Data is the backbone of modern businesses, driving everything from strategic decisions to daily operations. However, when that data is inaccurate, incomplete, or duplicated, it can do more harm than good. This is what’s commonly referred to as bad data—a silent but costly issue that undermines business performance. In today's fast-paced digital economy, ensuring clean and reliable data is no longer optional; it's essential.
The financial consequences of bad data are staggering. Studies show that poor data quality can cost companies between 10–25% of their total revenue. On a more granular level, the cost to fix a single data error can exceed $100, especially when that error impacts customer communications, inventory planning, or compliance. According to a Gartner survey, organizations report an average annual loss of $15 million due to bad data—highlighting just how critical it is to get data management right.
Bad data infiltrates systems through various sources—manual entry errors, system migrations, or poorly integrated platforms—and over time, it builds up into a serious liability. It leads to flawed analytics, misguided strategies, and operational inefficiencies that can erode customer trust and damage your brand’s reputation. Identifying and eliminating bad data early can save businesses time, money, and long-term setbacks.
This is where powerful, integrated platforms like Deskera ERP come into play. Deskera helps businesses maintain high-quality data through automated validation, real-time syncing across departments, and intelligent reporting. With built-in tools for inventory, sales, finance, and CRM, Deskera ensures your data stays consistent, accurate, and actionable—supporting better decisions and sustainable growth.
What Is Bad Data? Common Types and Real-World Examples
Bad data refers to any data that is inaccurate, incomplete, duplicated, outdated, inconsistent, or irrelevant—resulting in a negative impact on your business operations, decision-making, and analytics.
Whether it's a simple typo during data entry or a critical mismatch in business logic during processing, bad data infiltrates systems through multiple entry points. Over time, it degrades the quality of your data assets and the trust you place in them.
There are many reasons why data can be rejected or flagged as bad: missing references, validation failures, incorrect formats, or even logic violations within a pipeline.
When bad data isn’t addressed, it disrupts downstream processes like customer communications, deliveries, payments, or reporting. Worse, it can create systemic breakdowns that leave behind inconsistencies and require expensive fixes.
Common Types of Bad Data
Understanding the different types of bad data is key to identifying and correcting them:
- Inaccurate Data – Incorrect values due to entry errors, misreporting, or faulty sources.
- Outdated Data – Data that no longer reflects the current state, especially in fast-moving industries.
- Incomplete Data – Missing fields or partial records, like customer profiles without phone numbers.
- Duplicate Data – Repeated records that skew analysis and cause inefficiencies.
- Inconsistent Data – Mismatched formats (e.g., "NY" vs. "New York") across departments or systems.
- Irrelevant Data – Unnecessary data that clutters systems and complicates analytics.
- Unstructured Data – Difficult-to-analyze free-form text or multimedia files not properly categorized.
- Non-compliant Data – Data that violates regulations (e.g., GDPR, HIPAA), risking fines and reputational harm.
Real-World Examples of Costly Bad Data
1. Uber’s $45 Million Commission Miscalculation
From 2014 to 2017, Uber miscalculated its commission in New York by taking its cut based on gross fares instead of net fares, violating its own policies. As a result, thousands of drivers were underpaid. Uber had to reimburse affected drivers with interest—costing the company at least $45 million, not including the reputational damage it caused during a period of heightened scrutiny.
2. Samsung Securities’ $105 Billion Typo
In 2018, a data entry error at Samsung Securities led to the accidental issuance of 2.8 billion shares—worth roughly $105 billion—due to a fat-finger mistake that confused “shares” with “won.” Although the error was caught within 37 minutes, the damage was immediate: stock prices dropped nearly 12%, and the company lost trust among major clients, faced regulatory penalties, and even lost its co-CEO.
These examples demonstrate that bad data is more than just an inconvenience—it’s a business risk. Whether due to human error, outdated systems, or weak validation processes, poor-quality data can lead to financial losses, operational failures, and reputational damage. Businesses that proactively monitor and clean their data are better positioned to avoid such costly outcomes.
Why Bad Data Is a Silent Killer for Business Operations
At first glance, bad data might seem like a small technical nuisance—something that a quick fix or patch can solve. But beneath the surface, it slowly erodes the foundations of decision-making, operational efficiency, and customer trust. Often going unnoticed until significant damage is done, bad data acts as a silent killer for modern businesses.
1. Misguided Decision-Making
Business decisions are only as good as the data they’re based on. When sales forecasts, inventory counts, customer records, or financial metrics are skewed by inaccurate or incomplete data, the outcomes can be disastrous.
Whether it's overstocking products due to inflated demand data or launching a campaign based on outdated customer behavior, bad data leads to wrong turns that are costly to recover from.
2. Operational Inefficiencies
Bad data disrupts workflows at every level. In manufacturing and supply chains, for instance, a wrong part number or missing inventory update can delay production and delivery timelines.
In customer service, incorrect contact information can result in failed communications and frustrated clients. These inefficiencies compound over time, draining resources, productivity, and profitability.
3. Increased Costs
According to industry estimates, bad data can cost businesses up to 25% of their annual revenue. Each bad record costs time and money to correct—more than $100 per data error, on average.
Additionally, when poor-quality data triggers compliance violations, service failures, or product recalls, the financial impact grows exponentially, including penalties, refunds, and lost revenue.
4. Erosion of Customer Trust
Customers today expect personalized, accurate, and timely interactions. If a customer receives multiple promotional emails because of duplicate entries, or if their name is consistently misspelled, it degrades brand perception.
Worse, incorrect billing, shipping errors, or breach of data privacy due to bad data can lead to customer churn and long-term reputational harm.
5. Failure to Scale
As businesses scale, the volume and complexity of data grow rapidly. Without a robust data governance framework, errors multiply and propagate across interconnected systems.
A poor data foundation prevents automation, limits analytics capabilities, and makes it difficult to integrate advanced technologies like AI or machine learning.
How to Detect Bad Data in Your System: Key Warning Signs
Identifying bad data early is critical to maintaining data integrity and ensuring seamless operations. However, because bad data can be subtle and widespread, it’s not always easy to spot.
Here are some of the most common warning signs that signal bad data is lurking in your system:
1. Frequent Data Entry Errors and Misspellings
One of the clearest signs of bad data is the presence of typos, formatting inconsistencies, and misspelled entries. These often occur during manual data input and can cause major issues in database queries, customer communications, and automated workflows.
Example: Multiple versions of a customer name like “Jon Smith,” “John Smith,” and “J. Smith” can result in fragmented records and skewed analytics.
2. Duplicate Records Across Systems
Redundancy in data is not only inefficient—it’s risky. Duplicate records can lead to double billing, repeated marketing emails, and confused reporting. This is common when integration between platforms is weak or when systems fail to validate entries before submission.
Red Flag: Identical entries showing up across CRM, inventory, or accounting systems.
3. Incomplete or Missing Fields
If critical data fields are often left blank or filled with placeholders like “N/A” or “Unknown,” you’re dealing with incomplete data. This can hinder everything from reporting accuracy to regulatory compliance.
Impact: Missing contact details or SKU numbers can delay order fulfillment or customer service responses.
4. Inconsistent Formats and Units
When different teams or systems use varying formats for dates, currencies, units of measure, or product codes, the result is confusion and errors. This inconsistency is a key symptom of a lack of standardized data practices.
Scenario: One team uses “MM/DD/YYYY” while another uses “DD-MM-YYYY” for date inputs, resulting in sorting and calculation errors.
5. Discrepancies Between Systems
If your sales dashboard reports 1,500 units sold but your inventory system only shows 1,200 units deducted, that’s a red flag. These misalignments often point to data sync failures or broken integrations.
Indicator: Conflicting values in reports generated from different platforms.
6. Outdated or Irrelevant Entries
Old data that hasn’t been updated or records that no longer serve a purpose add clutter and noise. Worse, relying on outdated data can lead to wrong decisions and missed opportunities.
Example: Marketing campaigns sent to inactive or unsubscribed users based on outdated email lists.
7. Repeated System or Process Failures
Bad data can cause processes to break, trigger errors during automation, or halt workflows entirely. If systems frequently reject entries or crash during execution, it may be time to investigate data quality.
Clue: Frequent error logs, failed transactions, or stalled ETL processes.
Best Practices to Eliminate Bad Data from Your Databases
Maintaining clean, accurate data is essential for making informed business decisions and optimizing operations. Bad data can lead to costly mistakes, inefficiencies, and lost opportunities.
Implementing effective best practices ensures your databases remain reliable, trustworthy, and valuable assets to your organization.
1. Assess the Scope of the Issue
Before addressing bad data, it is crucial to understand its extent and origin. Conducting a thorough data audit helps identify primary sources of errors, their frequency, and the areas most affected.
This assessment provides a clear picture of the data quality challenges your organization faces and guides the prioritization of remediation efforts.
Tailoring your data management approach based on these insights ensures more effective and efficient solutions. Without a clear understanding of the problem’s scope, attempts to clean or improve data quality may be misguided or incomplete.
2. Implement Validation Checks
Validation checks enforce rules to ensure data entered into your systems meets predefined criteria, reducing the entry of incorrect or illogical data.
These checks can be configured to validate formats (e.g., “YYYY-MM-DD” for dates), value ranges, and mandatory fields. Implementing validation at the point of data entry helps prevent errors before they enter your databases.
This proactive approach reduces downstream cleansing efforts and improves overall data integrity. Regularly updating validation rules ensures they remain relevant as business needs evolve.
3. Automate Data Cleansing
Manual data cleansing is time-consuming and prone to errors; automating this process increases efficiency and accuracy. Data cleansing tools detect and correct duplicates, misspellings, inconsistencies, and other common errors automatically.
Automating these processes during data ingestion or at regular intervals maintains data quality over time. It also frees up resources to focus on more complex issues requiring human judgment. Integration of these tools with your data management systems ensures continuous monitoring and improvement without disrupting daily operations.
4. Train Your Team
Human error is a leading cause of bad data. Providing regular training for staff involved in data entry and management reduces mistakes and improves data quality awareness. Training should cover best practices for accurate data entry, the importance of data validation, and how errors impact business decisions.
Educated employees are more likely to follow standardized procedures and recognize data quality issues early. A culture that values data accuracy fosters proactive error prevention and ongoing improvement in data management.
5. Standardize Data Entry Procedures
Inconsistent data entry leads to variation and confusion. Establishing standardized procedures creates uniformity and reduces errors. Develop detailed data entry manuals or guidelines specifying acceptable formats, naming conventions, and data field requirements.
Implement tools like dropdown menus, pre-populated fields, and input masks to enforce consistency. Standardization improves data comparability and facilitates easier integration and analysis. Regular reviews and updates to these procedures keep them aligned with evolving business requirements.
6. Monitor Data Quality Continuously
Data quality can degrade over time if left unchecked. Establishing continuous monitoring enables early detection of issues before they impact business operations. Set up dashboards and reporting tools to track key data quality metrics such as completeness, accuracy, and consistency.
Regular assessments help identify emerging problems, unusual patterns, or recurring errors. Combining automated alerts with periodic manual reviews creates a robust quality control framework that maintains trust in your data.
7. Backup and Archive Data
Regularly backing up your data protects against accidental loss during cleansing or system failures. Having reliable backups allows you to restore data to a previous state, minimizing downtime and data corruption risks.
Archiving older, less frequently used data helps maintain database performance while preserving historical records for compliance or analysis.
A disciplined backup and archiving strategy ensures data availability and integrity throughout its lifecycle, supporting both operational needs and long-term strategic goals.
8. Collaborate with Data Providers
When using external data, clear communication of quality standards is essential. Engage regularly with data providers to review data quality, share feedback, and resolve issues collaboratively. Establish contracts or service-level agreements that specify acceptable data quality levels and remediation processes.
Building strong partnerships helps ensure data meets your organization’s accuracy and consistency requirements. Collaboration also facilitates proactive problem-solving and continuous improvement of shared data sources.
9. Review and Refine Data Management Practices
Data management is an ongoing process that requires regular evaluation and adaptation. Periodically review your data quality policies, tools, and procedures to identify gaps or areas for improvement. As business needs, technologies, and data sources evolve, refine your strategies to stay effective.
Continuous refinement ensures your data quality framework remains relevant and capable of addressing new challenges. Foster a culture of continuous learning and improvement to maintain high standards of data integrity.
10. Invest in Advanced Technology and Tools
Modern data management platforms offer sophisticated features to detect, correct, and prevent bad data. Investing in advanced tools such as data observability platforms, machine learning-based anomaly detection, and automated cleansing solutions enhances your data quality efforts.
These technologies provide real-time monitoring, detailed analytics, and actionable alerts that enable swift corrective actions. Allocating budget and resources for ongoing tool upgrades and training maximizes the return on investment and ensures your data management capabilities keep pace with growing business demands.
How to Prevent Bad Data from Entering Your System
Preventing bad data from entering your system is the first critical step toward maintaining data integrity and reliability. Without a solid foundation of clean data, even the best analytics and business processes can yield flawed results.
Below are essential best practices that organizations should adopt to minimize errors and ensure high-quality data capture from the start.
1. Establish a Standardized Data Collection Process
Creating clear protocols for data gathering and entry helps reduce inconsistencies and errors. Define required fields, acceptable value ranges, and data-entry procedures to guide users. Automated validation tools can then flag data that doesn’t meet these criteria, such as incorrect date formats or invalid values.
Using drop-down menus instead of free-text fields further reduces manual errors and ensures uniform formatting across teams. Standardization promotes consistency, enabling smoother data integration and more accurate analysis.
2. Conduct Regular Data Audits
Routine audits across all systems ensure data remains accurate, complete, and consistent. Scheduled data-quality reviews help identify anomalies, inconsistencies, and emerging issues before they cause major problems.
Many modern data platforms provide automated tools that flag outliers or unusual patterns. For regulated industries, such as healthcare under HIPAA, these audits also support compliance efforts. Regular audits not only catch errors but also reinforce a culture of data responsibility and continuous improvement.
3. Maintain Thorough Data Documentation
Comprehensive records of data sources, definitions, relationships, and usage guidelines are crucial for transparency and consistency. Keeping this documentation up to date and easily accessible supports onboarding, troubleshooting, and cross-team collaboration.
Well-documented data practices help analysts evaluate the effectiveness of current processes and recommend improvements. It also ensures that everyone understands the meaning and context of data, reducing misinterpretations and misuse.
4. Understand Your Data Sources and Instrumentation
A deep understanding of each data source’s characteristics, limitations, and update schedules is vital. Knowing calibration needs, potential failure points, and sensor accuracy helps identify and prevent unreliable data inflows.
Continuous monitoring of source performance enables early detection of issues, preventing flawed data from propagating through the system. This proactive oversight ensures data quality is preserved from origin to reporting.
5. Set Validation Standards for New Data Sources
Before integrating new data sources, establish quality criteria and compatibility checks. Onboarding procedures should include testing data migration for formatting, completeness, and error handling capabilities.
Standardized validation ensures new inputs meet your organization’s data standards and do not introduce inconsistencies. This approach avoids surprises that can disrupt workflows and ensures seamless expansion of your data ecosystem.
6. Develop Data Security and Access Control
Protecting data integrity involves implementing strong security measures, including user authentication, access privileges, and modification protocols.
Maintaining access logs and conducting regular system updates prevent unauthorized changes and reduce risks from software bugs or cyberattacks.
Ongoing training helps staff understand security practices, reinforcing vigilance against threats. Robust security safeguards ensure that only authorized users can modify data, preserving its accuracy and trustworthiness.
7. Review Data Backup and Recovery Procedures
Regular backups and tested recovery processes safeguard data against accidental loss or corruption. Multiple backup copies, verified backup integrity, and automated scheduling reduce risks of data unavailability.
Well-documented recovery procedures ensure that data can be restored quickly and accurately in emergencies. These measures provide peace of mind that your critical business data is protected and can be retrieved with minimal disruption.
8. Ensure Stakeholders Understand Data Through Periodic Training
Regular training programs educate staff on proper data handling, quality standards, and the importance of data reliability. Training keeps users updated on evolving standards, potential threats, and common data quality issues.
Role-specific examples make the learning relevant, demonstrating how poor data impacts workflows and decision-making. Empowered with this knowledge, employees are better equipped to prevent errors and contribute to maintaining clean, trustworthy data.
How Deskera ERP Can Help You Prevent Bad Data and Improve Data Quality
Deskera ERP can help you prevent bad data and improve data quality in the following ways:
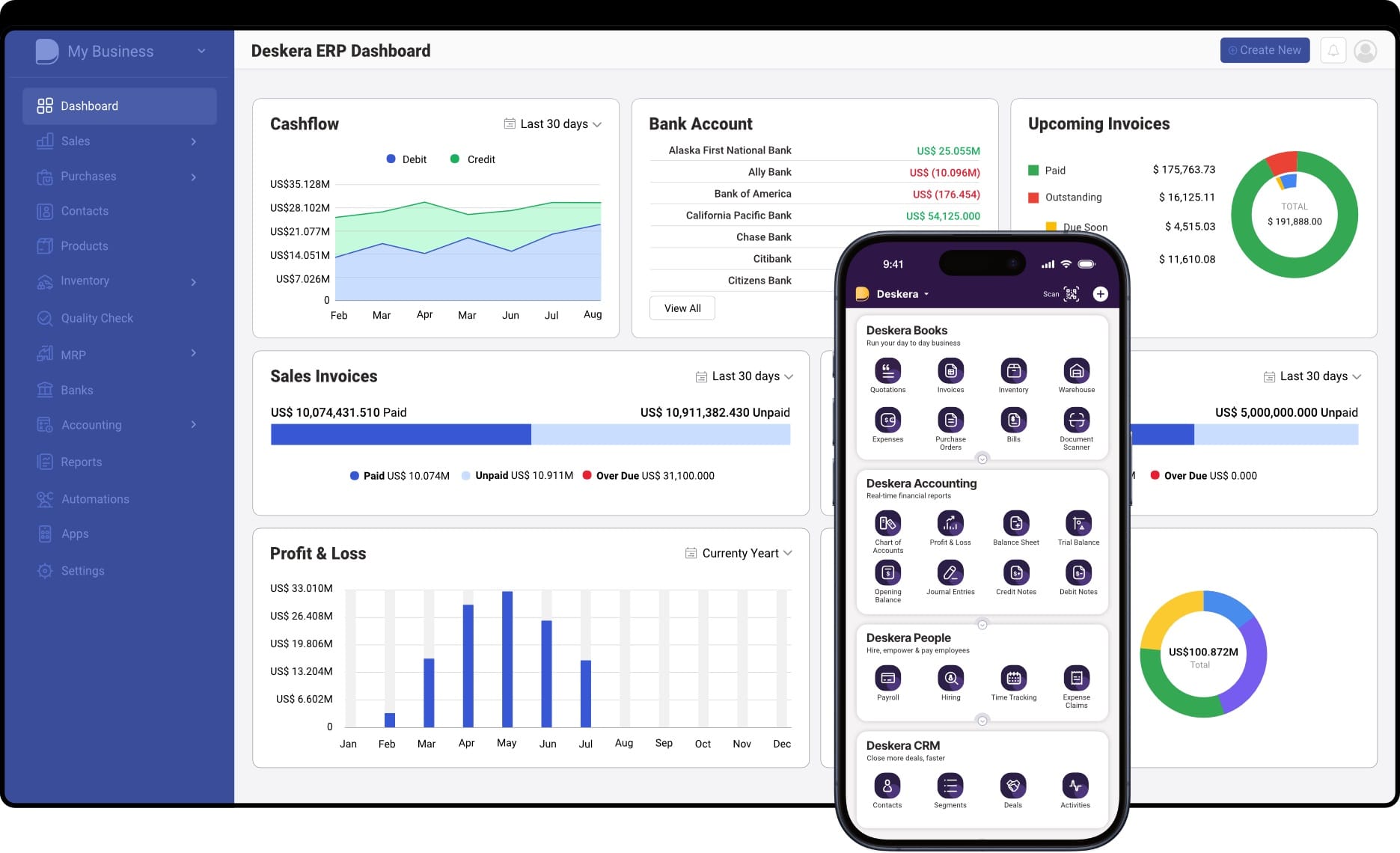
- Automates data entry with standardized templates to reduce manual errors and enforce consistency.
- Provides real-time dashboards and audit trails to continuously monitor data quality and quickly identify anomalies.
- Implements role-based access controls to ensure data security and prevent unauthorized modifications.
- Includes built-in backup and recovery features to protect data integrity in case of issues.
- Offers user-friendly interfaces and ongoing training resources to empower your team to maintain clean, reliable data.
Key Takeaways
- Bad data refers to inaccurate, incomplete, outdated, or duplicated information that negatively impacts business decisions, reporting, and operations.
- Poor-quality data leads to flawed insights, customer dissatisfaction, operational inefficiencies, and financial losses—making it a critical issue for any organization.
- Human errors, siloed systems, lack of standardized input methods, and inconsistent data sources are leading contributors to bad data in business environments.
- Regular audits, validation tools, anomaly detection, and pattern analysis can help uncover inconsistencies, missing values, and outliers in datasets.
- Implementing proactive strategies—like standardized data entry, access controls, training, and automated validation—can help remove existing bad data and prevent its recurrence.
- Establishing strong data governance, maintaining documentation, auditing sources, validating new data inputs, and training users are essential to stop bad data at the source.
- Deskera ERP offers built-in tools for data standardization, monitoring, access control, and backups—making it easier to manage data quality and reduce errors system-wide.
Related Articles
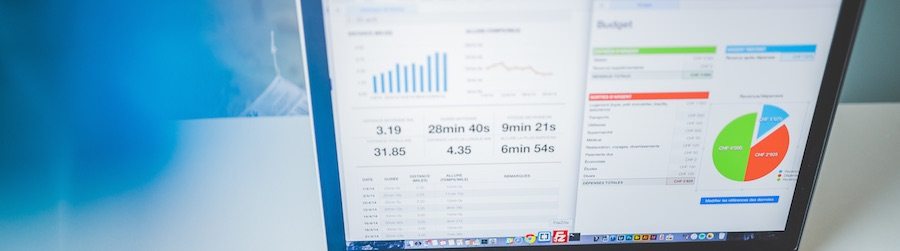
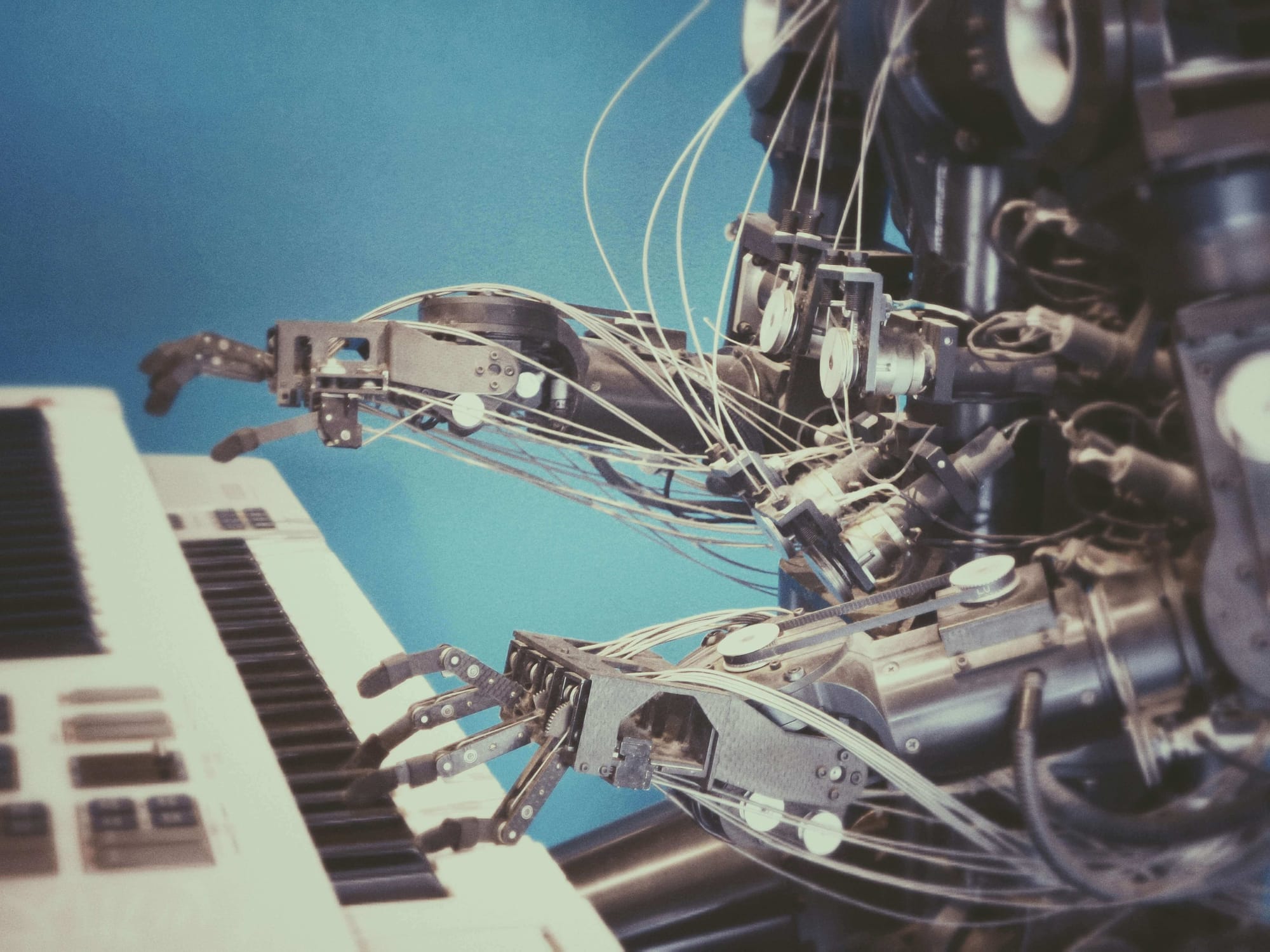
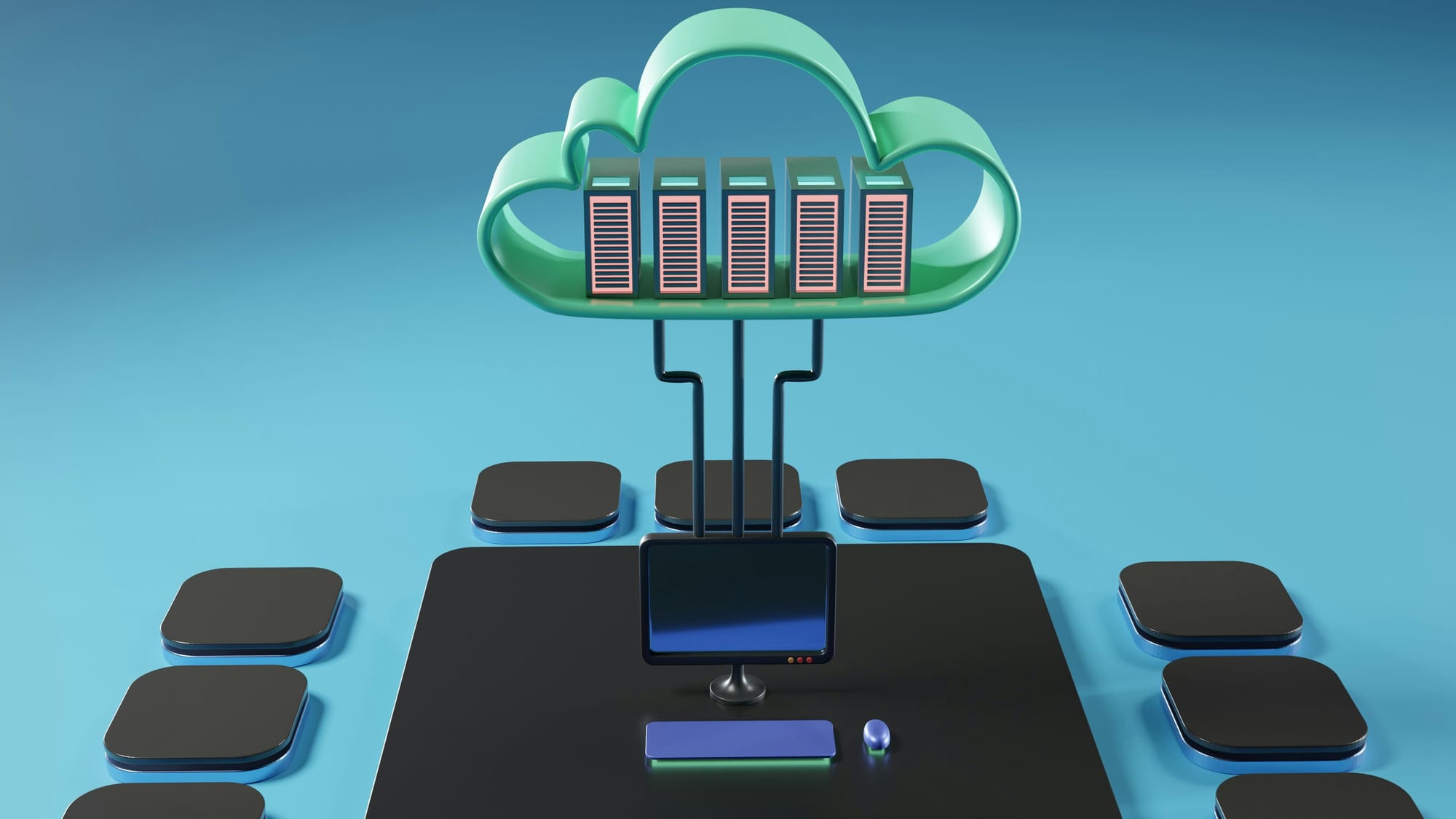
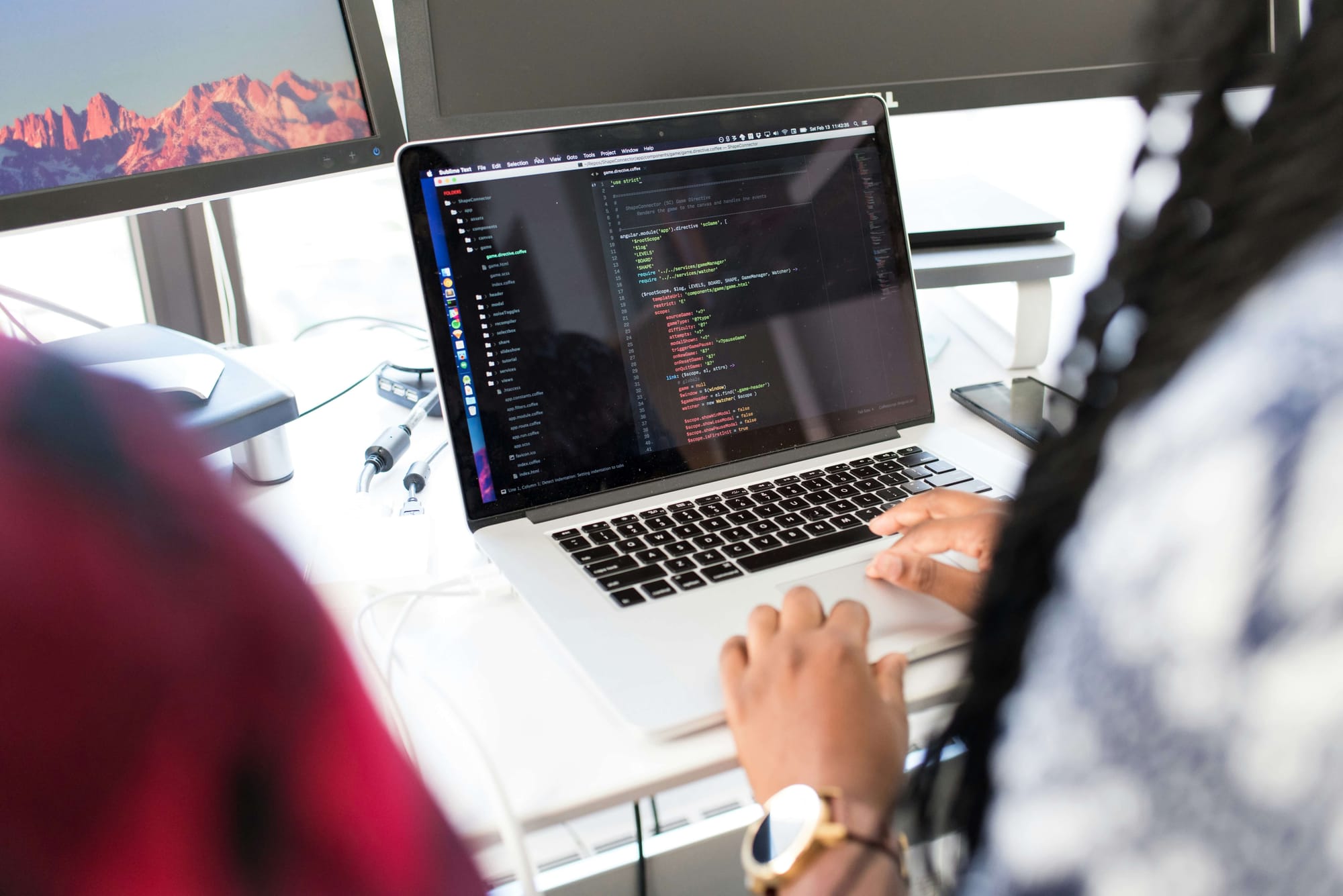
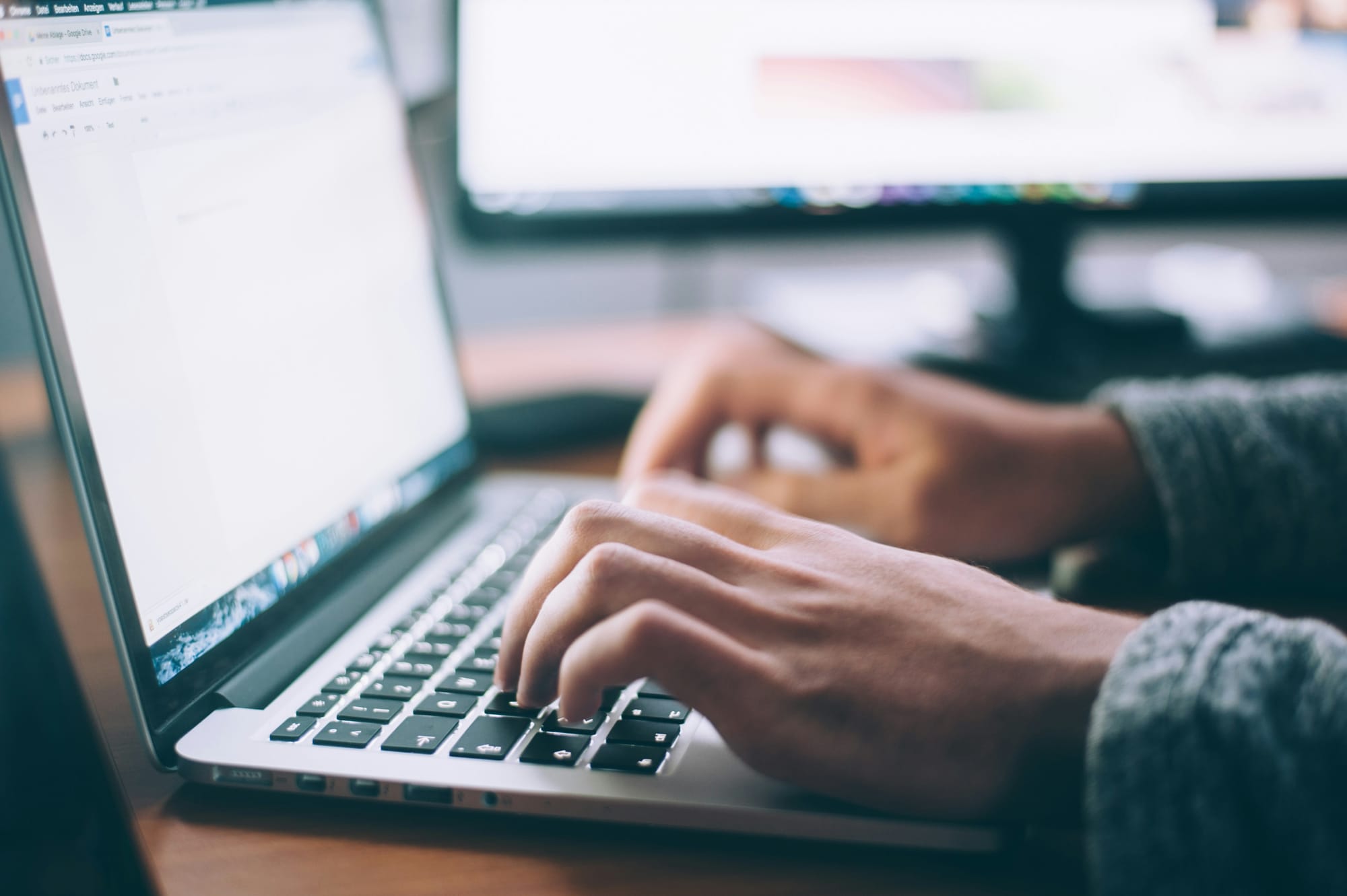
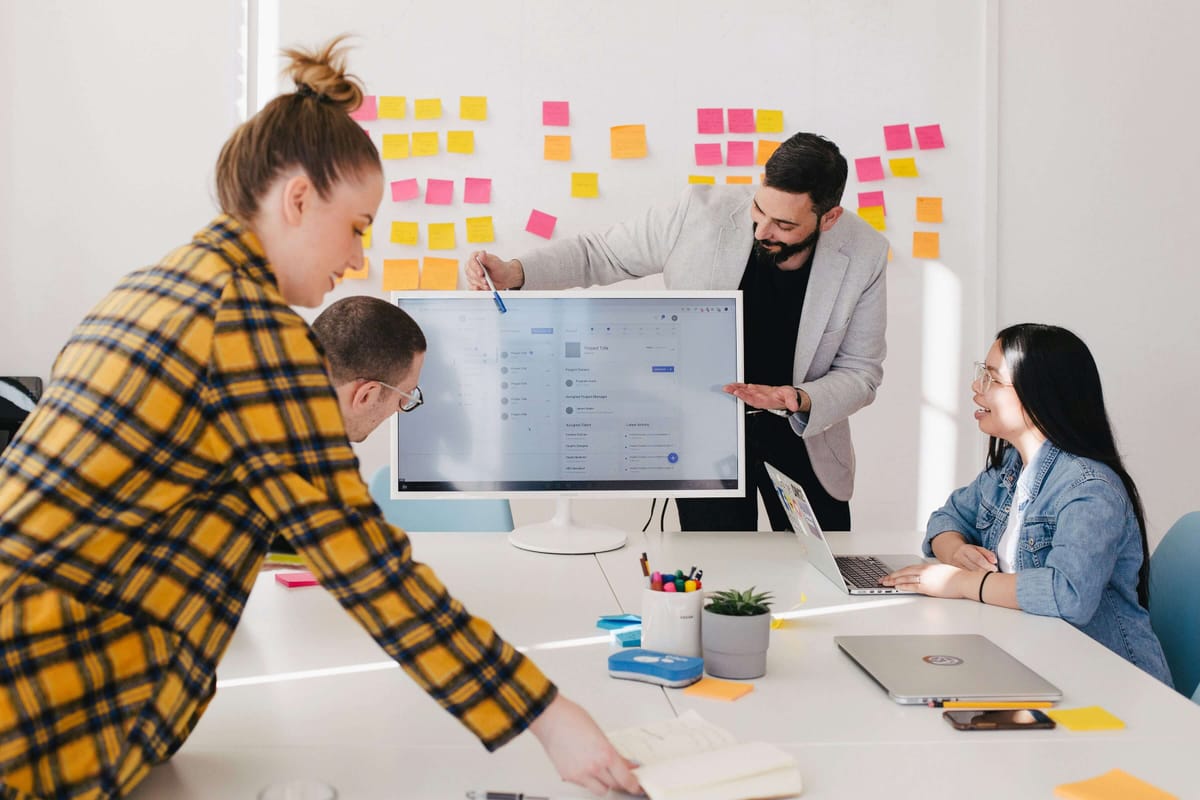