advantage helps businesses attract new customers, retain existing ones, and ultimately drive growth and profitability.
Scalability and Flexibility: Big data analytics solutions offer scalability, allowing businesses to handle large volumes of data as their operations grow. Whether it's expanding into new markets, introducing new product lines, or managing complex supply chains, big data analytics can scale to meet evolving business needs.
Additionally, big data analytics provides businesses with the flexibility to adapt quickly to changing market dynamics and make data-driven adjustments to their inventory management strategies.
Continuous Improvement: Big data analytics promotes a culture of continuous improvement in inventory management. By analyzing historical data, monitoring key performance indicators (KPIs), and implementing data-driven metrics, businesses can identify areas for improvement and implement targeted changes. This iterative approach enables businesses to continually optimize their inventory management processes and achieve operational excellence.
Conclusion
Big data brings a wealth of benefits to inventory control, including improved forecasting accuracy, demand planning, and supply chain optimization. Real-world applications have demonstrated its effectiveness in various industries, from retail and e-commerce to manufacturing and logistics. By harnessing the power of big data analytics, businesses can make data-driven decisions, enhance operational efficiency, and gain a competitive edge in the market.
However, implementing big data analytics for inventory control comes with its challenges and limitations. Issues related to data quality, governance, and integration need to be addressed to ensure reliable and accurate insights. Additionally, organizations must prioritize data security and privacy to protect sensitive information and maintain customer trust.
To effectively implement big data analytics in inventory control decision-making, organizations should adopt a collaborative and interdisciplinary approach. By fostering cross-functional collaboration, integrating specialized knowledge, and promoting effective communication, businesses can leverage the full potential of big data analytics and drive innovation in inventory management.
By embracing big data analytics in inventory control, businesses can optimize their decision-making processes, enhance operational efficiency, and achieve sustainable growth. The ability to leverage data-driven insights and adapt to evolving market dynamics will be crucial for organizations to stay competitive and meet customer demands in today's data-driven landscape.
With the right strategies, tools, and approaches, organizations can unlock the transformative power of big data in inventory control and pave the way for a more efficient and profitable future.
How can Deskera Help You?
Deskera ERP and MRP systems help you to keep your business units organized. The system's primary functions are as follows:
- Keep track of your raw materials and final items inventories
- Control production schedules and routings
- Keep a bill of materials
- Produce thorough reports
- Make your own dashboards
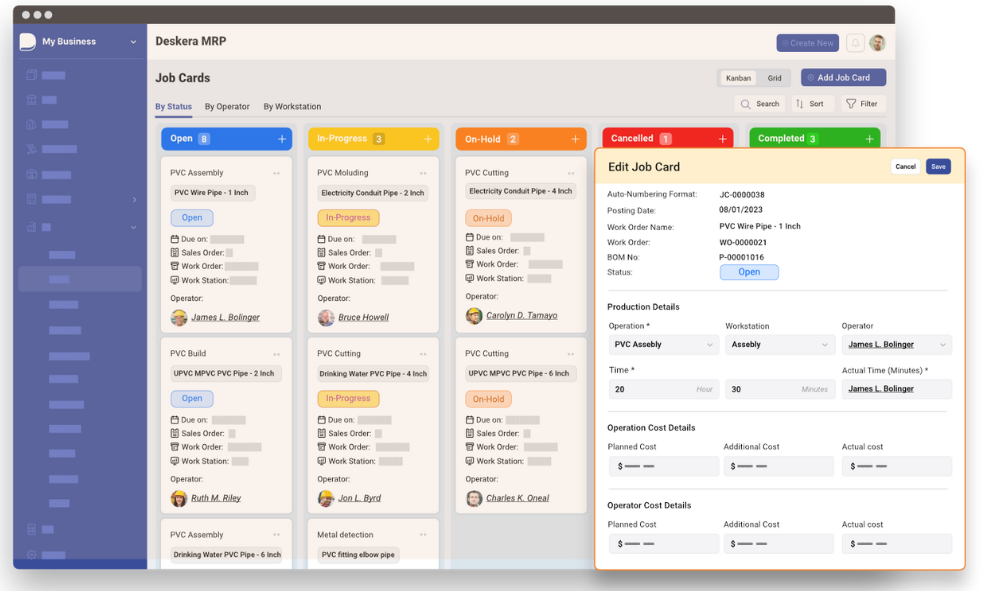
Deskera's integrated financial planning tools enable investors to better plan and track their investments. It can assist investors in making faster and more accurate decisions.
Deskera Books allows you to better manage your accounts and finances. Maintain good accounting practices by automating tasks like billing, invoicing, and payment processing.
Deskera CRM is a powerful solution that manages your sales and helps you close deals quickly. It not only enables you to perform critical tasks like lead generation via email, but it also gives you a comprehensive view of your sales funnel.
Deskera People is a straightforward tool for centralizing your human resource management functions.
Key Takeaways
- Inventory control plays a significant role in businesses by optimizing stock levels, reducing costs, and meeting customer demands.
- Traditional inventory control methods face challenges such as manual data entry, limited data analysis capabilities, and inadequate demand forecasting.
- Advanced techniques, including big data analytics, offer solutions to optimize inventory management and overcome traditional challenges.
- Big data brings several characteristics to inventory management, including large volume, variety, velocity, and veracity of data.
- Internal sources of big data for inventory control include sales records, customer data, and inventory management systems, while external sources include market trends, competitor data, and social media.
- Big data analytics enables businesses to make data-driven decisions, improve forecasting accuracy, and enhance demand planning and supply chain optimization.
- Real-world applications of big data analytics in inventory control have shown improvements in inventory turnover, stockouts reduction, and increased customer satisfaction.
- Implementing big data analytics in inventory control faces challenges such as data quality issues, data governance, and the need for data integration and advanced analytics capabilities.
- Best practices for effective implementation include establishing data governance frameworks, ensuring data security and privacy, and fostering a collaborative and interdisciplinary approach within organizations.
- Data security and privacy are crucial considerations when handling large-scale data, and organizations must prioritize compliance with regulations, protect sensitive information, and build customer trust.
Related Articles
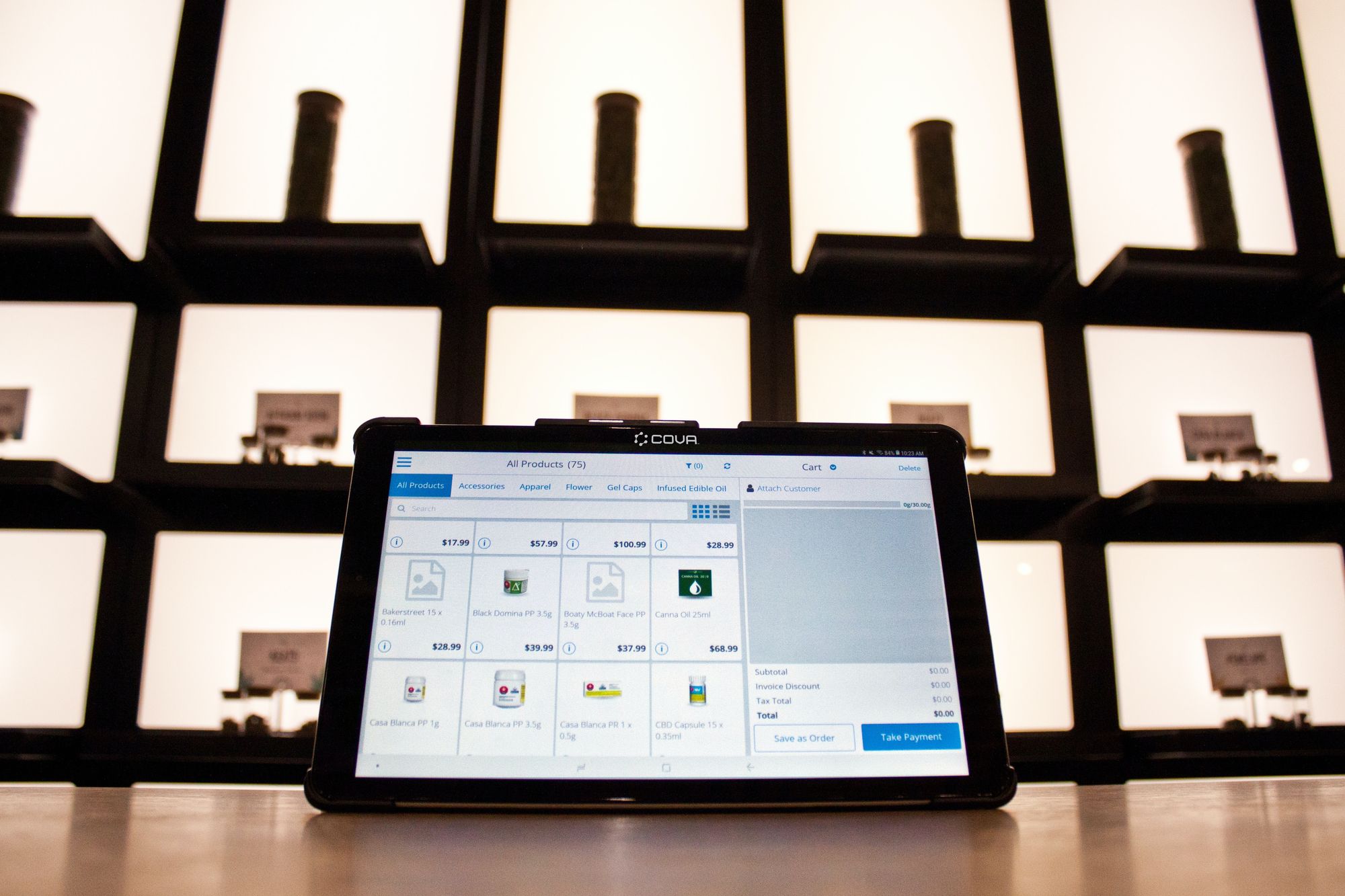
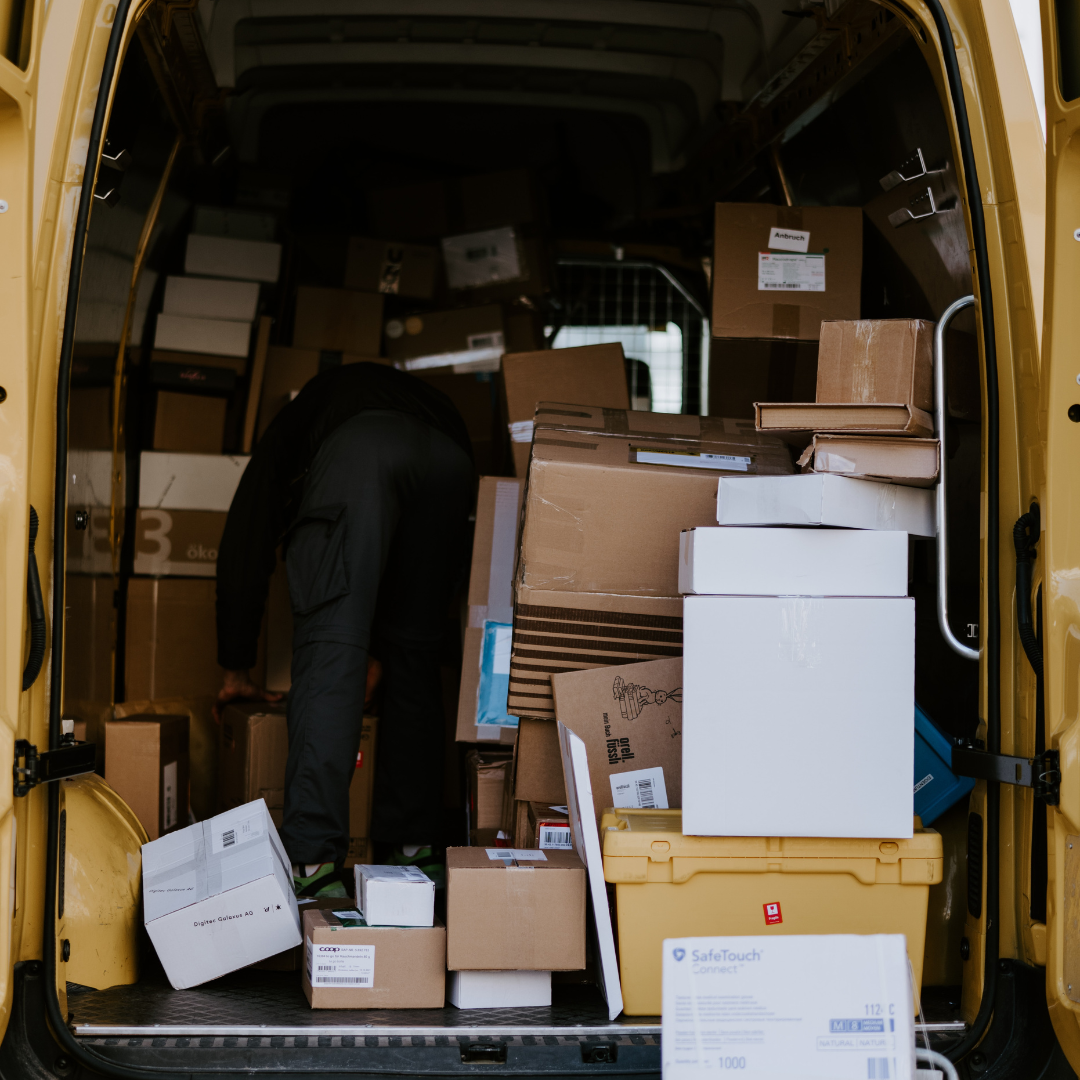
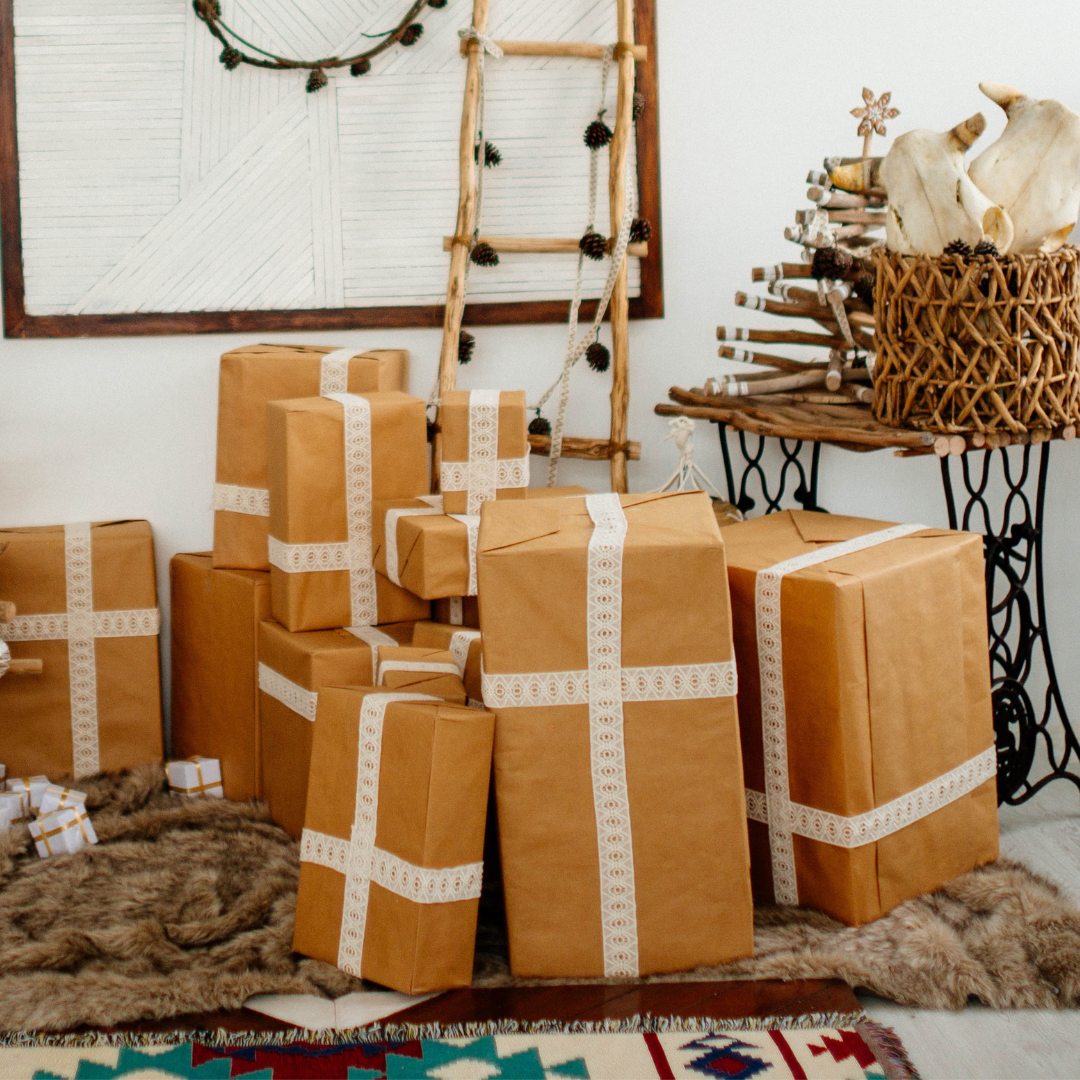
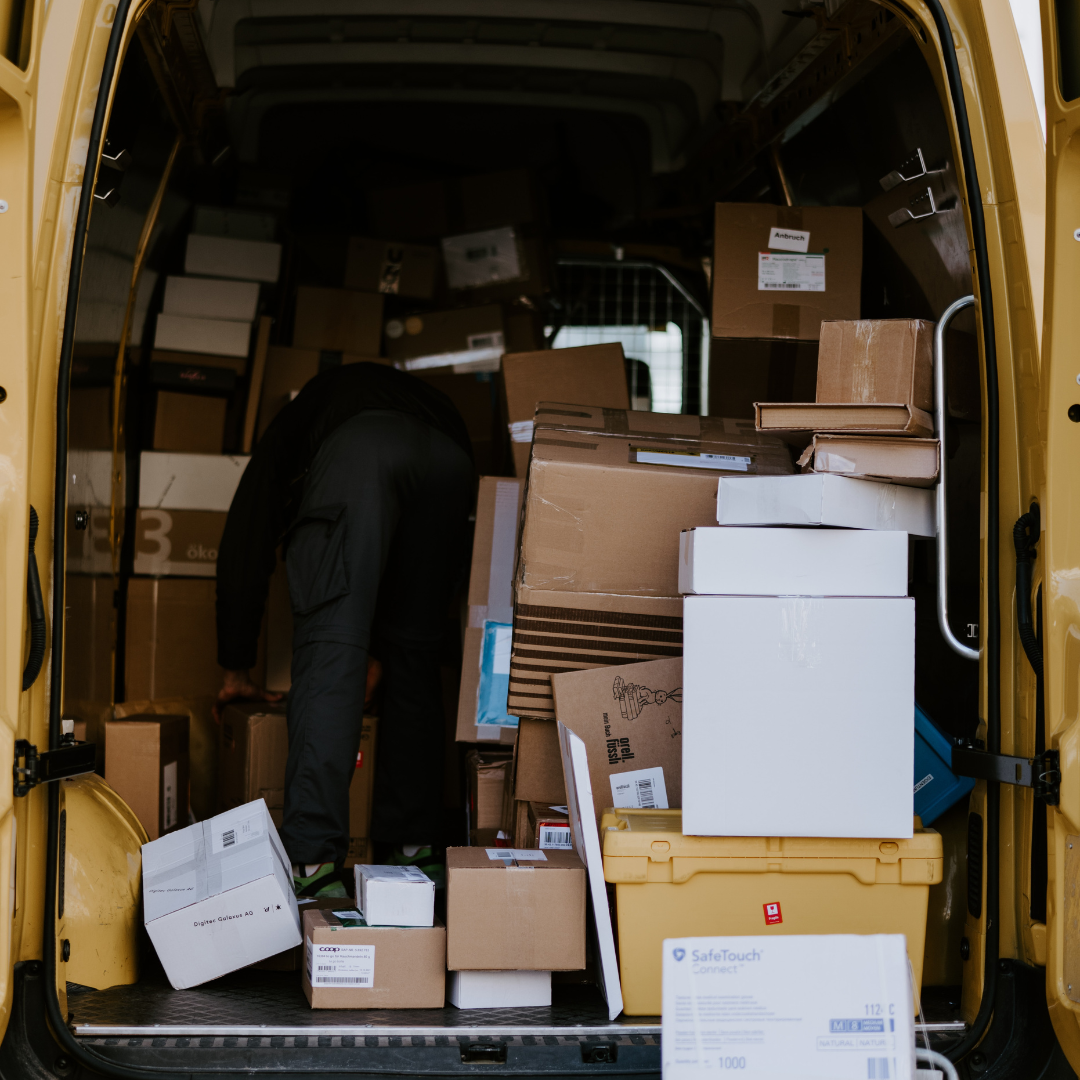