Manufacturing is a complex process that involves many moving parts, from production lines to supply chains to logistics. To optimize these processes and drive business success, manufacturers are turning to manufacturing analytics.
Manufacturing analytics involves the use of data and analytics to gain insights into manufacturing operations, identify areas for improvement, and make informed decisions.
In this article, we will explore the importance of manufacturing analytics and the benefits it can provide. We will also examine the challenges that manufacturers face when implementing manufacturing analytics. Let’s take a look at the table of content below:
- Meaning of Manufacturing Analytics
- Importance of Manufacturing Analytics
- Key Metrics in Manufacturing Analytics
- Important Key Metrics for Manufacturers
- Benefits of Manufacturing Analytics
- Implementing Manufacturing Analytics
- Challenges and Solutions
- Case Studies
- Future of Manufacturing Analytics
- Frequently Asked Questions (FAQs) Associated with Manufacturing Analytics Metrics
- Wrapping Up
- How Deskera Can Assist You?
- Final Takeaways
- Related Articles
Let's get started!
Meaning of Manufacturing Analytics
Manufacturing analytics refers to the use of advanced data analysis techniques, such as statistical analysis, predictive modeling, and machine learning, to extract insights and optimize manufacturing processes.
Furthermore, it involves collecting, analyzing, and visualizing data from various sources, such as sensors, production equipment, and supply chain systems, to improve efficiency, quality, and overall performance.
Moreover, the primary goal of manufacturing analytics is to provide actionable insights to manufacturing companies, enabling them to make data-driven decisions and achieve operational excellence.
Importance of Manufacturing Analytics
Manufacturing analytics is important for several reasons:
Improving Operational Efficiency:
By analyzing production data, manufacturers can identify inefficiencies and bottlenecks in the manufacturing process, allowing them to optimize production, reduce downtime, and minimize waste.
Enhancing Quality Control:
Manufacturing analytics helps to identify trends and patterns in the manufacturing process, allowing manufacturers to detect quality issues and take corrective actions before they become major problems.
Predictive Maintenance:
By analyzing equipment data, manufacturers can predict when equipment is likely to fail and schedule maintenance before it becomes a major problem.
Optimizing Supply Chain:
Manufacturing analytics can help manufacturers to optimize their supply chain, by identifying inefficiencies and bottlenecks in the supply chain, and enabling them to make data-driven decisions that can lead to cost savings and improved delivery times.
Improved Decision Making:
Manufacturing analytics provides real-time insights that enable manufacturers to make data-driven decisions that are based on accurate and timely information.
Overall, manufacturing analytics is essential for manufacturers who want to remain competitive and meet the demands of their customers in a rapidly changing business environment.
Key Metrics in Manufacturing Analytics
Key metrics in manufacturing analytics are specific measurements or indicators used to evaluate the performance of manufacturing processes. These metrics are used to assess the efficiency, effectiveness, and quality of production, and can be used to identify areas for improvement.
After all, the purpose of manufacturing is to rapidly and effectively manufacture items. Producers must constantly monitor their workflow and track a variety of key performance indicators (KPIs) to do so. Manufacturers can monitor a variety of KPIs, but many are more crucial than others.
Important Key Metrics for Manufacturers
Following, we’ve discussed some important key metrics for manufacturers.
Overall Equipment Effectiveness (OEE)
Overall Equipment Effectiveness (OEE) is a key metric used in manufacturing analytics to measure the efficiency and effectiveness of production equipment. It takes into account three factors: Availability, Performance, and Quality, and is expressed as a percentage.
The formula for calculating OEE is as follows:
OEE = Availability x Performance x Quality
Availability: This refers to the percentage of time that equipment is available for production. It takes into account factors such as equipment downtime, scheduled maintenance, and changeover time. The formula for calculating availability is:
Availability = (Operating Time - Downtime) / Operating Time
Performance: This refers to the percentage of the maximum achievable speed at which equipment is operating. It takes into account factors such as speed losses, minor stops, and reduced speed due to product changeovers. The formula for calculating performance is:
Performance = (Ideal Cycle Time x Total Count) / Operating Time
Quality: This refers to the percentage of good parts produced compared to the total number of parts produced. It takes into account factors such as defects, rework, and scrap. The formula for calculating quality is:
Quality = Good Count / Total Count
By multiplying the three factors of availability, performance, and quality, OEE provides a comprehensive measure of equipment efficiency, taking into account all factors that impact productivity.
OEE is used to identify areas for improvement in the production process and can help manufacturers to optimize equipment performance, reduce downtime, and improve quality. By continuously monitoring and improving OEE, manufacturers can improve the overall efficiency and effectiveness of their operations.
Cycle Time
Cycle time is a key metric used in manufacturing analytics to measure the time it takes to complete a production cycle or process. It is defined as the time between the start and end of a process, including any necessary pauses or delays.
Furthermore, cycle time is an important metric because it helps manufacturers to identify bottlenecks, inefficiencies, and areas for improvement in the production process. By analyzing cycle time data, manufacturers can identify where delays are occurring and take steps to eliminate them.
The formula for calculating cycle time is as follows:
Cycle Time = Operating Time / Total Units Produced
Where:
Operating Time: the total time that the equipment is available for production.
Total Units Produced: the total number of units produced during that time.
Cycle time can also be broken down into its components, such as setup time, processing time, and wait time. By analyzing these individual components, manufacturers can identify which parts of the process are taking the most time and take steps to reduce them.
Reducing cycle time can have several benefits, including increasing productivity, reducing lead times, and improving customer satisfaction. Manufacturers can achieve these benefits by streamlining processes, reducing waste, and improving the overall efficiency of the production line. By continuously monitoring and improving cycle time, manufacturers can optimize their operations and remain competitive in the marketplace.
Throughput
Throughput is a key metric used in manufacturing analytics to measure the rate at which a production system or process produces goods. It is defined as the amount of material or product that passes through a production system in a given period of time.
Furthermore, Throughput is an important metric because it indicates how efficiently a production system is operating, and it helps manufacturers to identify bottlenecks, inefficiencies, and areas for improvement in the production process.
Furthermore, by analyzing throughput data, manufacturers can identify where production is slowing down and take steps to eliminate bottlenecks and increase overall productivity.
The formula for calculating throughput is as follows:
Throughput = Total Units Produced / Processing Time
Where:
Total Units Produced: the total number of units produced during the processing time.
Processing Time: the total time that it takes to produce the units, including setup time and run time.
Throughput can be improved by optimizing production processes, reducing downtime, and minimizing waste. By increasing throughput, manufacturers can produce more goods in less time, which can result in cost savings and increased profitability.
However, it is important to note that increasing throughput should not be the sole focus of a manufacturing operation. Quality and customer satisfaction should also be taken into consideration.
Increasing throughput at the expense of quality or customer satisfaction can lead to increased costs, decreased customer loyalty, and a negative impact on the overall reputation of the business. Therefore, it is essential to strike a balance between throughput, quality, and customer satisfaction.
Quality Yield
Quality Yield is a key metric used in manufacturing analytics to measure the percentage of good quality products that are produced compared to the total number of products that are produced. Furthermore, it is a measure of the effectiveness of a production process in producing products that meet the required quality standards.
Quality Yield is an important metric because it indicates the overall quality of the production process and helps manufacturers to identify areas where improvements can be made to improve the quality of the products produced.
The formula for calculating Quality Yield is as follows:
Quality Yield = (Total Units Produced - Defective Units) / Total Units Produced
Where:
Total Units Produced: the total number of units produced during a specific period of time.
Defective Units: the total number of units that do not meet the required quality standards.
By monitoring and analyzing Quality Yield data, manufacturers can identify patterns and trends that indicate where defects are occurring in the production process. This information can then be used to implement corrective actions to reduce defects and improve the overall quality of the products produced.
Moreover, improving quality yield can have several benefits, including reducing scrap, rework, and warranty costs, improving customer satisfaction, and increasing profitability. Manufacturers can achieve these benefits by implementing quality control measures, improving the training and skills of employees, and using data analytics to identify and address quality issues.
It is important to note that Quality Yield should not be the only measure of quality in a manufacturing process. Other measures, such as customer satisfaction and product performance, should also be considered to ensure that the products produced meet the required quality standards and customer expectations.
Defect Rate
Defect Rate is a key metric used in manufacturing analytics to measure the percentage of defective products that are produced compared to the total number of products that are produced. It is a measure of the effectiveness of a production process in producing products that meet the required quality standards.
Furthermore, defect rate is an important metric because it indicates the quality of the production process and helps manufacturers to identify areas where improvements can be made to reduce defects and improve the overall quality of the products produced.
The formula for calculating Defect Rate is as follows:
Defect Rate = (Defective Units / Total Units Produced) x 100%
Where:
Total Units Produced: the total number of units produced during a specific period of time.
Defective Units: the total number of units that do not meet the required quality standards.
By monitoring and analyzing Defect Rate data, manufacturers can identify patterns and trends that indicate where defects are occurring in the production process. This information can then be used to implement corrective actions to reduce defects and improve the overall quality of the products produced.
Reducing Defect Rate can have several benefits, including reducing scrap, rework, and warranty costs, improving customer satisfaction, and increasing profitability. Manufacturers can achieve these benefits by implementing quality control measures, improving the training and skills of employees, and using data analytics to identify and address quality issues.
It is important to note that Defect Rate should not be the only measure of quality in a manufacturing process. Other measures, such as customer satisfaction and product performance, should also be considered to ensure that the products produced meet the required quality standards and customer expectations.
Inventory Turns
Inventory Turns is a key metric used in manufacturing analytics to measure the efficiency of a company's inventory management. It is a measure of how quickly a company can sell and replace its inventory within a specific period of time.
Inventory Turns is an important metric because it indicates how well a company is managing its inventory levels and cash flow. A high Inventory Turns ratio indicates that a company is selling its inventory quickly and efficiently, which can improve cash flow and reduce the risk of inventory obsolescence.
The formula for calculating Inventory Turns is as follows:
Inventory Turns = Cost of Goods Sold / Average Inventory
Where:
Cost of Goods Sold: the total cost of the products sold during a specific period of time.
Average Inventory: the average value of the inventory held during the same period of time.
By monitoring and analyzing Inventory Turns data, manufacturers can identify areas where improvements can be made to optimize inventory levels, reduce inventory holding costs, and improve cash flow.
For example, if Inventory Turns is low, it may indicate that a company is holding too much inventory, which can tie up cash flow and increase the risk of inventory obsolescence. In this case, the company can implement strategies to reduce inventory levels, such as implementing just-in-time (JIT) inventory management or improving supply chain management.
However, it is important to note that a high Inventory Turns ratio does not necessarily indicate good inventory management. A high ratio may be the result of a company running low on inventory, which can lead to stockouts and missed sales opportunities. Therefore, it is important to strike a balance between inventory levels, cash flow, and customer demand.
In summary, Inventory Turns is an important metric that can help manufacturers to optimize inventory levels, reduce inventory holding costs, improve cash flow, and meet customer demand.
Lead Time
Lead Time is a key metric used in manufacturing analytics to measure the amount of time it takes for a product to be manufactured and delivered to the customer. It is a measure of the efficiency and effectiveness of a company's manufacturing and supply chain processes.
Lead Time is an important metric because it impacts customer satisfaction and can also impact a company's ability to compete in the market. A longer Lead Time can result in longer delivery times, which can lead to customer dissatisfaction and lost sales.
The formula for calculating Lead Time is as follows:
Lead Time = Manufacturing Lead Time + Delivery Lead Time
Where:
Manufacturing Lead Time: the amount of time it takes to manufacture the product, from the time the order is received to the time the product is completed and ready for delivery.
Delivery Lead Time: the amount of time it takes to deliver the product to the customer, from the time the product is completed to the time it is delivered to the customer.
By monitoring and analyzing Lead Time data, manufacturers can identify areas where improvements can be made to optimize manufacturing and supply chain processes, reduce Lead Time, and improve customer satisfaction.
For example, if Manufacturing Lead Time is long, a company can implement strategies to improve production efficiency, such as implementing lean manufacturing techniques or improving workforce training. If Delivery Lead Time is long, a company can implement strategies to optimize its supply chain, such as improving transportation logistics or working with suppliers to improve lead times.
In summary, Lead Time is an important metric that can help manufacturers to optimize their manufacturing and supply chain processes, reduce Lead Time, and improve customer satisfaction. By implementing strategies to improve Lead Time, manufacturers can gain a competitive advantage in the market and increase profitability.
Capacity Utilization
Capacity Utilization is a key metric used in manufacturing analytics to measure the extent to which a company is utilizing its production capacity. It is a measure of the efficiency of a company's production process and its ability to meet demand.
Capacity Utilization is an important metric because it indicates how well a company is utilizing its resources and can help identify areas where improvements can be made to increase efficiency and profitability.
The formula for calculating Capacity Utilization is as follows:
Capacity Utilization = (Actual Output / Potential Output) x 100%
Where:
Actual Output: the total output produced during a specific period of time.
Potential Output: the maximum output that could be produced using the company's available resources, such as equipment, labor, and materials.
By monitoring and analyzing Capacity Utilization data, manufacturers can identify areas where improvements can be made to optimize production processes and increase efficiency.
For example, if Capacity Utilization is low, it may indicate that a company is not utilizing its resources effectively, which can lead to increased costs and reduced profitability. In this case, the company can implement strategies to improve production efficiency, such as implementing lean manufacturing techniques, optimizing production schedules, or increasing workforce training.
On the other hand, if Capacity Utilization is high, it may indicate that a company is at risk of overutilizing its resources, which can lead to increased downtime, increased maintenance costs, and reduced product quality. In this case, the company can implement strategies to optimize resource utilization, such as implementing preventive maintenance programs, investing in new equipment, or increasing workforce capacity.
In summary, Capacity Utilization is an important metric that can help manufacturers to optimize their production processes, increase efficiency, and improve profitability. By monitoring and analyzing Capacity Utilization data, manufacturers can identify areas where improvements can be made to optimize resource utilization and meet customer demand.
Downtime
Downtime is a key metric used in manufacturing analytics to measure the amount of time that production equipment is not in operation. It is a measure of the efficiency and effectiveness of a company's manufacturing processes.
Downtime is an important metric because it impacts production capacity, product quality, and ultimately, customer satisfaction. Excessive downtime can lead to lost production time, decreased output, and increased costs, which can impact a company's ability to compete in the market.
There are two main types of downtime: planned and unplanned. Planned downtime is scheduled in advance for maintenance, repairs, or equipment upgrades. Unplanned downtime, on the other hand, is unexpected and can result from equipment failures, breakdowns, or other issues.
The formula for calculating downtime is as follows:
Downtime = Planned Downtime + Unplanned Downtime
By monitoring and analyzing downtime data, manufacturers can identify the causes of downtime and implement strategies to reduce it. For example, if downtime is caused by equipment failures, a company can implement a preventive maintenance program to minimize the risk of equipment breakdowns. If downtime is caused by workforce-related issues, a company can implement training programs to improve employee skills and reduce errors.
Reducing downtime can also help improve product quality and customer satisfaction. By minimizing equipment downtime, a company can ensure that products are manufactured to high quality standards and delivered to customers on time.
In summary, downtime is an important metric that can help manufacturers to optimize their production processes, improve efficiency, and reduce costs. By monitoring and analyzing downtime data, manufacturers can identify the causes of downtime and implement strategies to minimize it, thereby improving product quality and customer satisfaction.
Benefits of Manufacturing Analytics
Manufacturing analytics refers to the use of data and analytics tools to improve manufacturing processes and increase operational efficiency. By leveraging data from various sources within the manufacturing ecosystem, including production lines, equipment, inventory systems, and supply chain partners, manufacturing analytics can provide insights and recommendations that can help manufacturers optimize their operations, reduce costs, and improve product quality.
Some of the key benefits of manufacturing analytics include:
Increased Efficiency and Productivity
Increased efficiency and productivity are two of the key benefits of manufacturing analytics. By leveraging data and analytics tools, manufacturers can identify inefficiencies in their operations, optimize production processes, and reduce waste and downtime, resulting in improved efficiency and productivity.
Manufacturing analytics can help manufacturers in several ways to increase efficiency and productivity:
Identifying bottlenecks: Manufacturing analytics can help manufacturers identify bottlenecks in their production processes, such as slow-moving equipment, inefficient workflows, or staffing issues. By addressing these bottlenecks, manufacturers can reduce delays and improve productivity.
Optimizing production schedules: Manufacturing analytics can help manufacturers optimize production schedules based on real-time data, demand forecasts, and inventory levels, ensuring that production resources are used efficiently.
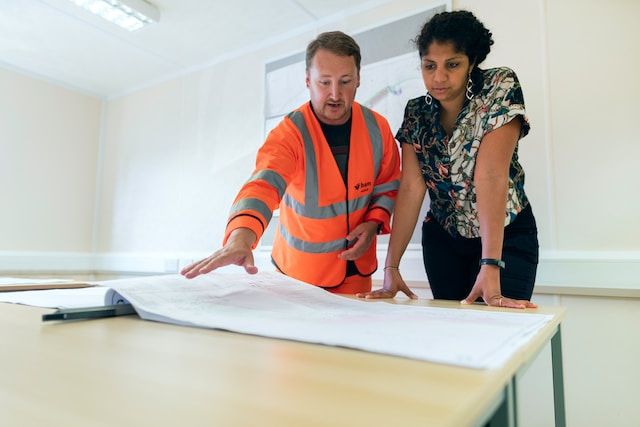
Reducing waste and downtime: Manufacturing analytics can help manufacturers identify areas where waste and downtime are occurring, such as overproduction, excess inventory, or equipment breakdowns. By addressing these issues, manufacturers can reduce waste and downtime, leading to improved efficiency and productivity.
Predictive maintenance: Manufacturing analytics can help manufacturers predict when equipment is likely to fail, allowing them to schedule maintenance before breakdowns occur. This can reduce downtime and improve productivity.
Overall, manufacturing analytics provides manufacturers with the tools and insights they need to optimize their operations and increase efficiency and productivity. By leveraging real-time data and analytics, manufacturers can make informed decisions about their operations, leading to improved performance and profitability.
Improved Quality and Customer Satisfaction
Improved quality and customer satisfaction are two of the most significant benefits that manufacturing analytics can bring to an organization. By analyzing data from production processes and quality control measures, manufacturers can identify areas of improvement and take proactive measures to ensure consistent quality standards, reduce defects in their products, and ultimately increase customer satisfaction.
For example, manufacturers can use analytics to identify the root cause of quality issues and take corrective action to prevent similar issues from happening in the future. By doing so, they can improve their reputation for quality and reliability, which can increase customer loyalty and generate positive word-of-mouth.
Additionally, manufacturing analytics can help manufacturers understand customer needs and preferences better, enabling them to deliver products that meet or exceed customer expectations. By analyzing customer feedback and product usage data, manufacturers can identify features or attributes that customers value most and use that information to inform product design and development decisions.
In summary, improved quality and customer satisfaction are essential benefits of manufacturing analytics that can help organizations increase their competitive advantage, build customer loyalty, and generate positive business outcomes.
Better Decision-Making
Better decision-making is another key benefit of manufacturing analytics. By analyzing data from production processes, supply chains, and customer feedback, manufacturers can gain real-time insights that enable them to make informed decisions quickly and accurately.
For example, manufacturers can use analytics to identify trends in demand and adjust production schedules accordingly, ensuring that they can meet customer needs without overproducing or wasting resources. They can also use analytics to optimize their supply chains by identifying bottlenecks and optimizing inventory levels, which can improve overall efficiency and reduce costs.
Additionally, manufacturers can use analytics to track equipment performance and predict when maintenance is needed, allowing them to schedule maintenance proactively rather than reactively. This can help reduce downtime, extend the lifespan of equipment, and ultimately improve overall efficiency.
By using manufacturing analytics to inform decision-making, manufacturers can make data-driven decisions that are grounded in real-world insights, reducing the risk of costly mistakes and ensuring that they can operate more efficiently and effectively. Overall, better decision-making is a critical benefit of manufacturing analytics that can help organizations improve their bottom line and gain a competitive advantage in the marketplace.
Cost Reduction
Cost reduction is another significant benefit that manufacturing analytics can bring to an organization. By analyzing data from production processes, supply chains, and financial records, manufacturers can identify areas where they can reduce waste, optimize production, and improve efficiency, which can result in cost savings.
For example, manufacturers can use analytics to identify areas of the production process where there is a high level of scrap or waste and take corrective action to reduce those levels. They can also use analytics to optimize production schedules, reduce setup times, and improve overall efficiency, which can result in lower production costs.
Manufacturers can also use analytics to optimize their supply chains by identifying opportunities to reduce lead times, lower inventory levels, and improve logistics. By doing so, they can reduce costs associated with inventory holding, transportation, and other supply chain-related expenses.
Overall, cost reduction is a critical benefit of manufacturing analytics that can help organizations improve their profitability, operate more efficiently, and gain a competitive advantage in the marketplace. By using analytics to identify areas for improvement and take proactive measures to address them, manufacturers can reduce costs and improve their bottom line.
Predictive Maintenance
Predictive maintenance is another important benefit of manufacturing analytics. By analyzing data from sensors and other monitoring devices, manufacturers can predict when maintenance is needed and schedule it proactively, rather than waiting for equipment to break down.
Furthermore, predictive maintenance can help manufacturers reduce downtime, extend the lifespan of equipment, and reduce maintenance costs. By identifying potential equipment failures before they happen, manufacturers can take corrective action before a breakdown occurs, minimizing the impact on production and reducing the need for costly emergency repairs.
In addition, predictive maintenance can help manufacturers improve the overall efficiency of their maintenance operations. By using analytics to prioritize maintenance activities based on the criticality of equipment and the likelihood of failure, manufacturers can optimize maintenance schedules and reduce unnecessary maintenance activities, which can result in cost savings.
Overall, predictive maintenance is a critical benefit of manufacturing analytics that can help manufacturers improve their reliability, reduce downtime, and extend the lifespan of their equipment. By using analytics to predict when maintenance is needed and take proactive measures to address potential issues, manufacturers can operate more efficiently and reduce costs associated with equipment maintenance and repair.
Implementing Manufacturing Analytics
Implementing manufacturing analytics involves collecting and analyzing data from various sources within the manufacturing process, including production processes, supply chains, and financial records. The goal is to gain insights that can be used to improve efficiency, reduce costs, increase quality, and ultimately, improve the bottom line.
Data collection and management
Data collection and management are critical components of implementing manufacturing analytics.
To gain insights that can be used to improve efficiency, reduce costs, and increase quality, manufacturers need to collect and manage data from various sources within the manufacturing process, including production processes, supply chains, and financial records.
Data collection involves the process of capturing and storing data from various sources in a centralized location. This may involve investing in sensors, monitoring devices, and other technology to capture data in real-time. Data can also be collected manually, such as through surveys or other forms of data entry.
Once data is collected, it needs to be managed to ensure it is accurate, consistent, and secure. Data management involves the process of organizing, storing, and retrieving data in a way that is efficient and effective. This may involve investing in data management software or partnering with a third-party provider to manage the data.
Manufacturers also need to ensure that the data they collect is of high quality. This requires implementing data quality controls to ensure that data is accurate, complete, and consistent. Data cleansing and data normalization techniques may be used to ensure data quality.
Data security is another critical component of data management. Manufacturers need to ensure that their data is stored securely and that it is protected from unauthorized access or use. This may involve implementing security protocols, such as encryption and access controls, to safeguard data.
Overall, effective data collection and management are critical to implementing manufacturing analytics. By investing in the right technology and processes, manufacturers can collect and manage data effectively, ensuring that they have the insights they need to improve their operations, reduce costs, and gain a competitive advantage in the marketplace.
Choosing the right analytics tools
Choosing the right analytics tools is critical to the success of implementing manufacturing analytics. The right tools can help organizations extract valuable insights from their data, improve decision-making, and drive better business outcomes. Here are some factors to consider when selecting analytics tools for manufacturing:
Scalability: Manufacturers should select analytics tools that can scale to meet the needs of their operations. As the volume of data grows, the analytics tools should be able to handle the increased load and still deliver insights in a timely manner.
Integration: Analytics tools should be able to integrate with the existing systems and processes that are already in place within the manufacturing organization. This may include ERP systems, SCADA systems, and other data sources.
User interface: The analytics tools should have a user-friendly interface that makes it easy for users to access and interpret the data. This may include data visualization tools that allow users to create custom reports and dashboards.
Flexibility: Analytics tools should be flexible enough to allow manufacturers to customize the analysis to meet their specific needs. This may include the ability to create custom metrics, define data filters, and perform ad-hoc analysis.
Support: Manufacturers should select analytics tools that have strong support and a robust community of users. This ensures that they can get help when they need it and stay up-to-date with the latest features and capabilities.
Cost: Finally, manufacturers should consider the cost of the analytics tools and ensure that they are getting good value for their investment. This may involve comparing the cost of different tools and weighing the features and capabilities against the cost.
Overall, selecting the right analytics tools is critical to the success of implementing manufacturing analytics. By considering factors such as scalability, integration, user interface, flexibility, support, and cost, manufacturers can select tools that deliver valuable insights and drive better business outcomes.
Data visualization and reporting
Data visualization and reporting are important aspects of implementing manufacturing analytics. Furthermore, visualization tools help to transform complex data sets into easy-to-understand visual representations, enabling manufacturers to gain insights and make informed decisions. Here are some key considerations when it comes to data visualization and reporting in manufacturing analytics:
Purpose: Manufacturers should start by determining the purpose of their data visualization and reporting. For example, are they trying to identify production bottlenecks, analyze quality control issues, or optimize their supply chain? Understanding the objective of the analysis will help to determine the appropriate visualization tools and reporting metrics.
Data sources: Manufacturers should identify the relevant data sources for their analysis. This may include data from production machines, quality control systems, and inventory management systems. Data should be collected and stored in a central location for easy access.
Visualization tools: There are various data visualization tools available for manufacturers, including software programs that allow users to create custom dashboards and reports. These tools should be intuitive and user-friendly, allowing manufacturers to easily explore and manipulate the data.
Metrics: Manufacturers should determine the key metrics that will be used in their reporting. These metrics may include machine uptime, cycle time, defect rates, or inventory levels. The metrics should be relevant to the objectives of the analysis and should be easily understandable by stakeholders.
Reporting: Reports should be generated on a regular basis to keep stakeholders informed of the progress and outcomes of the analysis. Reports may include charts, graphs, and other visual aids to help stakeholders understand the data.
Actionable insights: The goal of data visualization and reporting is to provide actionable insights that can be used to improve manufacturing operations. Reports should identify areas for improvement and provide recommendations for how to address them.
Overall, data visualization and reporting are critical components of implementing manufacturing analytics. By selecting the appropriate visualization tools, identifying relevant data sources, determining key metrics, generating reports, and providing actionable insights, manufacturers can use data to drive better business outcomes.
Integration with other systems
Integration with other systems is a crucial component of implementing manufacturing analytics. In many cases, manufacturers already have existing systems in place that store data related to production, supply chain, and other critical functions. Integrating these systems with the manufacturing analytics platform can provide a more comprehensive view of operations and help identify new insights that may have been missed.
Here are some key considerations when it comes to integrating manufacturing analytics with other systems:
Identify data sources: The first step in integration is identifying the relevant data sources that will be used in the manufacturing analytics platform. This may include data from ERP systems, SCADA systems, MES systems, and other sources. It is important to ensure that the data is accurate and up-to-date, and that it is stored in a way that is compatible with the manufacturing analytics platform.
Evaluate integration options: There are various options for integrating different systems with the manufacturing analytics platform, including APIs, data connectors, and middleware. Manufacturers should evaluate these options and select the one that is most appropriate for their needs.
Define data mappings: Data mappings define how the data from different systems will be translated into a common format for use in the manufacturing analytics platform. Manufacturers should work with their IT teams to define these mappings and ensure that they are accurate and complete.
Configure data flows: Once the data mappings have been defined, the next step is to configure the data flows between the different systems. This may involve setting up automated data transfers or real-time data feeds, depending on the needs of the organization.
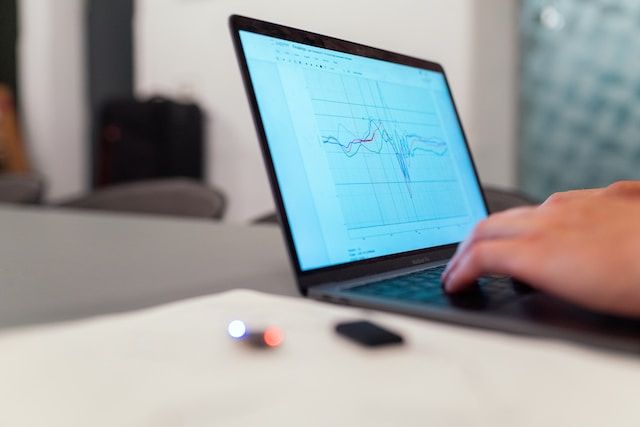
Test integration: Before deploying the integrated system, it is important to thoroughly test the integration to ensure that the data is flowing correctly and that the manufacturing analytics platform is receiving the data in the correct format.
Overall, integrating manufacturing analytics with other systems can provide a more comprehensive view of operations and help identify new insights that may have been missed. By identifying data sources, evaluating integration options, defining data mappings, configuring data flows, and testing integration, manufacturers can create a more effective and efficient data ecosystem.
Challenges and Solutions
Following, we've discussed challenges and solutions in manufacturing analytics. Let's discuss:
Data accuracy and completeness
Data accuracy and completeness are critical challenges in manufacturing analytics because the data collected from various sources can be complex and difficult to ensure accuracy and completeness. Inaccurate or incomplete data can lead to incorrect analysis and ineffective decision-making.
Following, we've discussed some important ways to address these challenges:
Implement data validation and cleansing processes: It is essential to implement data validation and cleansing processes to ensure that data is accurate and complete. Automated data validation tools can be used to minimize errors and ensure data consistency. The tools can identify and correct errors, fill in missing values, and remove duplicate data.
Define data standards: Manufacturers should define data standards and establish procedures for collecting and recording data. It is important to ensure that data is consistent across all systems and platforms.
Train employees: Employees responsible for collecting and recording data should be trained on the importance of data accuracy and completeness. They should also be trained on how to collect, record, and report data accurately.
Use technology: Technology can be used to collect, store, and analyze data accurately and efficiently. Implementing advanced analytics tools like machine learning and artificial intelligence can help in identifying patterns and trends in data.
Monitor data quality: It is important to monitor the quality of data regularly to identify errors or inconsistencies. Manufacturers should establish a system of checks and balances to ensure that data is accurate and complete. This could include conducting regular audits and reviews of data.
By implementing these solutions, manufacturers can ensure that their data is accurate and complete, enabling them to make informed decisions and optimize their operations.
Lack of skilled personnel
The lack of skilled personnel is a significant challenge that manufacturers face when implementing manufacturing analytics initiatives.
Furthermore, skilled personnel are critical to the success of these initiatives as they are responsible for analyzing and interpreting manufacturing data, identifying patterns and trends, and making informed decisions based on the data. Here are some solutions to address the challenge of the lack of skilled personnel:
Invest in training programs: Manufacturers can invest in training programs to develop internal talent. This includes training existing employees on the necessary skills, tools, and techniques required for manufacturing analytics. This approach not only helps to address the skills gap but also fosters employee engagement and retention.
Outsource to third-party providers: Manufacturers can outsource manufacturing analytics to a third-party provider with expertise in the field. Outsourcing can provide access to skilled personnel and resources that may not be available internally.
Collaborate with universities and technical schools: Manufacturers can collaborate with universities and technical schools to create educational programs that produce skilled personnel. This includes sponsoring research projects and internships that help students gain practical experience in manufacturing analytics.
Foster a culture of continuous learning: Manufacturers should foster a culture of continuous learning by providing opportunities for employees to develop their skills and knowledge. This includes investing in professional development programs and creating a learning and development framework that encourages employees to develop new skills.
By implementing these solutions, manufacturers can overcome the challenge of the lack of skilled personnel and ensure that they have the necessary talent to implement and leverage manufacturing analytics to drive business success.
Resistance to change
Resistance to change is a common challenge that manufacturers face when implementing manufacturing analytics initiatives. Resistance can come from employees who are resistant to new technologies and processes, or from management who are resistant to change due to concerns over cost, disruption, or loss of control. Here are some solutions to address the challenge of resistance to change:
Develop a clear communication strategy: Manufacturers should develop a clear communication strategy that helps employees understand the benefits of manufacturing analytics. This includes explaining the value of the initiative, how it will impact their job, and the importance of their participation in the process.
Involve employees in the implementation process: Employees should be involved in the implementation process to gain their buy-in and support. This includes involving them in the design, testing, and implementation of the manufacturing analytics initiative. Their feedback and input should be considered to ensure that the initiative meets their needs and expectations.
Provide training and support: Employees should be provided with the necessary training and support to adopt new technologies and processes. This includes providing training on the use of manufacturing analytics tools and techniques, as well as ongoing support to help employees address any challenges they may encounter.
Address concerns and objections: Manufacturers should address any concerns or objections raised by employees or management. This includes addressing concerns over cost, disruption, or loss of control, and providing solutions that alleviate their concerns.
Celebrate successes: Manufacturers should celebrate successes and milestones achieved during the implementation process. This includes recognizing and rewarding employees for their contributions and achievements, and sharing success stories with the broader organization.
By implementing these solutions, manufacturers can overcome resistance to change and ensure the successful implementation of manufacturing analytics initiatives.
Some Additional Points to Consider Tackling these Challenges
Following, we’ve discussed some additional points that manufacturers can consider to tackle these challenges. Let’s learn them:
Establish a cross-functional team: Manufacturers should establish a cross-functional team that includes representatives from various departments, including IT, operations, and finance. The team should be responsible for developing a comprehensive strategy for implementing manufacturing analytics.
Develop a data management plan: The team should develop a data management plan that outlines how data will be collected, stored, and analyzed. The plan should include procedures for data validation, cleansing, and standardization, as well as a framework for monitoring data quality.
Invest in technology: Manufacturers should invest in advanced analytics tools like machine learning and artificial intelligence that can help analyze large volumes of data and identify patterns and trends. The technology should be user-friendly and accessible to all employees, regardless of their level of technical expertise.
Provide training and support: Employees should be provided with the necessary training and support to adopt new technologies and processes. This includes providing training on the use of manufacturing analytics tools and techniques, as well as ongoing support to help employees address any challenges they may encounter.
Foster a culture of continuous improvement: Manufacturers should foster a culture of continuous improvement by encouraging employees to share their ideas and suggestions for improving operations. This includes creating a feedback mechanism that allows employees to provide feedback and suggestions for improvement.
Celebrate successes: Manufacturers should celebrate successes and milestones achieved during the implementation process. This includes recognizing and rewarding employees for their contributions and achievements, and sharing success stories with the broader organization.
By taking a holistic approach that addresses people, processes, and technology, manufacturers can overcome the challenges of data accuracy and completeness, lack of skilled personnel, and resistance to change. This will enable them to implement manufacturing analytics initiatives that drive business success and improve operational efficiency.
Case Studies
Following, we’ve discussed some examples of successful implementation of manufacturing analytics:
GE Aviation: GE Aviation implemented a manufacturing analytics initiative that uses machine learning algorithms to analyze sensor data from aircraft engines. The initiative has helped the company reduce engine downtime by up to 30%, resulting in significant cost savings.
Intel: Intel implemented a manufacturing analytics initiative that uses predictive analytics to identify potential equipment failures before they occur. The initiative has helped the company reduce downtime and improve the quality of its products, resulting in increased customer satisfaction.
Whirlpool: Whirlpool implemented a manufacturing analytics initiative that uses real-time data to monitor production lines and identify potential bottlenecks. The initiative has helped the company reduce downtime, improve product quality, and increase production efficiency.
BMW: BMW implemented a manufacturing analytics initiative that uses real-time data to monitor production lines and identify potential issues. The initiative has helped the company reduce downtime, improve product quality, and increase production efficiency, resulting in cost savings and increased customer satisfaction.
PepsiCo: PepsiCo implemented a manufacturing analytics initiative that uses predictive analytics to forecast demand for its products. The initiative has helped the company optimize its production schedules, reduce waste, and improve the accuracy of its inventory management, resulting in significant cost savings.
These manufacturing analytics initiatives have resulted in significant benefits for these companies, including reduced downtime, improved product quality, increased production efficiency, and cost savings. By leveraging the power of data and analytics, these companies have been able to gain valuable insights into their operations, identify areas for improvement, and make informed decisions that drive business success.
Future of Manufacturing Analytics
The future of manufacturing analytics is poised for significant growth and advancement, driven by emerging technologies and integration with other areas such as supply chain and logistics. Here are some trends and predictions for the future of manufacturing analytics:
Emerging Technologies: The emergence of new technologies such as the Internet of Things (IoT), Artificial Intelligence (AI), and Machine Learning (ML) will continue to transform the manufacturing industry. These technologies will enable manufacturers to collect and analyze vast amounts of data in real-time, leading to more accurate and timely insights that can be used to improve operations and drive business growth.
Integration with other areas: As manufacturing becomes more integrated with other areas such as supply chain and logistics, the importance of analytics will only increase. Manufacturers will need to be able to analyze data across multiple areas in order to optimize their operations and make informed decisions.
Predictive Maintenance: Predictive maintenance will become more prevalent as manufacturers use machine learning algorithms and real-time data to predict when machines will fail. This will enable them to schedule maintenance activities before a machine fails, reducing downtime and increasing productivity.
Personalized Manufacturing: As consumer demand for customized products grows, manufacturers will need to be able to quickly adapt their production processes. Analytics will play a key role in enabling manufacturers to optimize their production processes and meet the demands of their customers.
Sustainability: With increasing focus on sustainability, manufacturers will need to be able to analyze their operations and supply chains to identify areas for improvement. This will require the use of analytics to track and measure energy usage, waste, and emissions.
Ultimately, the future of manufacturing analytics is bright, driven by emerging technologies, integration with other areas, and a focus on sustainability. Manufacturers who embrace analytics and use data-driven insights to optimize their operations will be well positioned to drive business growth and success in the years ahead.
Frequently Asked Questions (FAQs) Associated with Manufacturing Analytics Metrics
Following, we’ve thoroughly discussed some crucial frequently asked questions (FAQs) associated with manufacturing analytics metrics. Let’s discuss:
Que 1: How can manufacturing analytics metrics help improve operations?
Answer: Manufacturing analytics metrics can help identify areas for improvement and enable manufacturers to make data-driven decisions. By analyzing data and identifying trends, manufacturers can optimize their processes, reduce downtime, improve product quality, and increase productivity.
Que 2: What role do emerging technologies play in manufacturing analytics metrics?
Answer: Emerging technologies such as the Internet of Things (IoT), Artificial Intelligence (AI), and Machine Learning (ML) are driving advancements in manufacturing analytics. By integrating data from sensors and other sources, manufacturers can gain a more comprehensive view of their operations and use AI and ML to identify patterns and predict outcomes.
Que 3: How can manufacturing analytics metrics be integrated with other areas such as supply chain and logistics?
Answer: Manufacturing analytics metrics can be integrated with other areas such as supply chain and logistics by sharing data and insights. By analyzing data across the entire value chain, manufacturers can optimize their operations and improve the efficiency of their supply chain and logistics processes.
Que 4: What are some predictions for the future of manufacturing analytics metrics?
Answer: Predictions for the future of manufacturing analytics metrics include increased adoption of AI and ML, greater integration with other areas such as supply chain and logistics, and the emergence of new metrics that capture the holistic nature of manufacturing operations.
Que 5: How can manufacturers get started with implementing manufacturing analytics metrics?
Answer: Manufacturers can get started with implementing manufacturing analytics metrics by identifying key metrics that align with their business goals and investing in data management systems and employee training. It is also important to create a culture of innovation and continuous improvement to ensure that manufacturing analytics becomes an integral part of the organization's operations.
Que 6: How can manufacturers ensure the accuracy and completeness of their data for manufacturing analytics metrics?
Answer: Manufacturers can ensure the accuracy and completeness of their data by investing in data management systems, implementing data governance policies, and conducting regular data quality checks. They can also use data analytics tools to identify and correct data anomalies and outliers.
Que 7: What benefits can manufacturers expect from successfully implementing manufacturing analytics metrics?
Answer: Manufacturers can expect to see benefits such as increased efficiency, improved product quality, reduced downtime, and cost savings. By gaining insights into their operations and making data-driven decisions, manufacturers can optimize their processes, reduce waste, and improve overall business performance.
Que 8: Are manufacturing analytics metrics only useful for large manufacturers?
Answer: Manufacturing analytics metrics are useful for all manufacturers, regardless of size. Even small and medium-sized manufacturers can benefit from the insights gained by analyzing their operations and making data-driven decisions.
Que 9: How can manufacturers ensure that their employees are equipped to use manufacturing analytics metrics effectively?
Answer: Manufacturers can ensure that their employees are equipped to use manufacturing analytics metrics effectively by providing regular training and support. This can include training on data analysis tools, data visualization, and interpretation of results. It is also important to foster a culture of data-driven decision-making and encourage employees to engage with manufacturing analytics metrics in their day-to-day work.
Que 10: Can manufacturers use manufacturing analytics metrics to track environmental sustainability and social responsibility?
Answer: Yes, manufacturers can use manufacturing analytics metrics to track environmental sustainability and social responsibility. By analyzing metrics such as energy usage, waste production, and emissions, manufacturers can identify areas for improvement and track progress towards sustainability goals. Similarly, by tracking metrics related to labor practices and social responsibility, manufacturers can ensure that they are operating ethically and responsibly.
Que 11: How can manufacturers ensure that they are using the right manufacturing analytics metrics for their specific operations?
Answer: Manufacturers can ensure that they are using the right manufacturing analytics metrics for their specific operations by aligning their metrics with their business goals and objectives. They can also consult with industry experts and benchmark against best practices in their sector. It is important to continuously monitor and adjust metrics as needed to ensure that they remain relevant and provide meaningful insights.
Wrapping Up
In conclusion, manufacturing analytics is a powerful tool that can help manufacturers gain valuable insights into their operations, identify areas for improvement, and make informed decisions that drive business success.
However, there are challenges to implementing manufacturing analytics, including data accuracy and completeness, lack of skilled personnel, and resistance to change.
To address these challenges, manufacturers must invest in data management systems, provide training and support for their employees, and create a culture of innovation and continuous improvement.
The successful implementation of manufacturing analytics has been demonstrated by companies such as GE Aviation, Intel, Whirlpool, BMW, and PepsiCo, who have achieved significant benefits, including reduced downtime, improved product quality, increased production efficiency, and cost savings.
Looking ahead, the future of manufacturing analytics is bright, with emerging technologies such as the Internet of Things (IoT), Artificial Intelligence (AI), and Machine Learning (ML) driving advancements in the field. Manufacturers who embrace analytics and use data-driven insights to optimize their operations will be well positioned to drive business growth and success in the years ahead.
As the manufacturing industry continues to evolve and become more integrated with other areas such as supply chain and logistics, the importance of analytics will only increase. Ultimately, manufacturing analytics will play a critical role in helping manufacturers stay competitive and meet the changing needs of their customers.
How Deskera Can Assist You?
Deskera's integrated financial planning tools allow investors to better plan their investments and track their progress. It can help investors make decisions faster and more accurately.
Deskera Books enables you to manage your accounts and finances more effectively. Maintain sound accounting practices by automating accounting operations such as billing, invoicing, and payment processing.
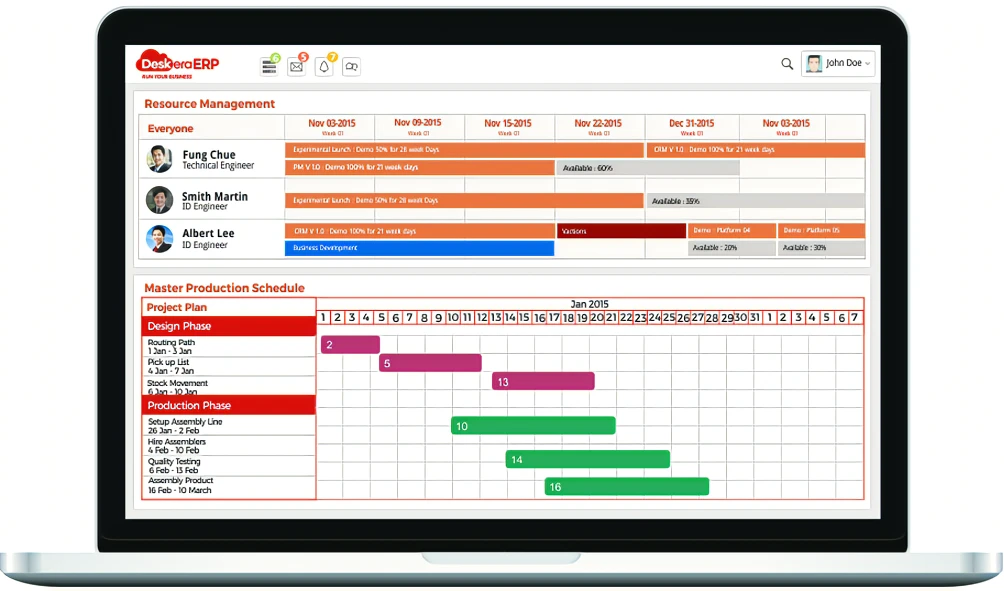
Deskera CRM is a strong solution that manages your sales and assists you in closing agreements quickly. It not only allows you to do critical duties such as lead generation via email, but it also provides you with a comprehensive view of your sales funnel.
Deskera People is a simple tool for taking control of your human resource management functions. The technology not only speeds up payroll processing but also allows you to manage all other activities such as overtime, benefits, bonuses, training programs, and much more. This is your chance to grow your business, increase earnings, and improve the efficiency of the entire production process.
Final Takeaways
We've arrived at the last section of this guide. Let's have a look at some of the most important points to remember:
- Manufacturing analytics refers to the use of advanced data analysis techniques, such as statistical analysis, predictive modeling, and machine learning, to extract insights and optimize manufacturing processes.
- The main goal of manufacturing analytics is to provide actionable insights to manufacturing companies, enabling them to make data-driven decisions and achieve operational excellence.
- Key metrics in manufacturing analytics are specific measurements or indicators used to evaluate the performance of manufacturing processes. These metrics are used to assess the efficiency, effectiveness, and quality of production, and can be used to identify areas for improvement.
- Availability: This refers to the percentage of time that equipment is available for production. It takes into account factors such as equipment downtime, scheduled maintenance, and changeover time. The formula for calculating availability is: Availability = (Operating Time - Downtime) / Operating Time
- Manufacturers can achieve these benefits by streamlining processes, reducing waste, and improving the overall efficiency of the production line. By continuously monitoring and improving cycle time, manufacturers can optimize their operations and remain competitive in the marketplace.
- Throughput is an important metric because it indicates how efficiently a production system is operating, and it helps manufacturers to identify bottlenecks, inefficiencies, and areas for improvement in the production process. By analyzing throughput data, manufacturers can identify where production is slowing down and take steps to eliminate bottlenecks and increase overall productivity.
- Quality Yield is a key metric used in manufacturing analytics to measure the percentage of good quality products that are produced compared to the total number of products that are produced. It is a measure of the effectiveness of a production process in producing products that meet the required quality standards.
- Reducing downtime can also help improve product quality and customer satisfaction. By minimizing equipment downtime, a company can ensure that products are manufactured to high quality standards and delivered to customers on time.
Related Articles
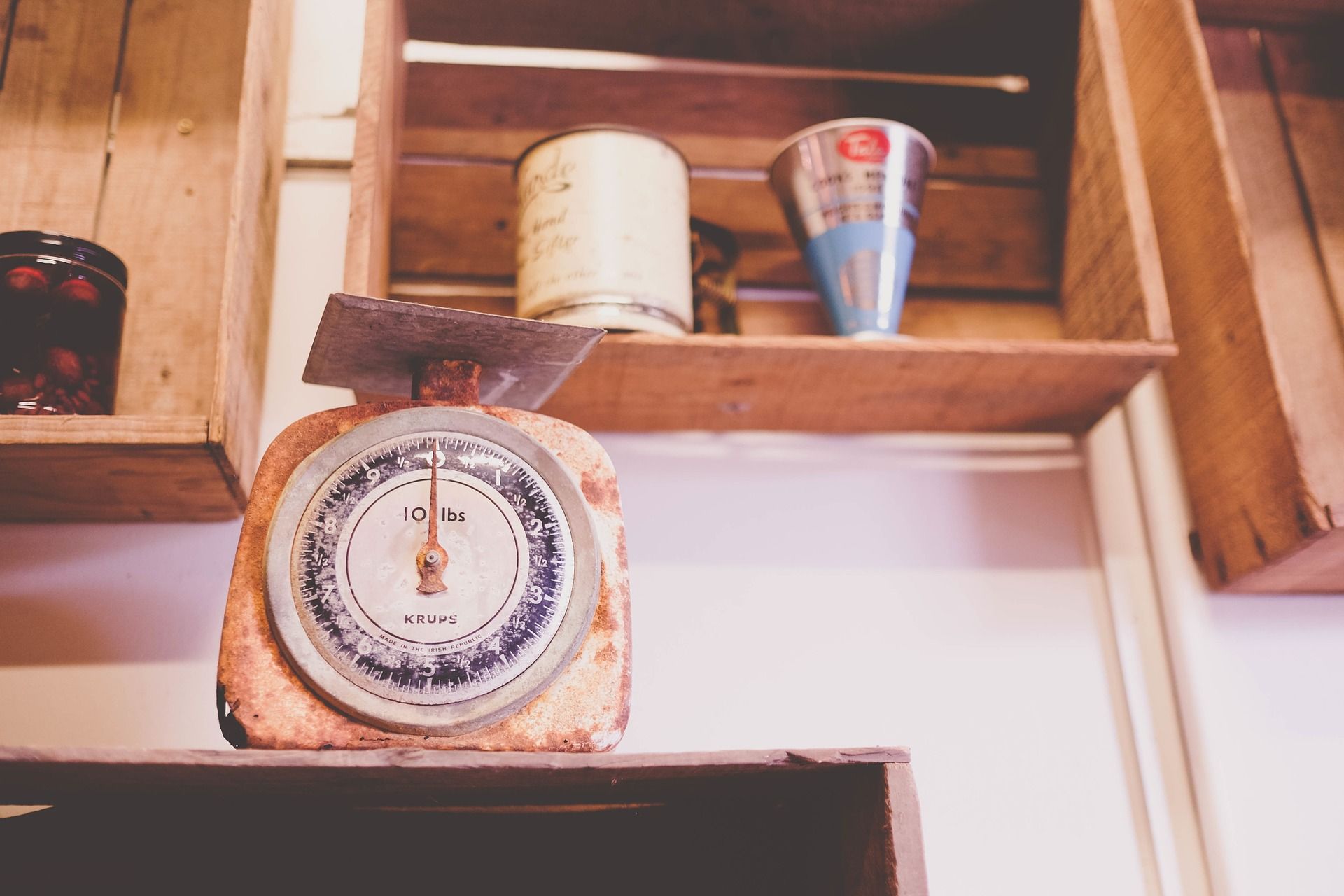
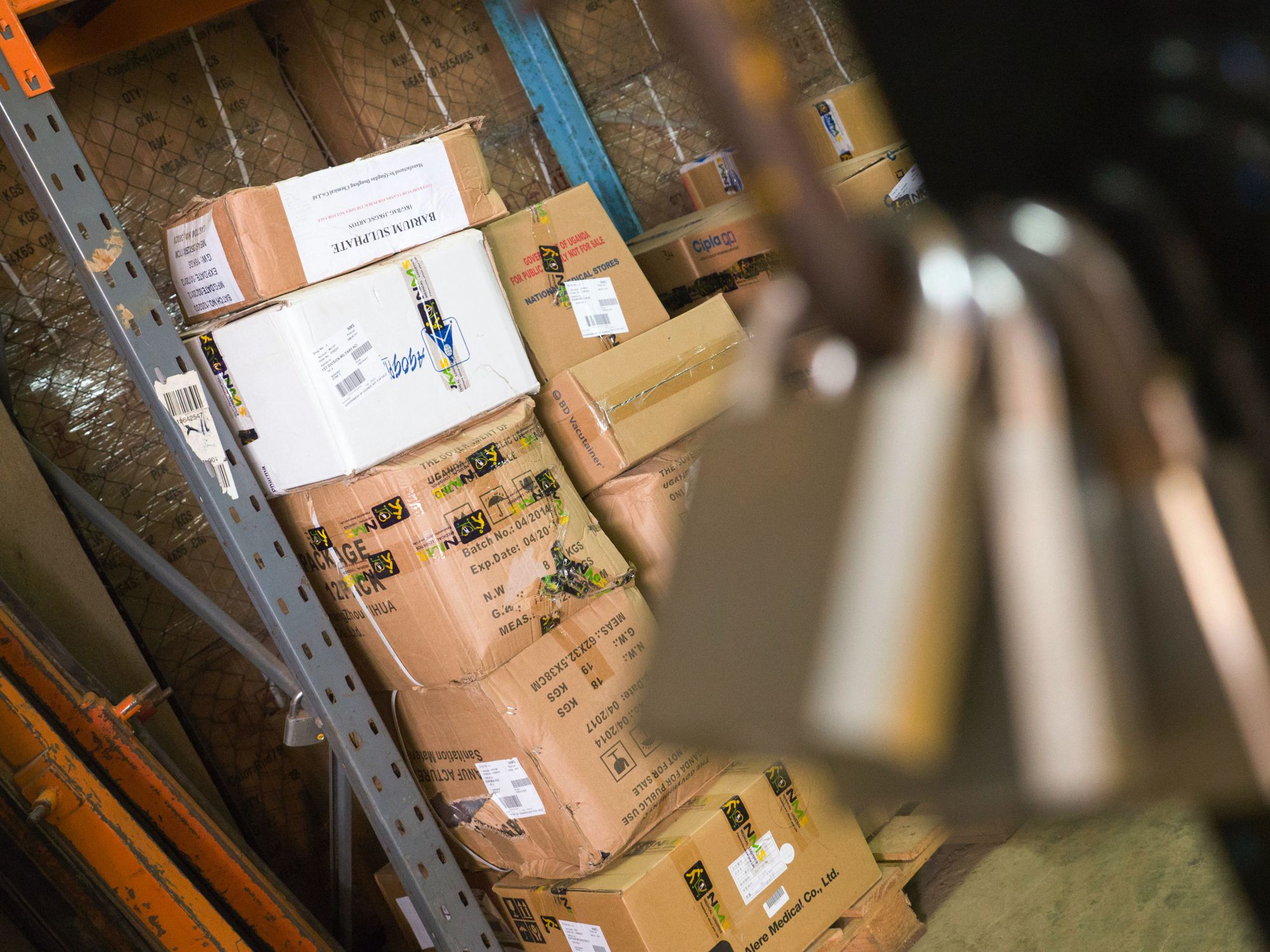
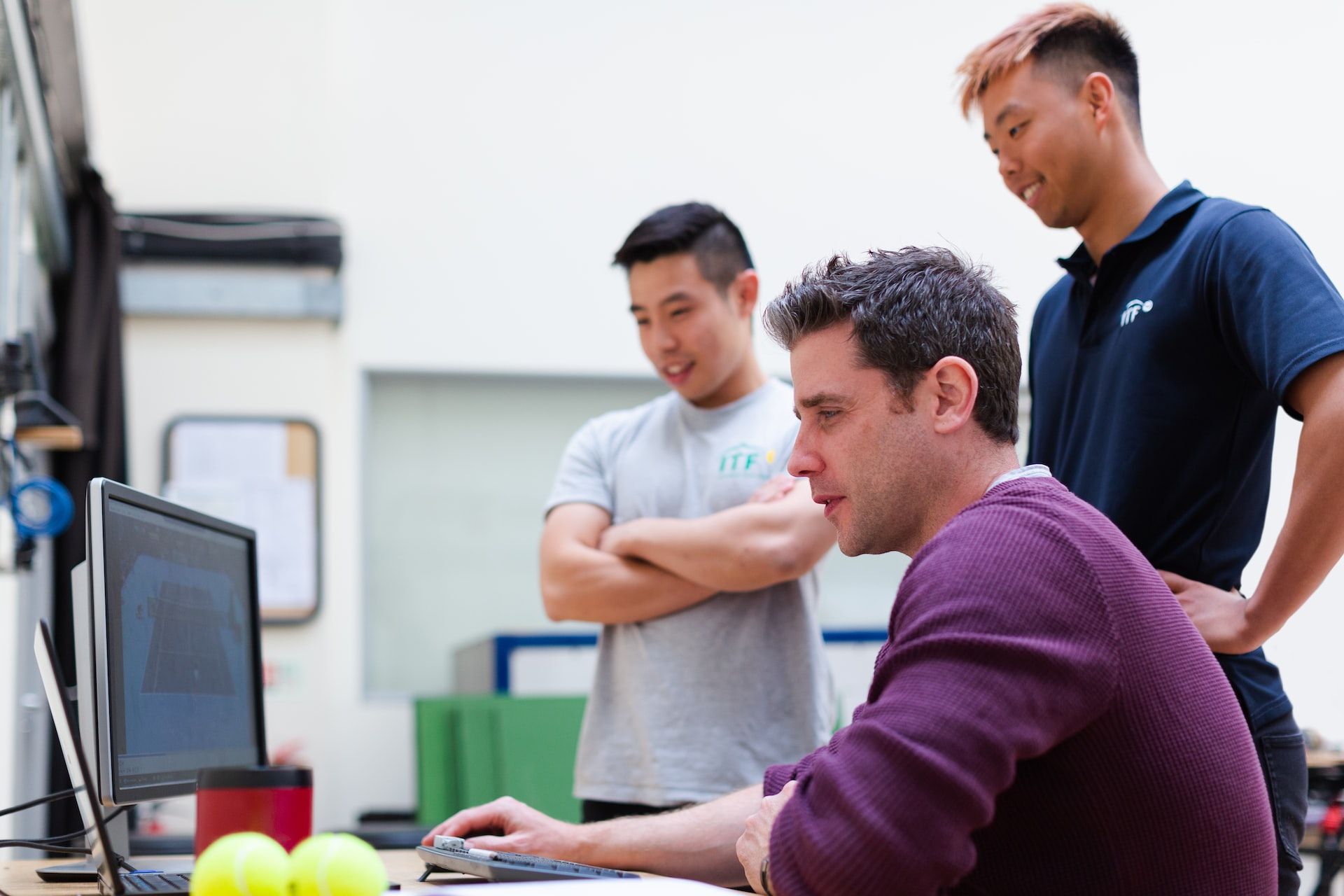
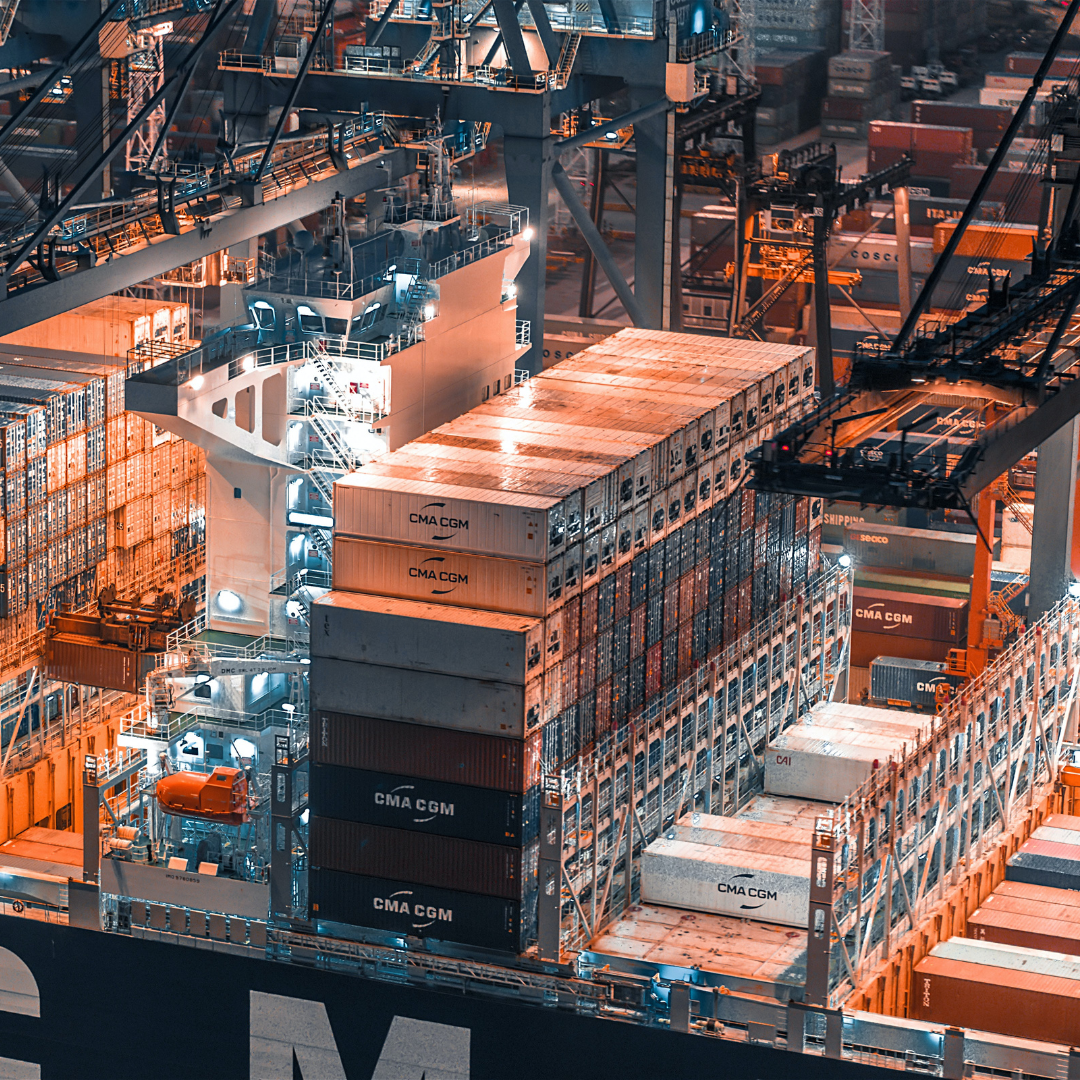
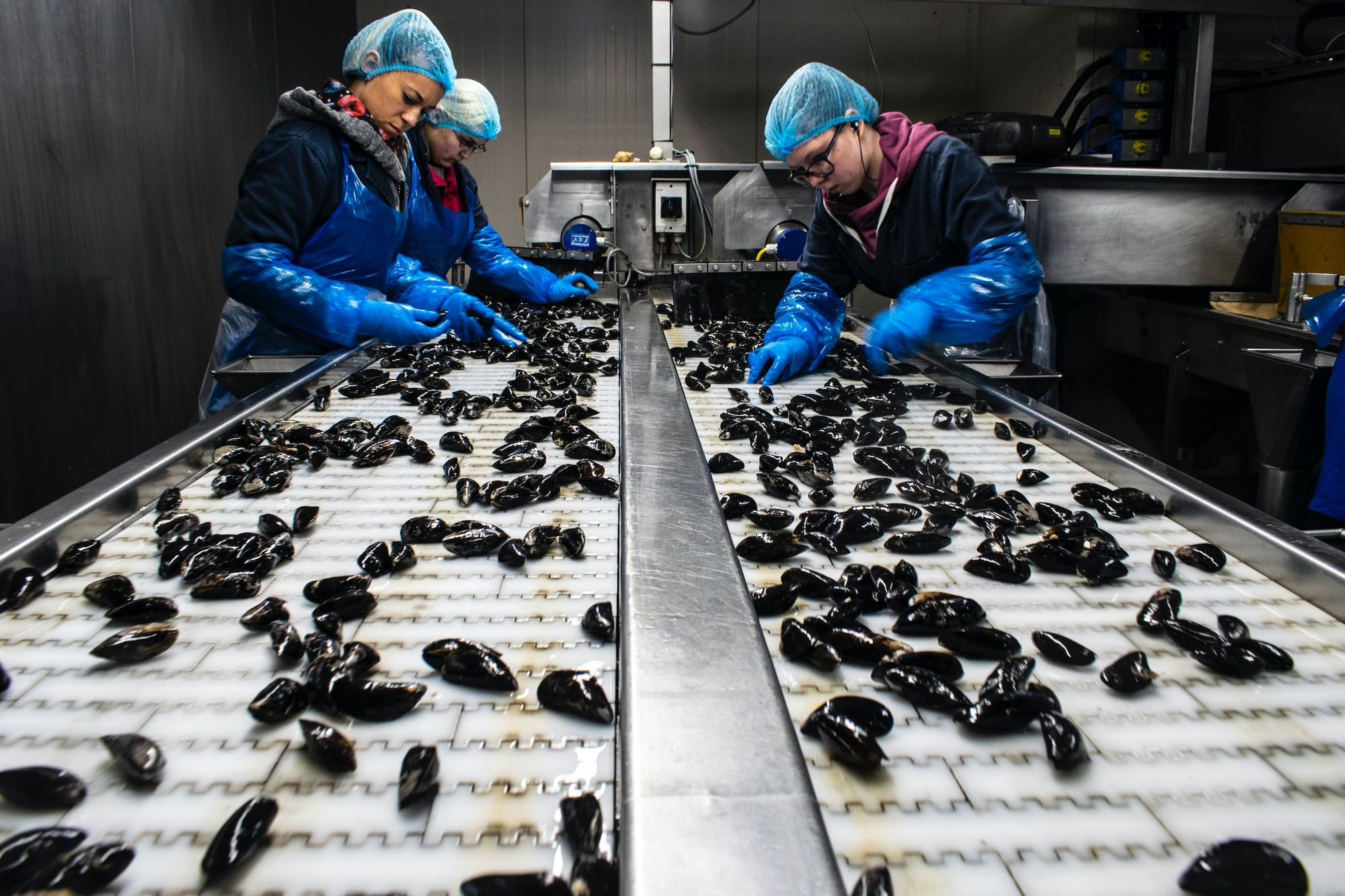